MGTSC 352 Lecture 9 Aggregate Planning Case 2
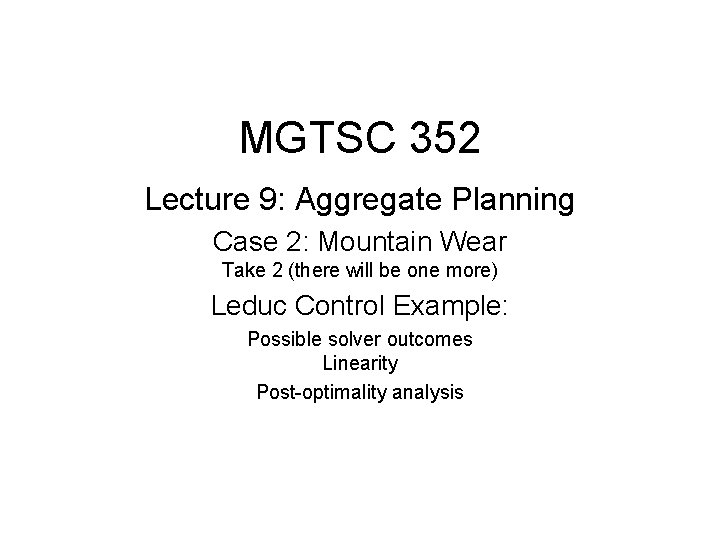
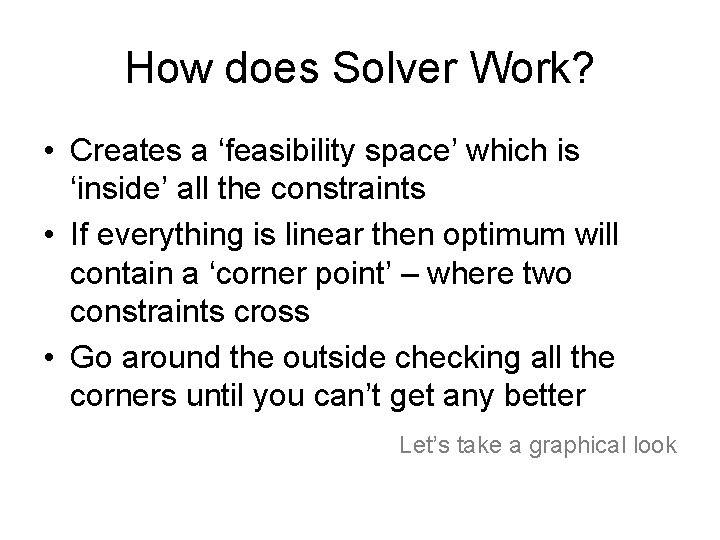
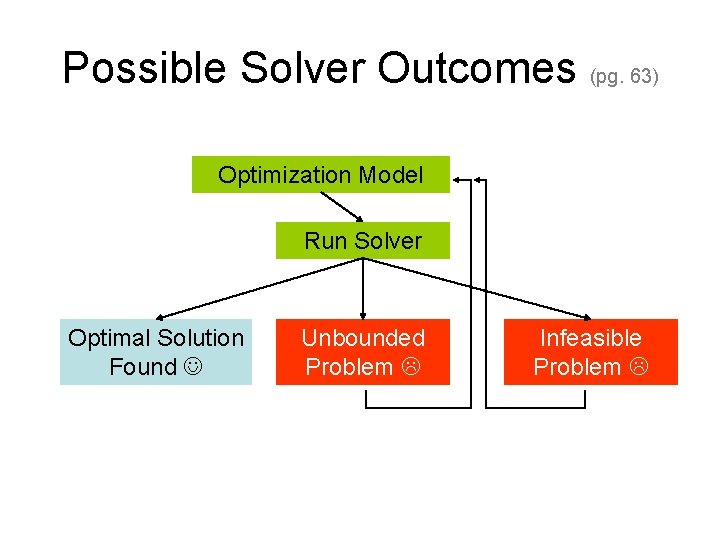
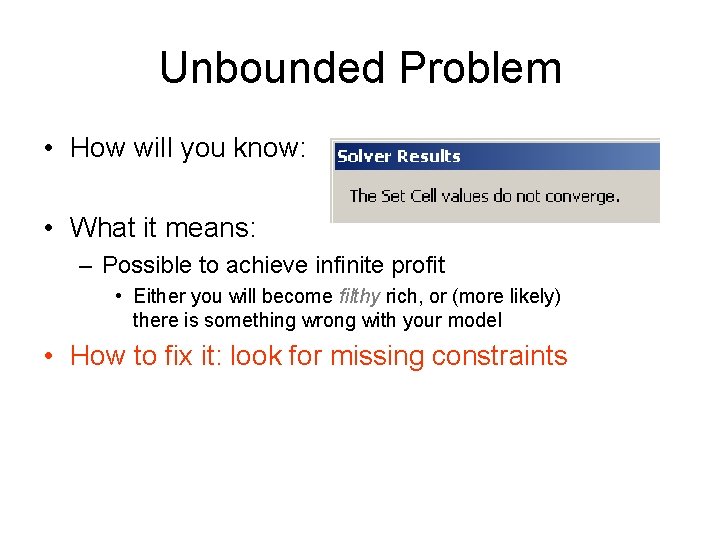
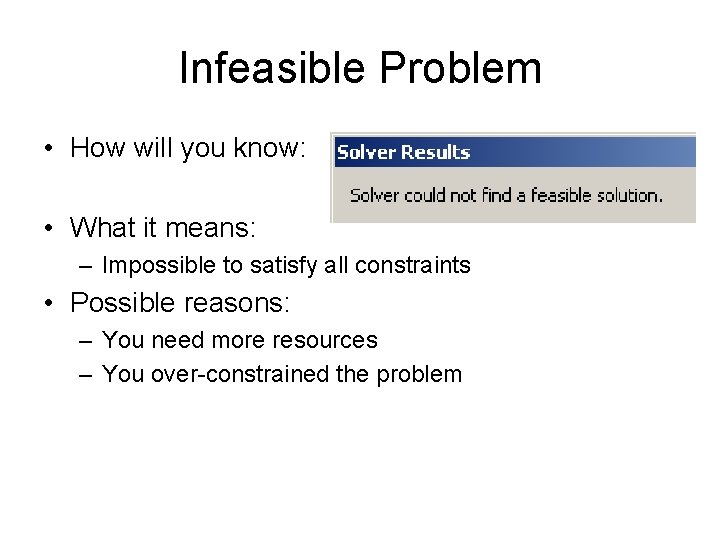
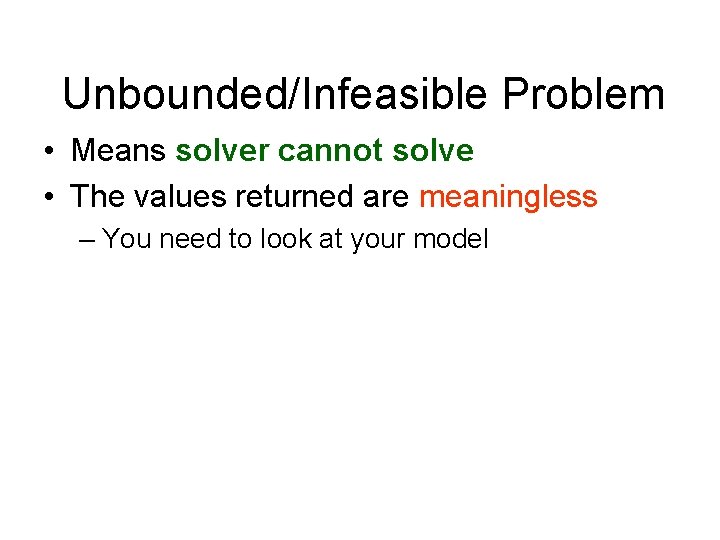
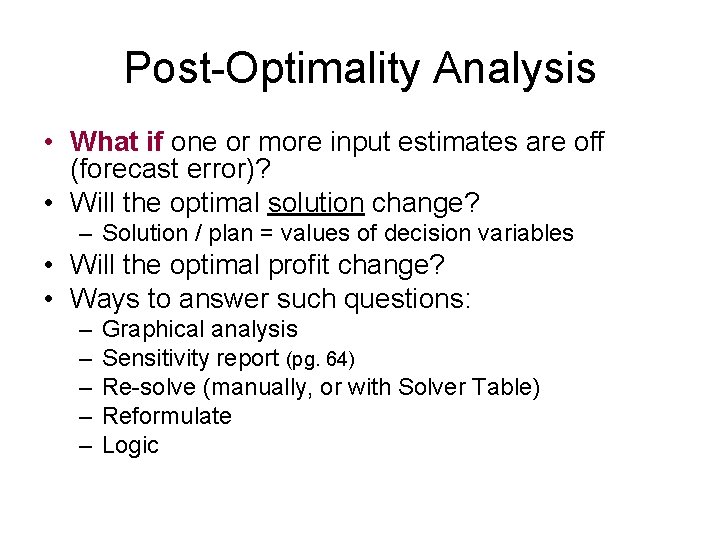
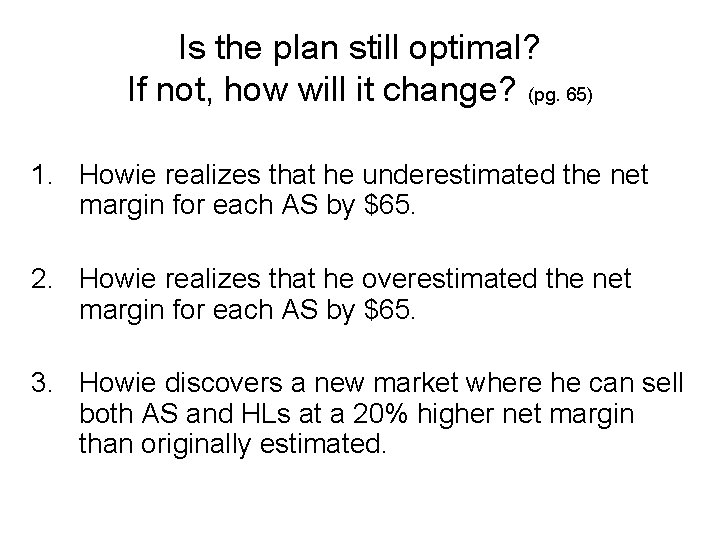
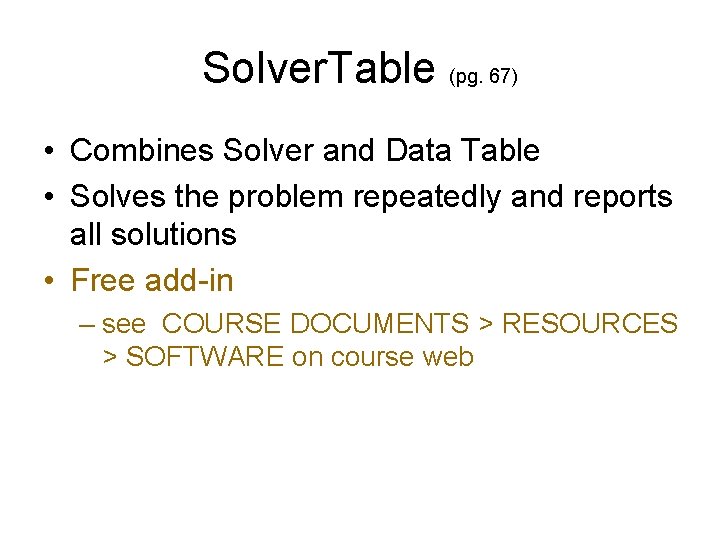
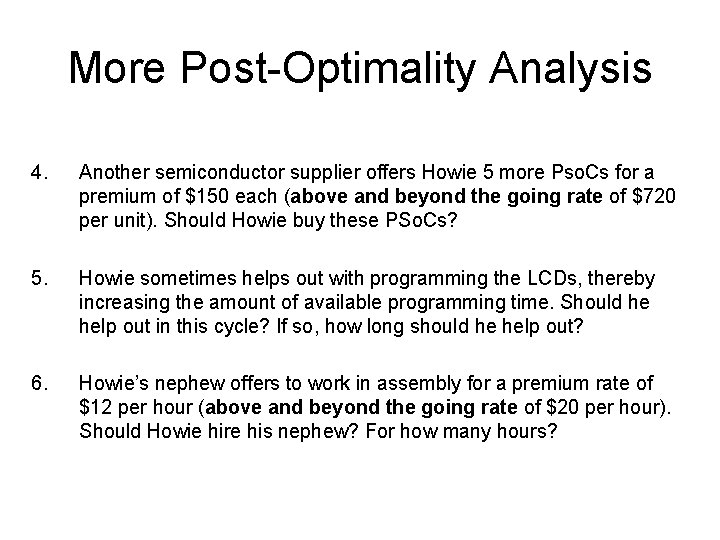
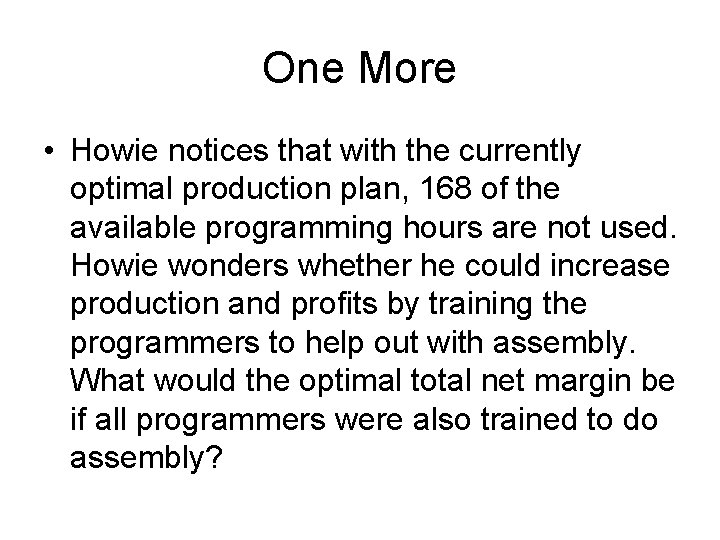
