Lecture 12 Credit Risk Management What is credit
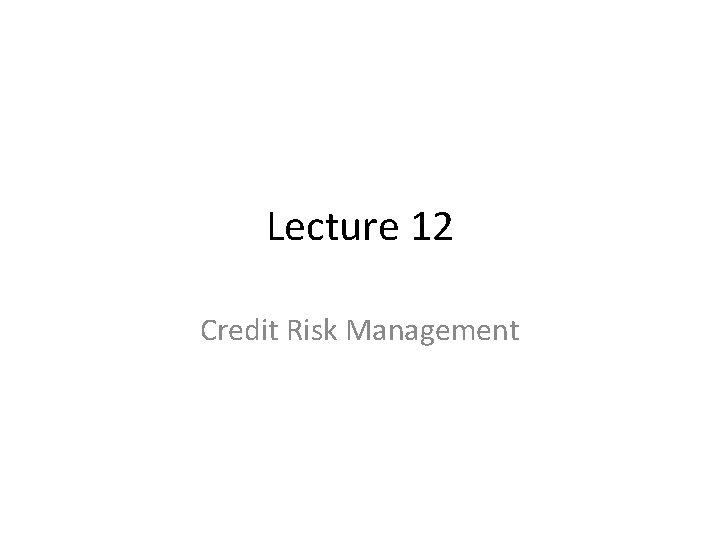
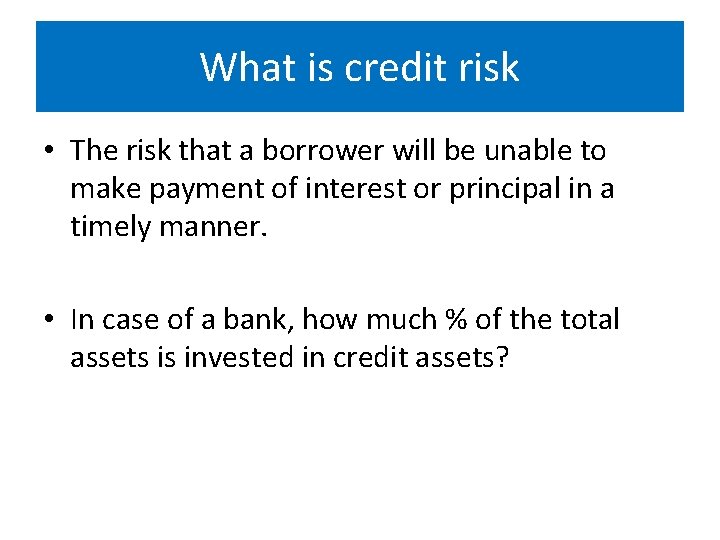
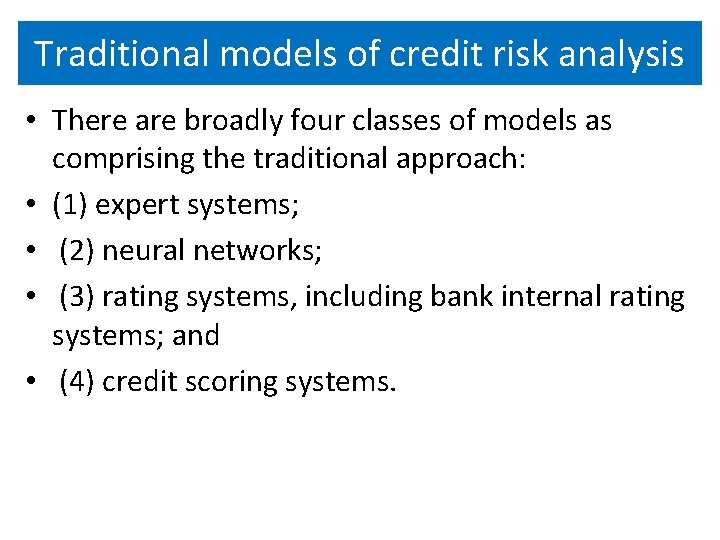
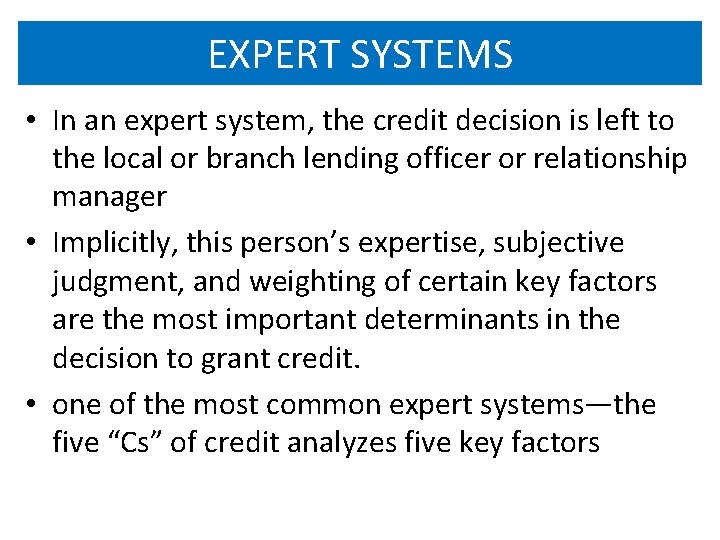
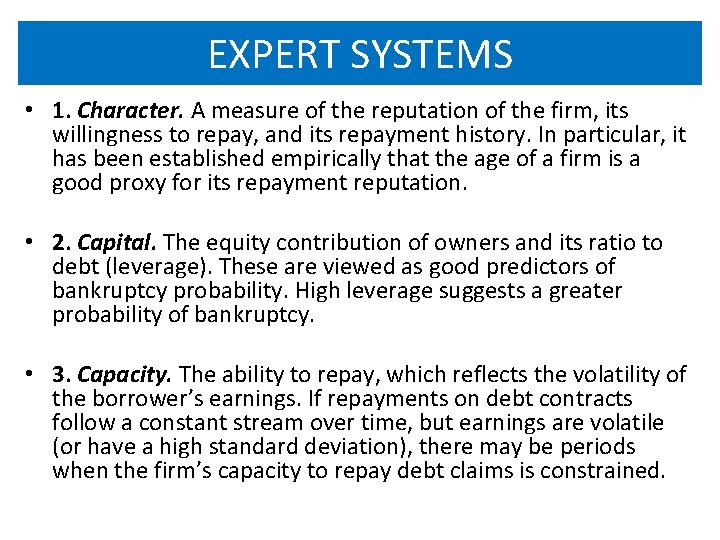
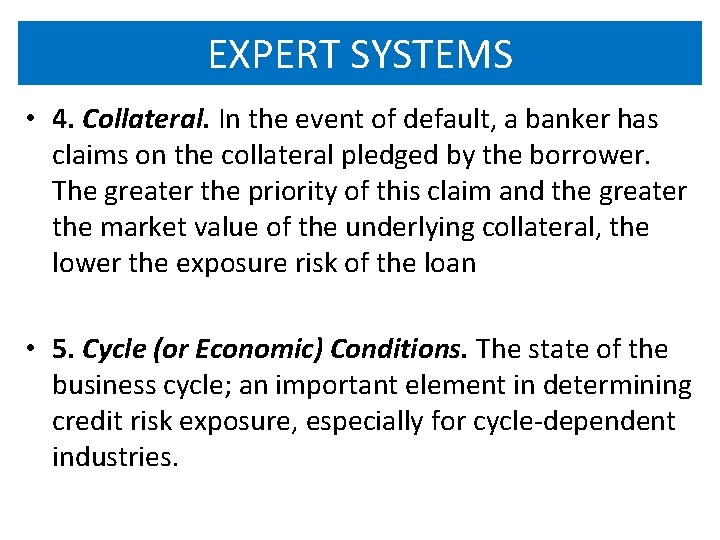
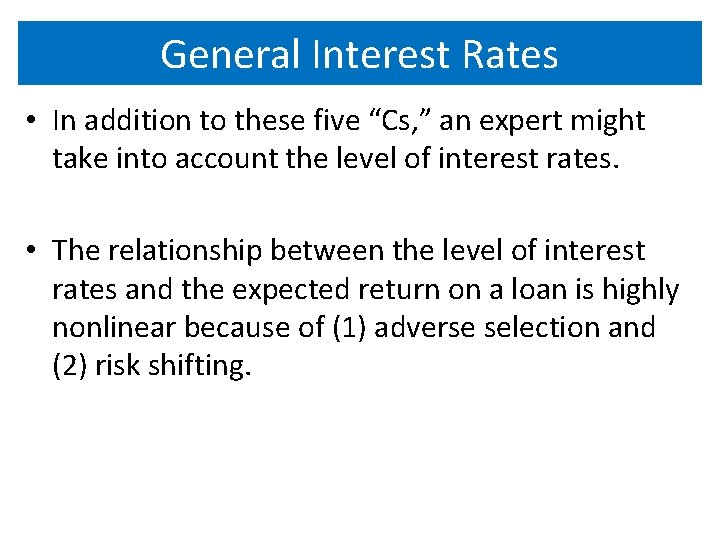
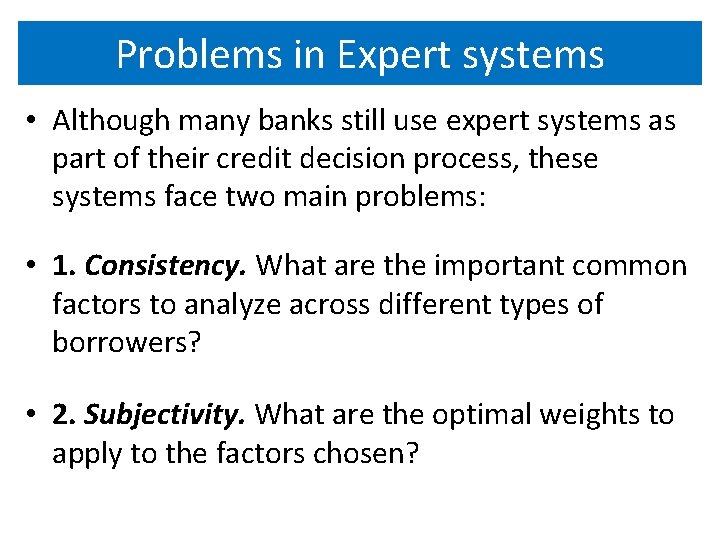
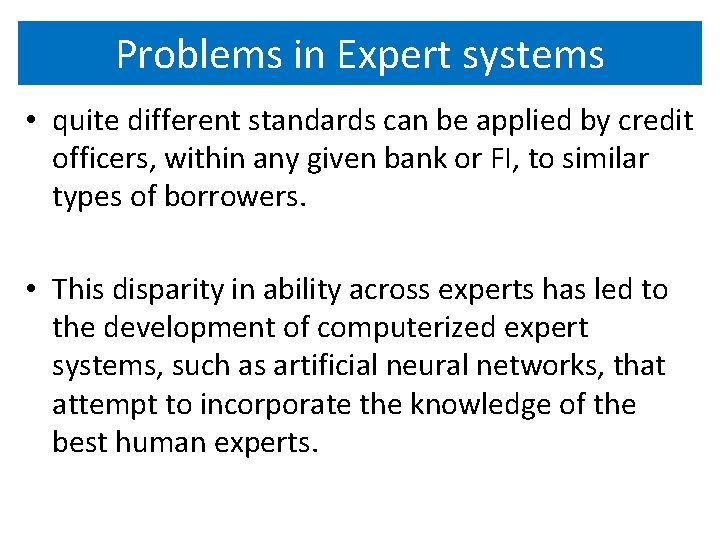
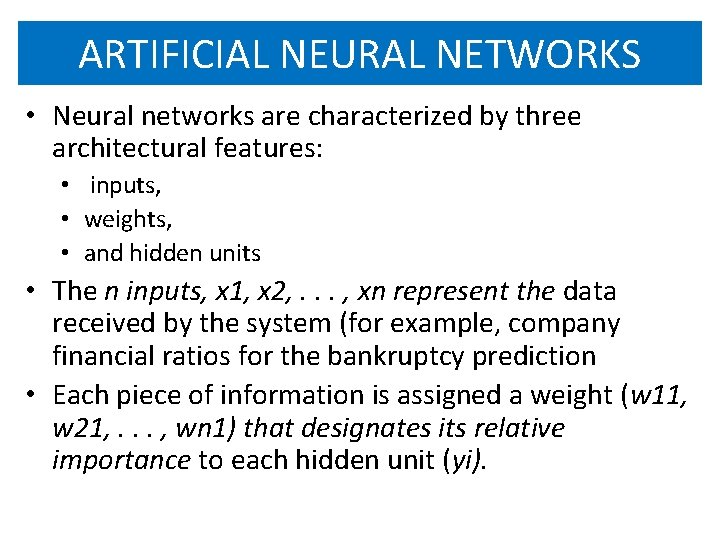
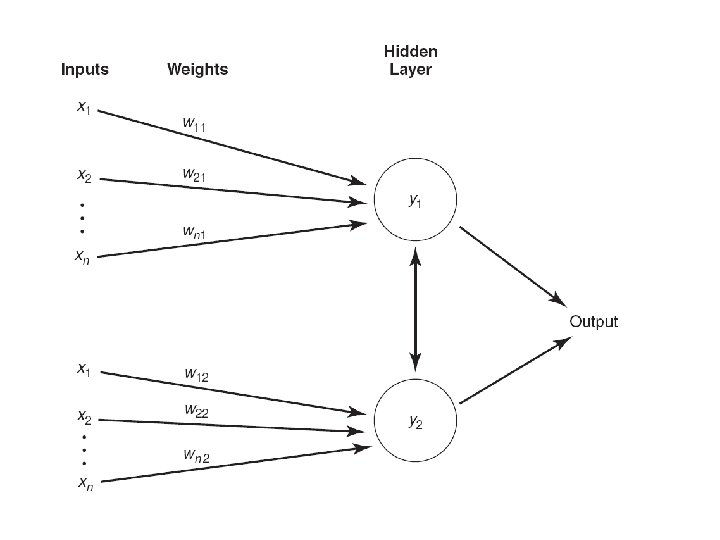
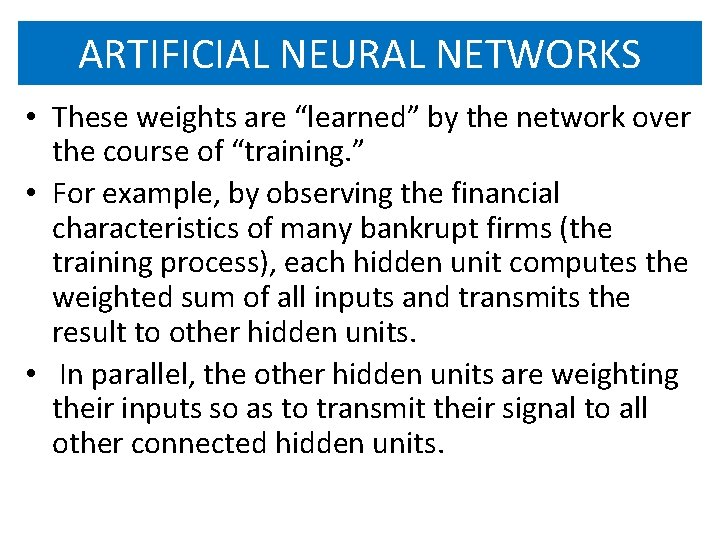
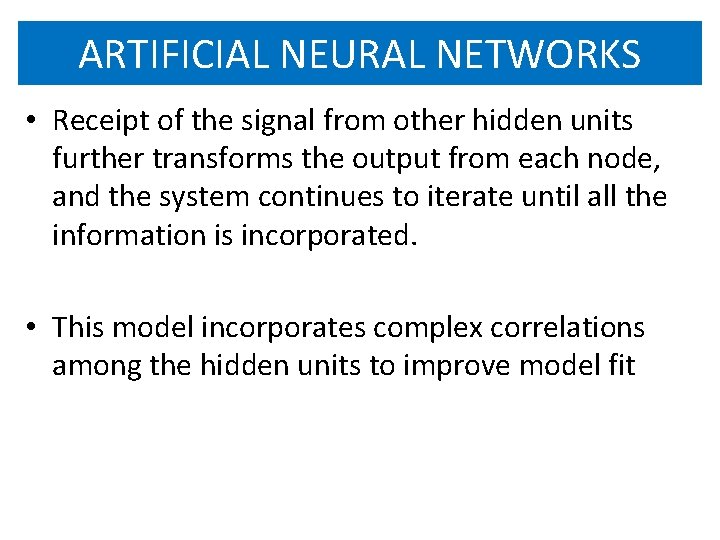
