Forecasting Models3 et Method A 2 1 5
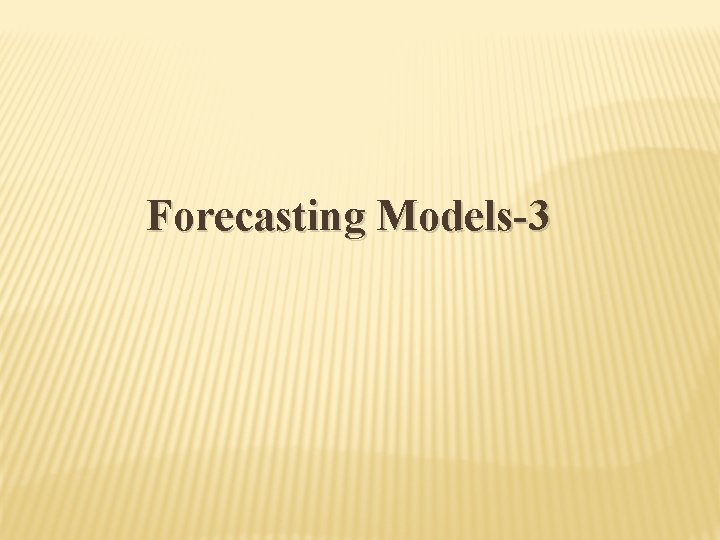
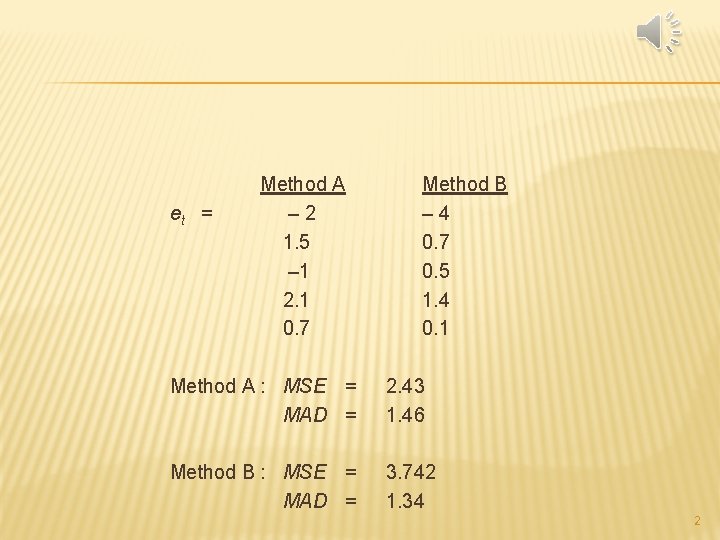
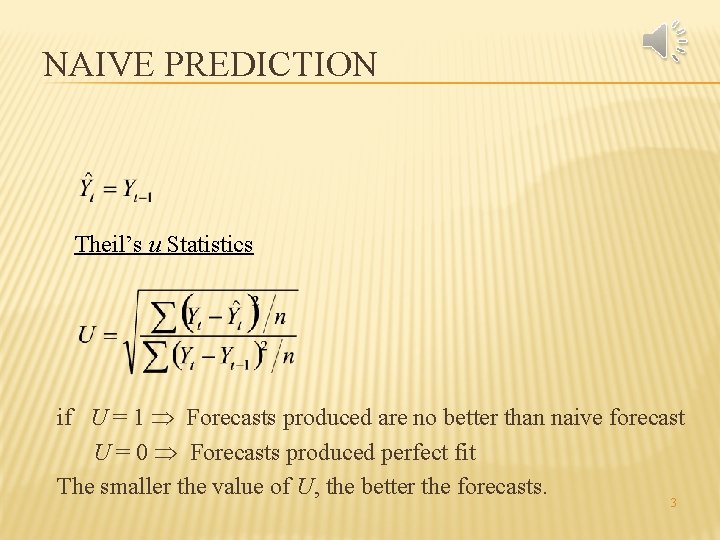
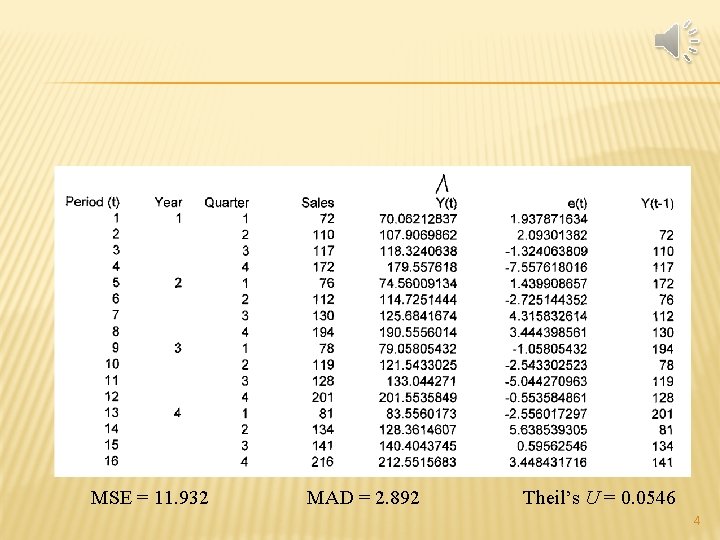
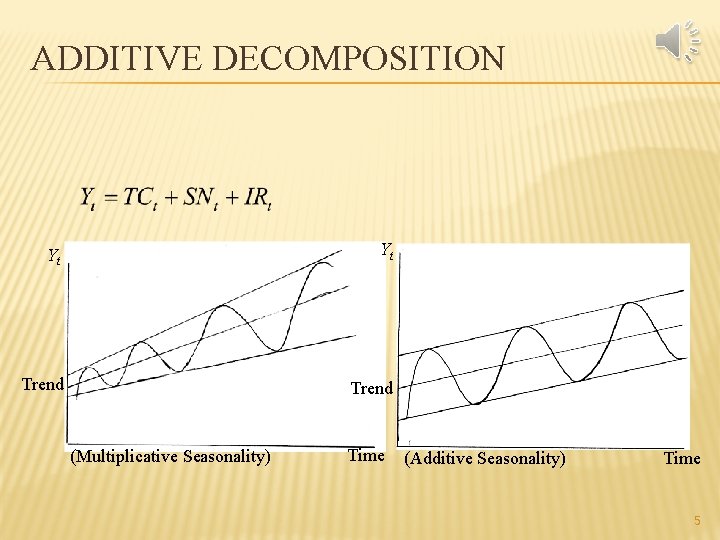
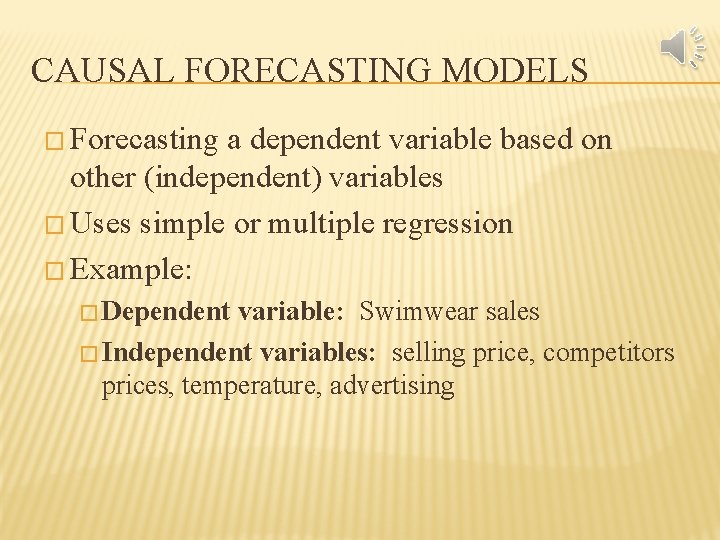
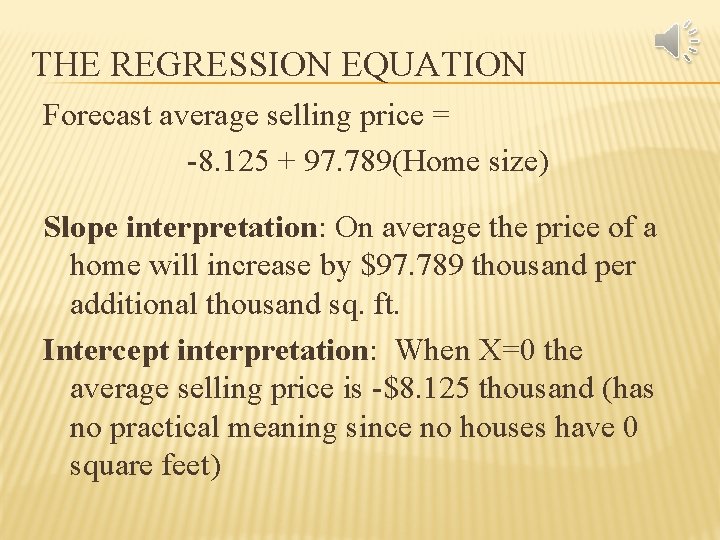
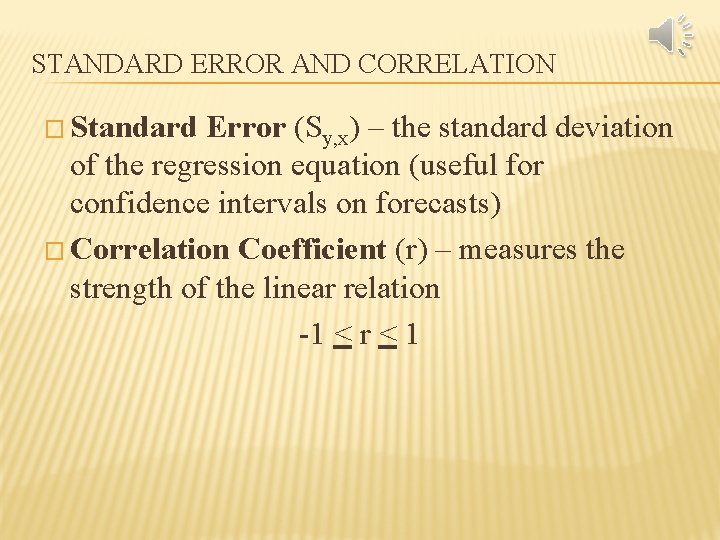
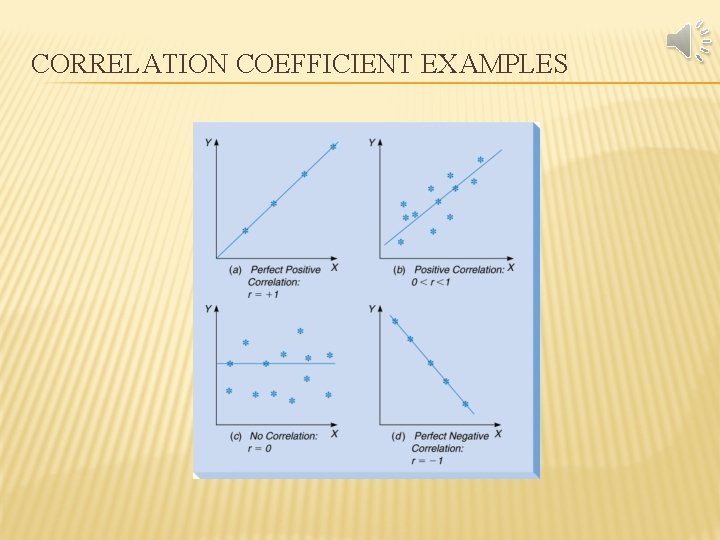
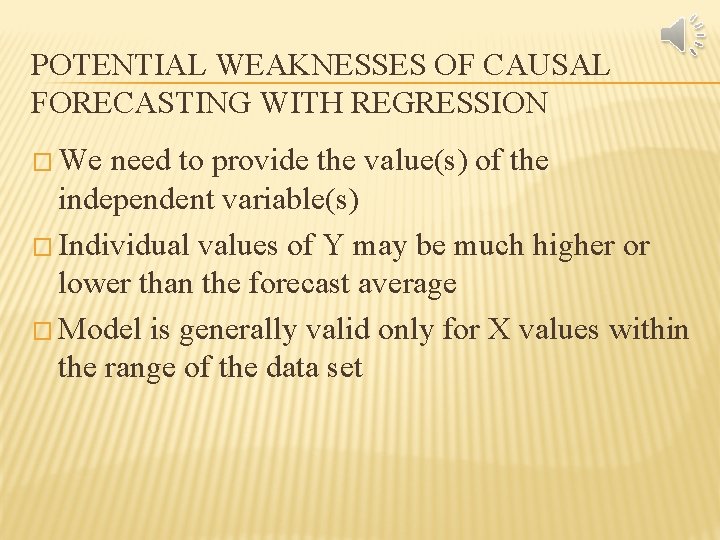
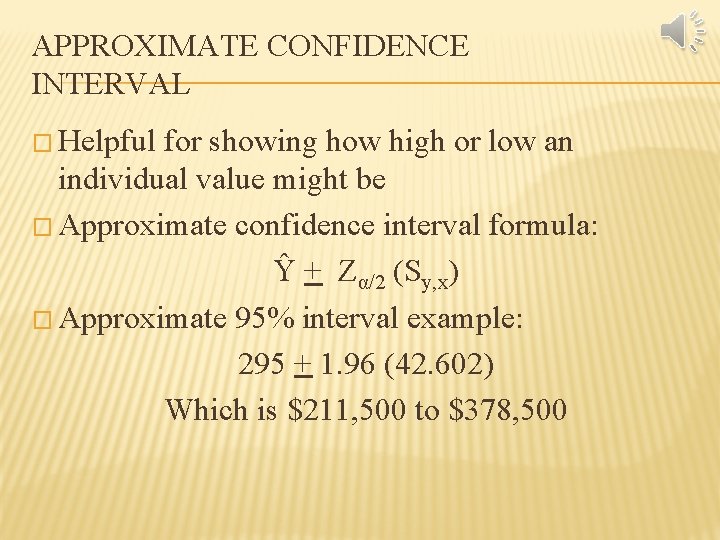
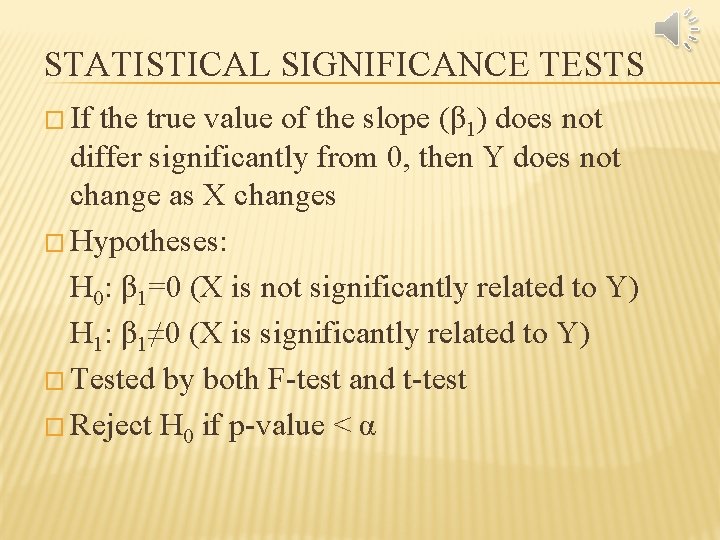
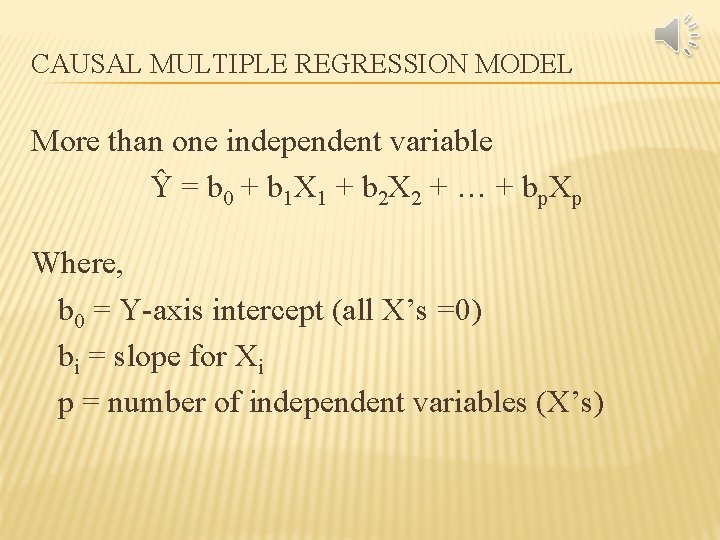
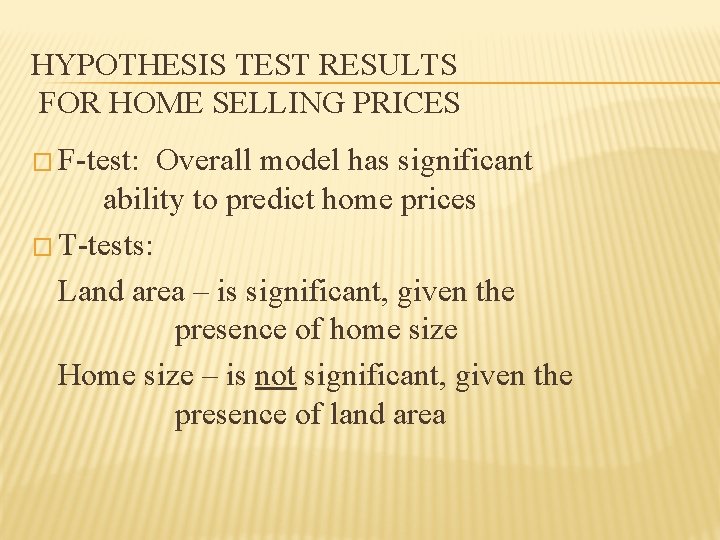