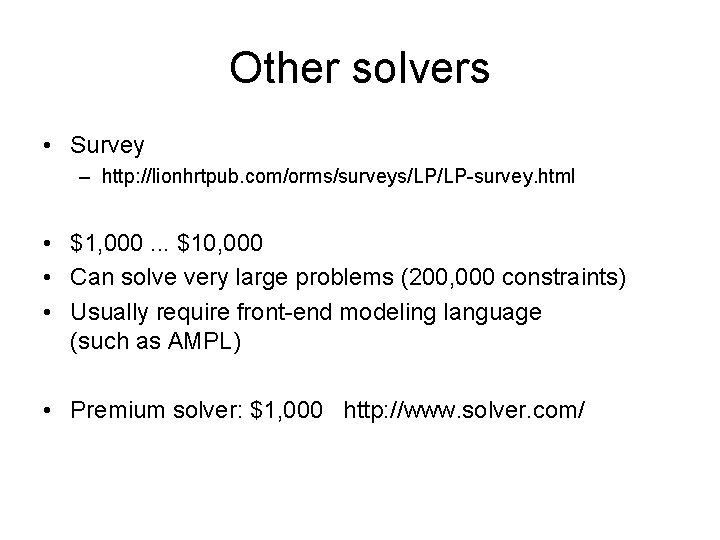
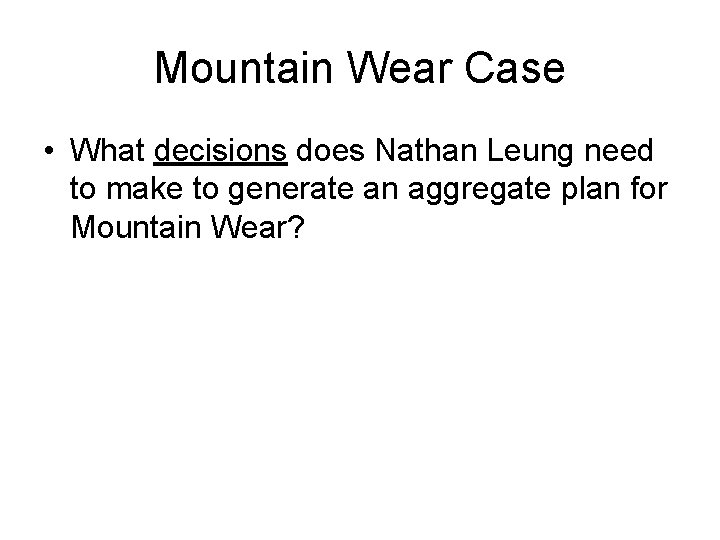
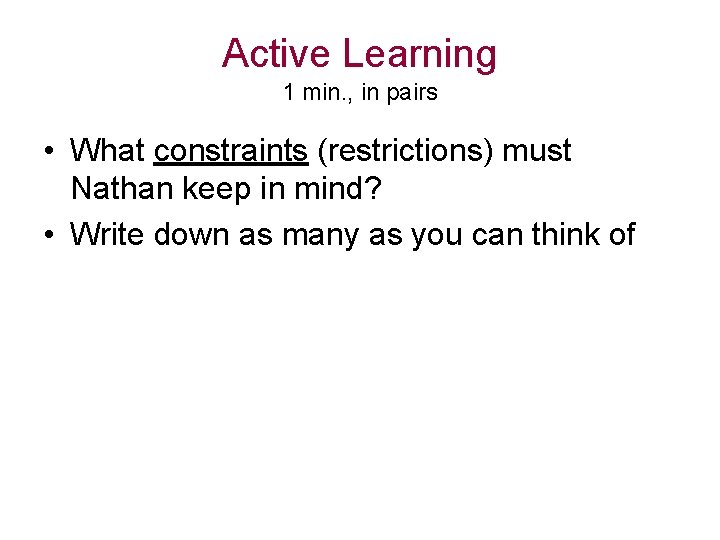
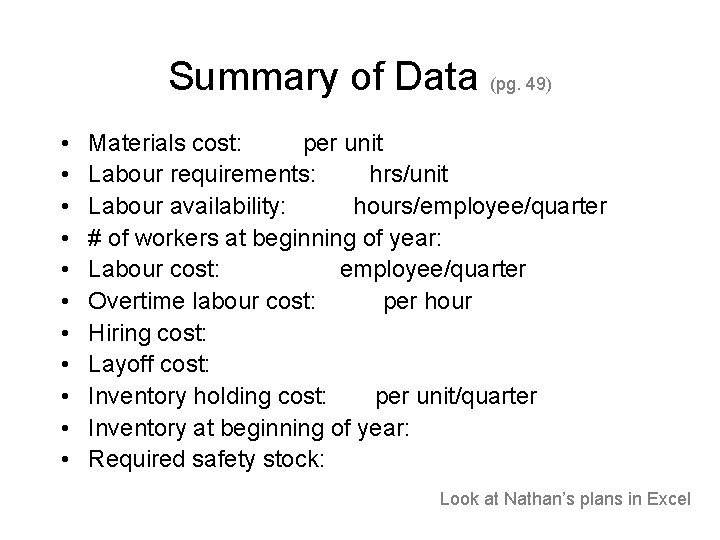
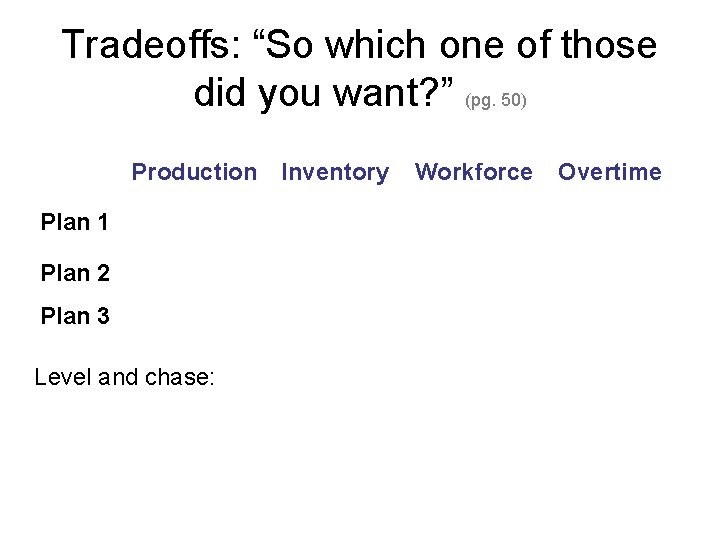
- Slides: 16
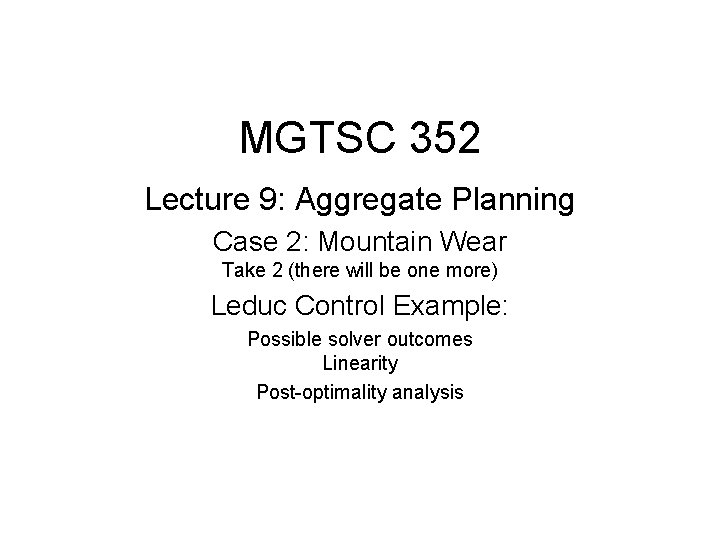
MGTSC 352 Lecture 9: Aggregate Planning Case 2: Mountain Wear Take 2 (there will be one more) Leduc Control Example: Possible solver outcomes Linearity Post-optimality analysis
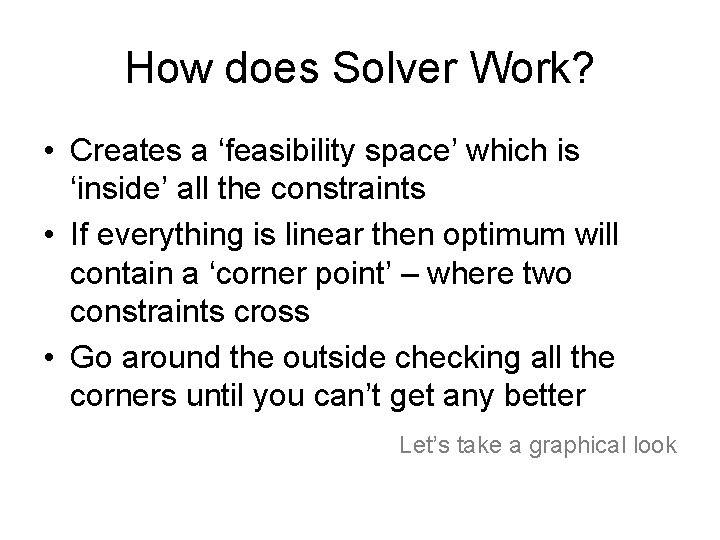
