Knitter Fast Resilient SingleUser Indoor Floor Plan Construction
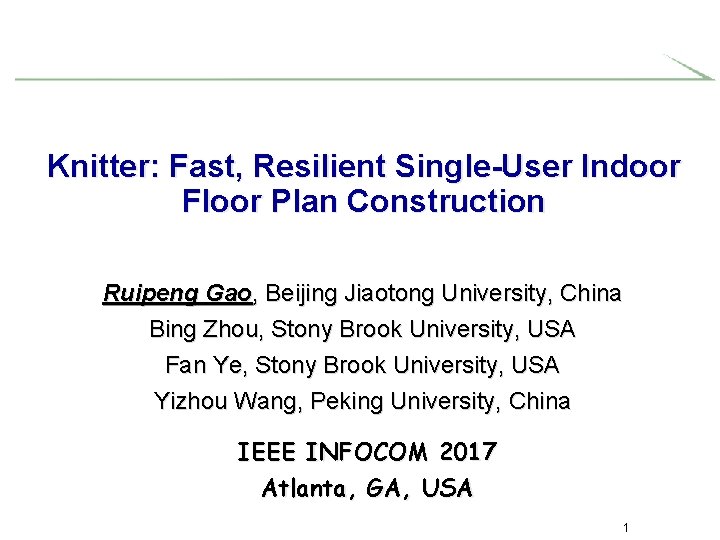
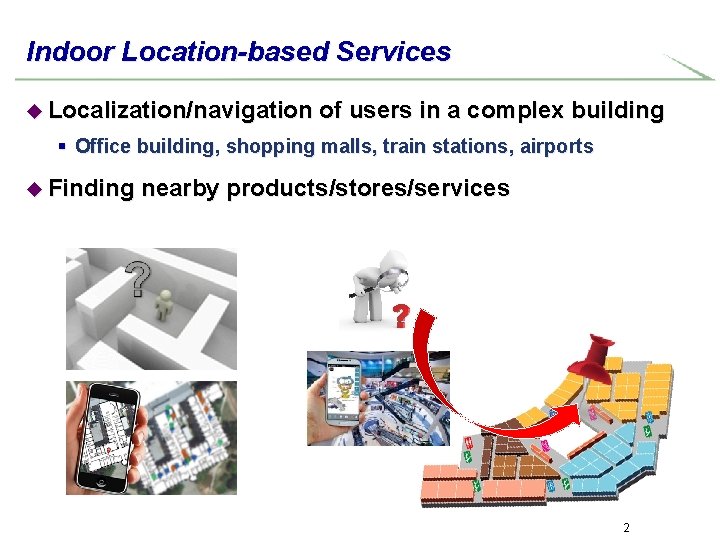
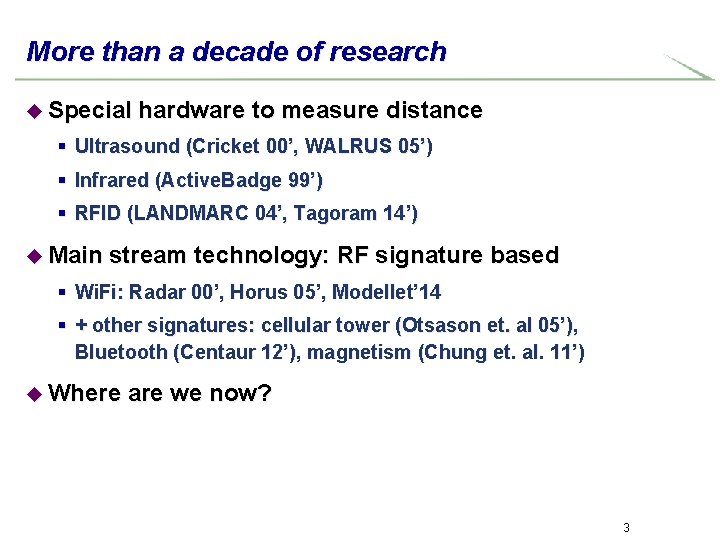
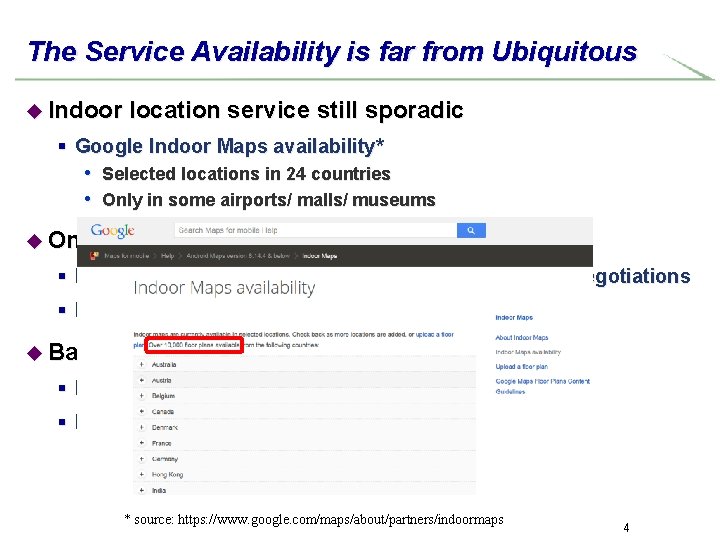
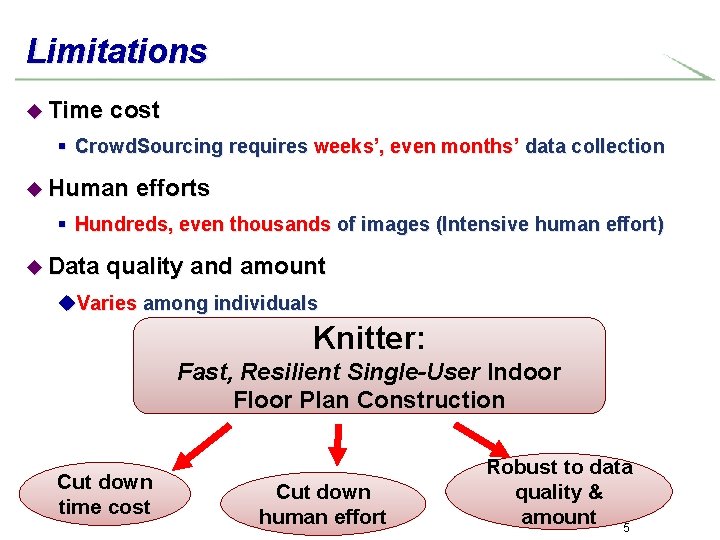
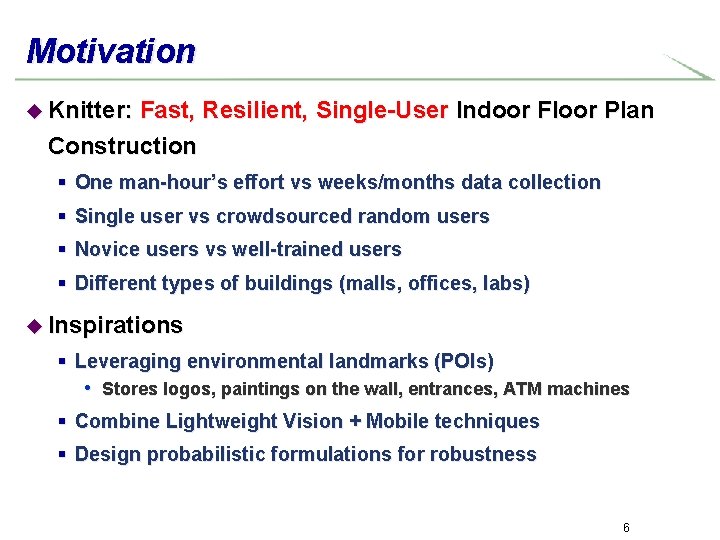
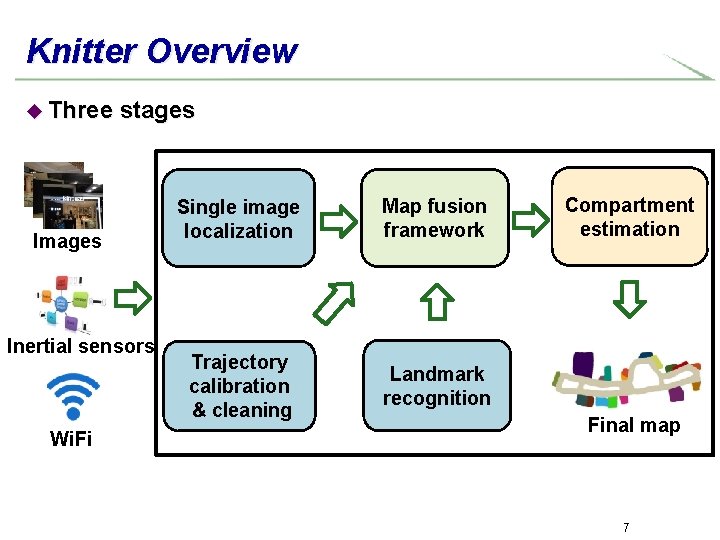
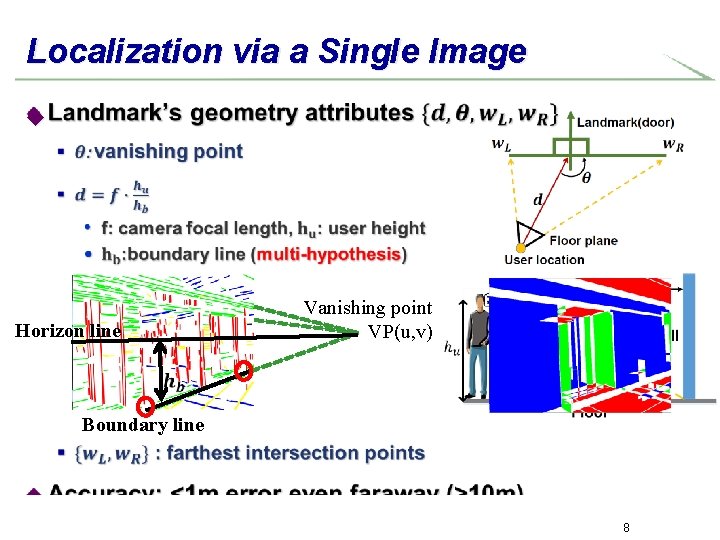
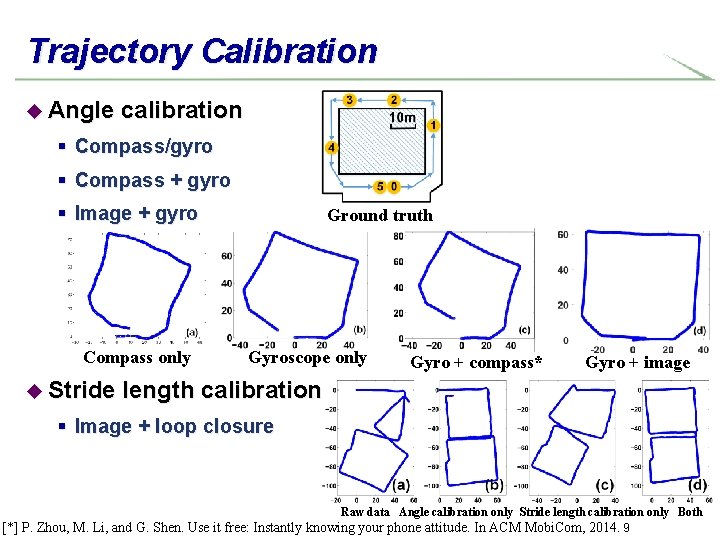
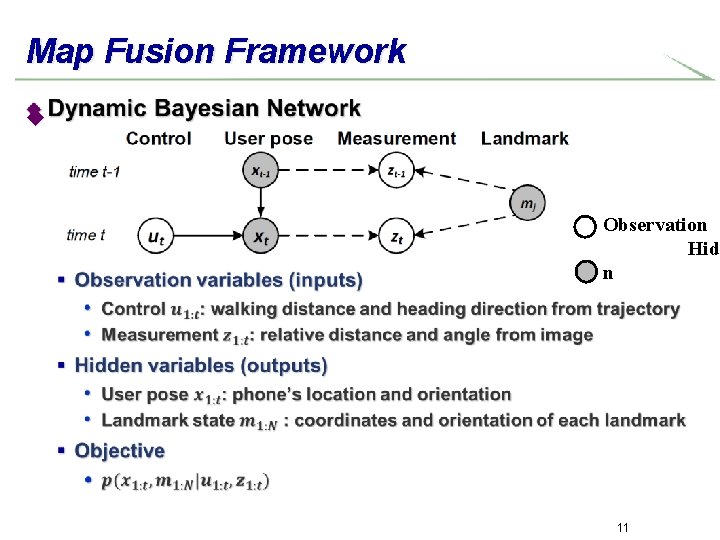
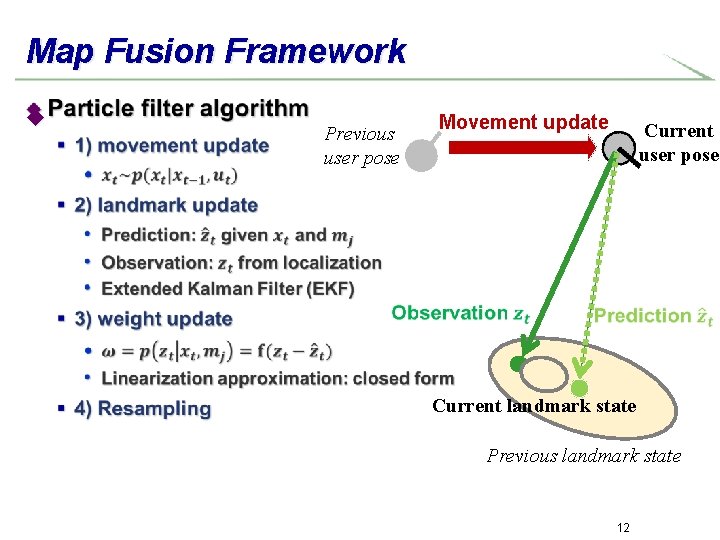
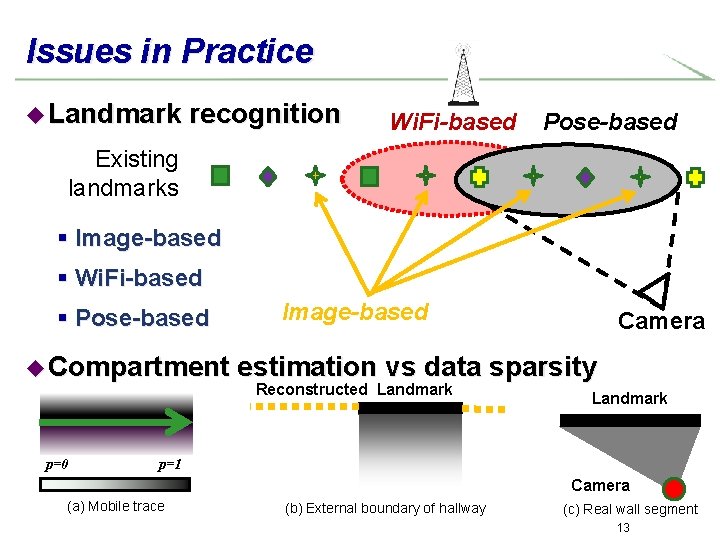
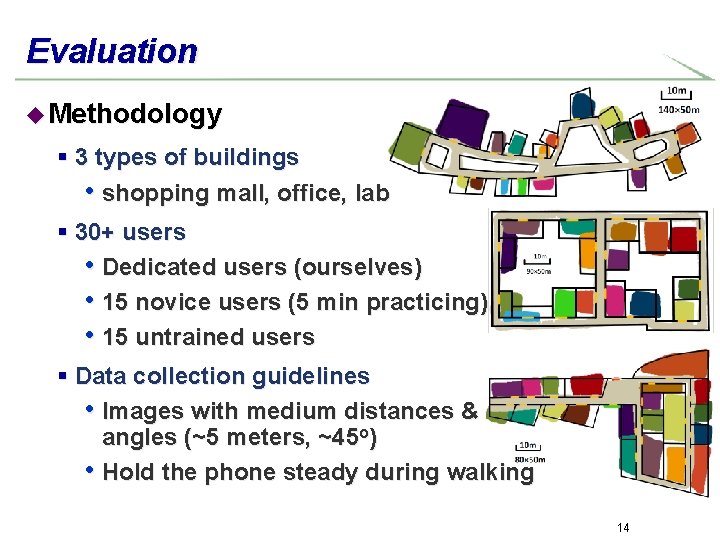
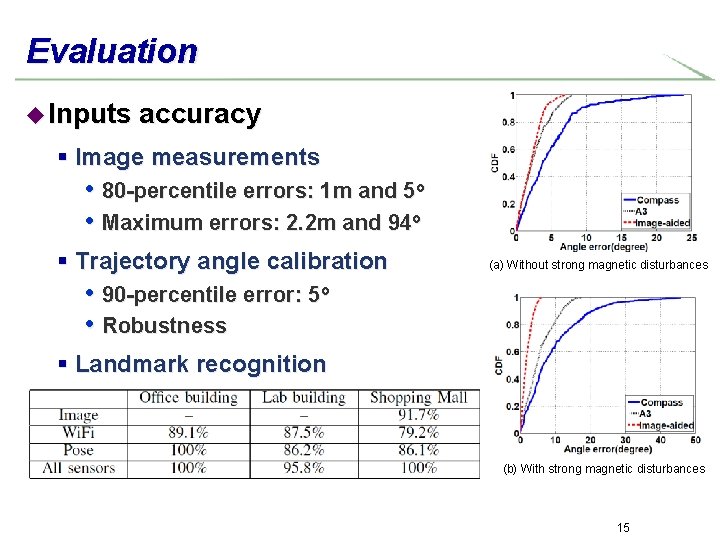
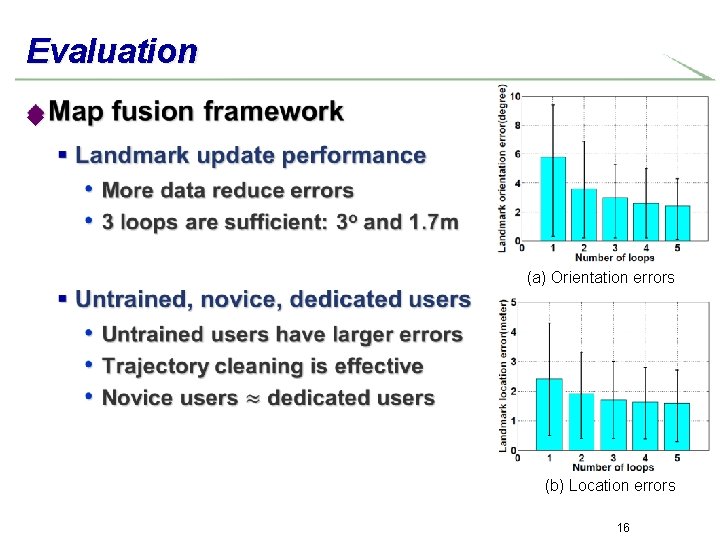
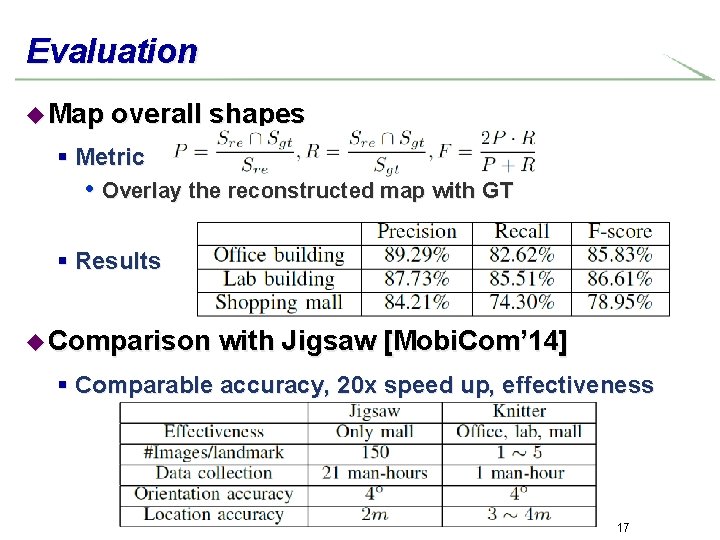
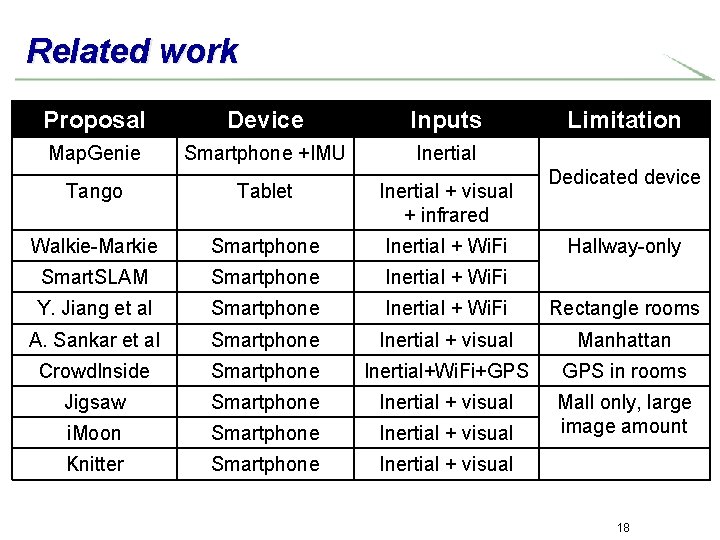
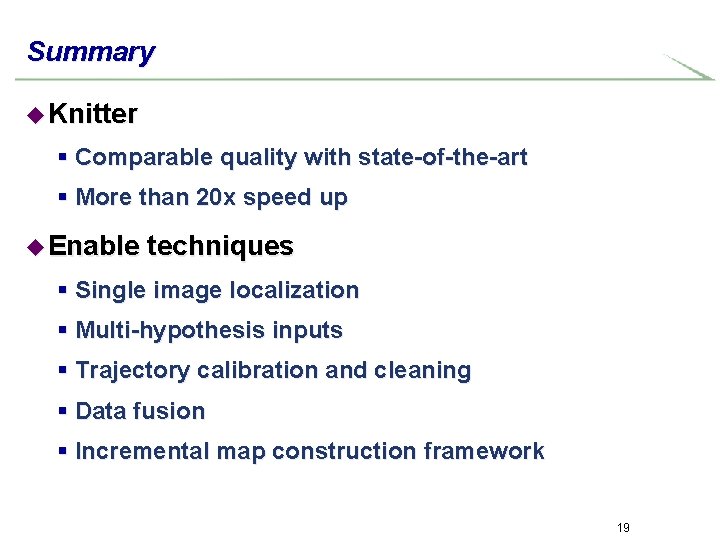
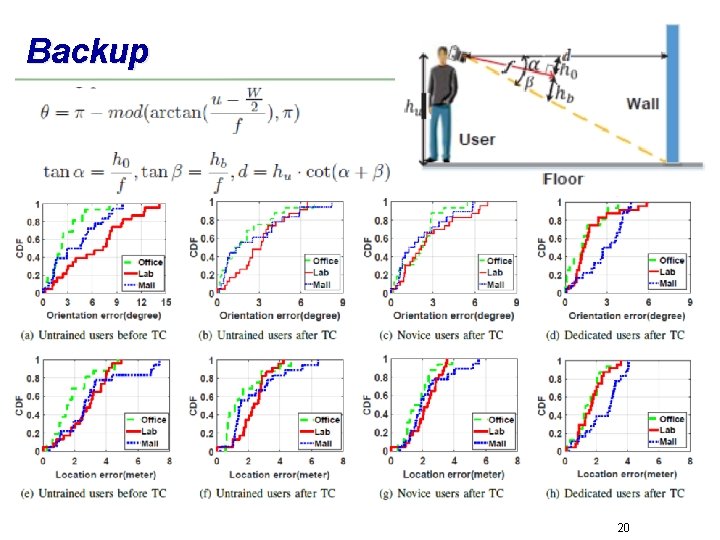
- Slides: 19
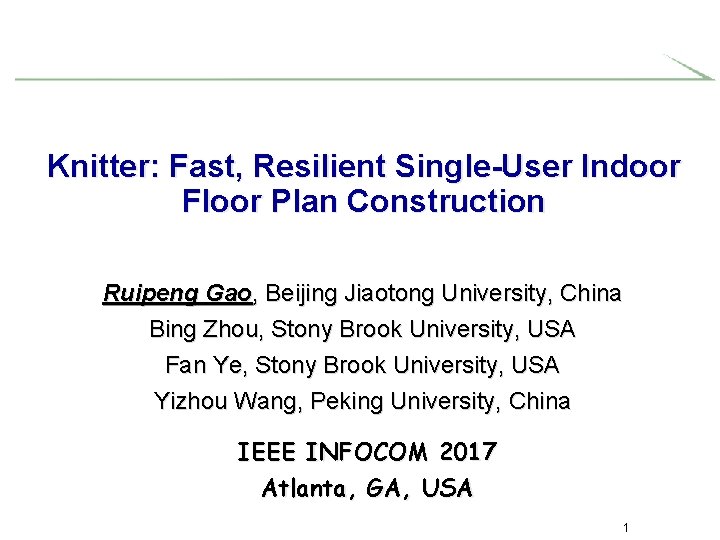