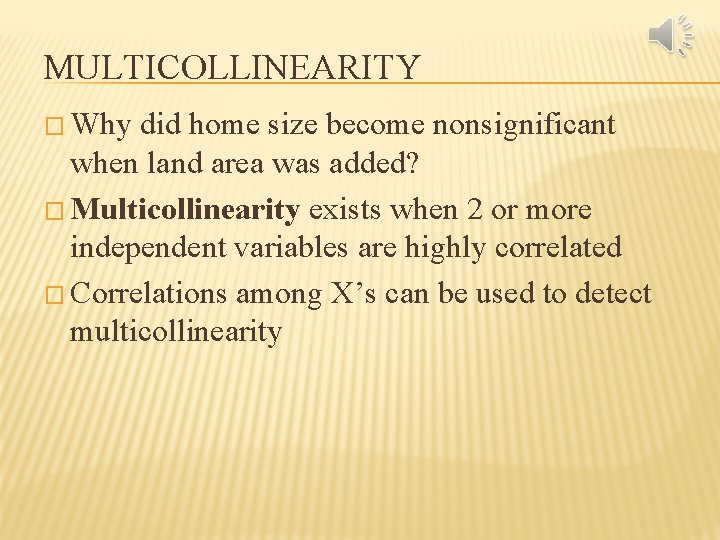
- Slides: 15
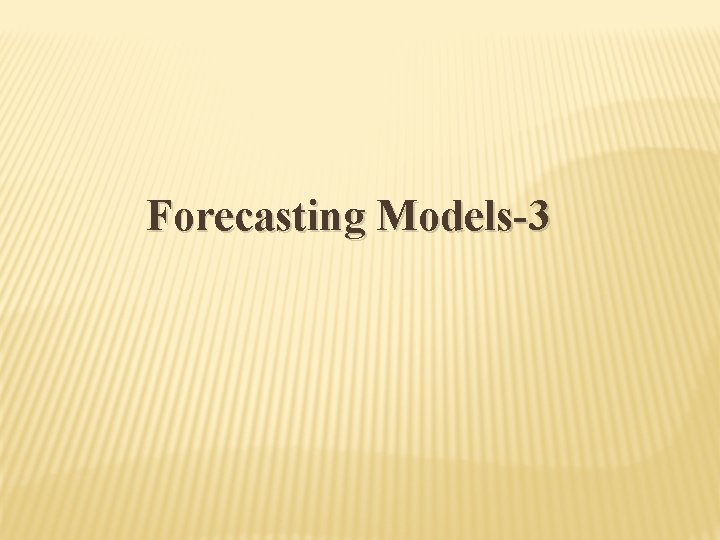
Forecasting Models-3
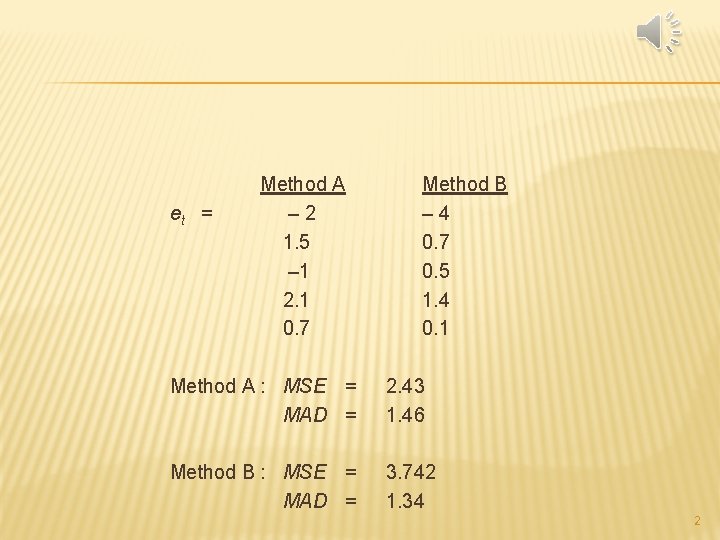
et = Method A – 2 1. 5 – 1 2. 1 0. 7 Method B – 4 0. 7 0. 5 1. 4 0. 1 Method A : MSE = MAD = 2. 43 1. 46 Method B : MSE = MAD = 3. 742 1. 34 2
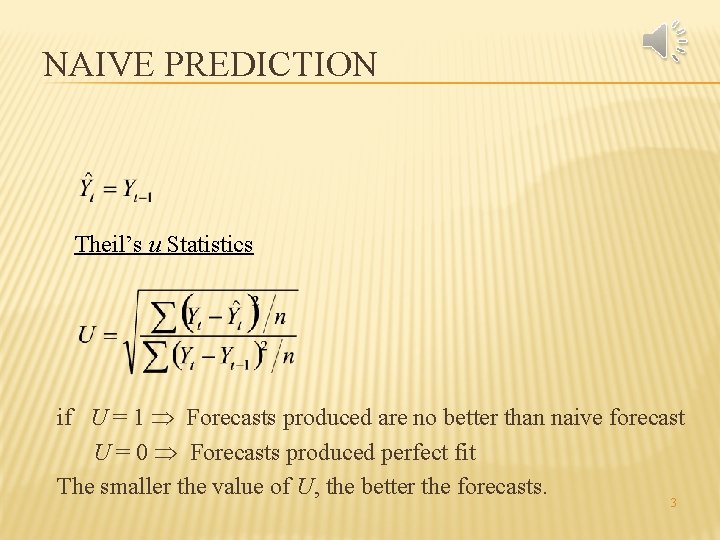
NAIVE PREDICTION Theil’s u Statistics if U = 1 Forecasts produced are no better than naive forecast U = 0 Forecasts produced perfect fit The smaller the value of U, the better the forecasts. 3
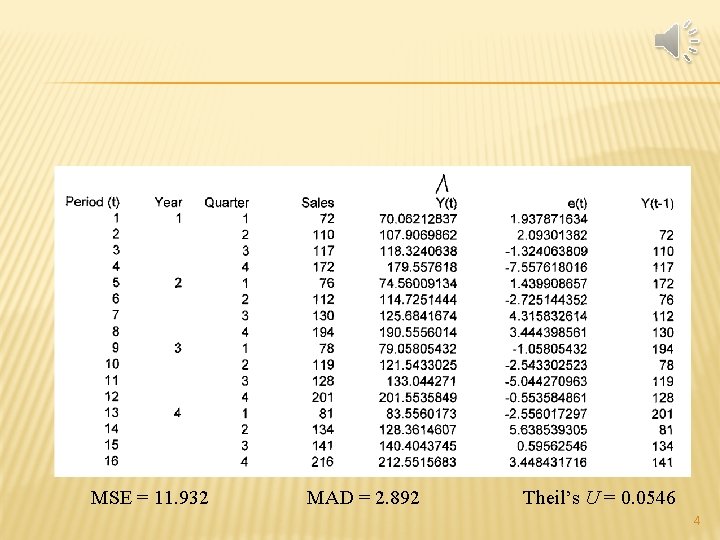