How does Solver Work? • Creates a ‘feasibility space’ which is ‘inside’ all the constraints • If everything is linear then optimum will contain a ‘corner point’ – where two constraints cross • Go around the outside checking all the corners until you can’t get any better Let’s take a graphical look
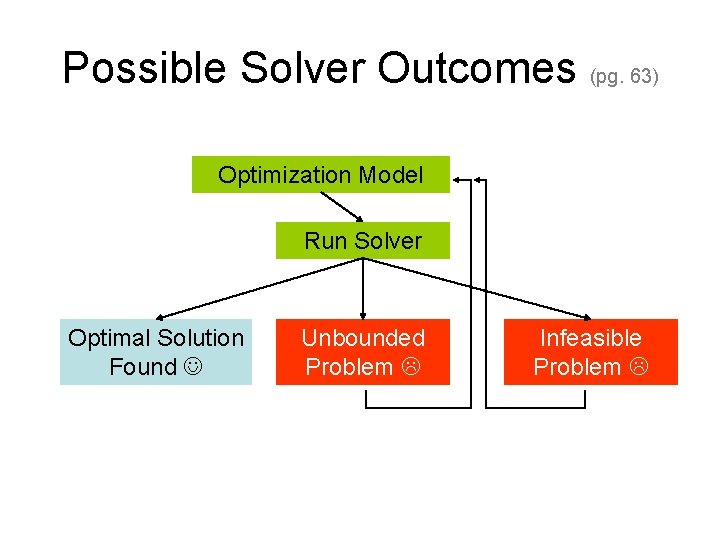
Possible Solver Outcomes (pg. 63) Optimization Model Run Solver Optimal Solution Found Unbounded Problem Infeasible Problem
