Face recognition using Histograms of Oriented Gradients O
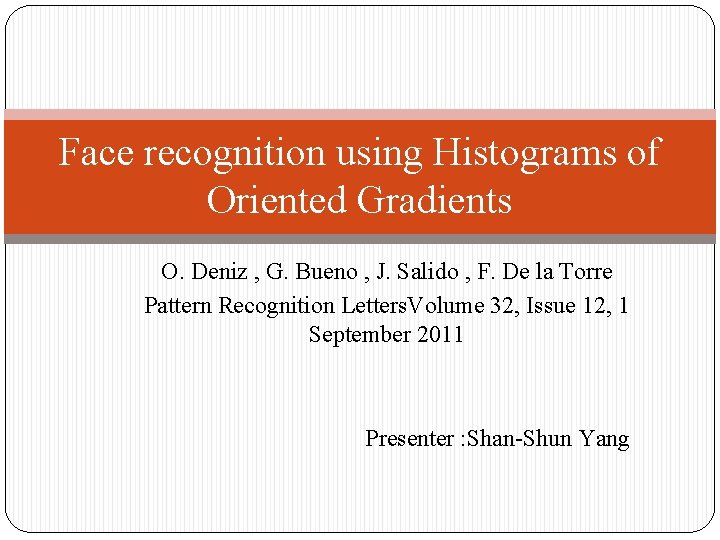
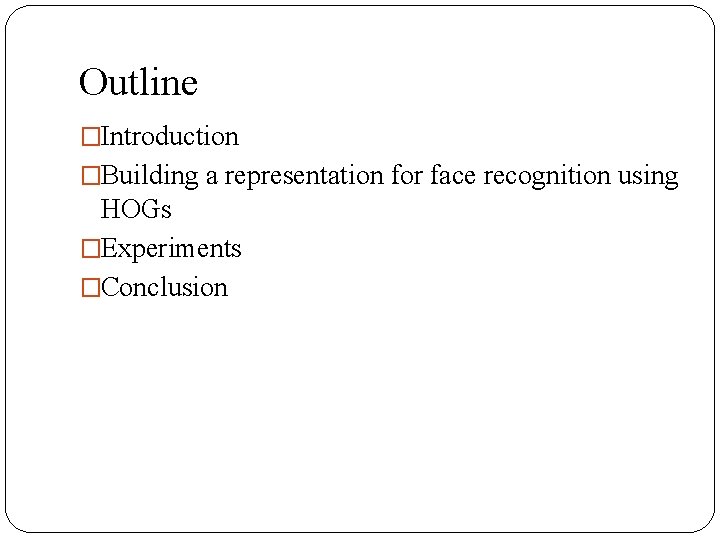
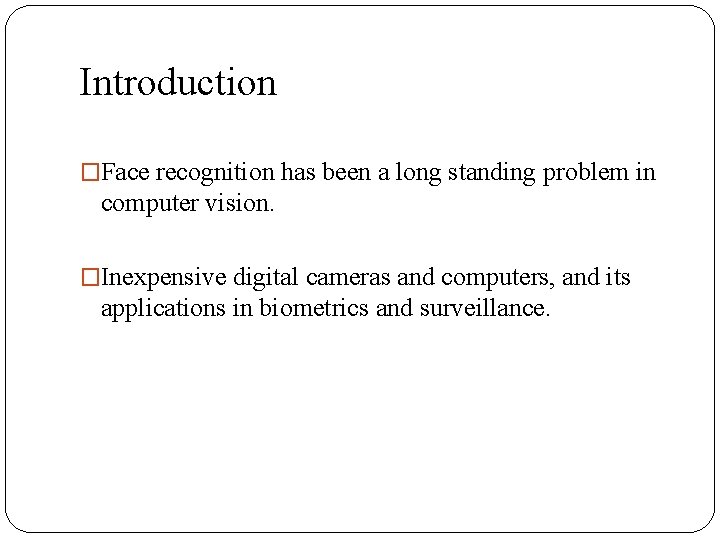
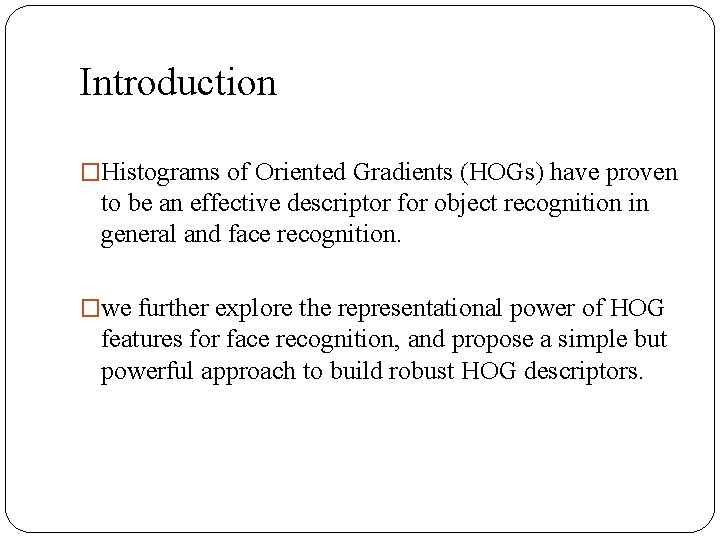
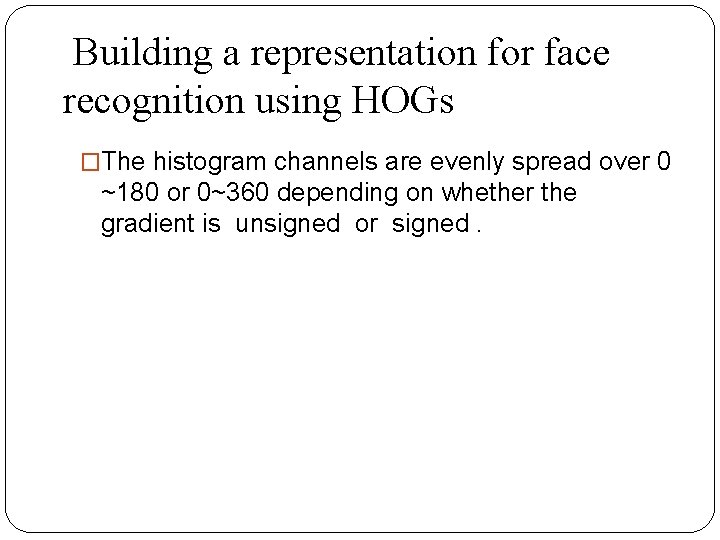
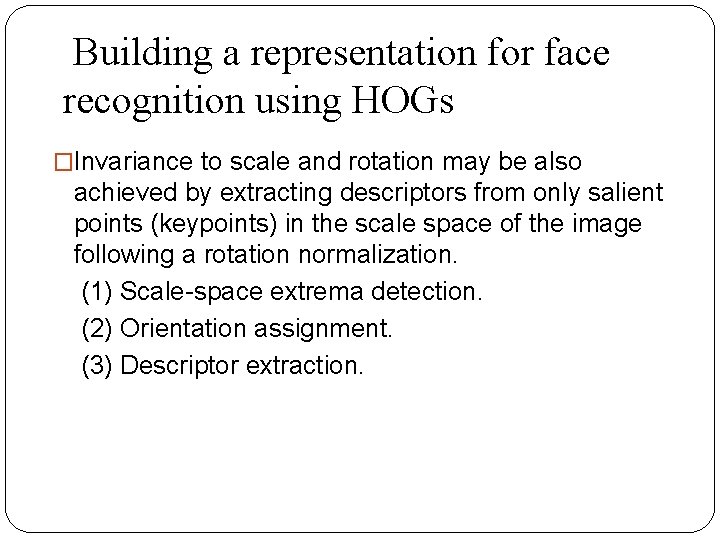
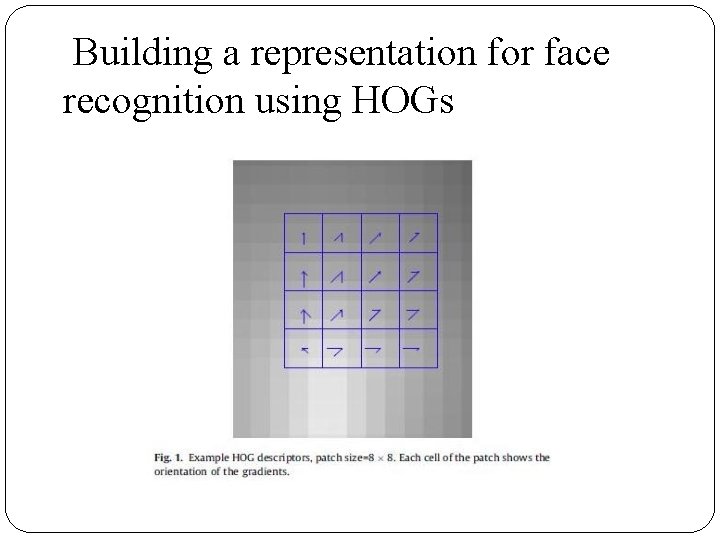
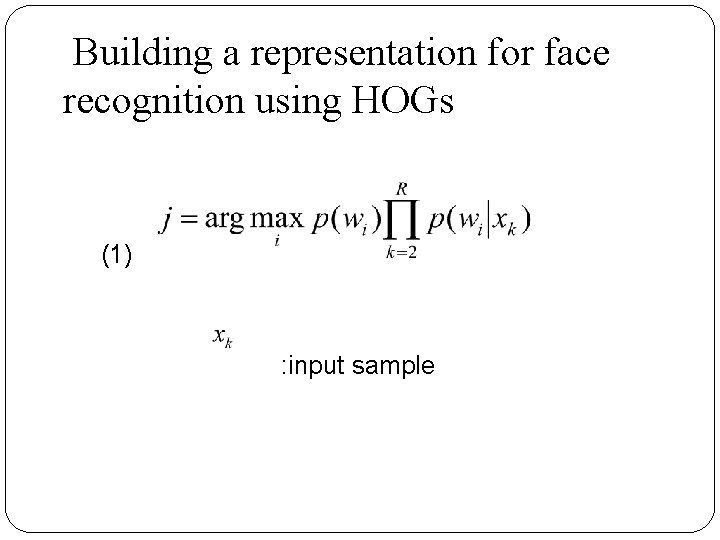
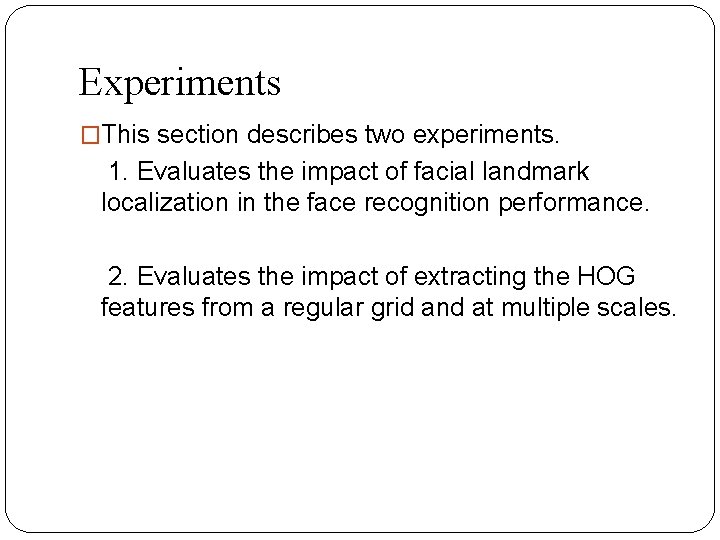