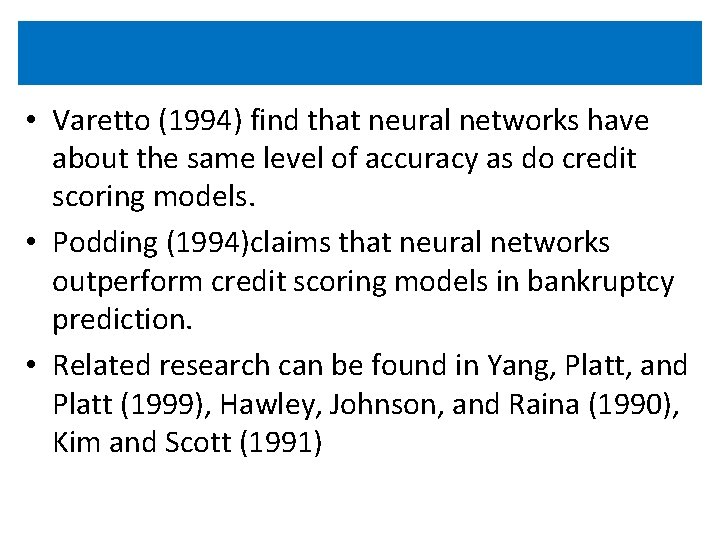
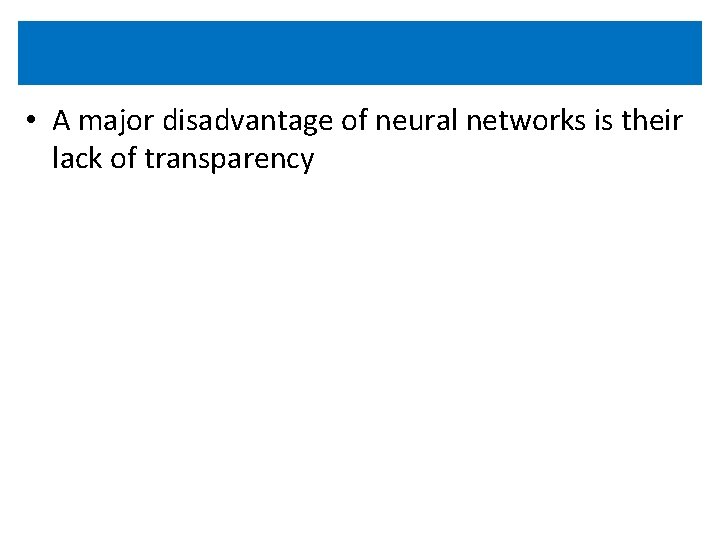
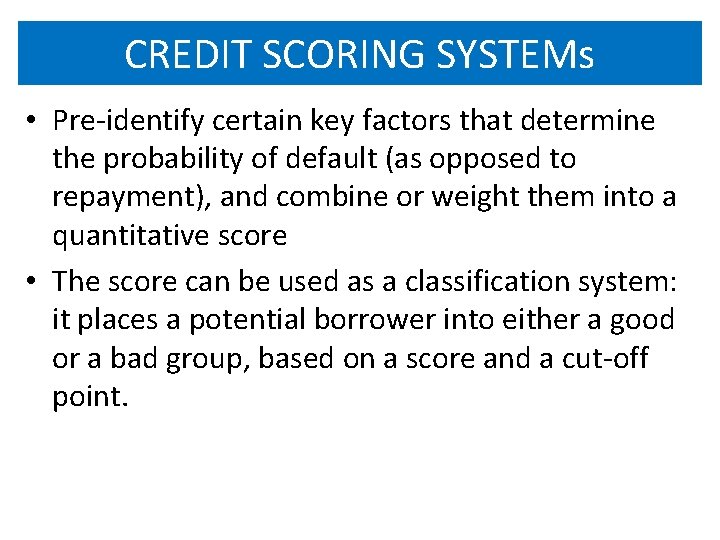
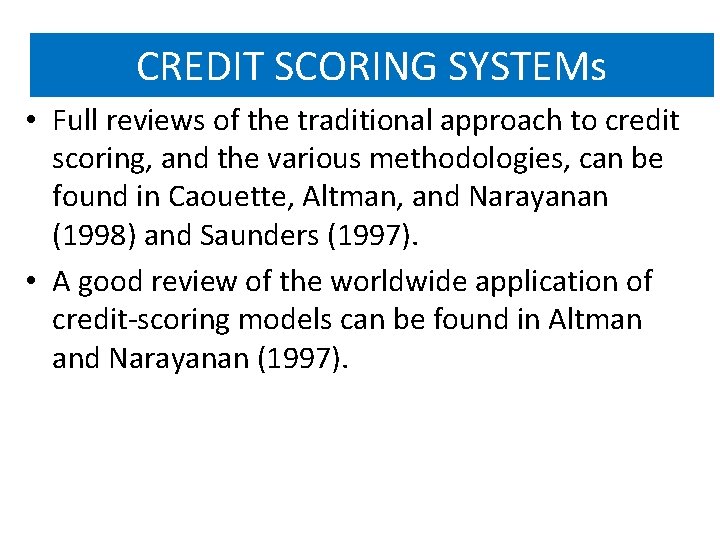
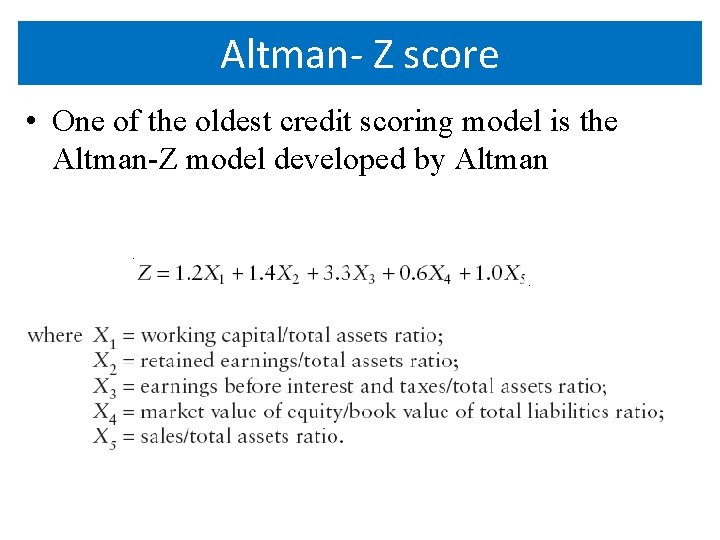
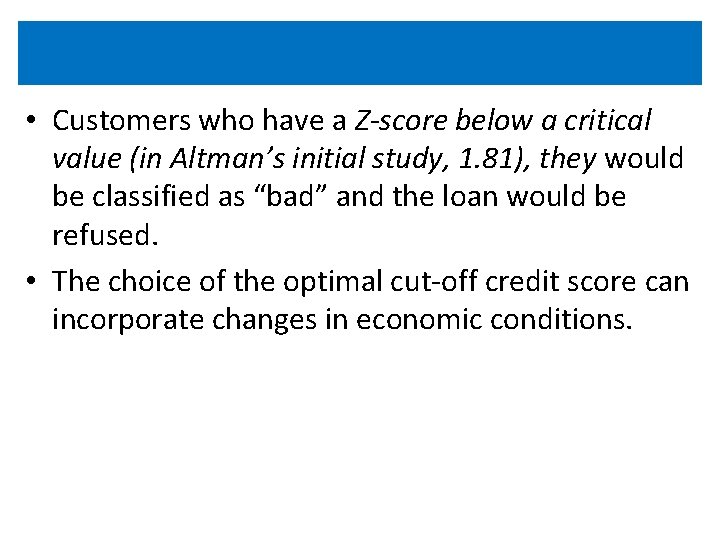
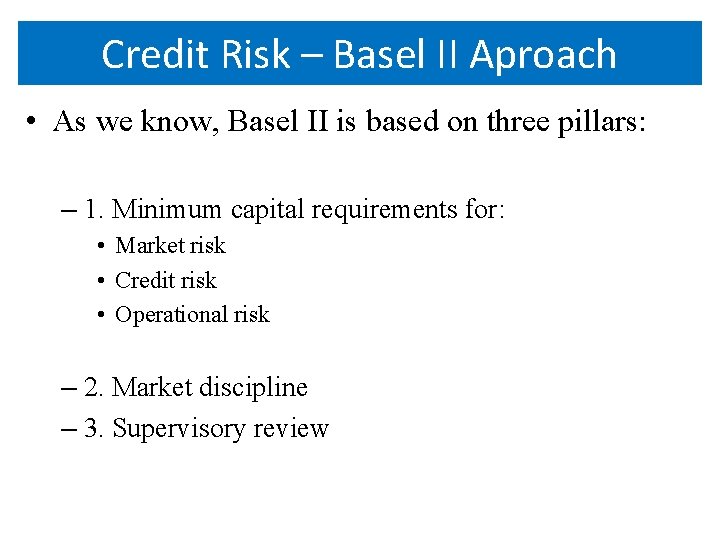
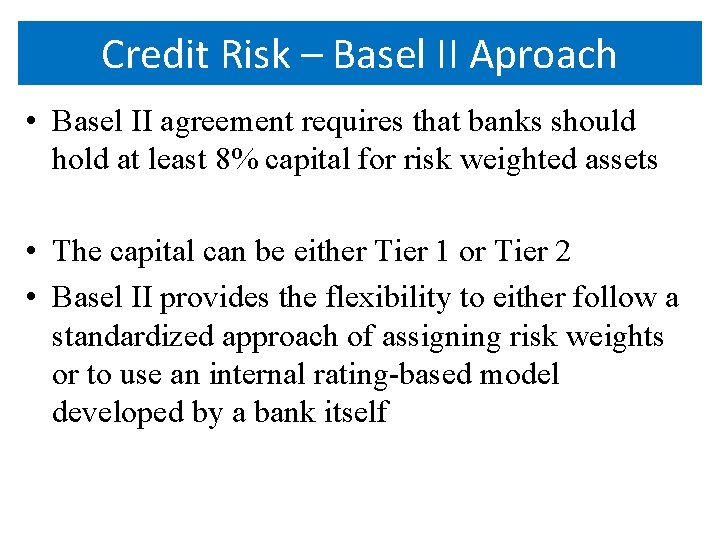
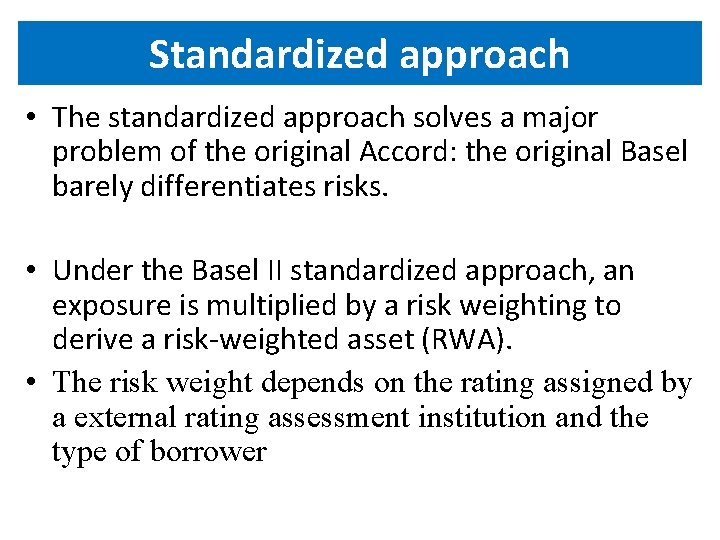
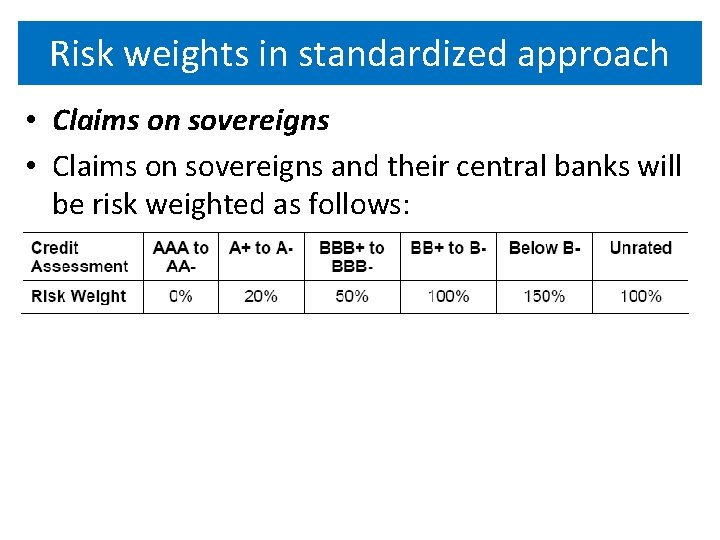
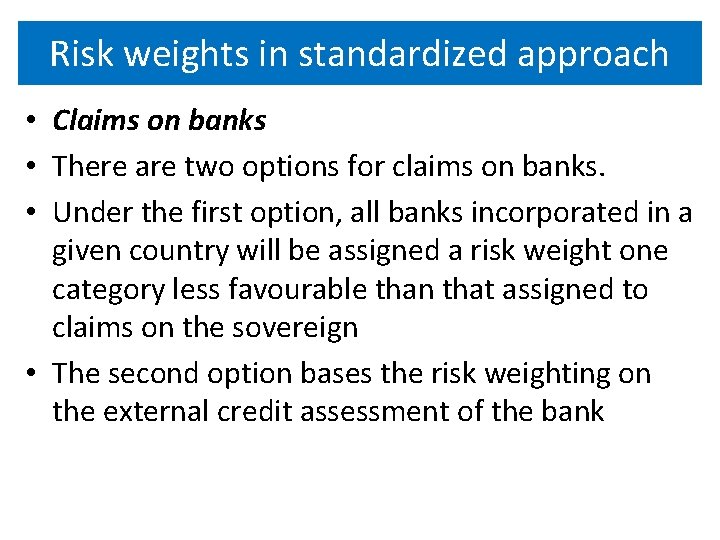
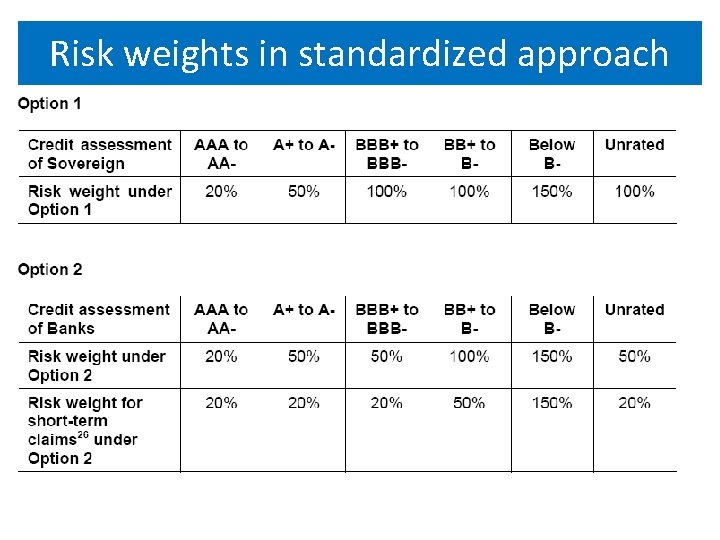
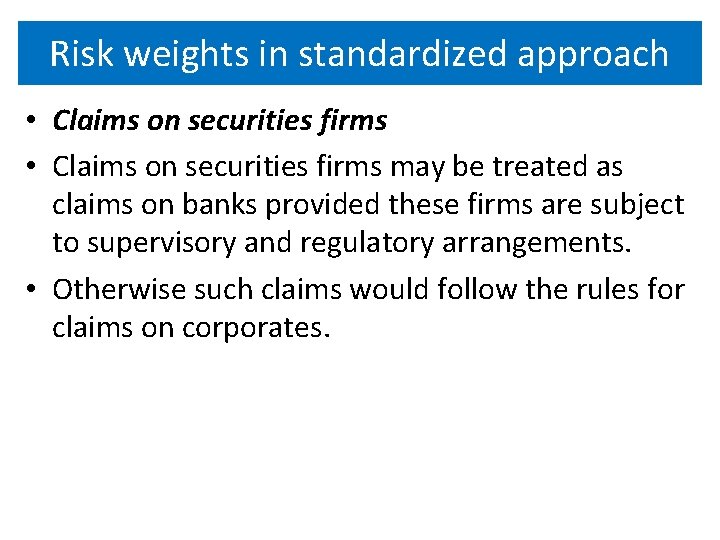