MSE = 11. 932 MAD = 2. 892 Theil’s U = 0. 0546 4
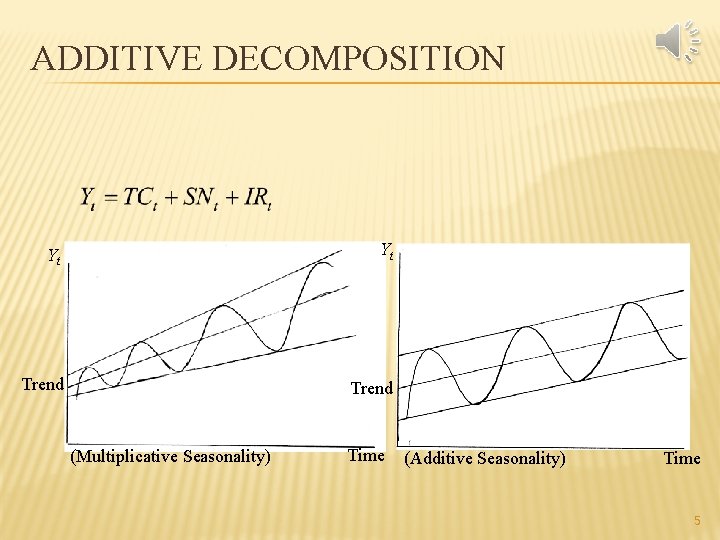
ADDITIVE DECOMPOSITION Yt Yt Trend (Multiplicative Seasonality) Time (Additive Seasonality) Time 5
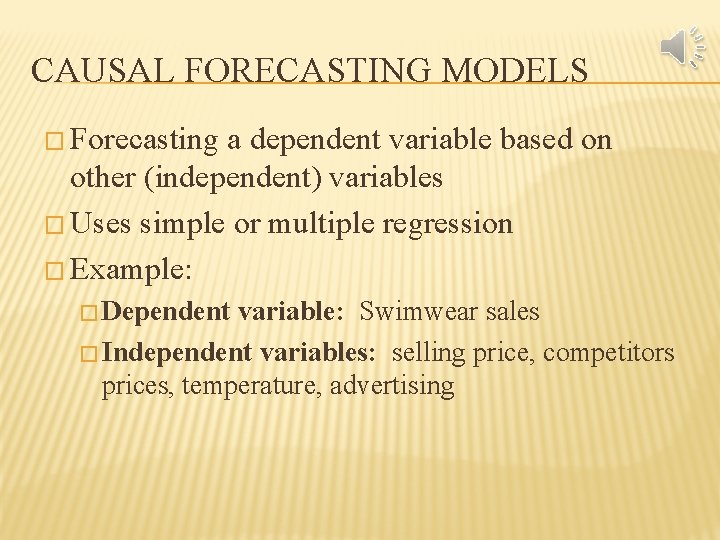
CAUSAL FORECASTING MODELS � Forecasting a dependent variable based on other (independent) variables � Uses simple or multiple regression � Example: � Dependent variable: Swimwear sales � Independent variables: selling price, competitors prices, temperature, advertising
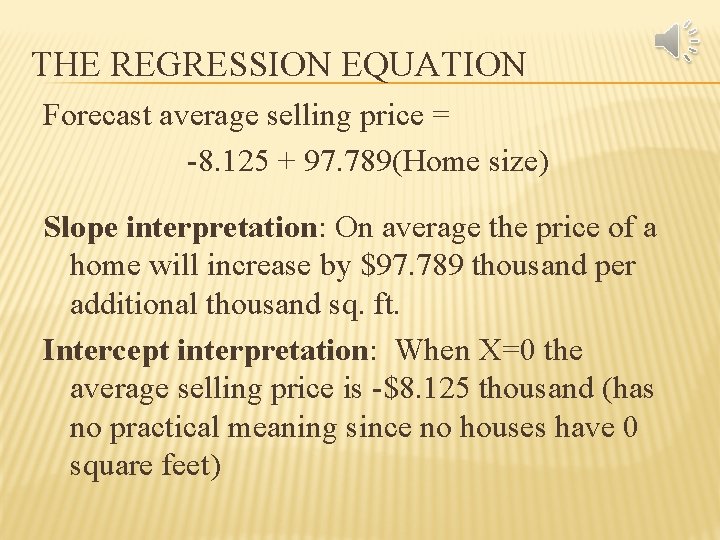