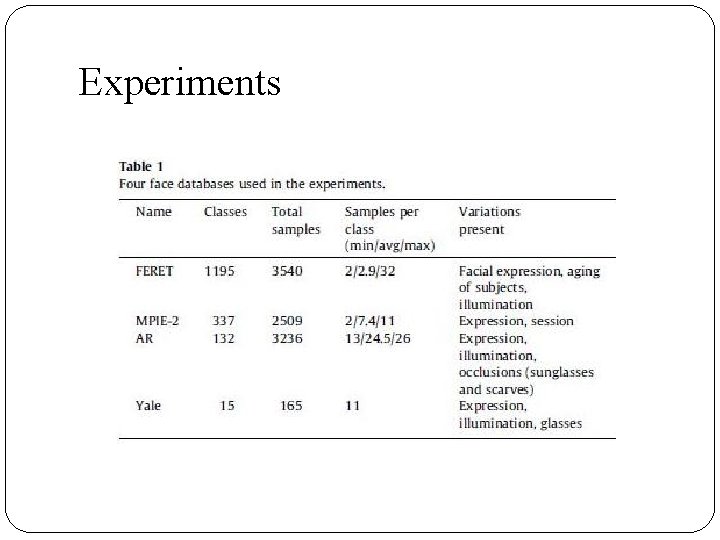
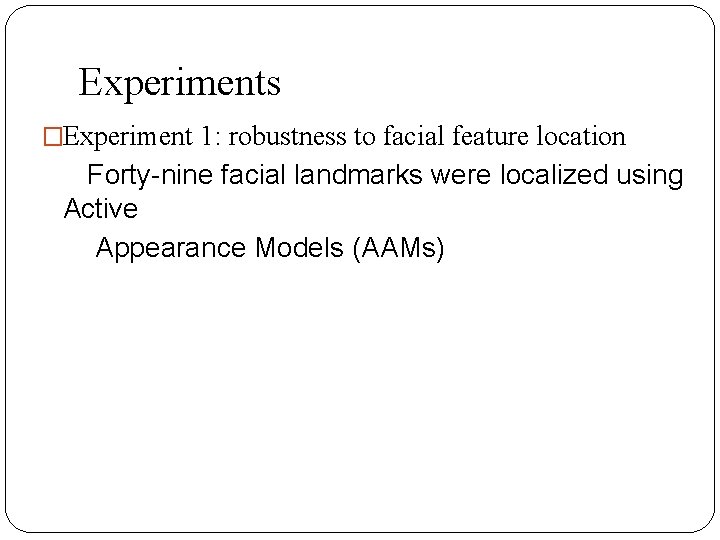
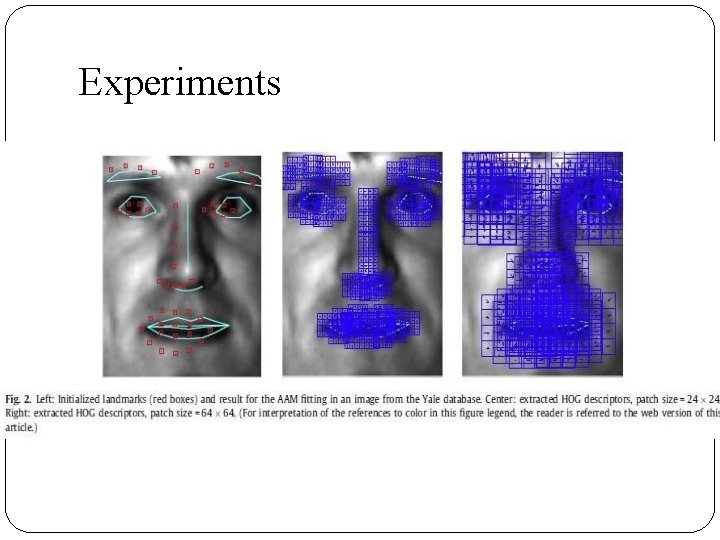
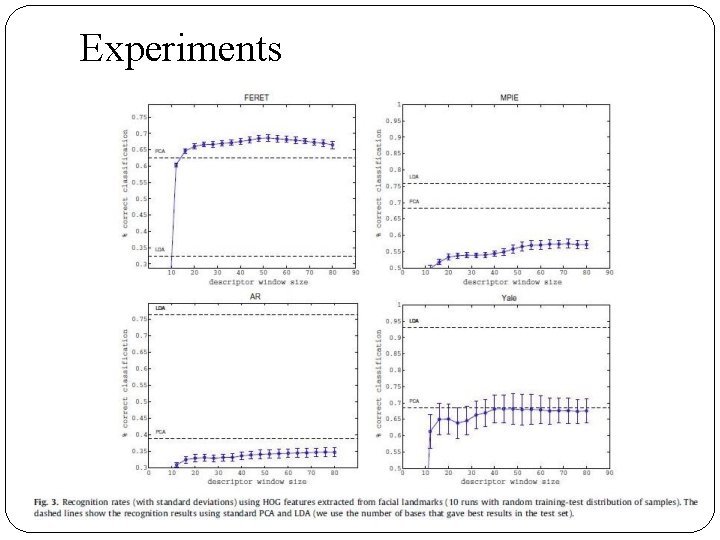
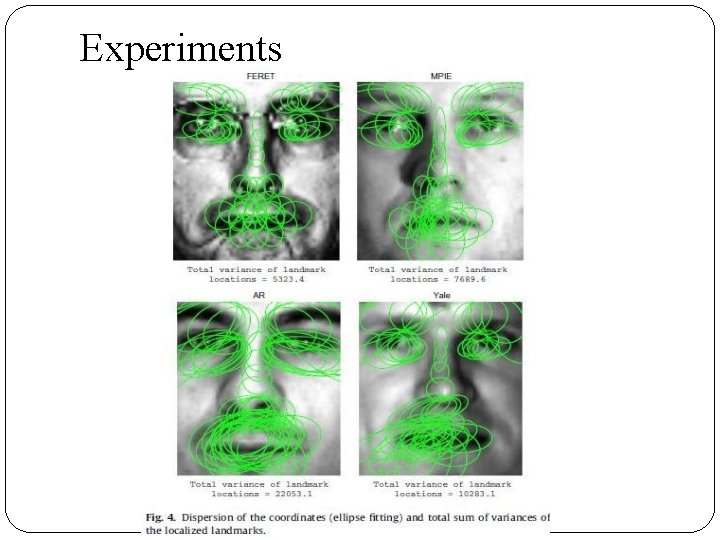
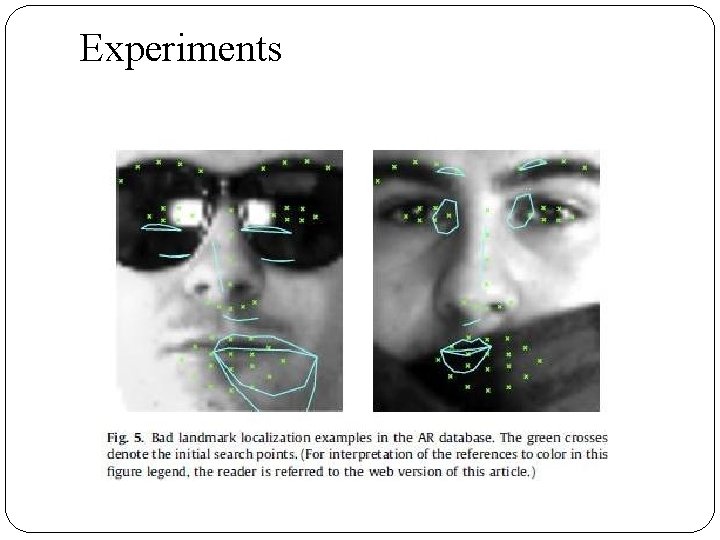
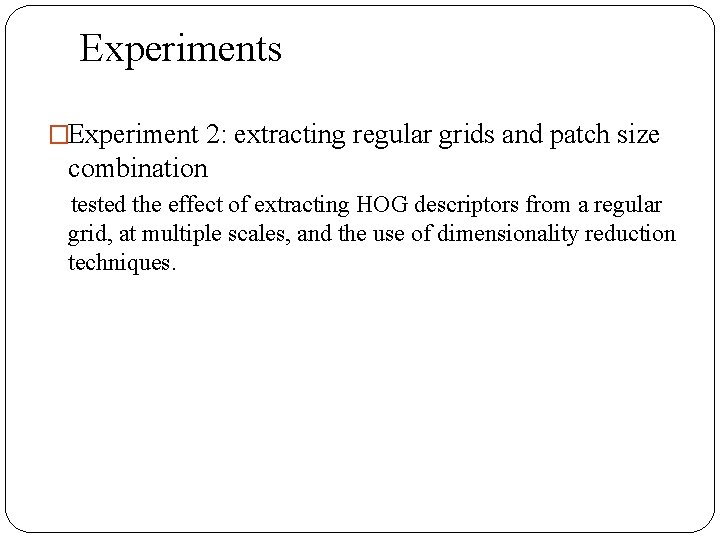
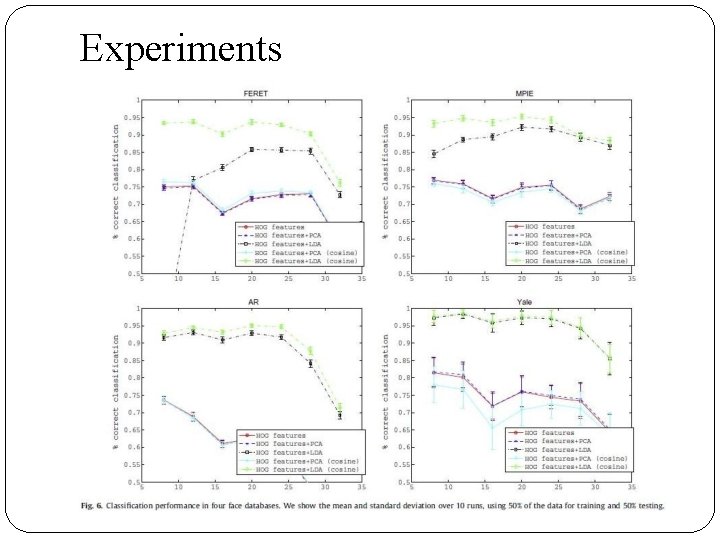
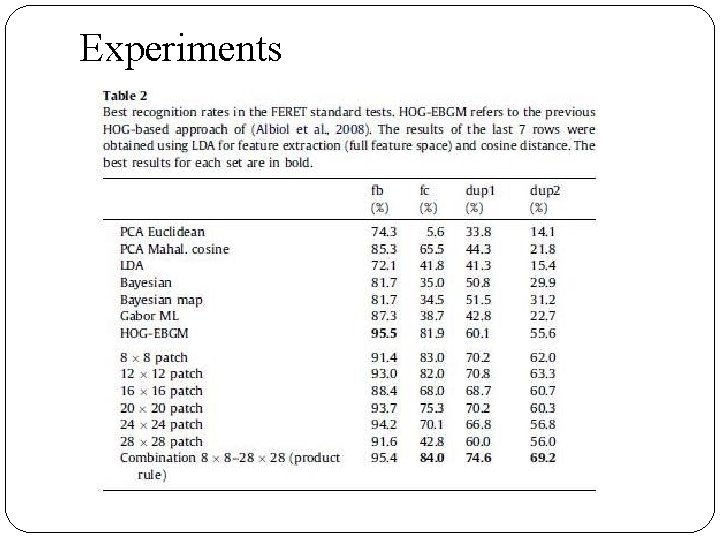
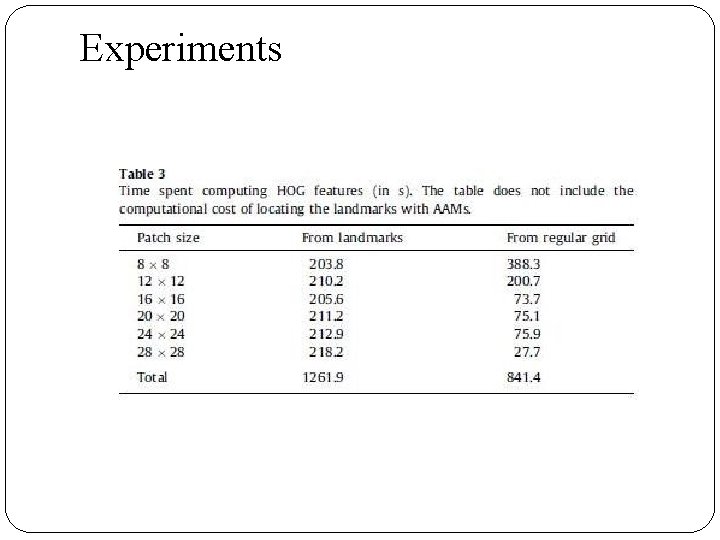