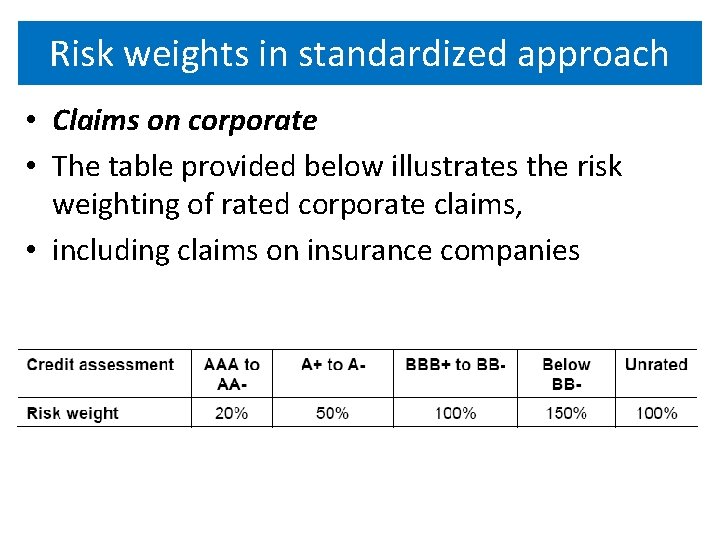
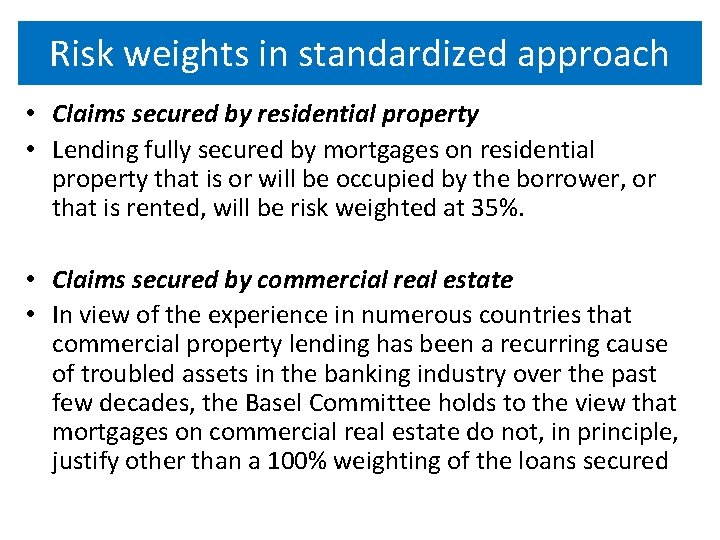
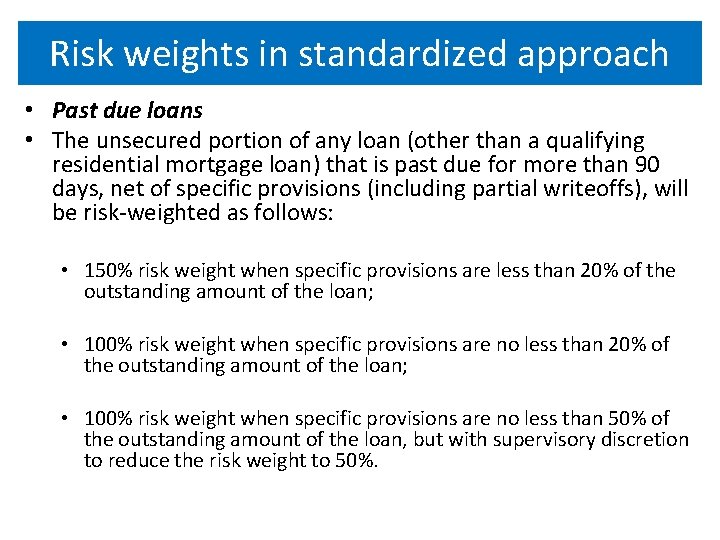
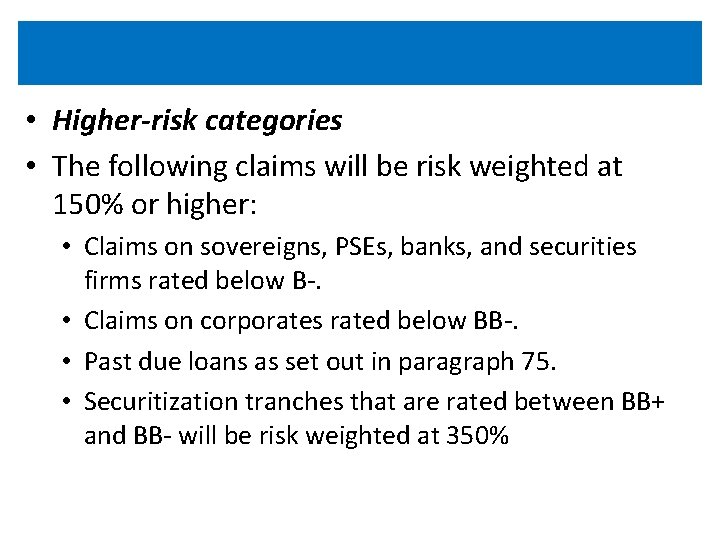
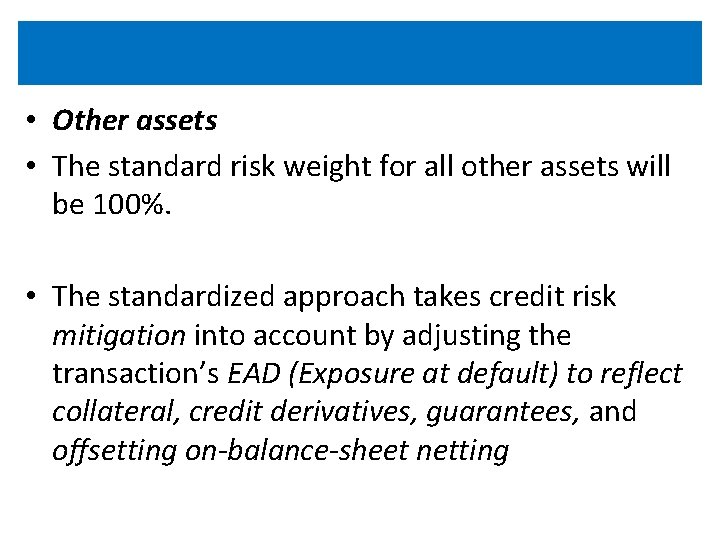
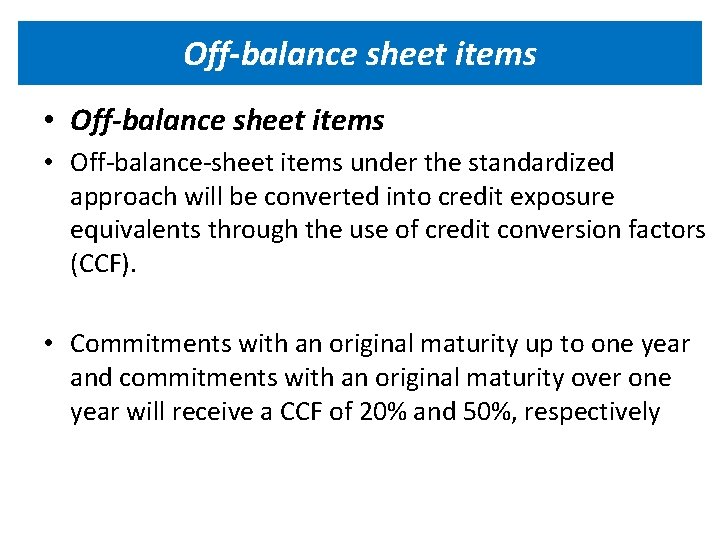
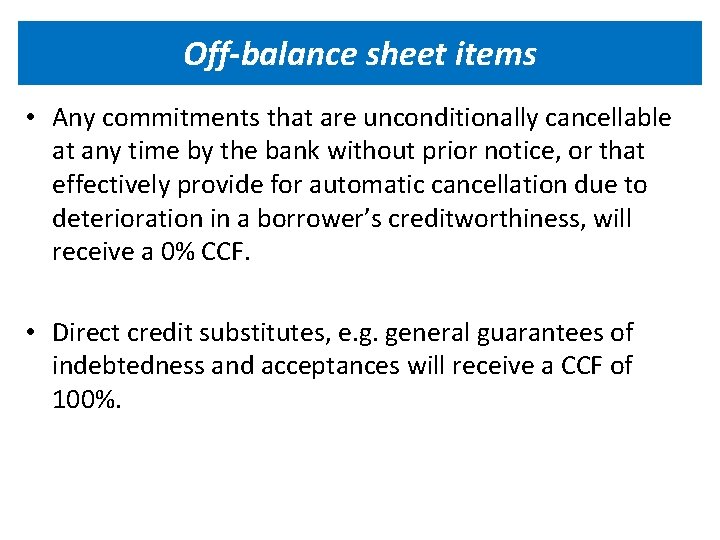
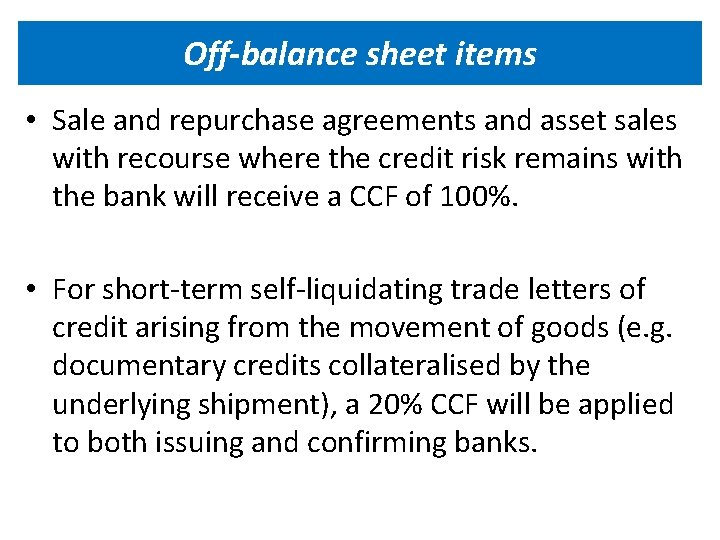
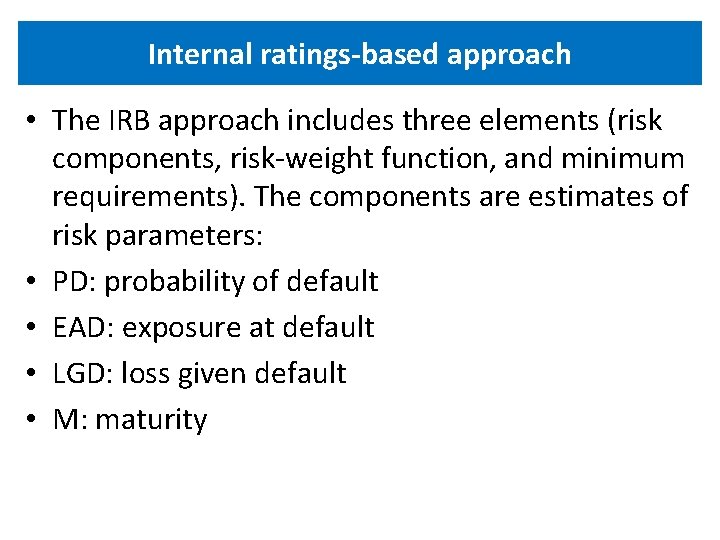
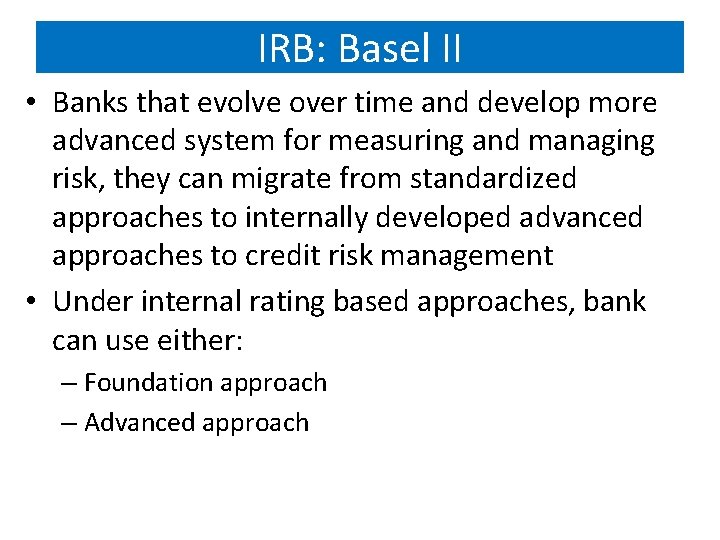
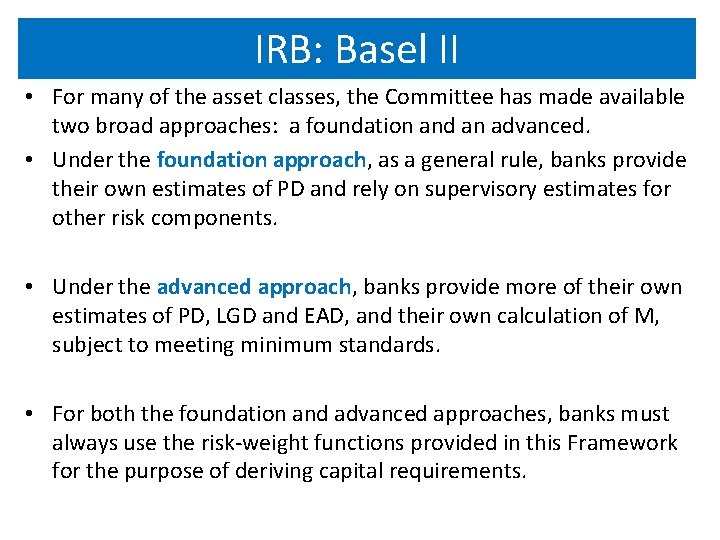
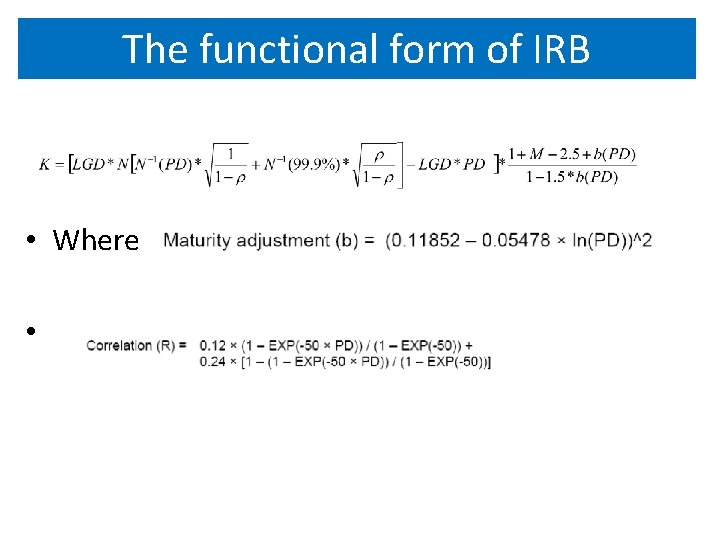
- Slides: 38
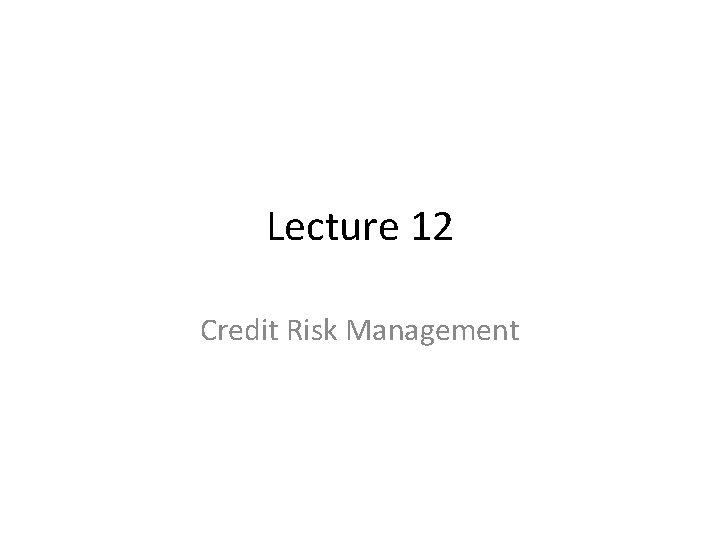
Lecture 12 Credit Risk Management