THE REGRESSION EQUATION Forecast average selling price = -8. 125 + 97. 789(Home size) Slope interpretation: On average the price of a home will increase by $97. 789 thousand per additional thousand sq. ft. Intercept interpretation: When X=0 the average selling price is -$8. 125 thousand (has no practical meaning since no houses have 0 square feet)
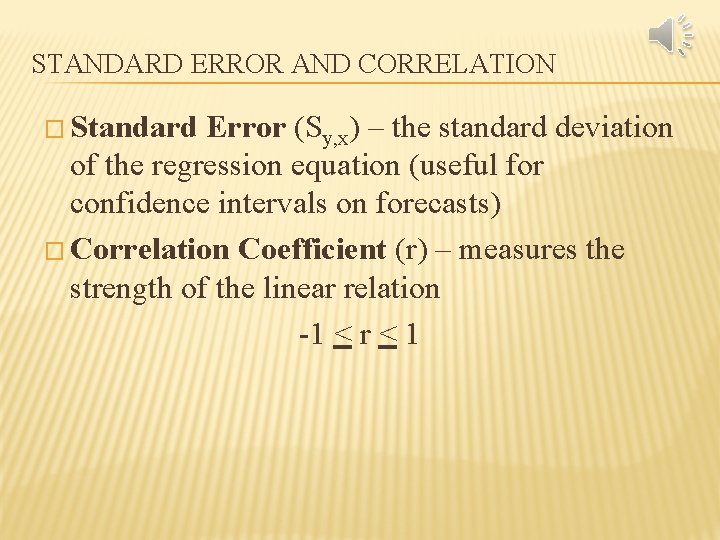
STANDARD ERROR AND CORRELATION � Standard Error (Sy, x) – the standard deviation of the regression equation (useful for confidence intervals on forecasts) � Correlation Coefficient (r) – measures the strength of the linear relation -1 < r < 1
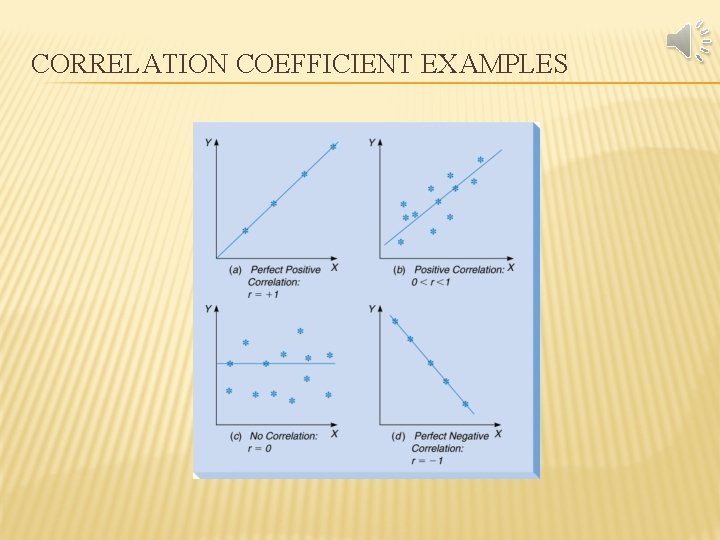