Knitter: Fast, Resilient Single-User Indoor Floor Plan Construction Ruipeng Gao, Beijing Jiaotong University, China Bing Zhou, Stony Brook University, USA Fan Ye, Stony Brook University, USA Yizhou Wang, Peking University, China IEEE INFOCOM 2017 Atlanta, GA, USA 1
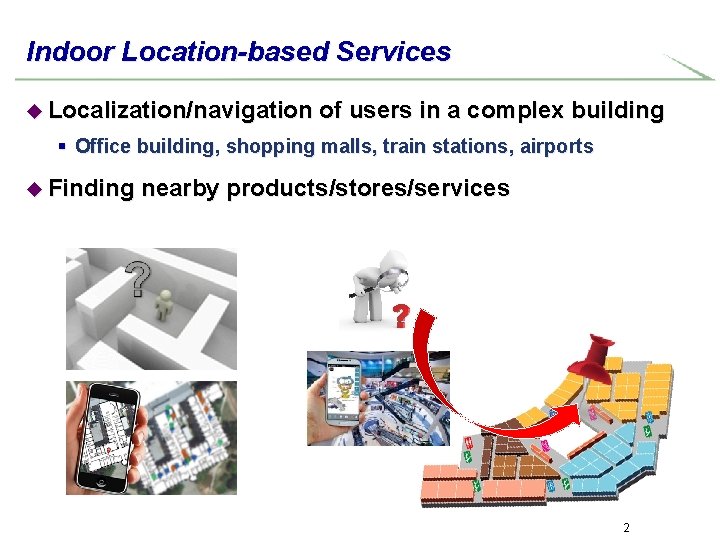
Indoor Location-based Services u Localization/navigation of users in a complex building § Office building, shopping malls, train stations, airports u Finding nearby products/stores/services 2
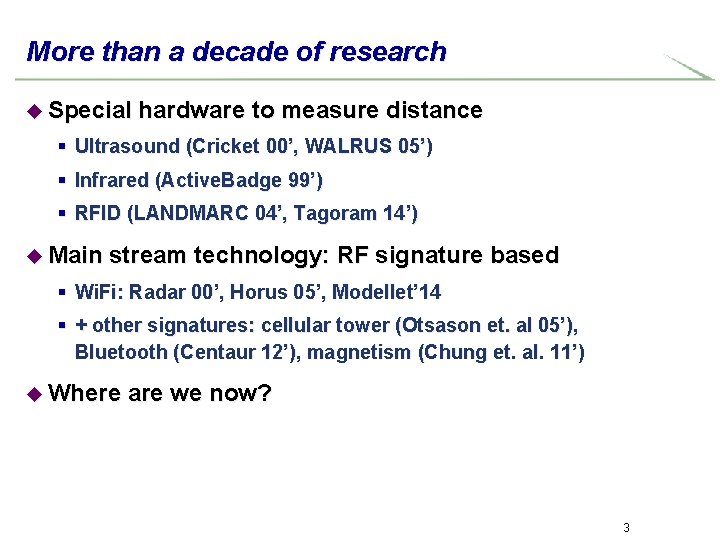
More than a decade of research u Special hardware to measure distance § Ultrasound (Cricket 00’, WALRUS 05’) § Infrared (Active. Badge 99’) § RFID (LANDMARC 04’, Tagoram 14’) u Main stream technology: RF signature based § Wi. Fi: Radar 00’, Horus 05’, Modellet’ 14 § + other signatures: cellular tower (Otsason et. al 05’), Bluetooth (Centaur 12’), magnetism (Chung et. al. 11’) u Where are we now? 3