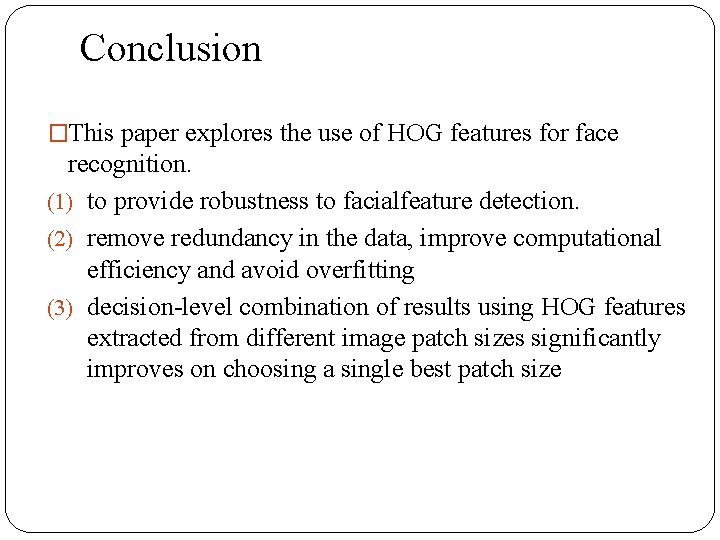
- Slides: 20
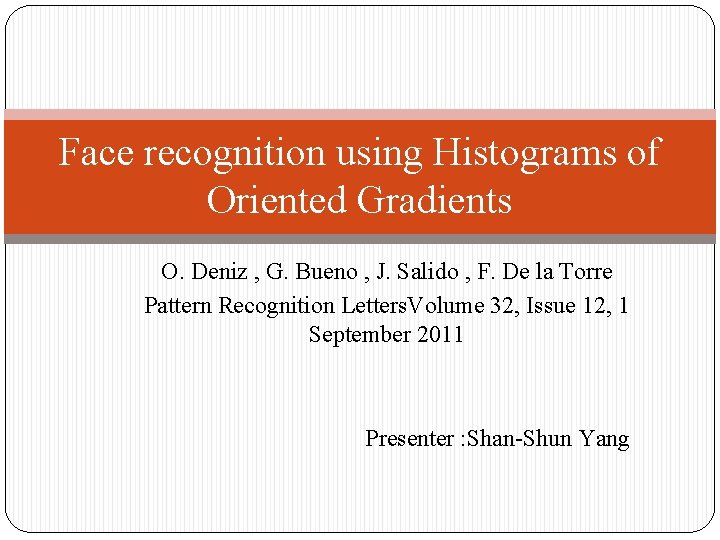
Face recognition using Histograms of Oriented Gradients O. Deniz , G. Bueno , J. Salido , F. De la Torre Pattern Recognition Letters. Volume 32, Issue 12, 1 September 2011 Presenter : Shan-Shun Yang
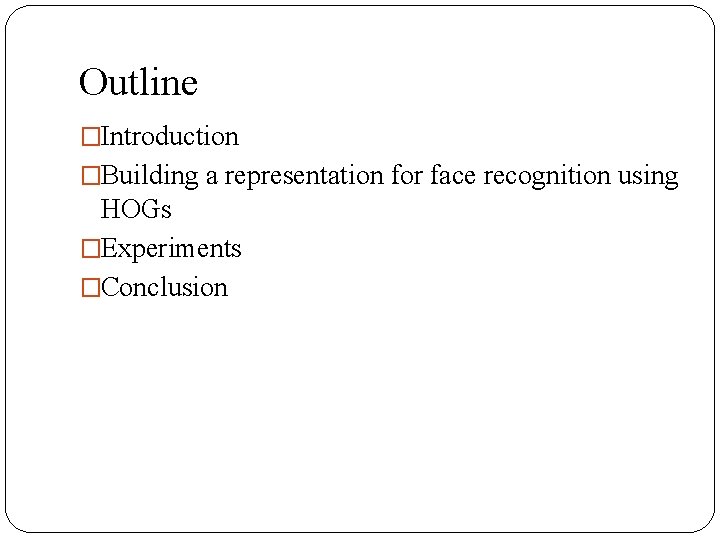
Outline �Introduction �Building a representation for face recognition using HOGs �Experiments �Conclusion