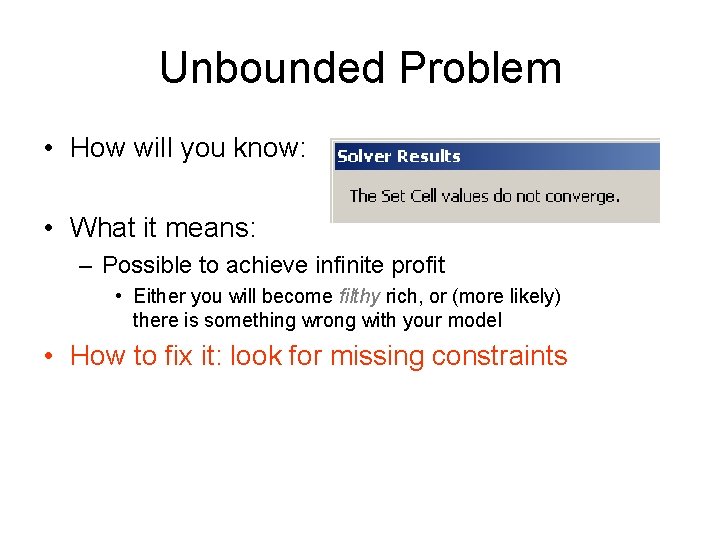
Unbounded Problem • How will you know: • What it means: – Possible to achieve infinite profit • Either you will become filthy rich, or (more likely) there is something wrong with your model • How to fix it: look for missing constraints
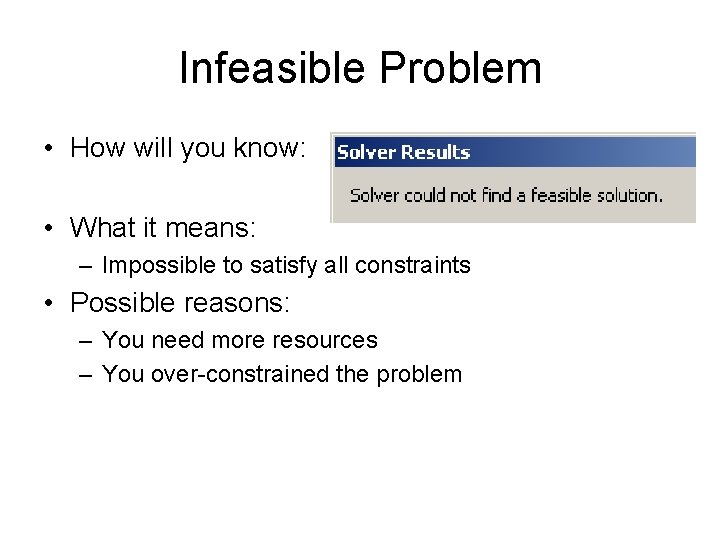
Infeasible Problem • How will you know: • What it means: – Impossible to satisfy all constraints • Possible reasons: – You need more resources – You over-constrained the problem
