Federal Department of Home Affairs FDHA Federal Office
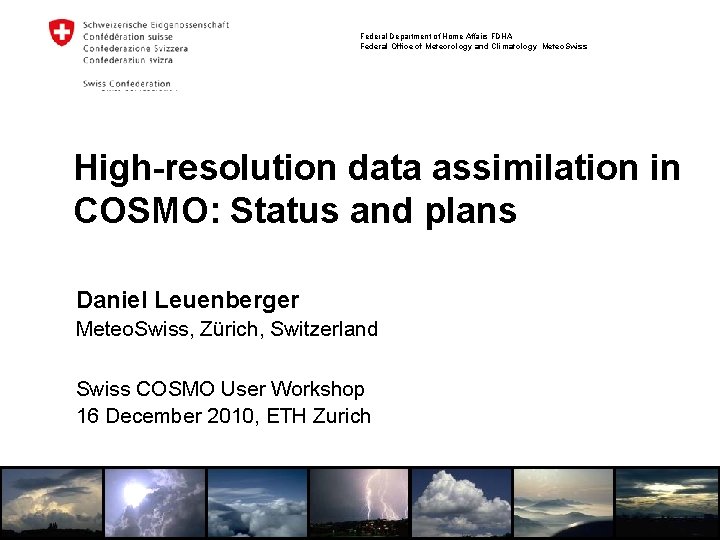
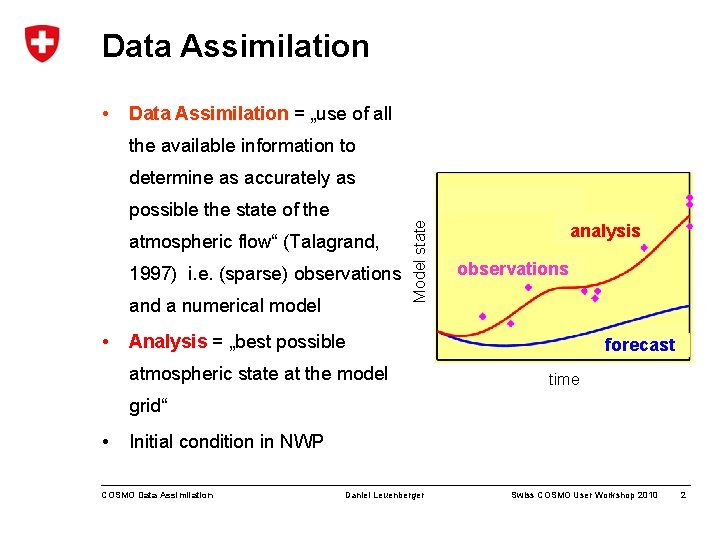
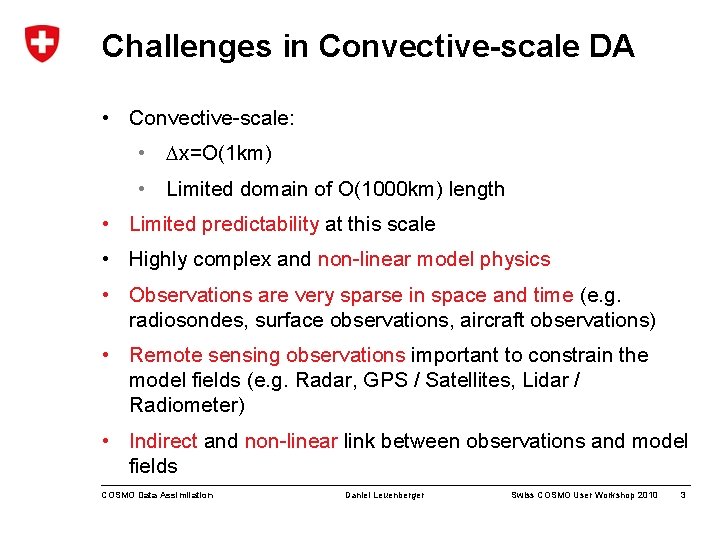
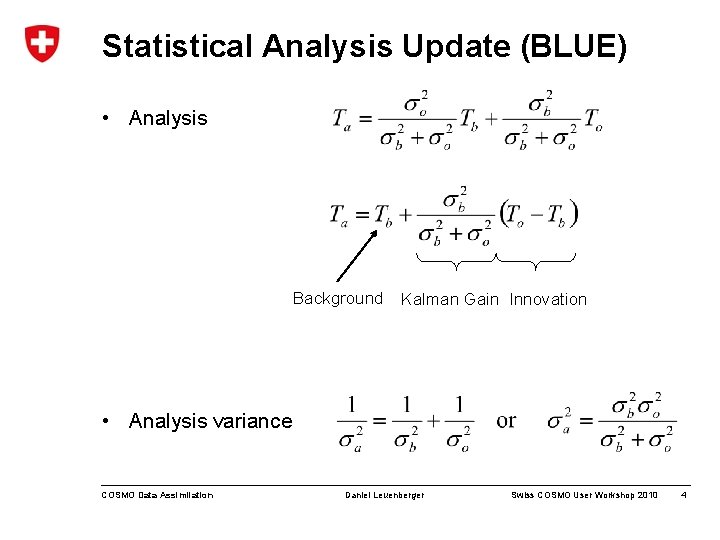
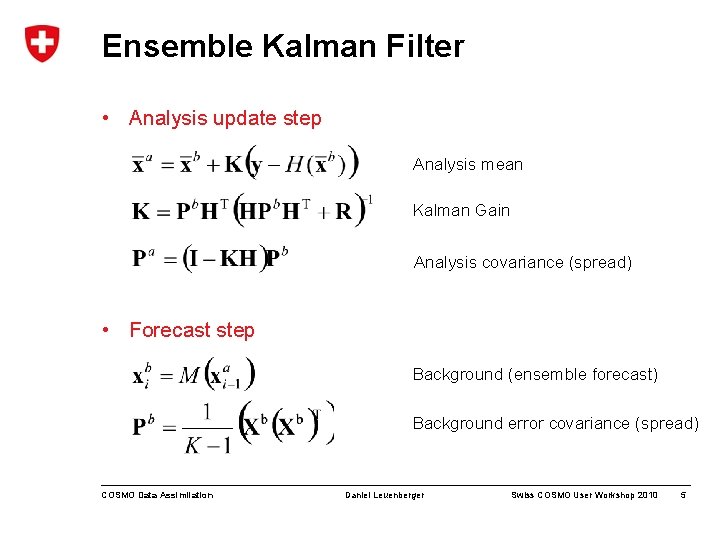
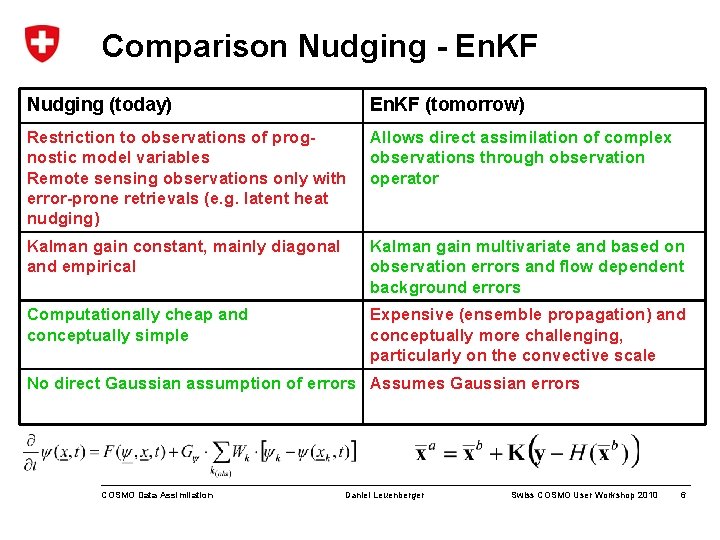
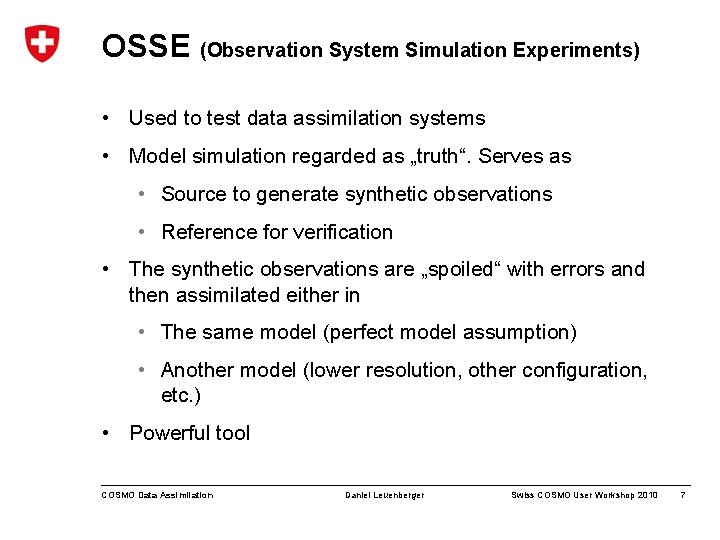
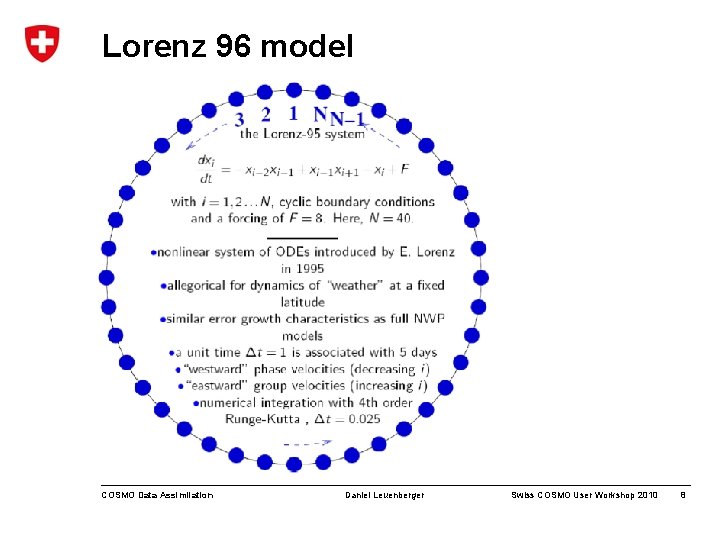
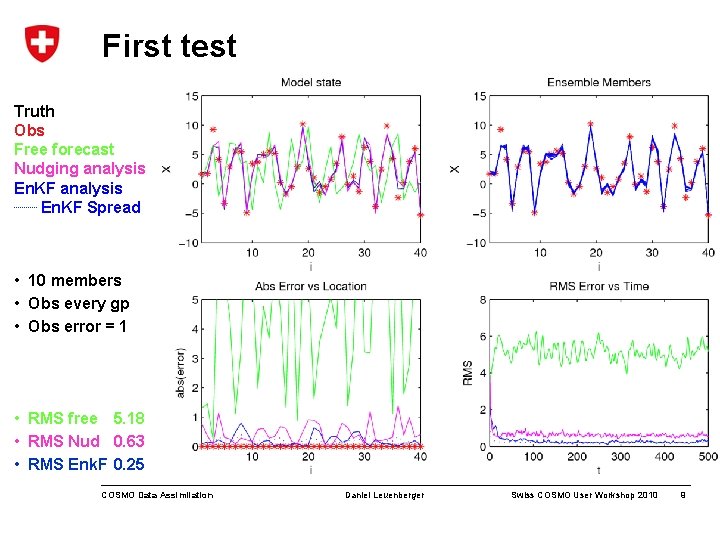
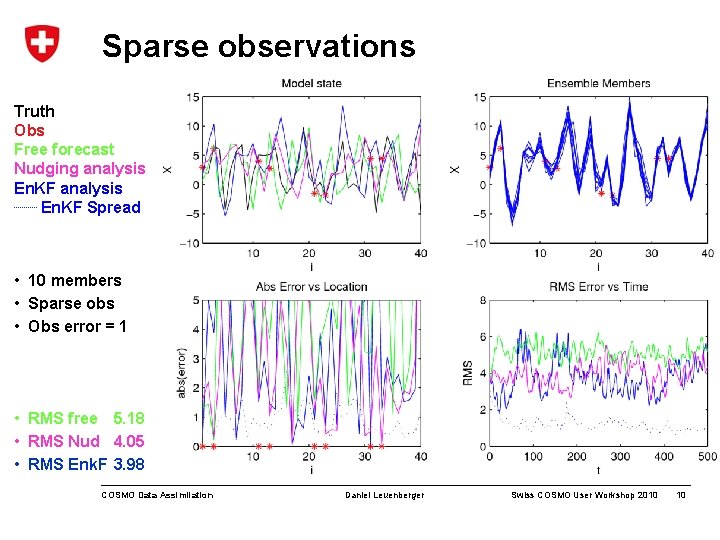
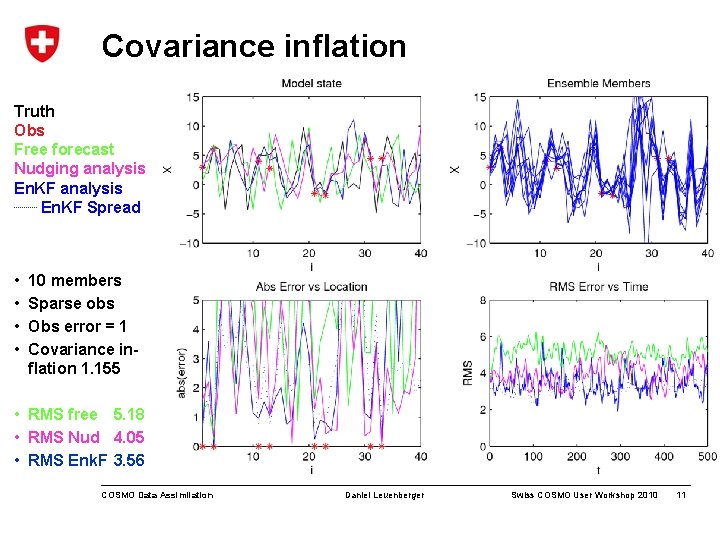
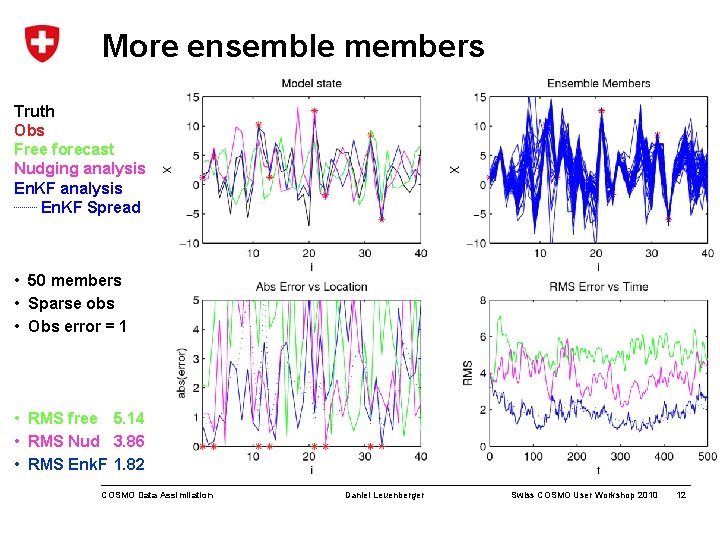
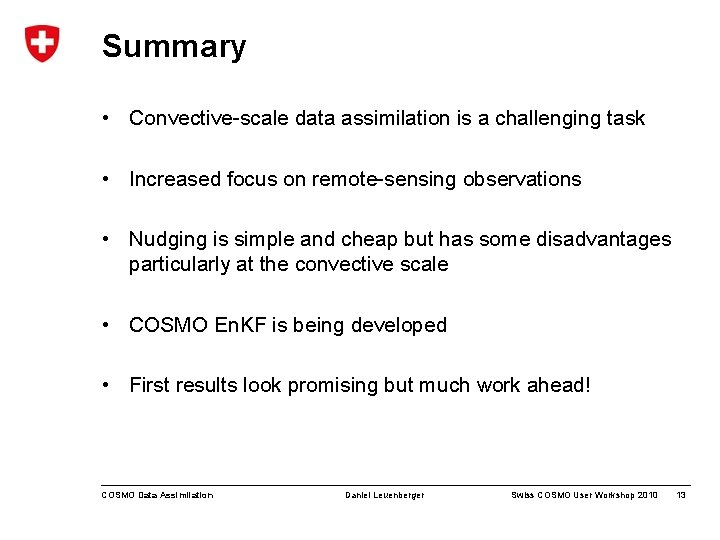
- Slides: 13
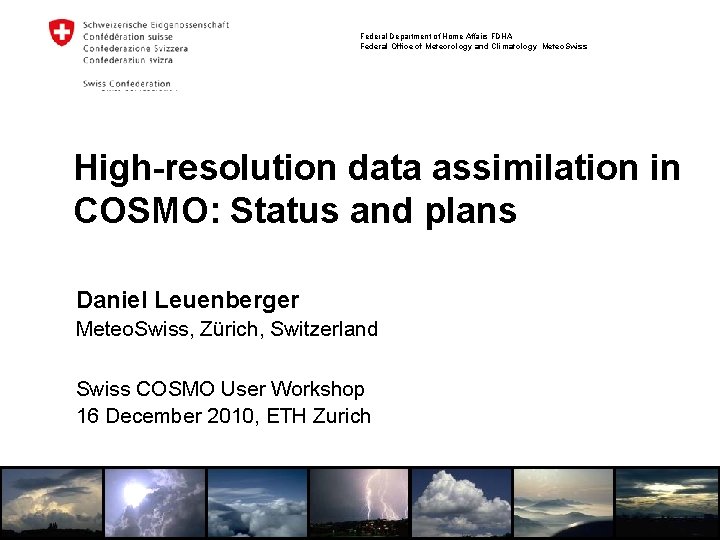