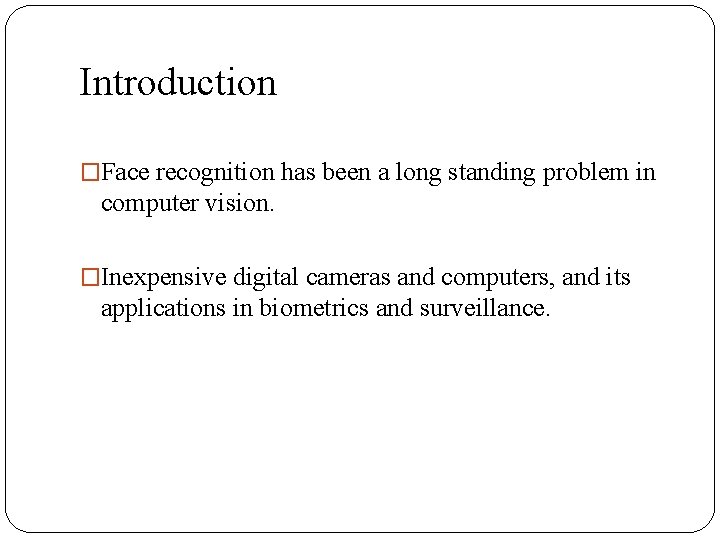
Introduction �Face recognition has been a long standing problem in computer vision. �Inexpensive digital cameras and computers, and its applications in biometrics and surveillance.
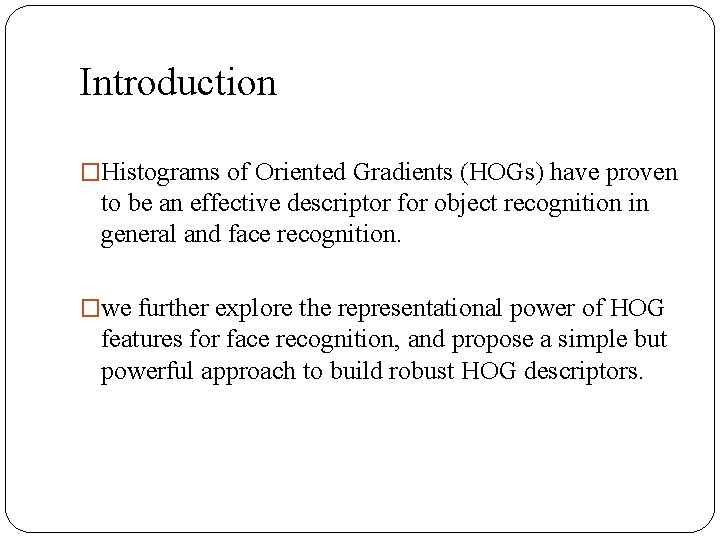
Introduction �Histograms of Oriented Gradients (HOGs) have proven to be an effective descriptor for object recognition