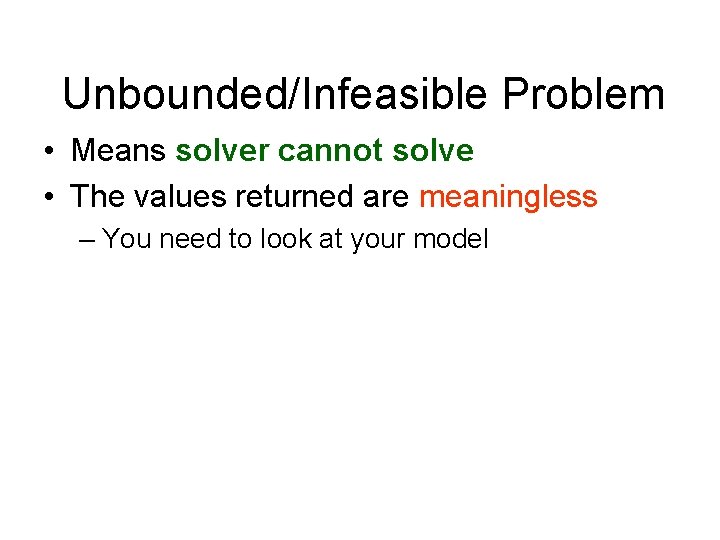
Unbounded/Infeasible Problem • Means solver cannot solve • The values returned are meaningless – You need to look at your model
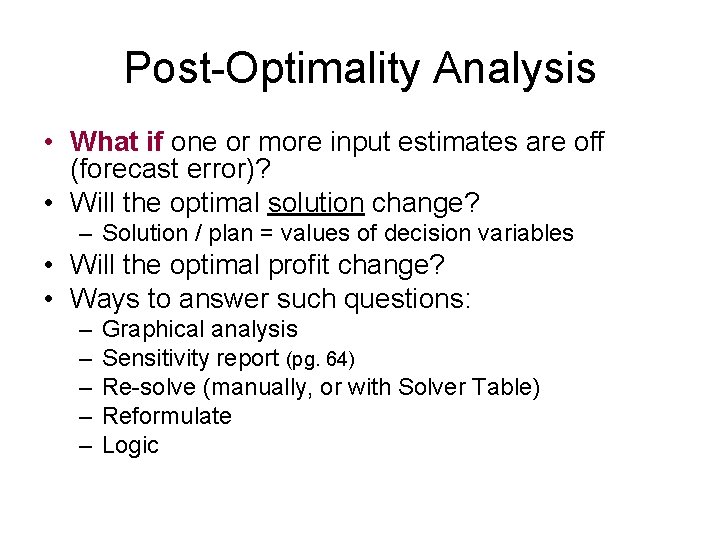
Post-Optimality Analysis • What if one or more input estimates are off (forecast error)? • Will the optimal solution change? – Solution / plan = values of decision variables • Will the optimal profit change? • Ways to answer such questions: – – – Graphical