Federal Department of Home Affairs FDHA Federal Office of Meteorology and Climatology Meteo. Swiss High-resolution data assimilation in COSMO: Status and plans Daniel Leuenberger Meteo. Swiss, Zürich, Switzerland Swiss COSMO User Workshop 16 December 2010, ETH Zurich
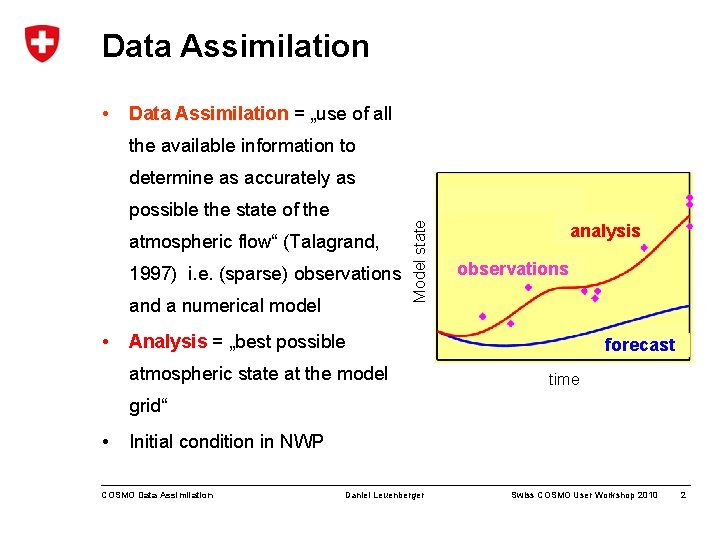
Data Assimilation • Data Assimilation = „use of all the available information to determine as accurately as atmospheric flow“ (Talagrand, 1997) i. e. (sparse) observations and a numerical model • Model state possible the state of the analysis observations Analysis = „best possible atmospheric state at the model forecast time grid“ • Initial condition in NWP COSMO Data Assimilation Daniel Leuenberger Swiss COSMO User Workshop 2010 2
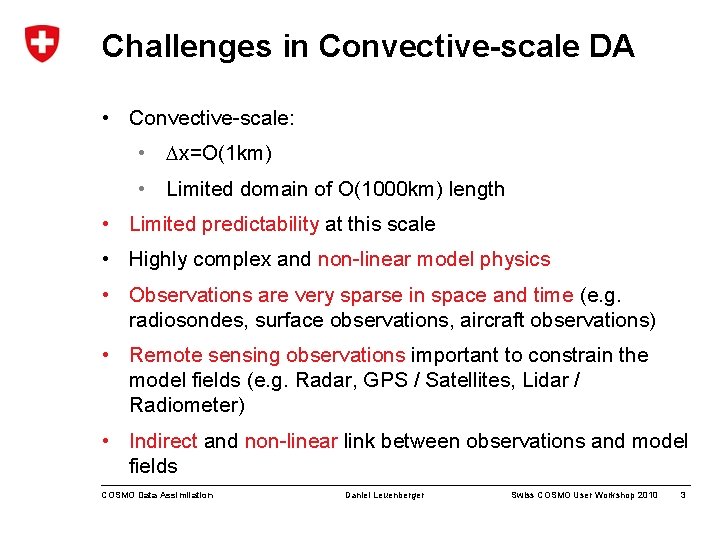