in general and face recognition. �we further explore the representational power of HOG features for face recognition, and propose a simple but powerful approach to build robust HOG descriptors.
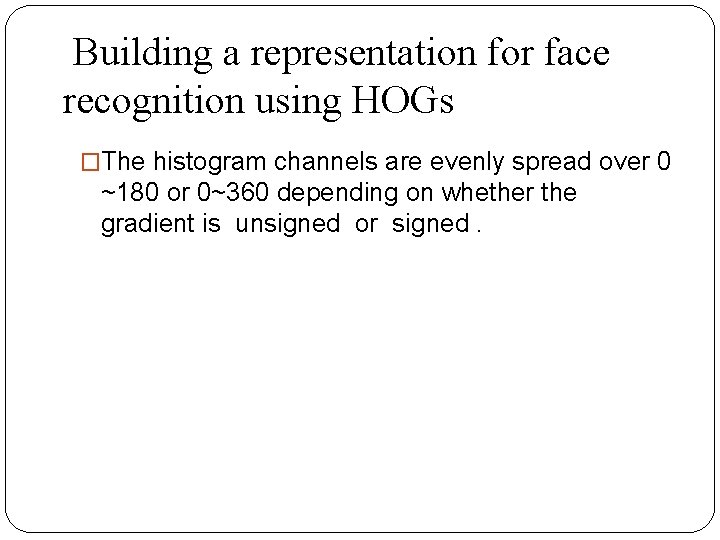
Building a representation for face recognition using HOGs �The histogram channels are evenly spread over 0 ~180 or 0~360 depending on whether the gradient is unsigned or signed.