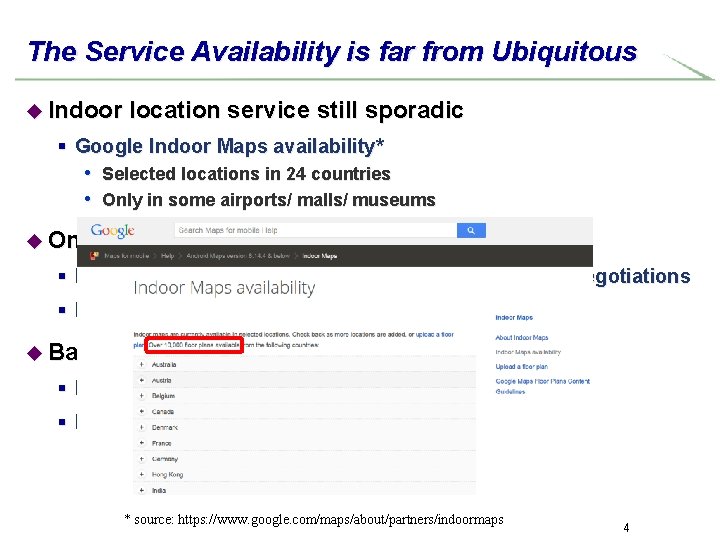
The Service Availability is far from Ubiquitous u Indoor location service still sporadic § Google Indoor Maps availability* • Selected locations in 24 countries • Only in some airports/ malls/ museums u One major obstacle: lacking of floor maps § Need effort-intensive and time-consuming business negotiations § Hire dedicated personnel u Background: crowdsourcing based § Mobile traces & Wi. Fi signatures: noisy § Images: large amounts * source: https: //www. google. com/maps/about/partners/indoormaps 4
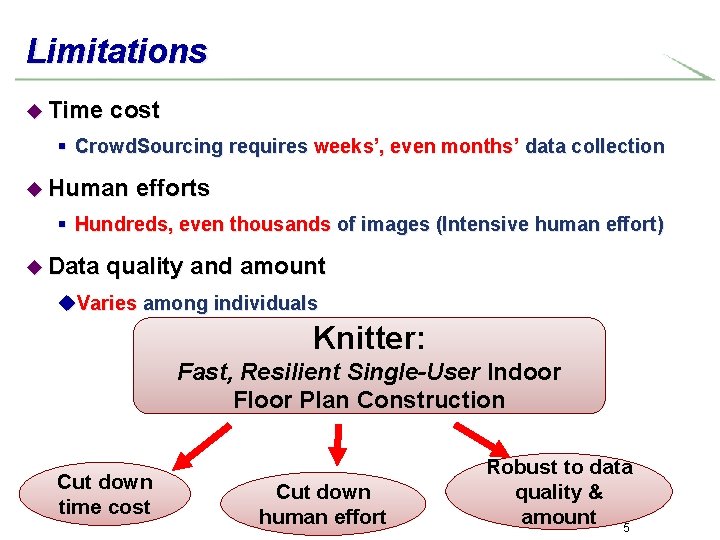
Limitations u Time cost § Crowd. Sourcing requires weeks’, even months’ data collection u Human efforts § Hundreds, even thousands of images (Intensive human effort) u Data quality and amount u. Varies among individuals Knitter: Fast, Resilient Single-User Indoor Floor Plan Construction Cut down time cost Cut down human effort Robust to data quality & amount 5