Challenges in Convective-scale DA • Convective-scale: • Dx=O(1 km) • Limited domain of O(1000 km) length • Limited predictability at this scale • Highly complex and non-linear model physics • Observations are very sparse in space and time (e. g. radiosondes, surface observations, aircraft observations) • Remote sensing observations important to constrain the model fields (e. g. Radar, GPS / Satellites, Lidar / Radiometer) • Indirect and non-linear link between observations and model fields COSMO Data Assimilation Daniel Leuenberger Swiss COSMO User Workshop 2010 3
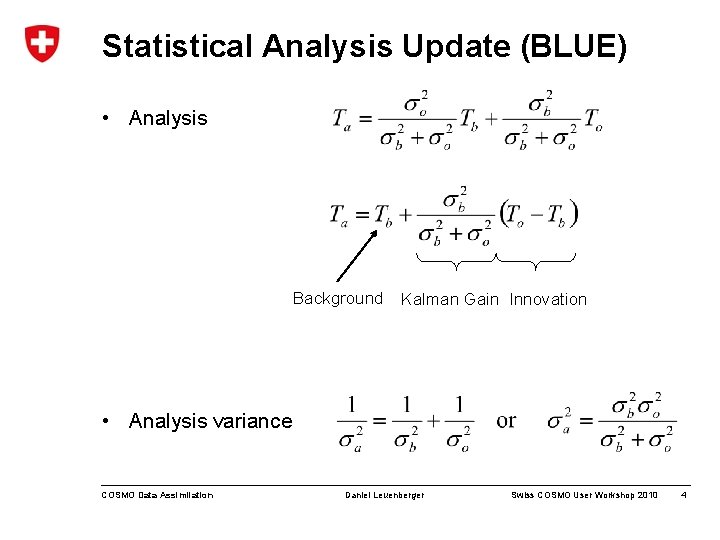
Statistical Analysis Update (BLUE) • Analysis Background Kalman Gain Innovation • Analysis variance COSMO Data Assimilation Daniel Leuenberger Swiss COSMO User Workshop 2010 4
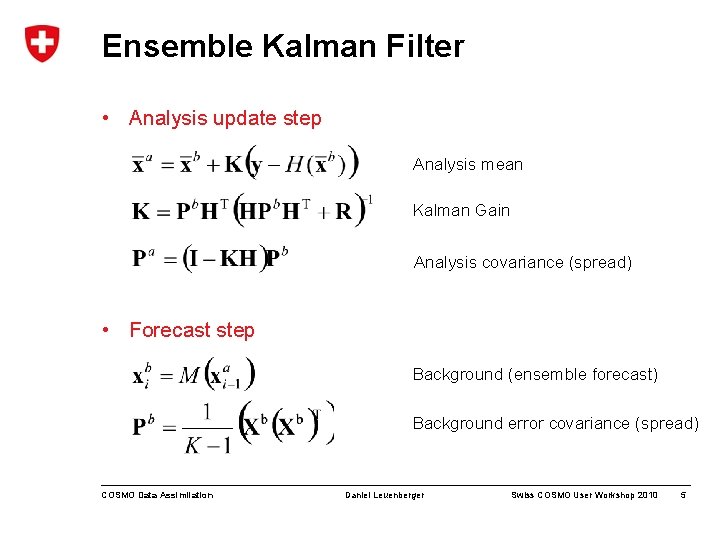