CORRELATION COEFFICIENT EXAMPLES
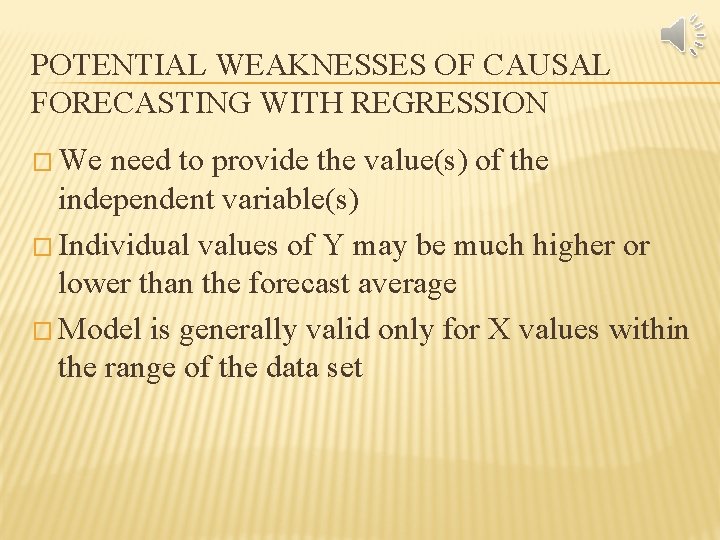
POTENTIAL WEAKNESSES OF CAUSAL FORECASTING WITH REGRESSION � We need to provide the value(s) of the independent variable(s) � Individual values of Y may be much higher or lower than the forecast average � Model is generally valid only for X values within the range of the data set
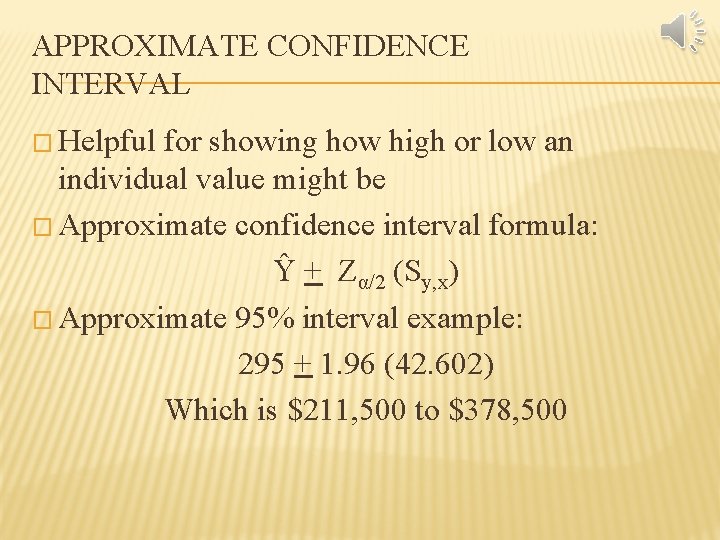
APPROXIMATE CONFIDENCE INTERVAL � Helpful for showing how high or low an individual value might be � Approximate confidence interval formula: Ŷ + Zα/2 (Sy, x) � Approximate 95% interval example: 295 + 1. 96 (42. 602) Which is $211, 500 to $378, 500