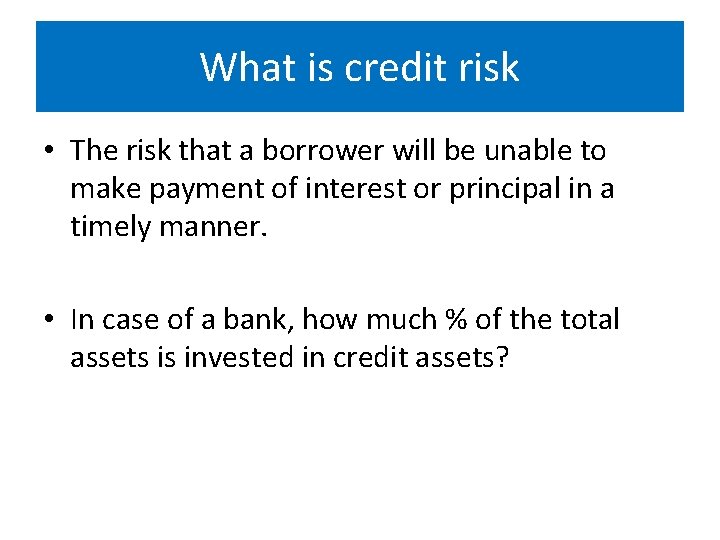
What is credit risk • The risk that a borrower will be unable to make payment of interest or principal in a timely manner. • In case of a bank, how much % of the total assets is invested in credit assets?
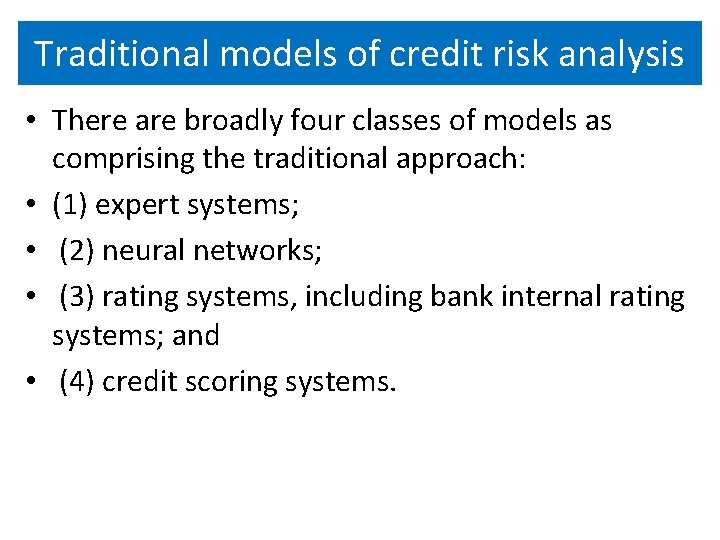
Traditional models of credit risk analysis • There are broadly four classes of models as comprising the traditional approach: • (1) expert systems; • (2) neural networks; • (3) rating systems, including bank internal rating systems; and • (4) credit scoring systems.