Ensemble Kalman Filter • Analysis update step Analysis mean Kalman Gain Analysis covariance (spread) • Forecast step Background (ensemble forecast) Background error covariance (spread) COSMO Data Assimilation Daniel Leuenberger Swiss COSMO User Workshop 2010 5
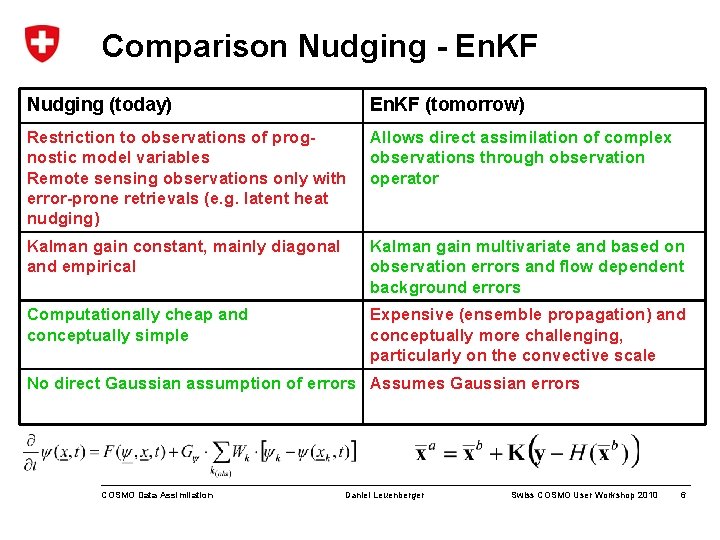
Comparison Nudging - En. KF Nudging (today) En. KF (tomorrow) Restriction to observations of prognostic model variables Remote sensing observations only with error-prone retrievals (e. g. latent heat nudging) Allows direct assimilation of complex observations through observation operator Kalman gain constant, mainly diagonal and empirical Kalman gain multivariate and based on observation errors and flow dependent background