analysis Sensitivity report (pg. 64) Re-solve (manually, or with Solver Table) Reformulate Logic
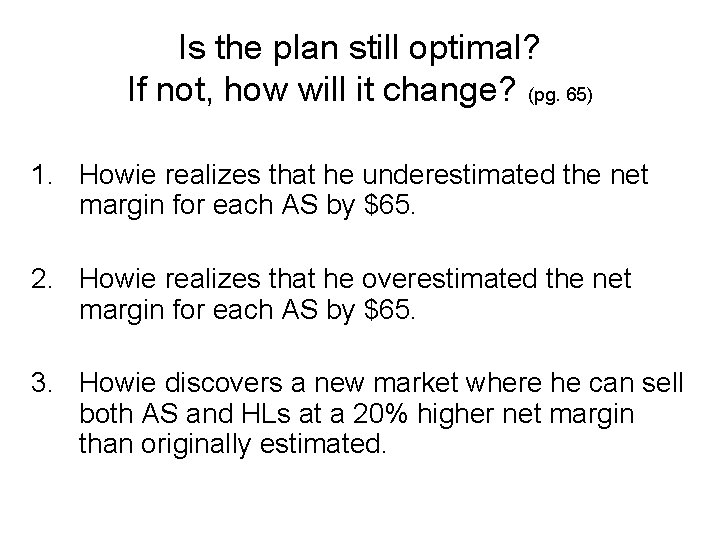
Is the plan still optimal? If not, how will it change? (pg. 65) 1. Howie realizes that he underestimated the net margin for each AS by $65. 2. Howie realizes that he overestimated the net margin for each AS by $65. 3. Howie discovers a new market where he can sell both AS and HLs at a 20% higher net margin than originally estimated.