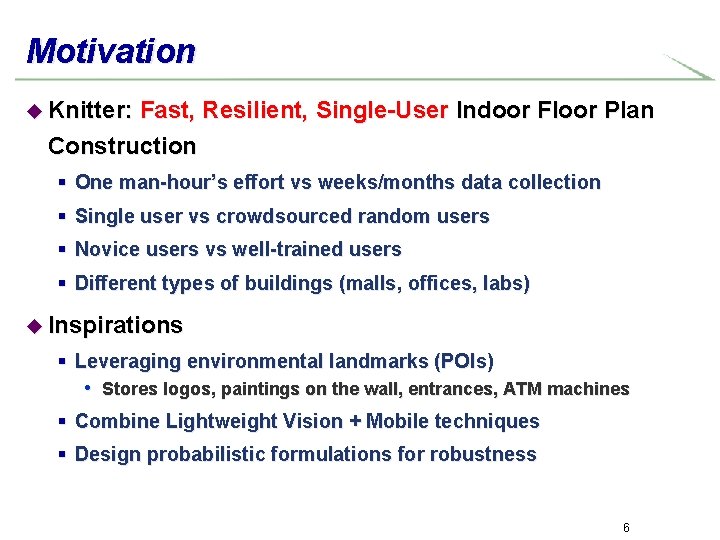
Motivation u Knitter: Fast, Resilient, Single-User Indoor Floor Plan Construction § One man-hour’s effort vs weeks/months data collection § Single user vs crowdsourced random users § Novice users vs well-trained users § Different types of buildings (malls, offices, labs) u Inspirations § Leveraging environmental landmarks (POIs) • Stores logos, paintings on the wall, entrances, ATM machines § Combine Lightweight Vision + Mobile techniques § Design probabilistic formulations for robustness 6
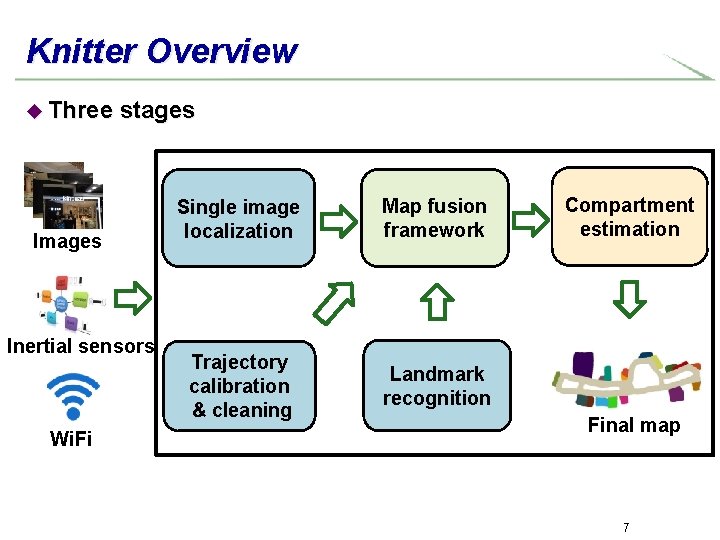
Knitter Overview u Three stages Images Inertial sensors Wi. Fi Single image localization Map fusion framework Trajectory calibration & cleaning Landmark recognition Compartment estimation Final map 7
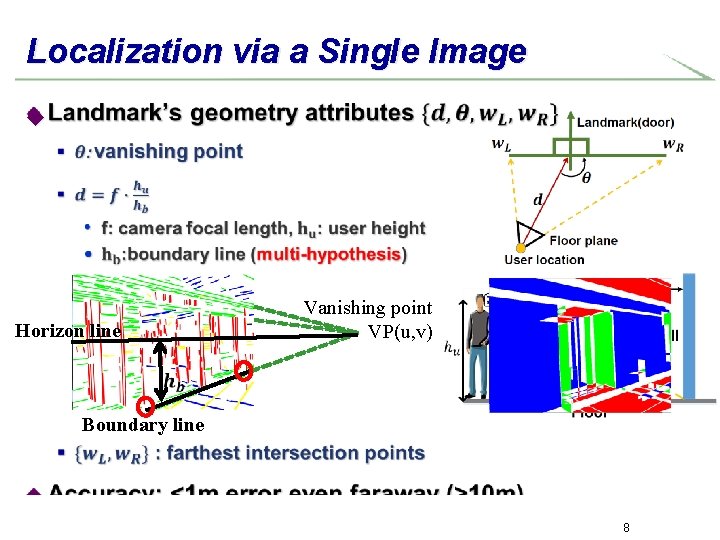