errors Computationally cheap and conceptually simple Expensive (ensemble propagation) and conceptually more challenging, particularly on the convective scale No direct Gaussian assumption of errors Assumes Gaussian errors COSMO Data Assimilation Daniel Leuenberger Swiss COSMO User Workshop 2010 6
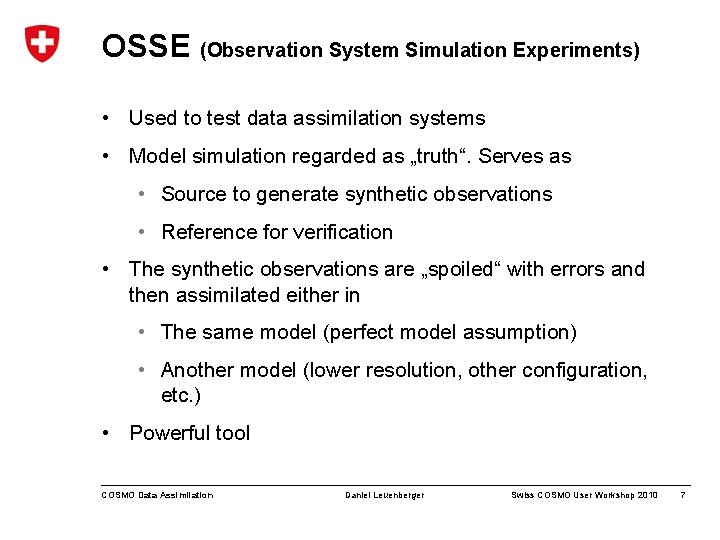
OSSE (Observation System Simulation Experiments) • Used to test data assimilation systems • Model simulation regarded as „truth“. Serves as • Source to generate synthetic observations • Reference for verification • The synthetic observations are „spoiled“ with errors and then assimilated either in • The same model (perfect model assumption) • Another model (lower resolution, other configuration, etc. ) • Powerful tool COSMO Data Assimilation Daniel Leuenberger Swiss COSMO User Workshop 2010 7