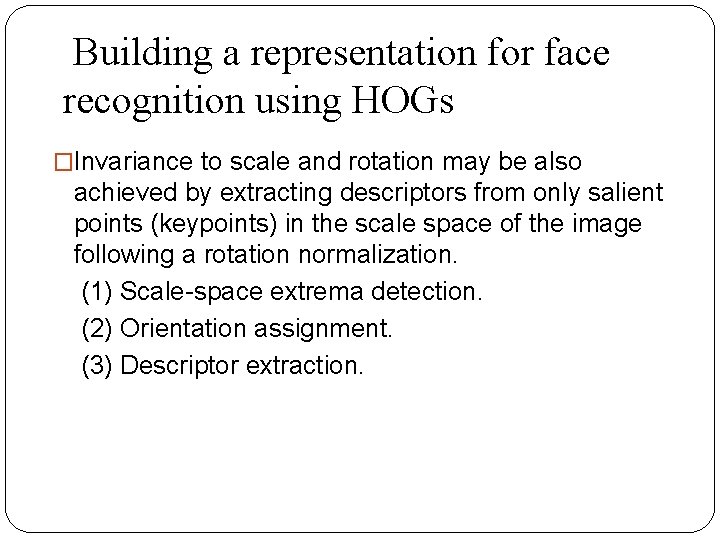
Building a representation for face recognition using HOGs �Invariance to scale and rotation may be also achieved by extracting descriptors from only salient points (keypoints) in the scale space of the image following a rotation normalization. (1) Scale-space extrema detection. (2) Orientation assignment. (3) Descriptor extraction.
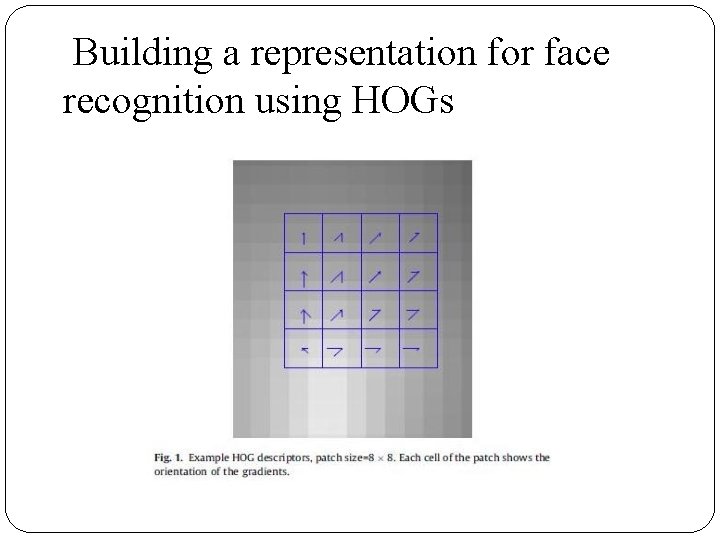
Building a representation for face recognition using HOGs
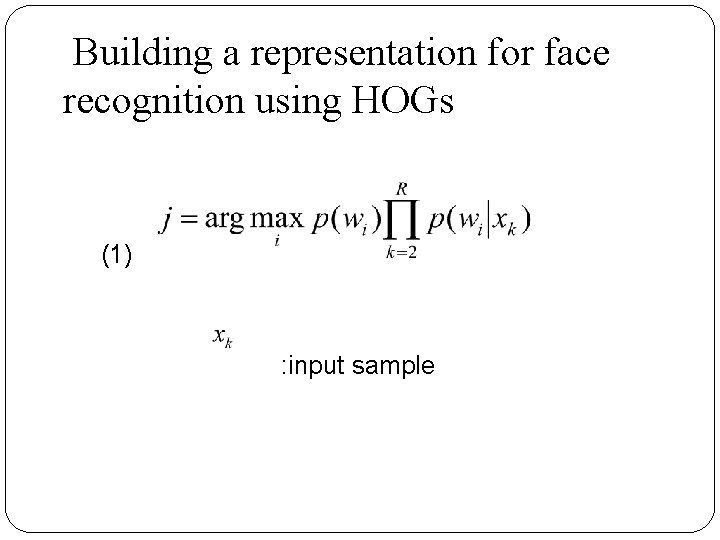
Building a representation for face recognition using HOGs (1) : input sample
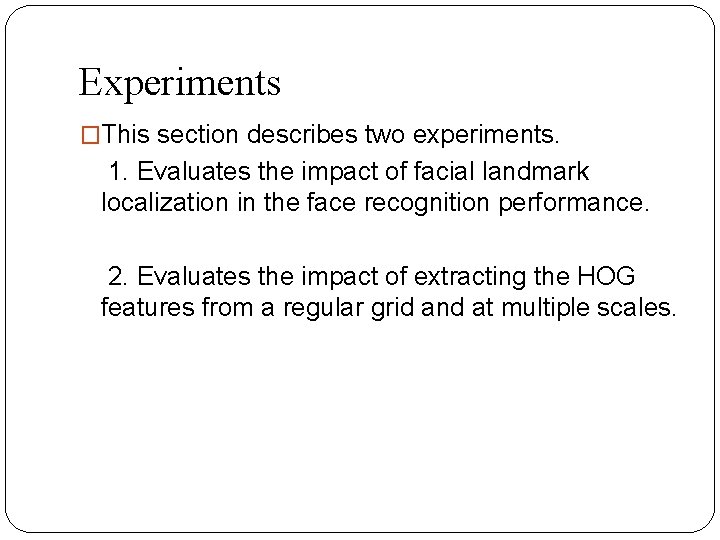
Experiments �This section describes two experiments. 1. Evaluates the impact of facial landmark localization in the face recognition performance. 2. Evaluates the impact of extracting the HOG features from a regular grid and at multiple scales.