Localization via a Single Image u Vanishing point VP(u, v) Horizon line Boundary line 8
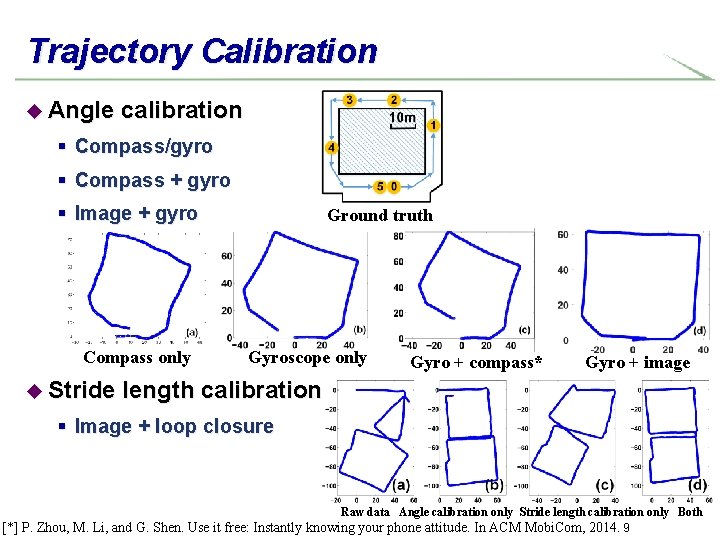
Trajectory Calibration u Angle calibration § Compass/gyro § Compass + gyro § Image + gyro Compass only Ground truth Gyroscope only Gyro + compass* Gyro + image u Stride length calibration § Image + loop closure Raw data Angle calibration only Stride length calibration only Both [*] P. Zhou, M. Li, and G. Shen. Use it free: Instantly knowing your phone attitude. In ACM Mobi. Com, 2014. 9
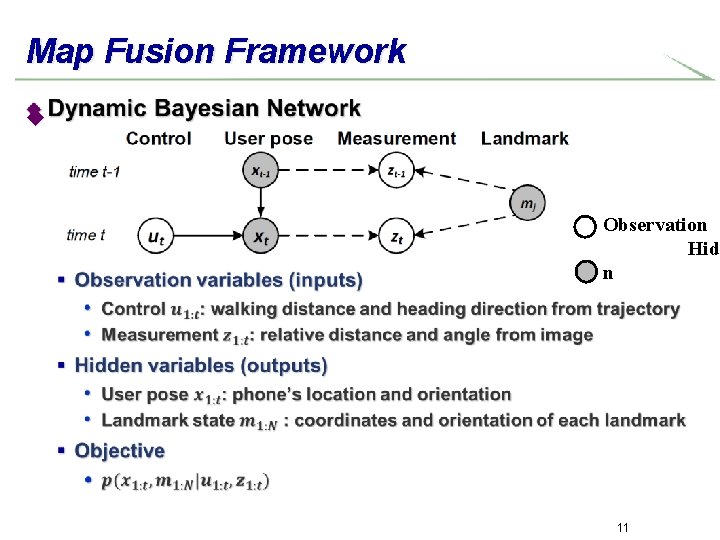
Map Fusion Framework u Observation Hidd n 11
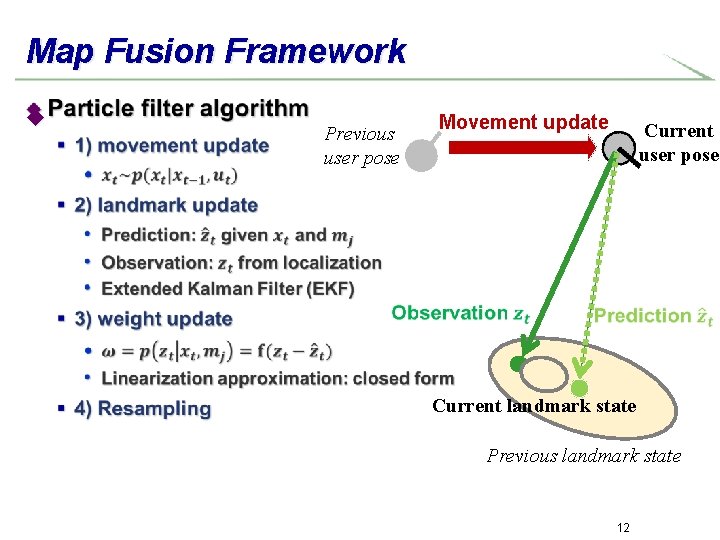
Map Fusion Framework u Previous user pose Movement update Current user pose Current landmark state Previous landmark state 12
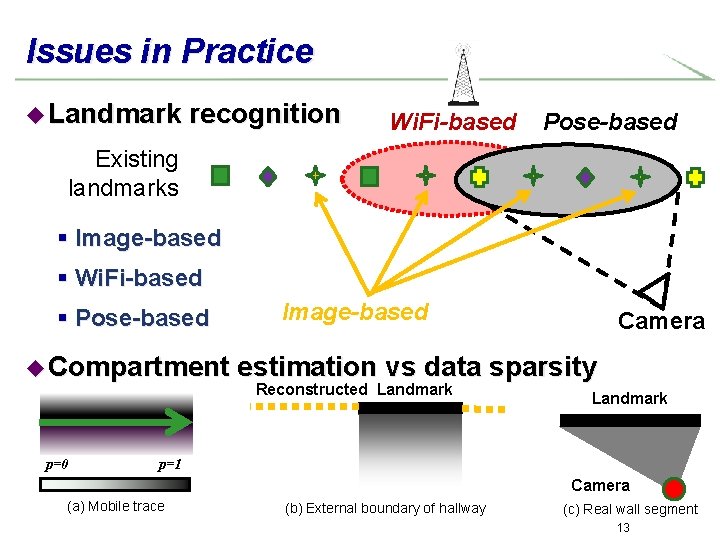