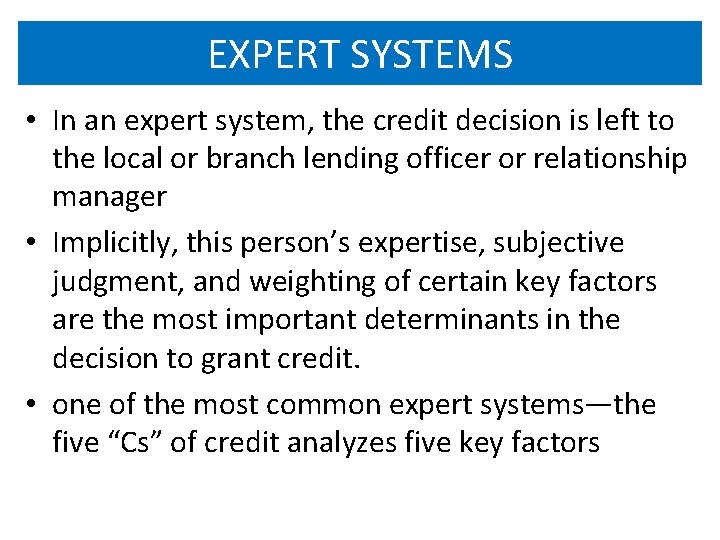
EXPERT SYSTEMS • In an expert system, the credit decision is left to the local or branch lending officer or relationship manager • Implicitly, this person’s expertise, subjective judgment, and weighting of certain key factors are the most important determinants in the decision to grant credit. • one of the most common expert systems—the five “Cs” of credit analyzes five key factors
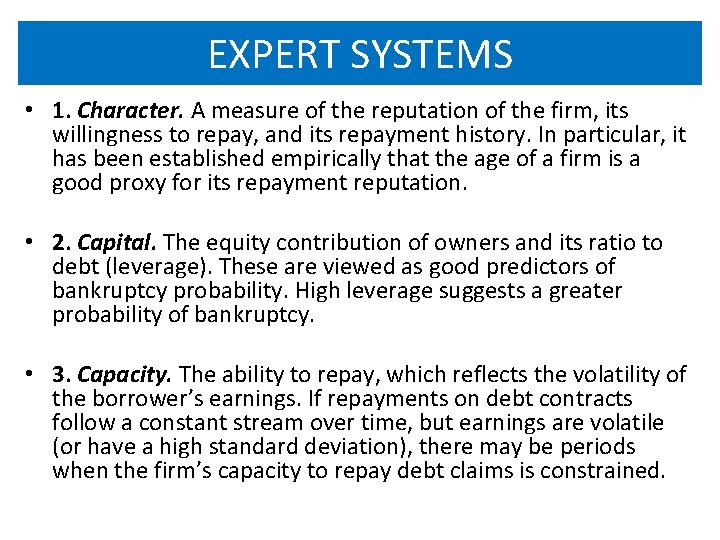
EXPERT SYSTEMS • 1. Character. A measure of the reputation of the firm, its willingness to repay, and its repayment history. In particular, it has been established empirically that the age of a firm is a good proxy for its repayment reputation. • 2. Capital. The equity contribution of owners and its ratio to debt (leverage). These are viewed as good predictors of bankruptcy probability. High leverage suggests a greater probability of bankruptcy. • 3. Capacity. The ability to repay, which reflects the volatility of the borrower’s earnings. If repayments on debt contracts follow a constant stream over time, but earnings are volatile (or have a high standard deviation), there may be periods when the firm’s capacity to repay debt claims is constrained.
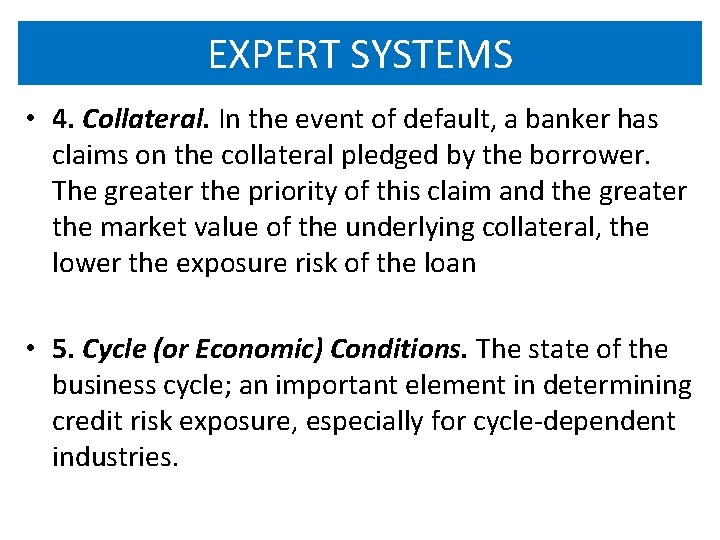
EXPERT SYSTEMS • 4. Collateral. In the event of default, a banker has claims on the collateral pledged by the borrower. The greater the priority of this claim and the greater the market value of the underlying collateral, the lower the exposure risk of the loan • 5. Cycle (or Economic) Conditions. The state of the business cycle; an important element in determining credit risk exposure, especially for cycle-dependent industries.
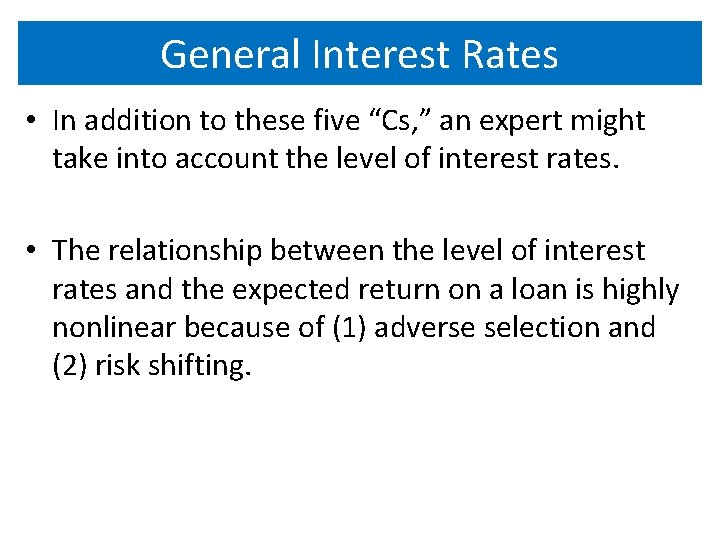
General Interest Rates • In addition to these five “Cs, ” an expert might take into account the level of interest rates. • The relationship between the level of interest rates and the expected return on a loan is highly nonlinear because of (1) adverse selection and (2) risk shifting.
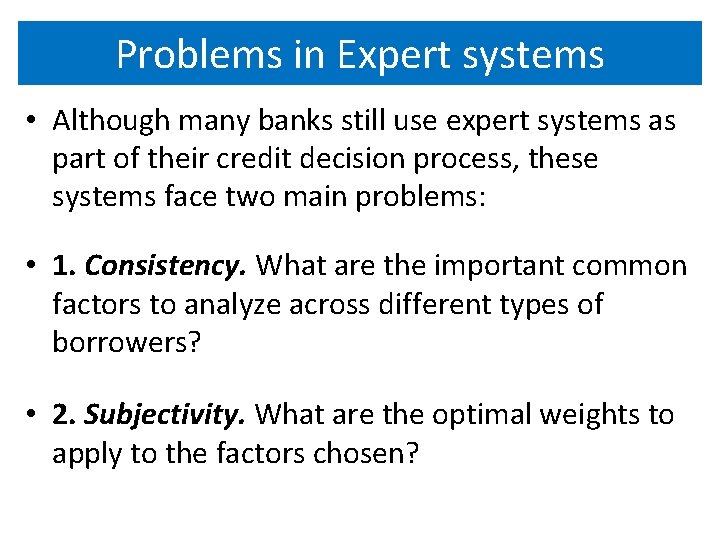
Problems in Expert systems • Although many banks still use expert systems as part of their credit decision process, these systems face two main problems: • 1. Consistency. What are the important common factors to analyze across different types of borrowers? • 2. Subjectivity. What are the optimal weights to apply to the factors chosen?
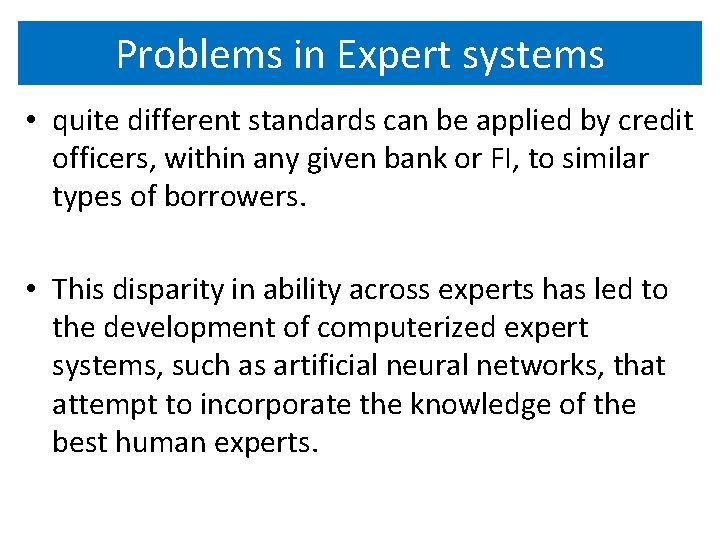
Problems in Expert systems • quite different standards can be applied by credit officers, within any given bank or FI, to similar types of borrowers. • This disparity in ability across experts has led to the development of computerized expert systems, such as artificial neural networks, that attempt to incorporate the knowledge of the best human experts.
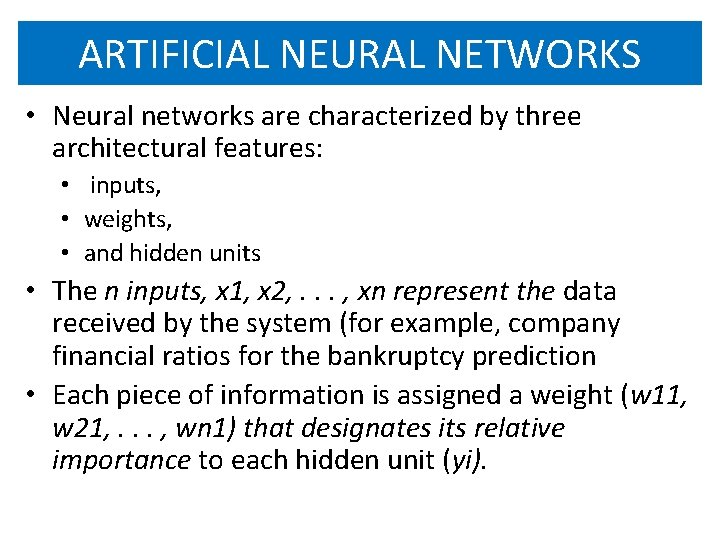
ARTIFICIAL NEURAL NETWORKS • Neural networks are characterized by three architectural features: • inputs, • weights, • and hidden units • The n inputs, x 1, x 2, . . . , xn represent the data received by the system (for example, company financial ratios for the bankruptcy prediction • Each piece of information is assigned a weight (w 11, w 21, . . . , wn 1) that designates its relative importance to each hidden unit (yi).
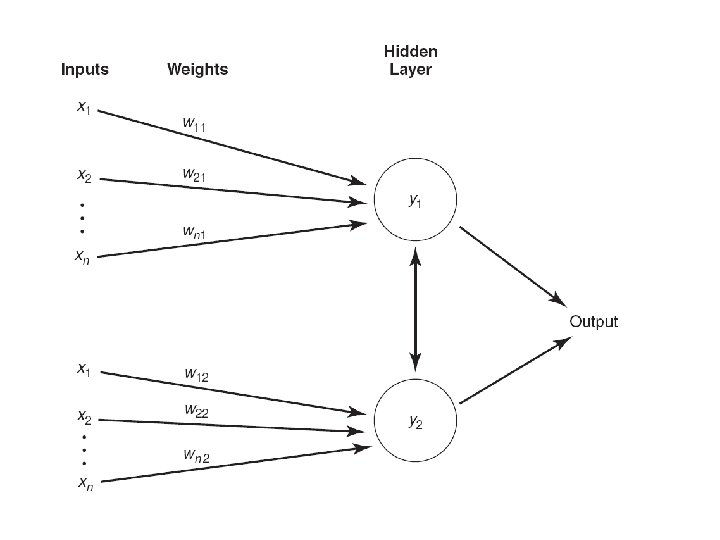
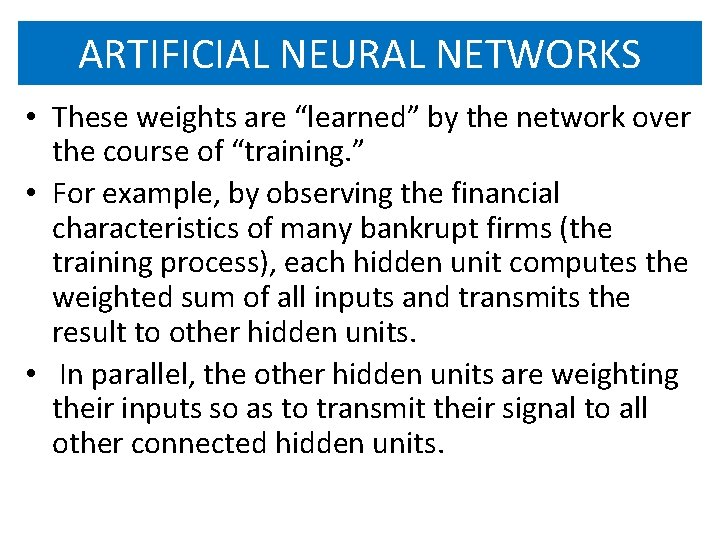
ARTIFICIAL NEURAL NETWORKS • These weights are “learned” by the network over the course of “training. ” • For example, by observing the financial characteristics of many bankrupt firms (the training process), each hidden unit computes the weighted sum of all inputs and transmits the result to other hidden units. • In parallel, the other hidden units are weighting their inputs so as to transmit their signal to all other connected hidden units.