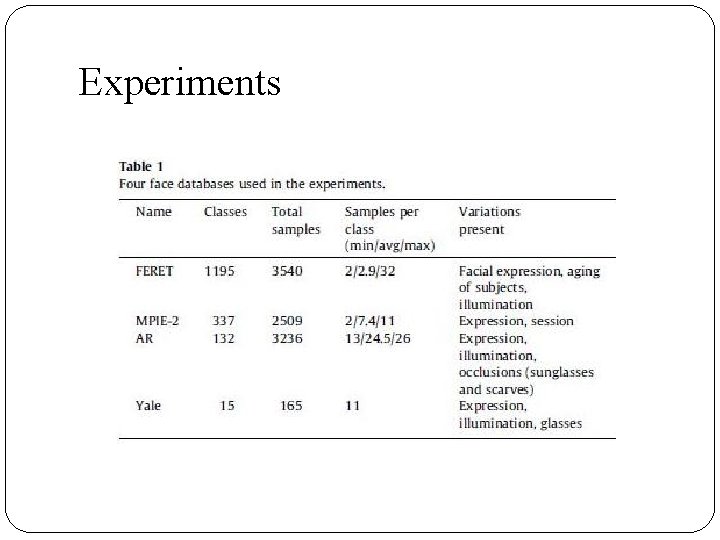
Experiments
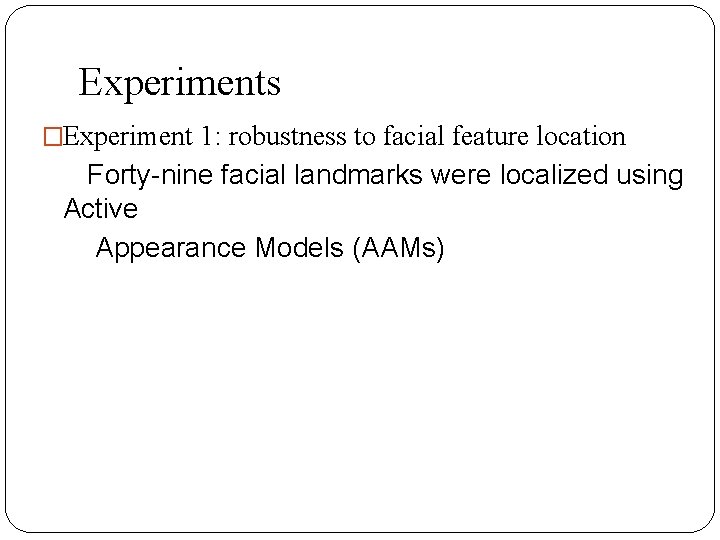
Experiments �Experiment 1: robustness to facial feature location Forty-nine facial landmarks were localized using Active Appearance Models (AAMs)
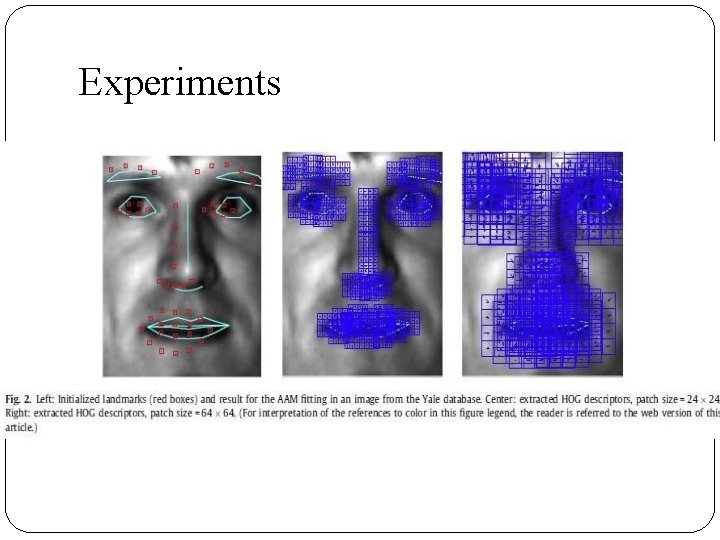
Experiments
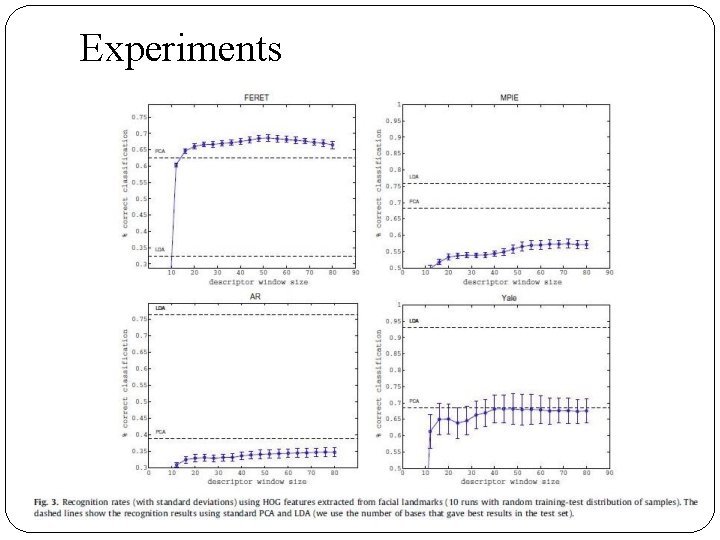
Experiments
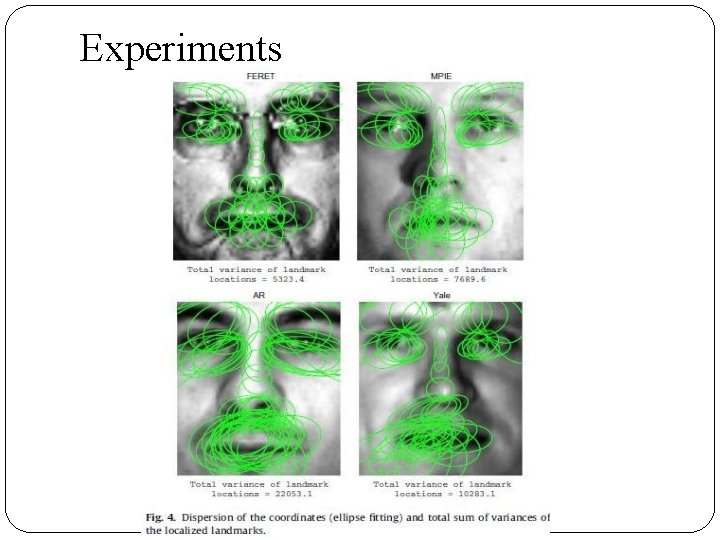
Experiments
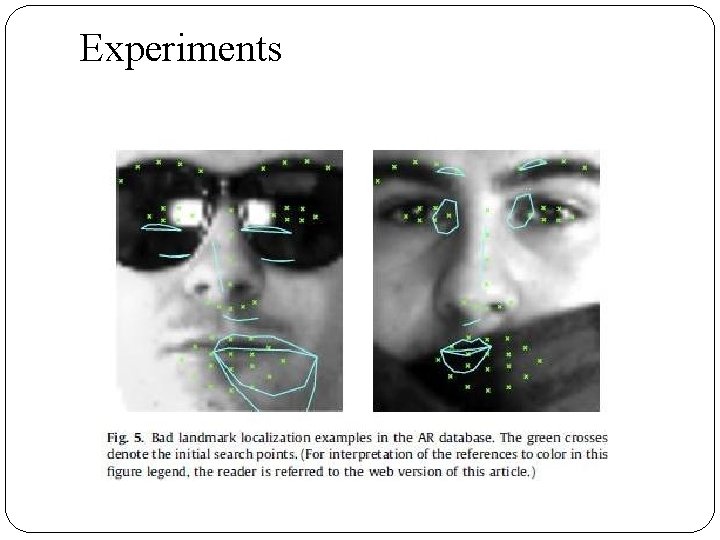