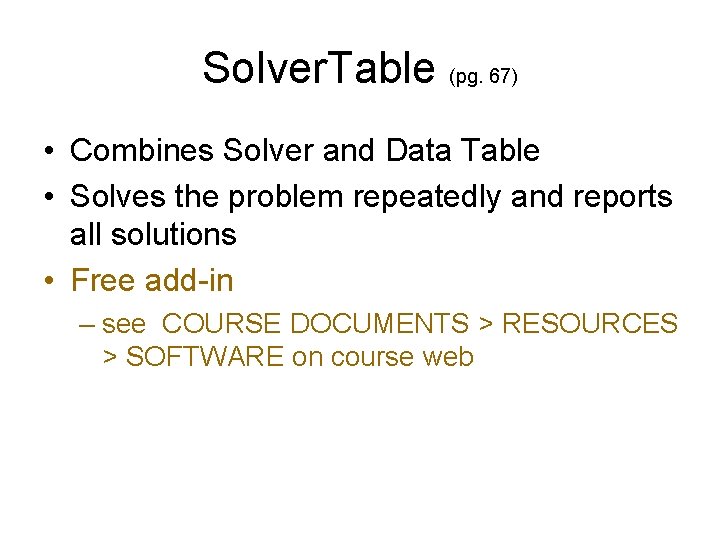
Solver. Table (pg. 67) • Combines Solver and Data Table • Solves the problem repeatedly and reports all solutions • Free add-in – see COURSE DOCUMENTS > RESOURCES > SOFTWARE on course web
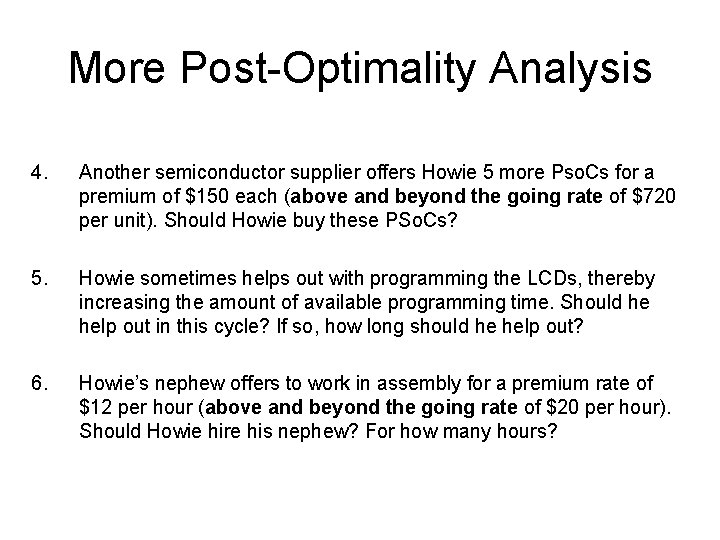
More Post-Optimality Analysis 4. Another semiconductor supplier offers Howie 5 more Pso. Cs for a premium of $150 each (above and beyond the going rate of $720 per unit). Should Howie buy these PSo. Cs? 5. Howie sometimes helps out with programming the LCDs, thereby increasing the amount of available programming time. Should he help out in this cycle? If so, how long should he help out? 6. Howie’s nephew offers to work in assembly for a premium rate of $12 per hour (above and beyond the going rate of $20 per hour). Should Howie hire his nephew? For how many hours?
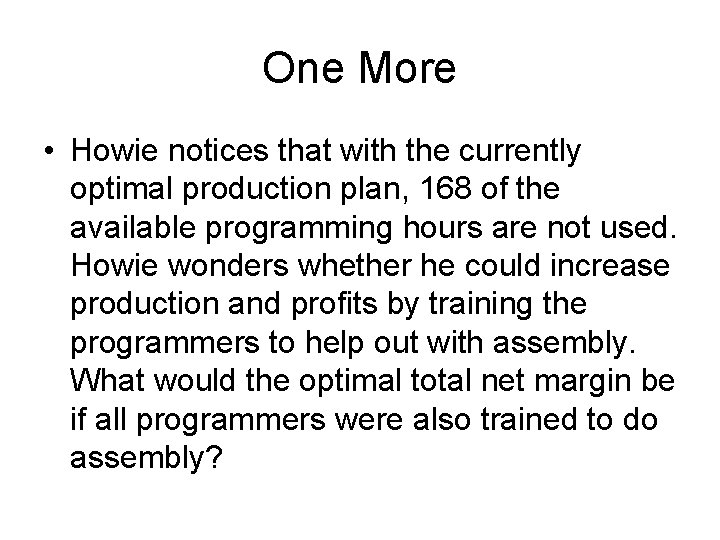
One More • Howie notices that with the currently optimal production plan, 168 of the available programming hours are not used. Howie wonders whether he could increase production and profits by training the programmers to help out with assembly. What would the optimal total net margin be if all programmers were also trained to do assembly?
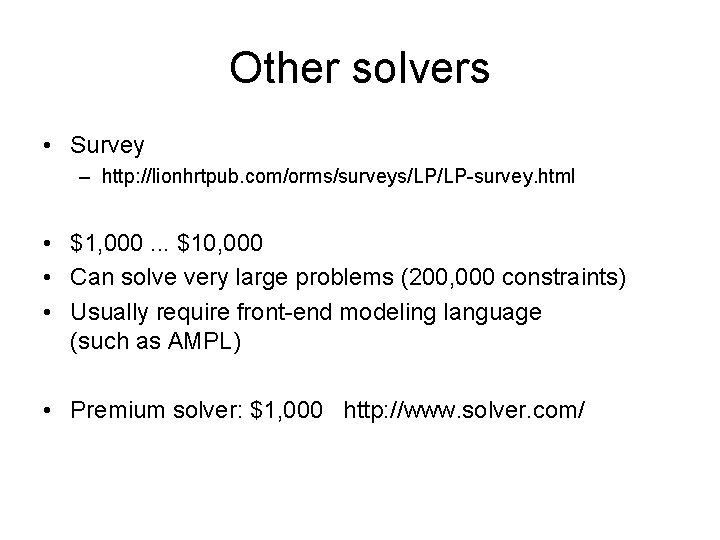
Other solvers • Survey – http: //lionhrtpub. com/orms/surveys/LP/LP-survey. html • $1, 000. . . $10, 000 • Can solve very large problems (200, 000 constraints) • Usually require front-end modeling language (such as AMPL) • Premium solver: $1, 000 http: //www. solver. com/
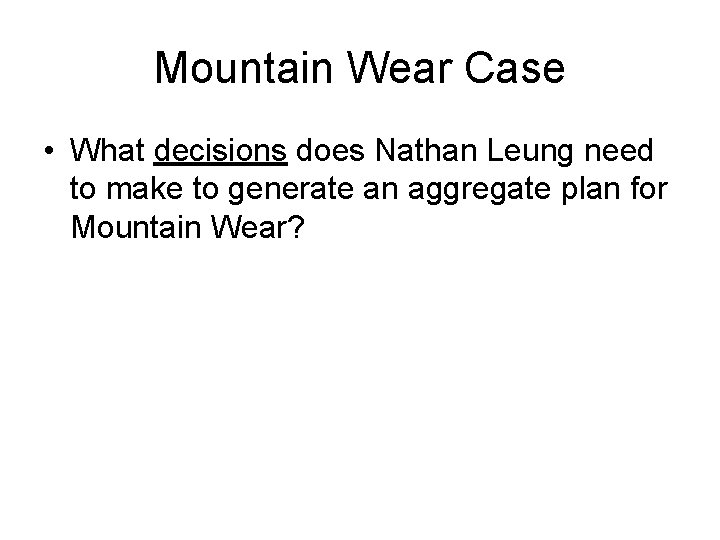
Mountain Wear Case • What decisions does Nathan Leung need to make to generate an aggregate plan for Mountain Wear?