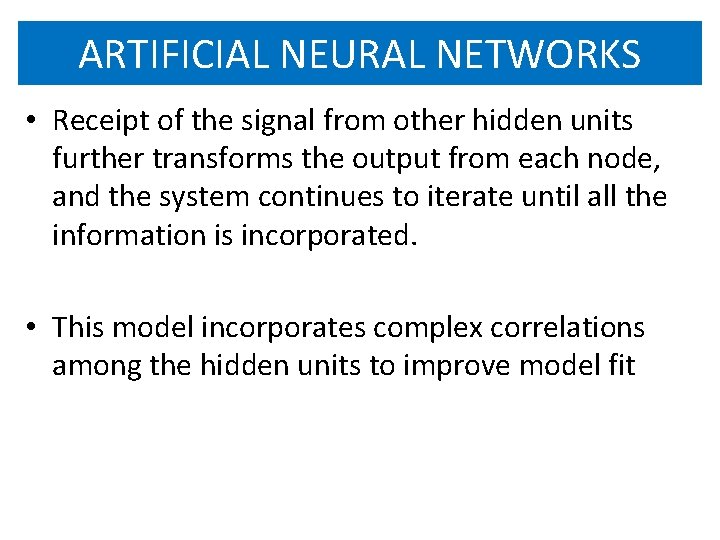
ARTIFICIAL NEURAL NETWORKS • Receipt of the signal from other hidden units further transforms the output from each node, and the system continues to iterate until all the information is incorporated. • This model incorporates complex correlations among the hidden units to improve model fit
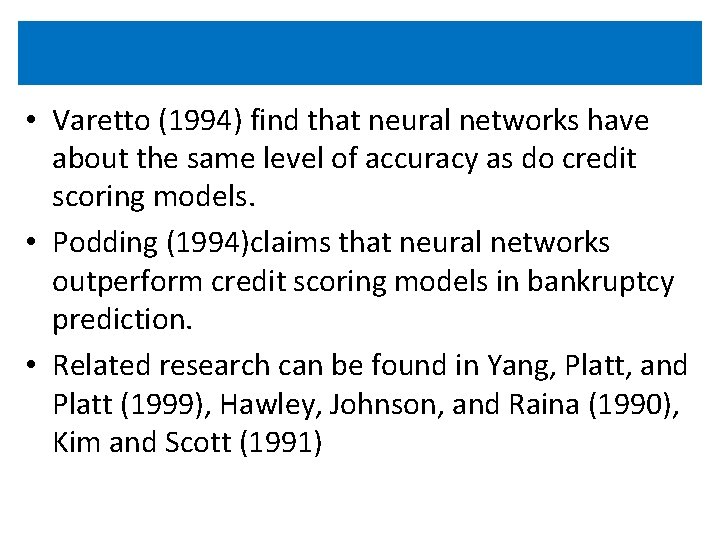
• Varetto (1994) find that neural networks have about the same level of accuracy as do credit scoring models. • Podding (1994)claims that neural networks outperform credit scoring models in bankruptcy prediction. • Related research can be found in Yang, Platt, and Platt (1999), Hawley, Johnson, and Raina (1990), Kim and Scott (1991)
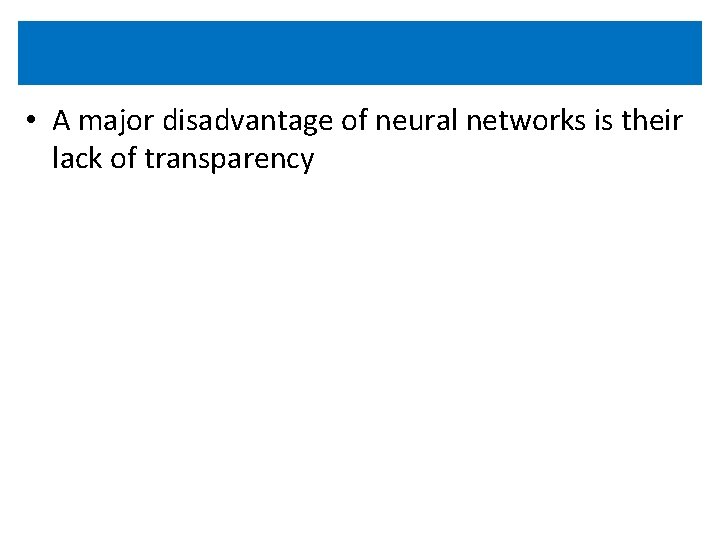
• A major disadvantage of neural networks is their lack of transparency
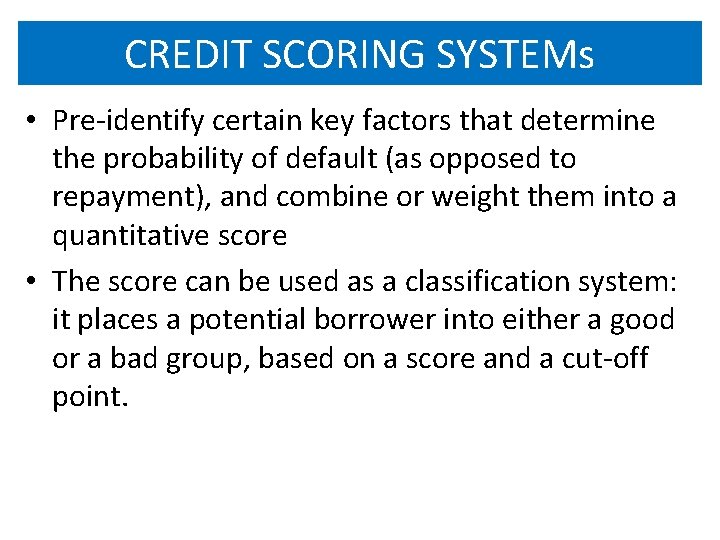
CREDIT SCORING SYSTEMs • Pre-identify certain key factors that determine the probability of default (as opposed to repayment), and combine or weight them into a quantitative score • The score can be used as a classification system: it places a potential borrower into either a good or a bad group, based on a score and a cut-off point.
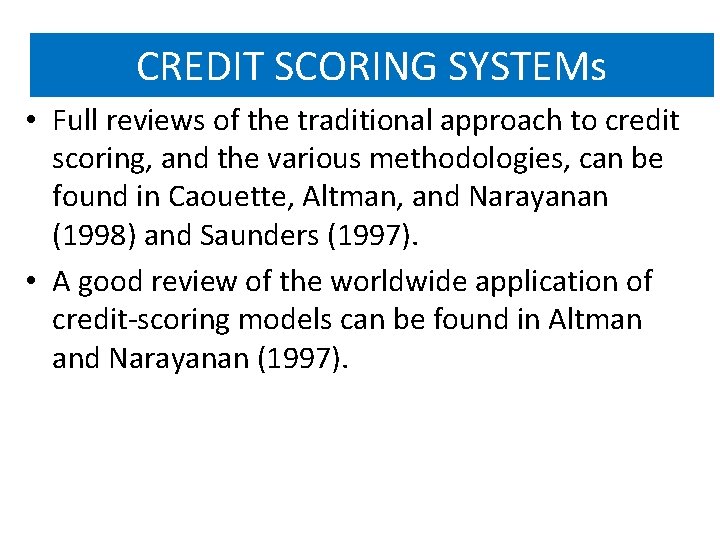
CREDIT SCORING SYSTEMs • Full reviews of the traditional approach to credit scoring, and the various methodologies, can be found in Caouette, Altman, and Narayanan (1998) and Saunders (1997). • A good review of the worldwide application of credit-scoring models can be found in Altman and Narayanan (1997).
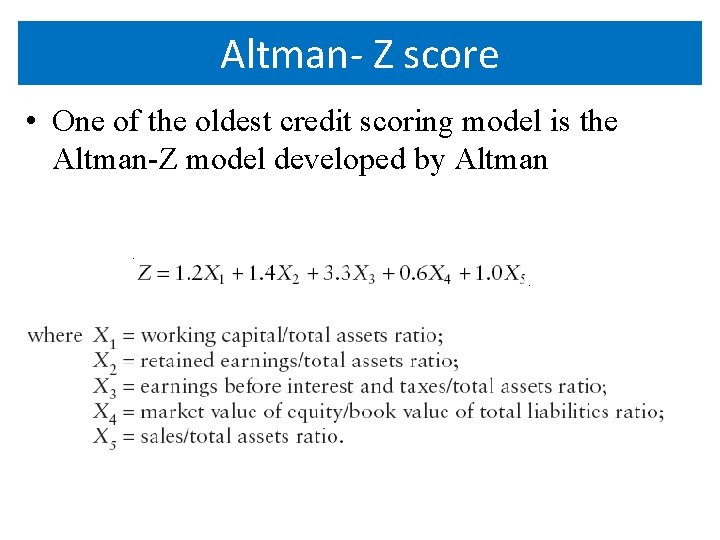
Altman- Z score • One of the oldest credit scoring model is the Altman-Z model developed by Altman
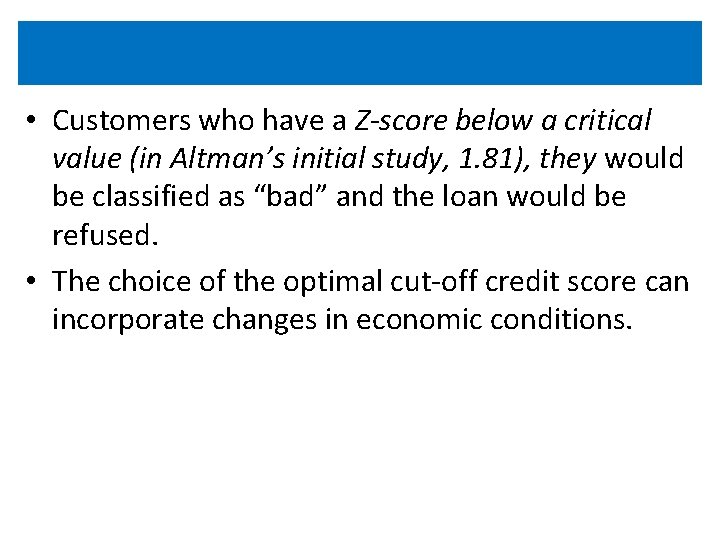
• Customers who have a Z-score below a critical value (in Altman’s initial study, 1. 81), they would be classified as “bad” and the loan would be refused. • The choice of the optimal cut-off credit score can incorporate changes in economic conditions.