Issues in Practice u Landmark recognition Wi. Fi-based Pose-based Existing landmarks § Image-based § Wi. Fi-based § Pose-based Image-based Camera u Compartment estimation vs data sparsity Reconstructed Landmark p=0 Landmark p=1 Camera (a) Mobile trace (b) External boundary of hallway (c) Real wall segment 13
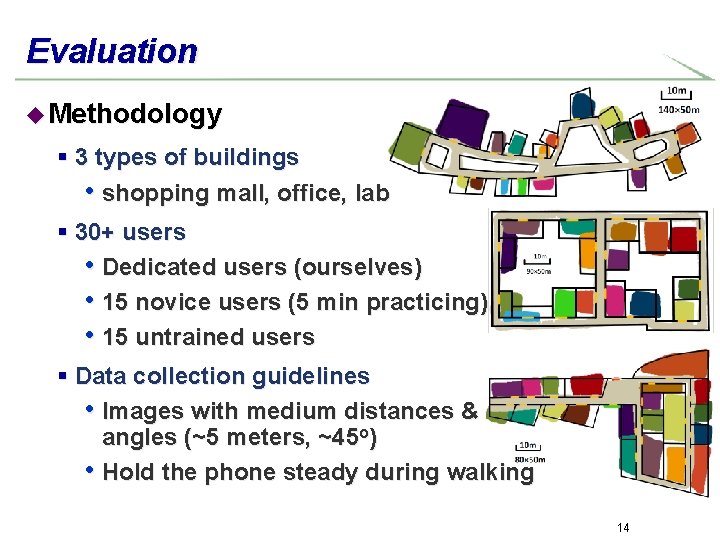
Evaluation u Methodology § 3 types of buildings • shopping mall, office, lab § 30+ users • Dedicated users (ourselves) • 15 novice users (5 min practicing) • 15 untrained users § Data collection guidelines • Images with medium distances & angles (~5 meters, ~45 o) • Hold the phone steady during walking 14
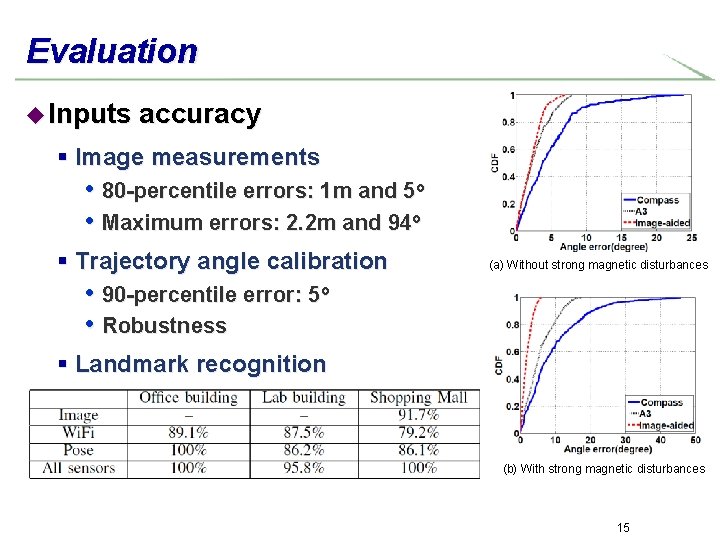
Evaluation u Inputs accuracy § Image measurements • 80 -percentile errors: 1 m and 5 o • Maximum errors: 2. 2 m and 94 o § Trajectory angle calibration (a) Without strong magnetic disturbances • 90 -percentile error: 5 o • Robustness § Landmark recognition (b) With strong magnetic disturbances 15