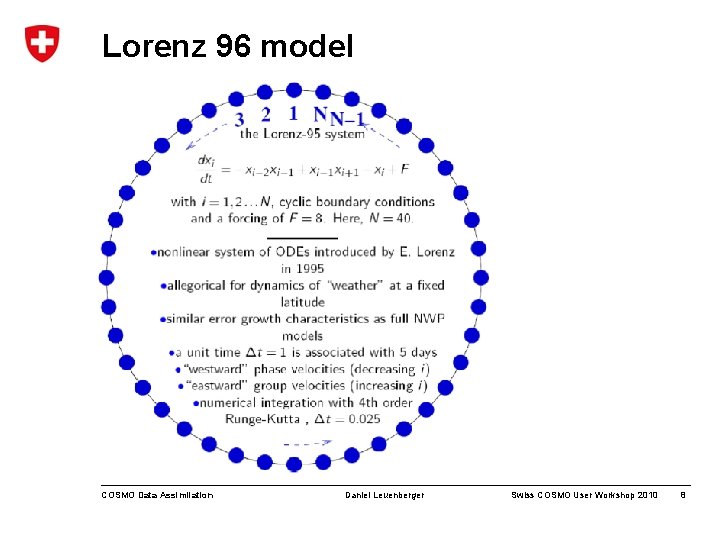
Lorenz 96 model COSMO Data Assimilation Daniel Leuenberger Swiss COSMO User Workshop 2010 8
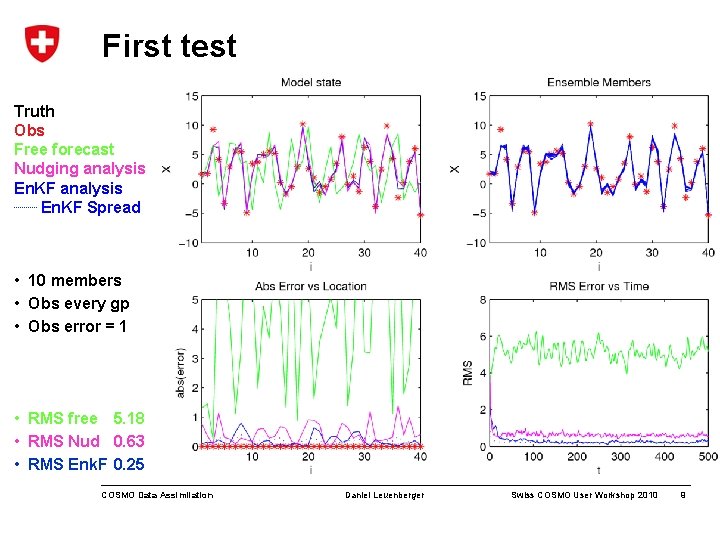
First test Truth Obs Free forecast Nudging analysis En. KF Spread • 10 members • Obs every gp • Obs error = 1 • RMS free 5. 18 • RMS Nud 0. 63 • RMS Enk. F 0. 25 COSMO Data Assimilation Daniel Leuenberger Swiss COSMO User Workshop 2010 9
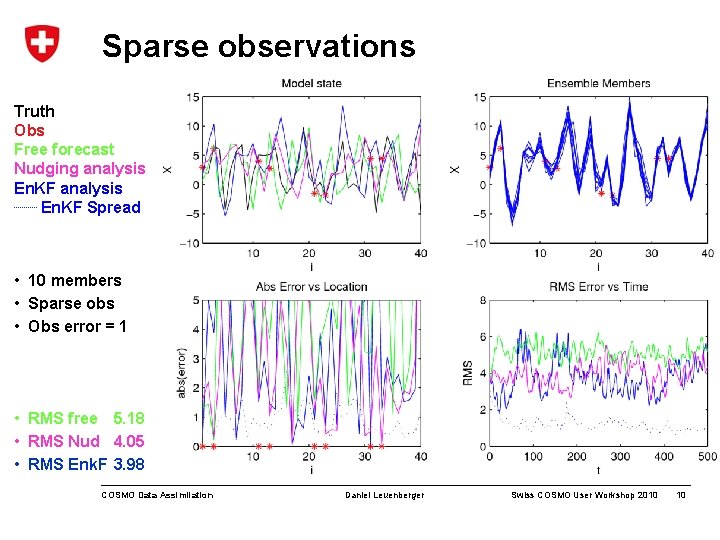
Sparse observations Truth Obs Free forecast Nudging analysis En. KF Spread • 10 members • Sparse obs • Obs error = 1 • RMS free 5. 18 • RMS Nud 4. 05 • RMS Enk. F 3. 98 COSMO Data Assimilation Daniel Leuenberger Swiss COSMO User Workshop 2010 10