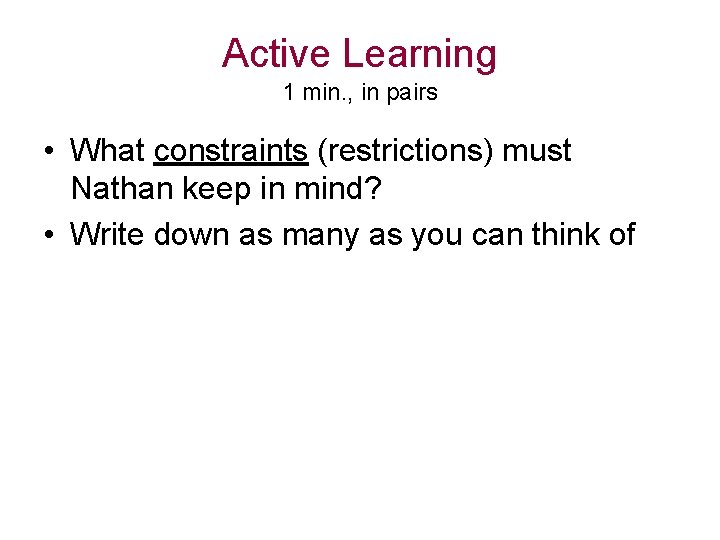
Active Learning 1 min. , in pairs • What constraints (restrictions) must Nathan keep in mind? • Write down as many as you can think of
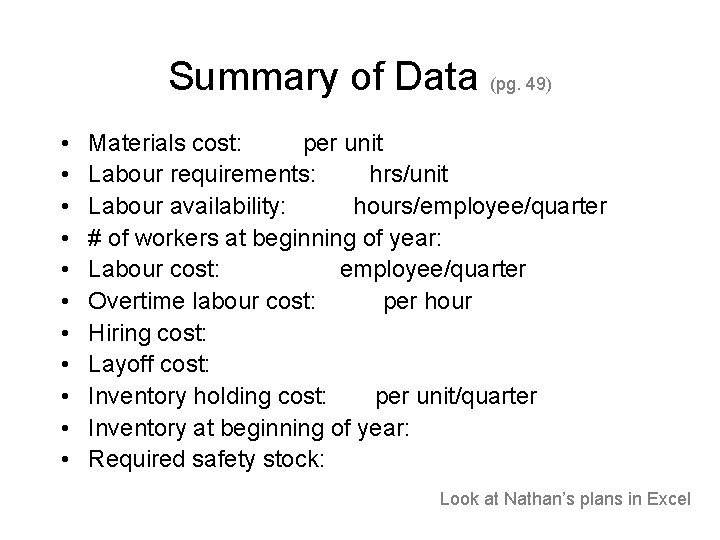
Summary of Data (pg. 49) • • • Materials cost: per unit Labour requirements: hrs/unit Labour availability: hours/employee/quarter # of workers at beginning of year: Labour cost: employee/quarter Overtime labour cost: per hour Hiring cost: Layoff cost: Inventory holding cost: per unit/quarter Inventory at beginning of year: Required safety stock: Look at Nathan’s plans in Excel
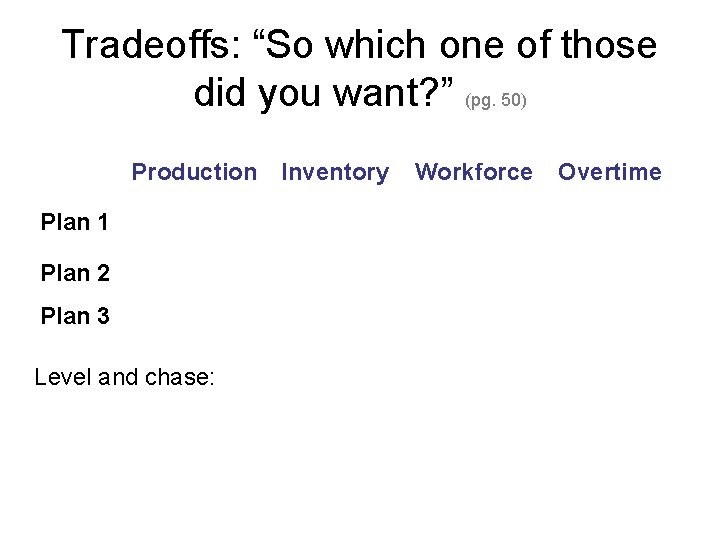
Tradeoffs: “So which one of those did you want? ” (pg. 50) Production Inventory Plan 1 Plan 2 Plan 3 Level and chase: Workforce Overtime
Mgtsc
Perencanaan agregat
List the strategic objectives of aggregate planning
Aggregate planning is capacity planning for
Chapter 33 aggregate demand and aggregate supply
How to calculate aggregate demand
Tableau cannot mix aggregate and non aggregate
Unit 3 aggregate demand and aggregate supply
Unit 3 aggregate demand aggregate supply and fiscal policy
Unit 3 aggregate demand aggregate supply and fiscal policy
Best worst and average case
01:640:244 lecture notes - lecture 15: plat, idah, farad
Transportation matrix example
Chase strategy examples
Level strategy production planning
Chase demand strategy formula
Aggregate planning solved examples