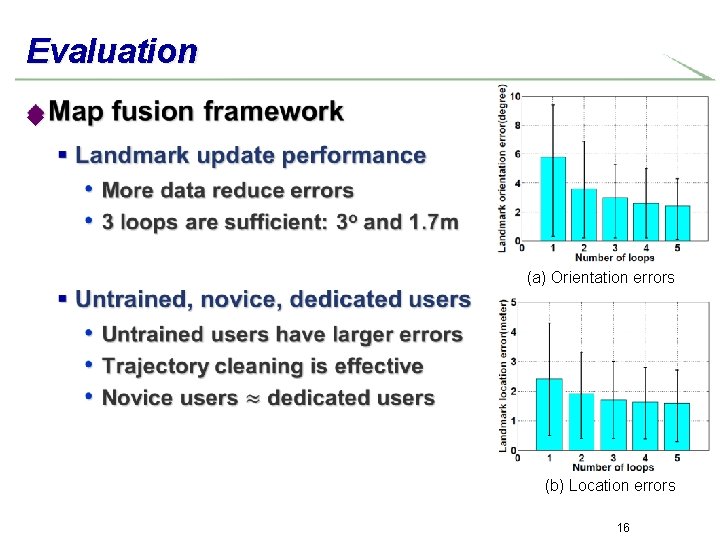
Evaluation u (a) Orientation errors (b) Location errors 16
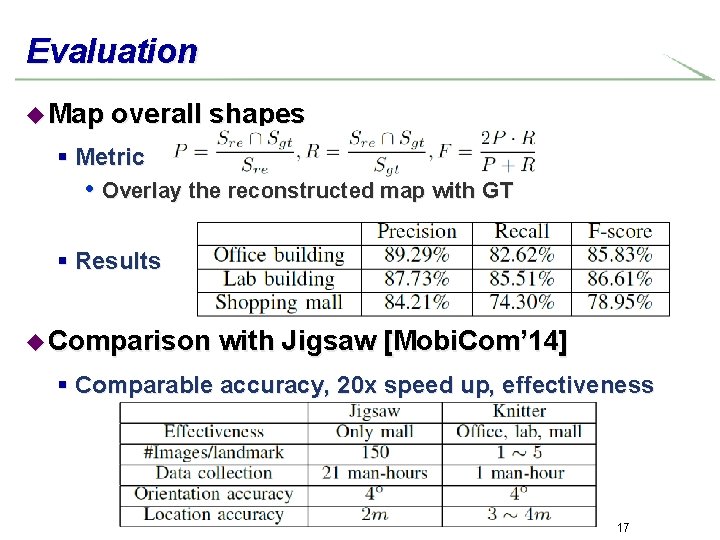
Evaluation u Map overall shapes § Metric • Overlay the reconstructed map with GT § Results u Comparison with Jigsaw [Mobi. Com’ 14] § Comparable accuracy, 20 x speed up, effectiveness 17
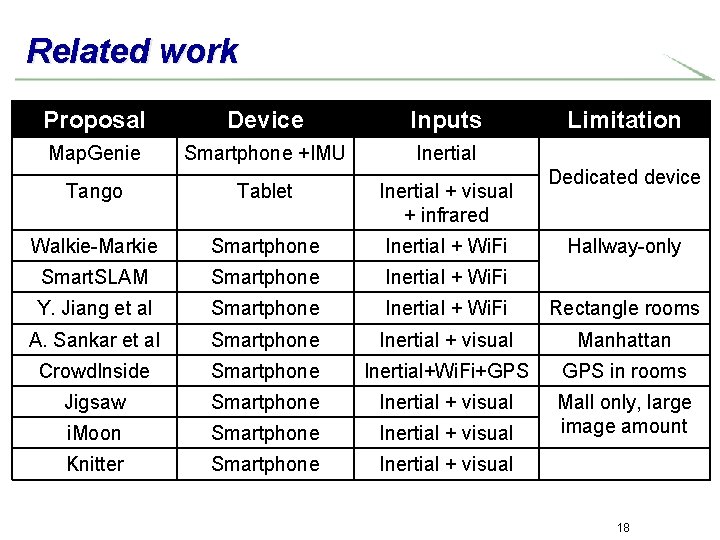
Related work Proposal Device Inputs Map. Genie Smartphone +IMU Inertial Limitation Dedicated device Tango Tablet Inertial + visual + infrared Walkie-Markie Smartphone Inertial + Wi. Fi Smart. SLAM Smartphone Inertial + Wi. Fi Y. Jiang et al Smartphone Inertial + Wi. Fi Rectangle rooms A. Sankar et al Smartphone Inertial + visual Manhattan Crowd. Inside Smartphone Inertial+Wi. Fi+GPS in rooms Jigsaw Smartphone Inertial + visual i. Moon Smartphone Inertial + visual Mall only, large image amount Knitter Smartphone Inertial + visual Hallway-only 18