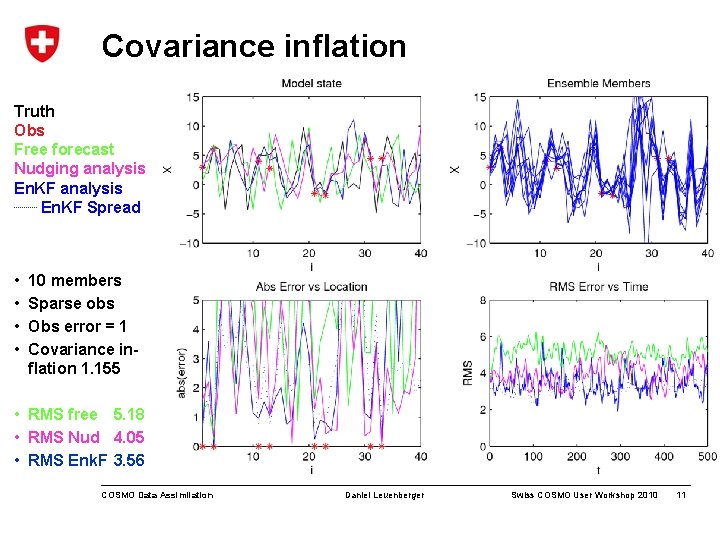
Covariance inflation Truth Obs Free forecast Nudging analysis En. KF Spread • • 10 members Sparse obs Obs error = 1 Covariance inflation 1. 155 • RMS free 5. 18 • RMS Nud 4. 05 • RMS Enk. F 3. 56 COSMO Data Assimilation Daniel Leuenberger Swiss COSMO User Workshop 2010 11
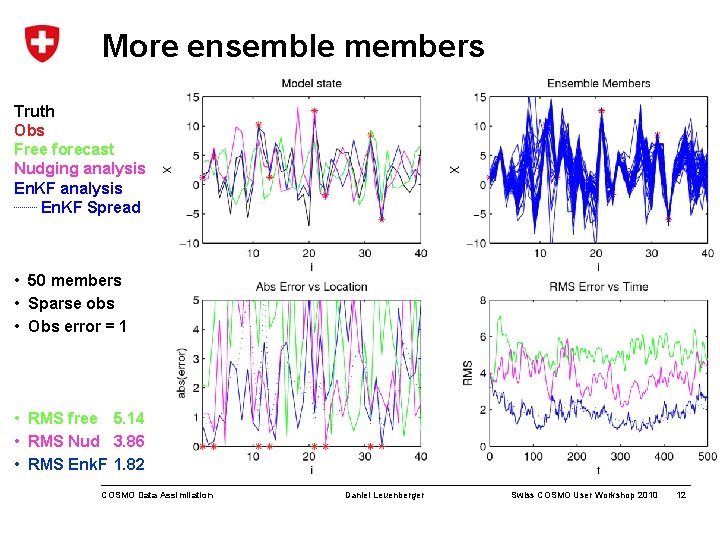
More ensemble members Truth Obs Free forecast Nudging analysis En. KF Spread • 50 members • Sparse obs • Obs error = 1 • RMS free 5. 14 • RMS Nud 3. 86 • RMS Enk. F 1. 82 COSMO Data Assimilation Daniel Leuenberger Swiss COSMO User Workshop 2010 12
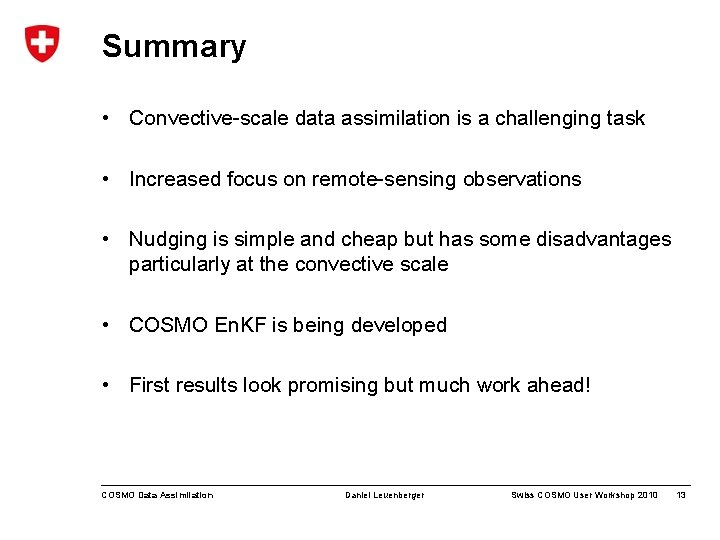
Summary • Convective-scale data assimilation is a challenging task • Increased focus on remote-sensing observations • Nudging is simple and cheap but has some disadvantages particularly at the convective scale • COSMO En. KF is being developed • First results look promising but much work ahead! COSMO Data Assimilation Daniel Leuenberger Swiss COSMO User Workshop 2010 13
Federal department of home affairs fdha
Federal department of home affairs fdha
Federal department of home affairs fdha
Va form 10-250
Fdha loan
Michigan department of licensing and regulation
Department of commerce and consumer affairs
Department of local affairs
Hhs office of population affairs
Ntust gym
Hms office of faculty affairs
Arti dari home care
Perbedaan home care dan home visit
Mobile home parks for sale in sc