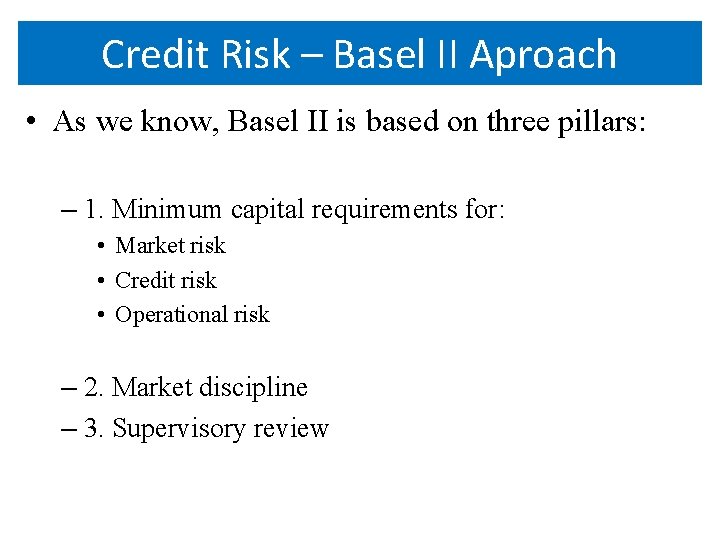
Credit Risk – Basel II Aproach • As we know, Basel II is based on three pillars: – 1. Minimum capital requirements for: • Market risk • Credit risk • Operational risk – 2. Market discipline – 3. Supervisory review
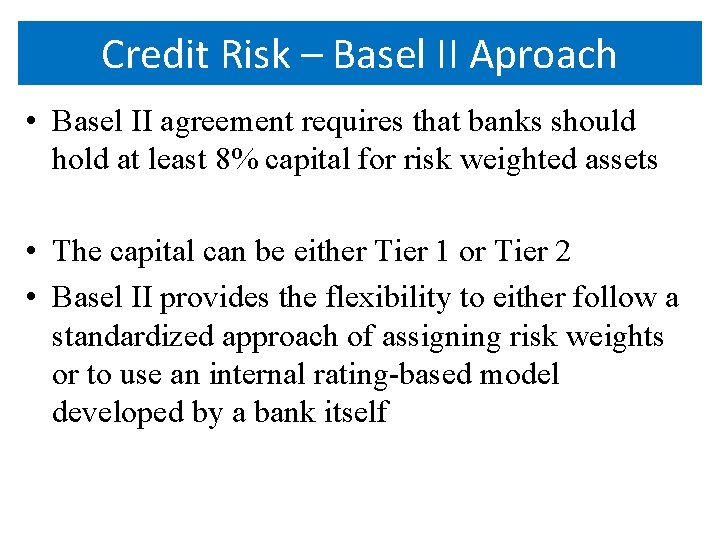
Credit Risk – Basel II Aproach • Basel II agreement requires that banks should hold at least 8% capital for risk weighted assets • The capital can be either Tier 1 or Tier 2 • Basel II provides the flexibility to either follow a standardized approach of assigning risk weights or to use an internal rating-based model developed by a bank itself
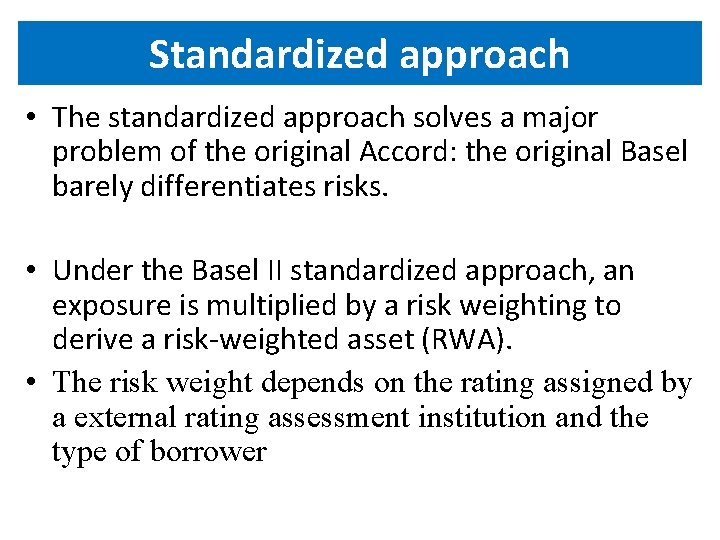
Standardized approach • The standardized approach solves a major problem of the original Accord: the original Basel barely differentiates risks. • Under the Basel II standardized approach, an exposure is multiplied by a risk weighting to derive a risk-weighted asset (RWA). • The risk weight depends on the rating assigned by a external rating assessment institution and the type of borrower
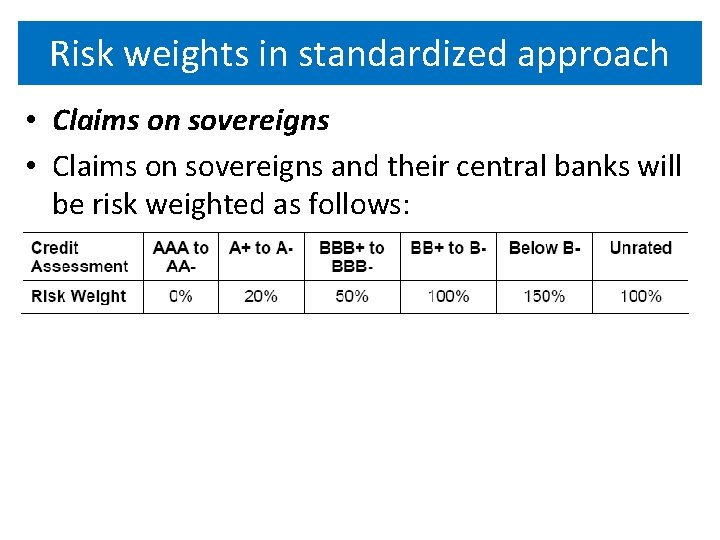
Risk weights in standardized approach • Claims on sovereigns and their central banks will be risk weighted as follows:
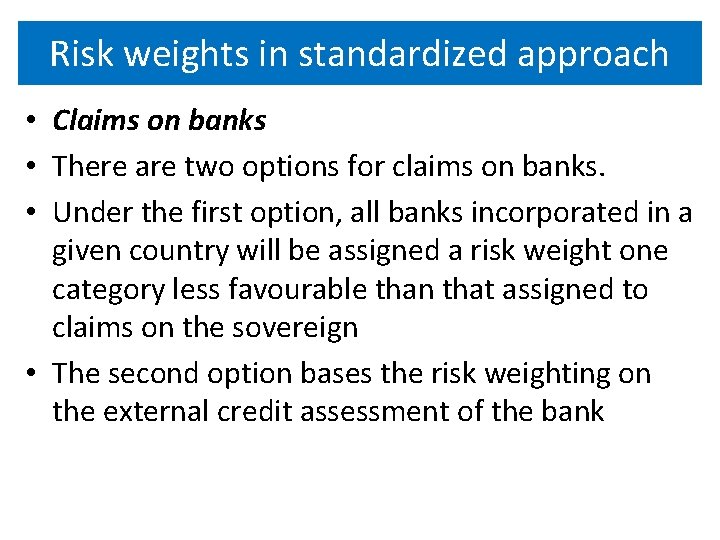
Risk weights in standardized approach • Claims on banks • There are two options for claims on banks. • Under the first option, all banks incorporated in a given country will be assigned a risk weight one category less favourable than that assigned to claims on the sovereign • The second option bases the risk weighting on the external credit assessment of the bank
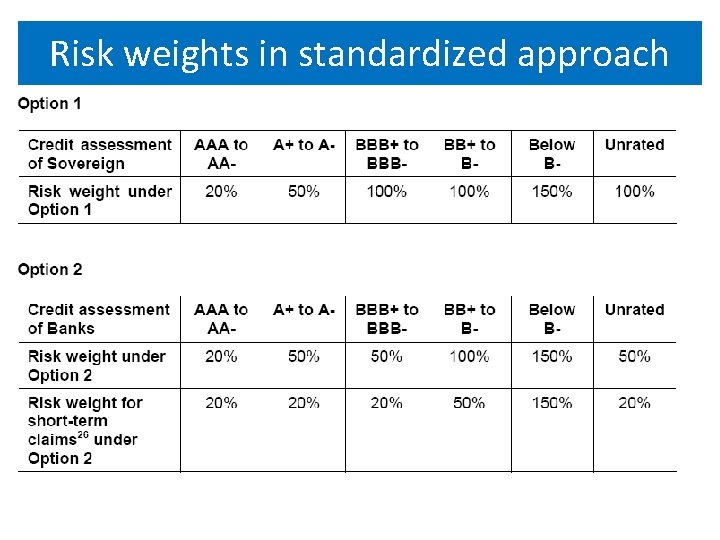
Risk weights in standardized approach
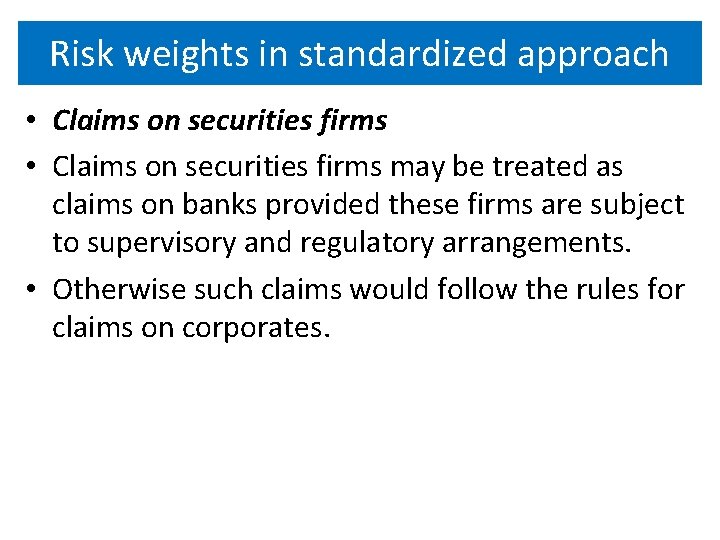
Risk weights in standardized approach • Claims on securities firms may be treated as claims on banks provided these firms are subject to supervisory and regulatory arrangements. • Otherwise such claims would follow the rules for claims on corporates.
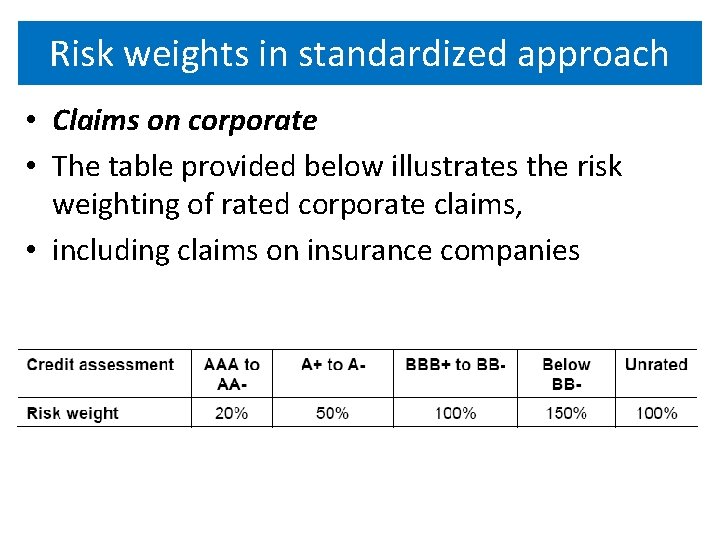
Risk weights in standardized approach • Claims on corporate • The table provided below illustrates the risk weighting of rated corporate claims, • including claims on insurance companies
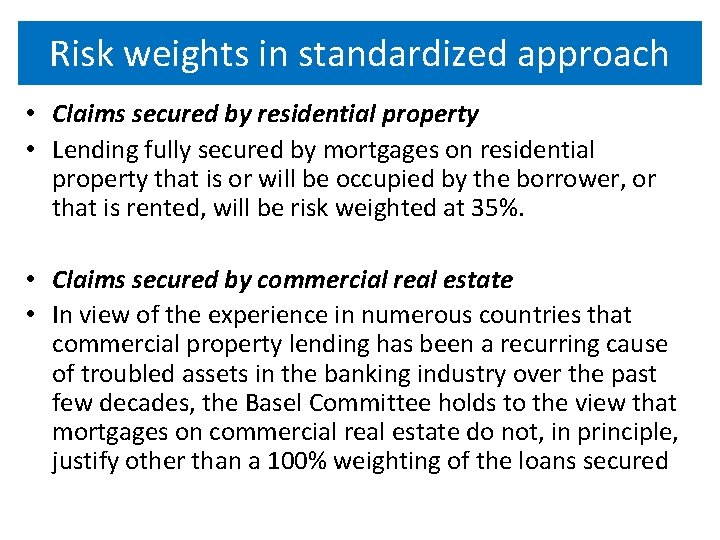
Risk weights in standardized approach • Claims secured by residential property • Lending fully secured by mortgages on residential property that is or will be occupied by the borrower, or that is rented, will be risk weighted at 35%. • Claims secured by commercial real estate • In view of the experience in numerous countries that commercial property lending has been a recurring cause of troubled assets in the banking industry over the past few decades, the Basel Committee holds to the view that mortgages on commercial real estate do not, in principle, justify other than a 100% weighting of the loans secured
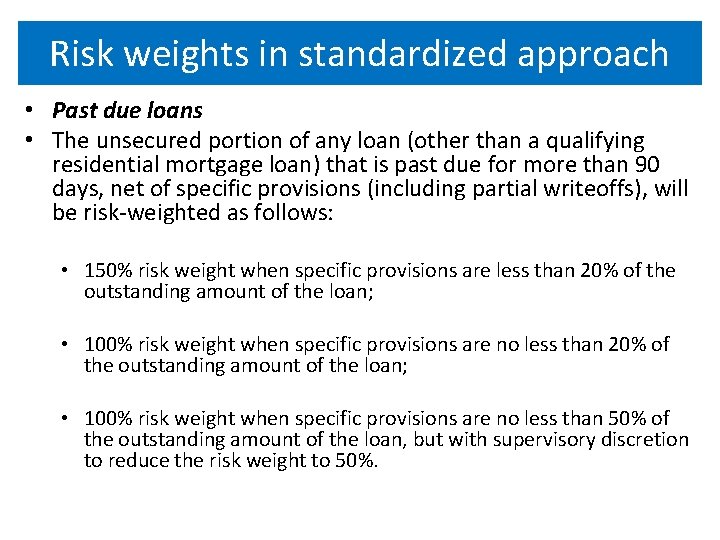
Risk weights in standardized approach • Past due loans • The unsecured portion of any loan (other than a qualifying residential mortgage loan) that is past due for more than 90 days, net of specific provisions (including partial writeoffs), will be risk-weighted as follows: • 150% risk weight when specific provisions are less than 20% of the outstanding amount of the loan; • 100% risk weight when specific provisions are no less than 50% of the outstanding amount of the loan, but with supervisory discretion to reduce the risk weight to 50%.