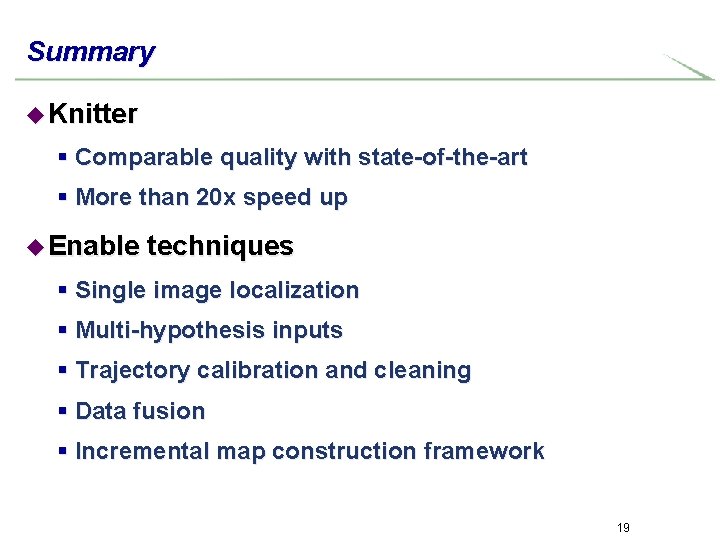
Summary u Knitter § Comparable quality with state-of-the-art § More than 20 x speed up u Enable techniques § Single image localization § Multi-hypothesis inputs § Trajectory calibration and cleaning § Data fusion § Incremental map construction framework 19
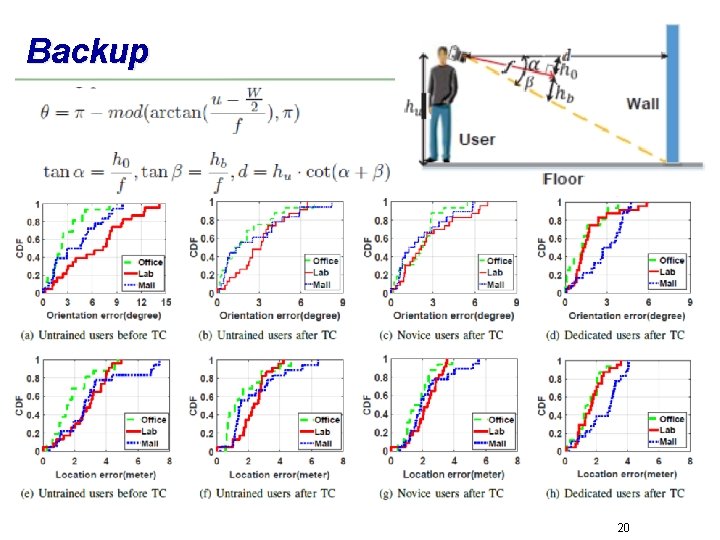
Backup 20
Felix huerkamp
Non acid fast bacteria
Acid fast and non acid fast bacteria
Fast bvh construction on gpus
Platform floor construction
Honeycomb sleeper wall
Rhmd: evasion-resilient hardware malware detectors
Resilient pnt
Pnt gnss
Resilient attitude
It came on great oiled resilient striding legs
How can be more resilient
Supply chain risk register
How can more resilient
Col shape gingiva
How can be more resilient
How can be more resilient
Mark tinka
Token ring and resilient packet ring
Resilient distributed datasets