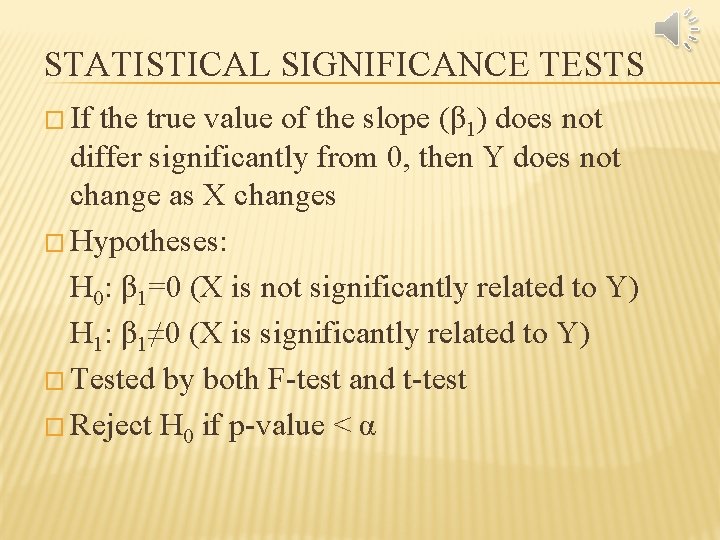
STATISTICAL SIGNIFICANCE TESTS � If the true value of the slope (β 1) does not differ significantly from 0, then Y does not change as X changes � Hypotheses: H 0: β 1=0 (X is not significantly related to Y) H 1: β 1≠ 0 (X is significantly related to Y) � Tested by both F-test and t-test � Reject H 0 if p-value < α
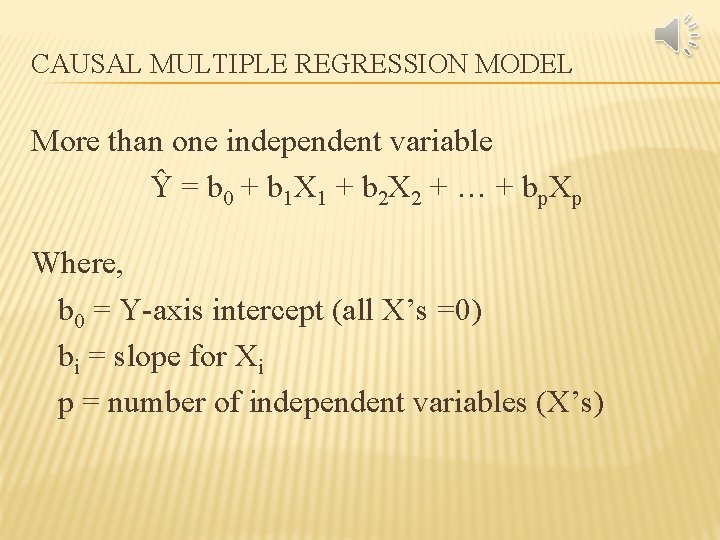
CAUSAL MULTIPLE REGRESSION MODEL More than one independent variable Ŷ = b 0 + b 1 X 1 + b 2 X 2 + … + bp. Xp Where, b 0 = Y-axis intercept (all X’s =0) bi = slope for Xi p = number of independent variables (X’s)