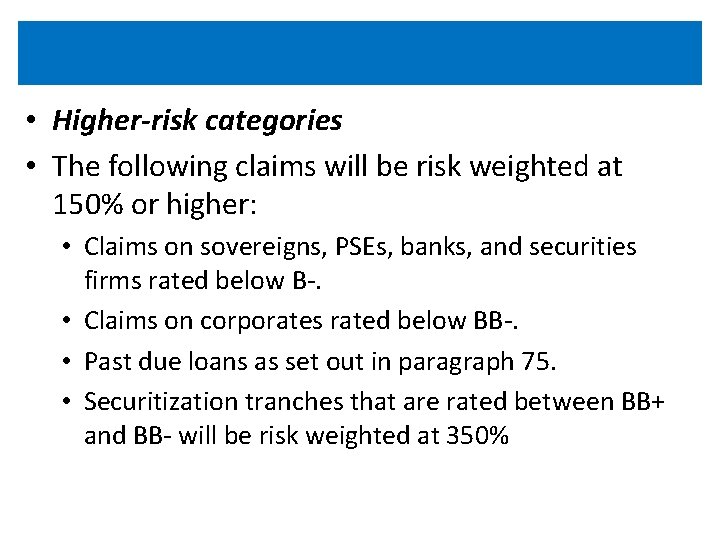
• Higher-risk categories • The following claims will be risk weighted at 150% or higher: • Claims on sovereigns, PSEs, banks, and securities firms rated below B-. • Claims on corporates rated below BB-. • Past due loans as set out in paragraph 75. • Securitization tranches that are rated between BB+ and BB- will be risk weighted at 350%
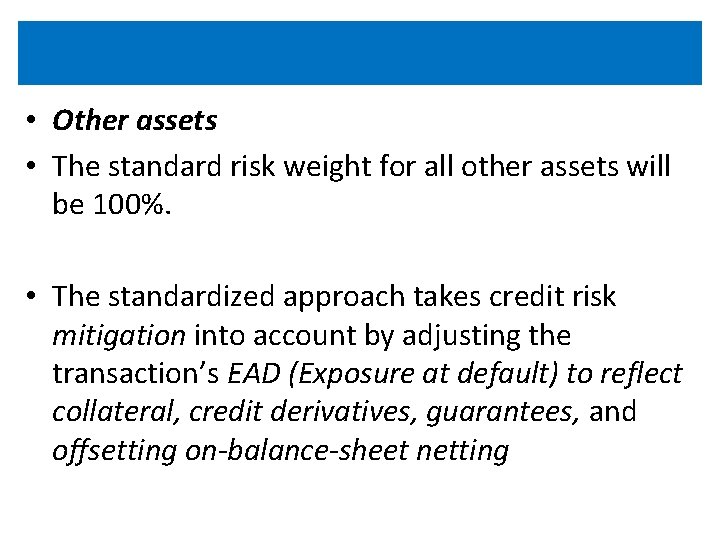
• Other assets • The standard risk weight for all other assets will be 100%. • The standardized approach takes credit risk mitigation into account by adjusting the transaction’s EAD (Exposure at default) to reflect collateral, credit derivatives, guarantees, and offsetting on-balance-sheet netting
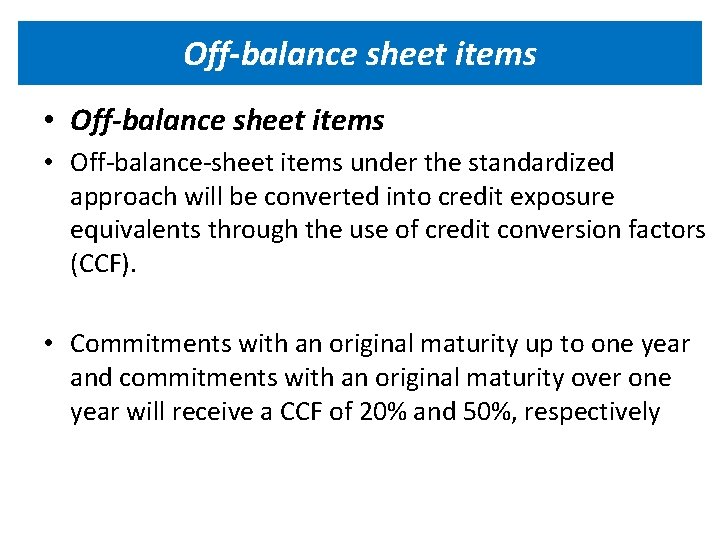
Off-balance sheet items • Off-balance-sheet items under the standardized approach will be converted into credit exposure equivalents through the use of credit conversion factors (CCF). • Commitments with an original maturity up to one year and commitments with an original maturity over one year will receive a CCF of 20% and 50%, respectively
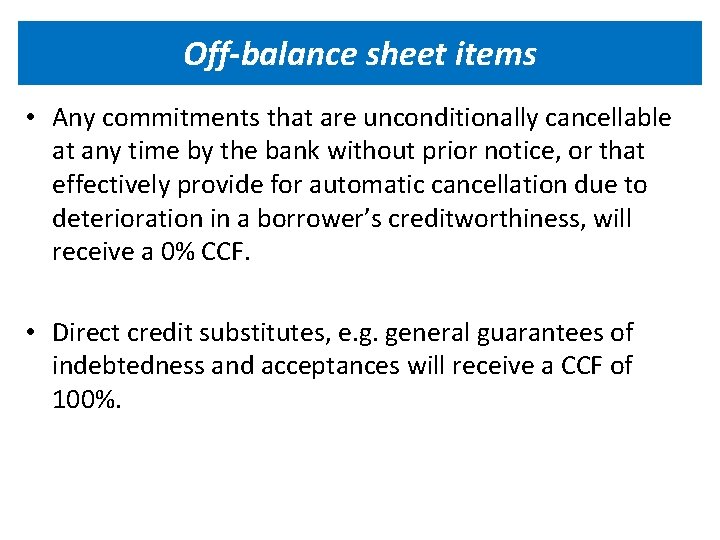
Off-balance sheet items • Any commitments that are unconditionally cancellable at any time by the bank without prior notice, or that effectively provide for automatic cancellation due to deterioration in a borrower’s creditworthiness, will receive a 0% CCF. • Direct credit substitutes, e. g. general guarantees of indebtedness and acceptances will receive a CCF of 100%.
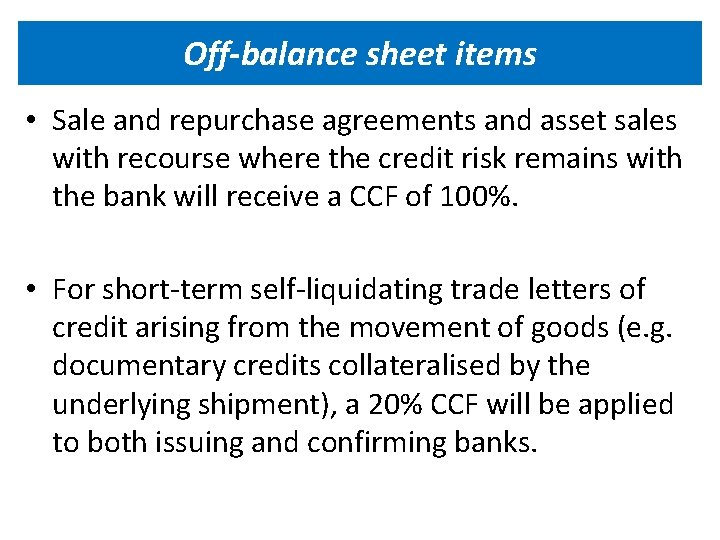
Off-balance sheet items • Sale and repurchase agreements and asset sales with recourse where the credit risk remains with the bank will receive a CCF of 100%. • For short-term self-liquidating trade letters of credit arising from the movement of goods (e. g. documentary credits collateralised by the underlying shipment), a 20% CCF will be applied to both issuing and confirming banks.
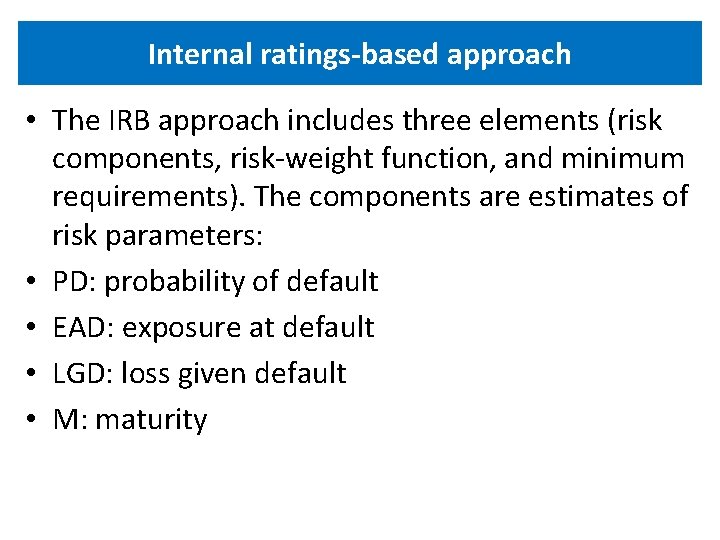
Internal ratings-based approach • The IRB approach includes three elements (risk components, risk-weight function, and minimum requirements). The components are estimates of risk parameters: • PD: probability of default • EAD: exposure at default • LGD: loss given default • M: maturity
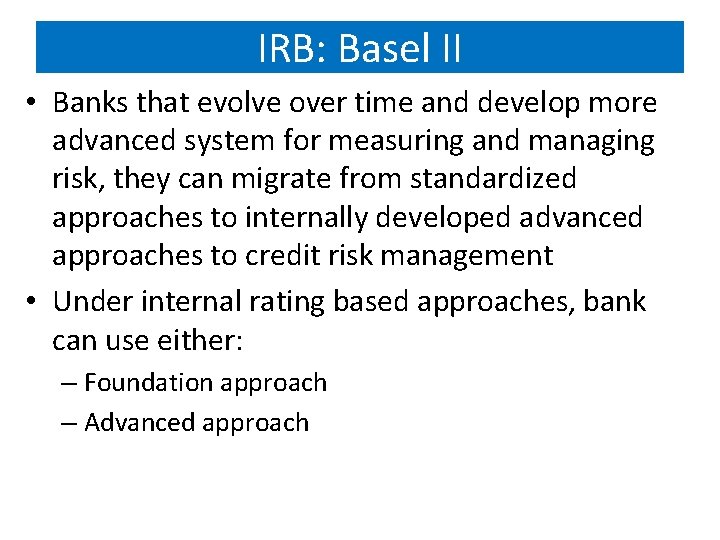
IRB: Basel II • Banks that evolve over time and develop more advanced system for measuring and managing risk, they can migrate from standardized approaches to internally developed advanced approaches to credit risk management • Under internal rating based approaches, bank can use either: – Foundation approach – Advanced approach
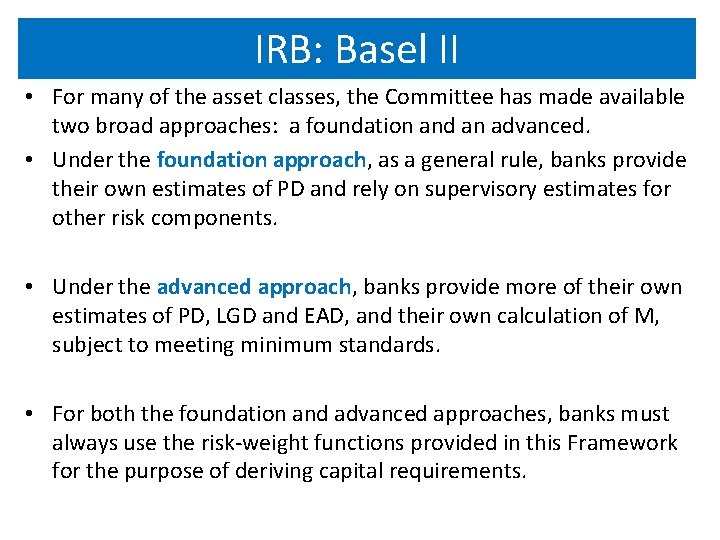
IRB: Basel II • For many of the asset classes, the Committee has made available two broad approaches: a foundation and an advanced. • Under the foundation approach, as a general rule, banks provide their own estimates of PD and rely on supervisory estimates for other risk components. • Under the advanced approach, banks provide more of their own estimates of PD, LGD and EAD, and their own calculation of M, subject to meeting minimum standards. • For both the foundation and advanced approaches, banks must always use the risk-weight functions provided in this Framework for the purpose of deriving capital requirements.
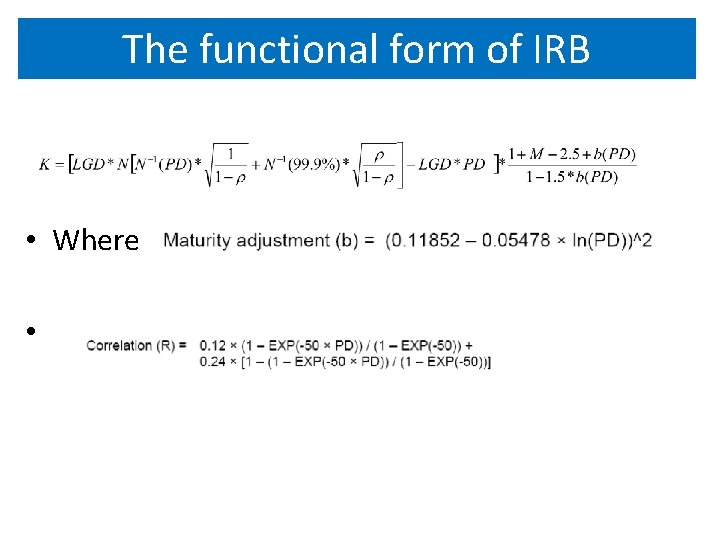
The functional form of IRB • Where •
Market risk assessment
Risk management lecture
01:640:244 lecture notes - lecture 15: plat, idah, farad
Inherent risks examples
Credit risk management and advisory goldman sachs
Credit risk
Christoffersen elements of financial risk management
Crm credit risk management
Credit risk management framework
Key risk indicators financial risk management
Risk map risk management
This can be avoided by giving credit where credit is due.
Hedge fund credit risk
Anz risk management framework
Lending policies and procedures managing credit risk
Credit risk in international trade
Measuring and managing credit risk
Quantifying credit risk
Hedging credit risk with options
Ncua aires questionnaire
Risk projection
Risk avoidance
Absolute risk vs relative risk
Residual risk and secondary risk pmp
Ar = ir x cr x dr
Absolute risk vs relative risk
Activity sheet 2: to risk or not to risk?
Risk classification system
Pembiayaan risiko (risk financing)
The biggest risk is not taking any risks
Business risk vs audit risk
Business risk and financial risk leverage
Relative risk
Relative risk
Project procurement management lecture notes
Strategic management lecture
4 p's of project management in software engineering
Project quality management lecture notes
Financial management lecture