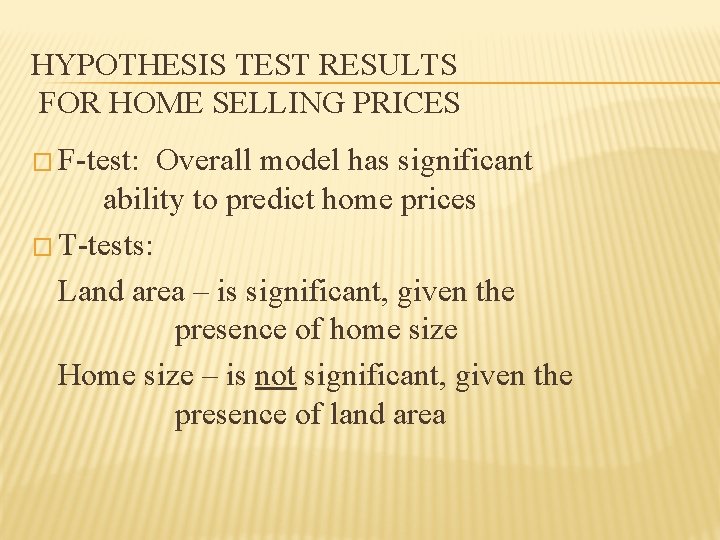
HYPOTHESIS TEST RESULTS FOR HOME SELLING PRICES � F-test: Overall model has significant ability to predict home prices � T-tests: Land area – is significant, given the presence of home size Home size – is not significant, given the presence of land area
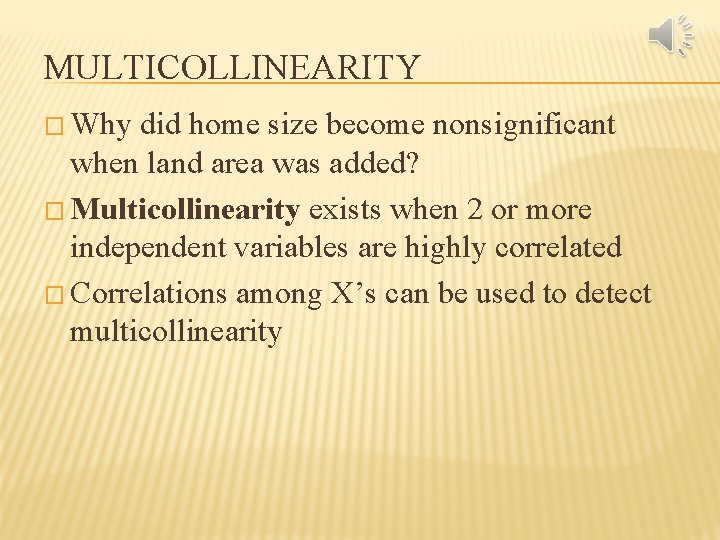
MULTICOLLINEARITY � Why did home size become nonsignificant when land area was added? � Multicollinearity exists when 2 or more independent variables are highly correlated � Correlations among X’s can be used to detect multicollinearity
Logistic curve method
Naive method forecasting
Chain ratio method of demand forecasting
Geometric increase method of population forecasting
Curvilinear method population estimation
Static forecasting method
A static method of forecasting
The primary method for associative forecasting is:
Forecasted financial statements
Dr ramdhani
Simultaneous equation method in demand forecasting
Arithmetic regression method for population forecasting
Chain ratio method of demand forecasting
Persistence method of weather forecasting
Static forecasting method
Symposium advantages and disadvantages