Experiments
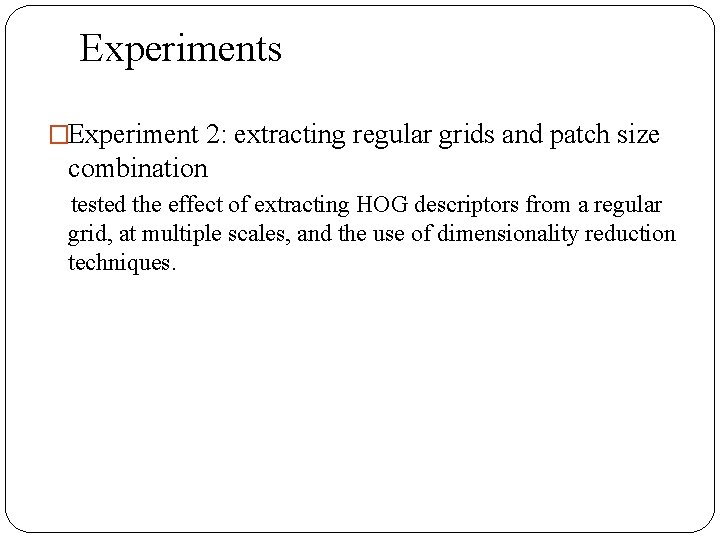
Experiments �Experiment 2: extracting regular grids and patch size combination tested the effect of extracting HOG descriptors from a regular grid, at multiple scales, and the use of dimensionality reduction techniques.
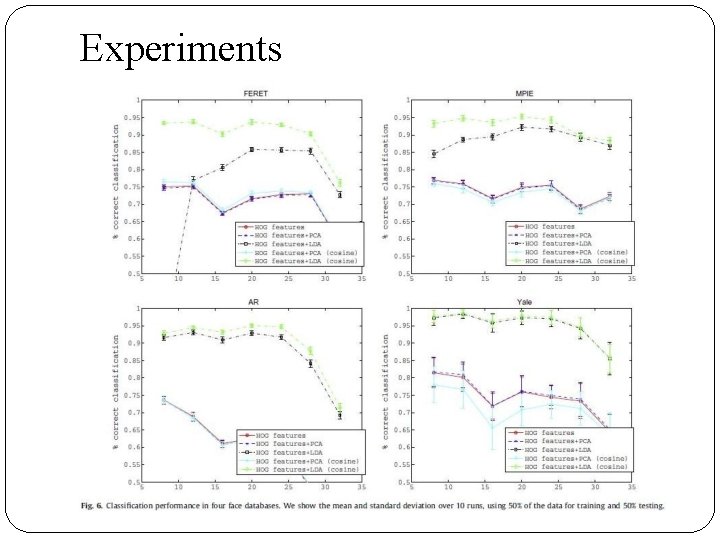
Experiments
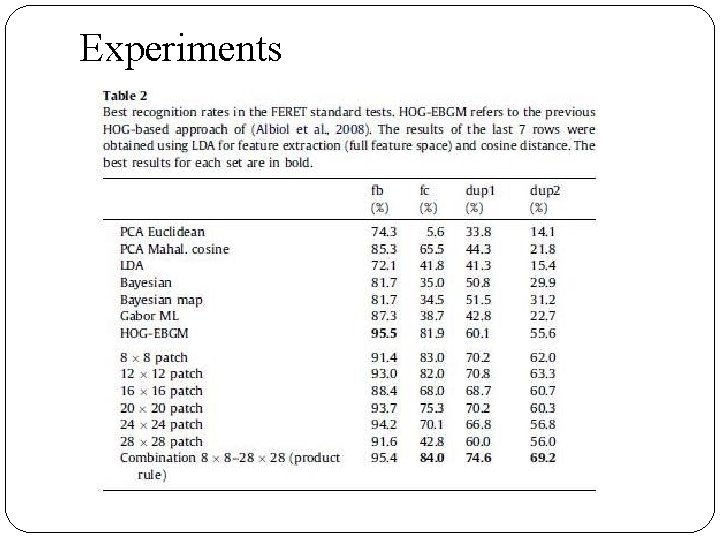
Experiments
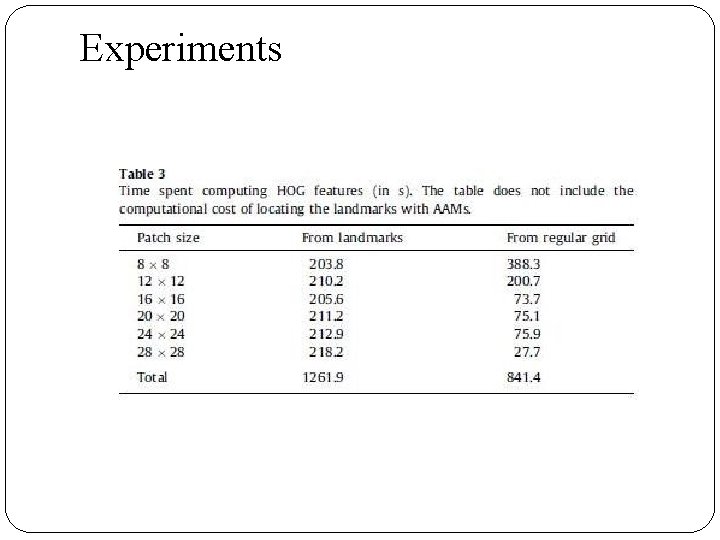
Experiments
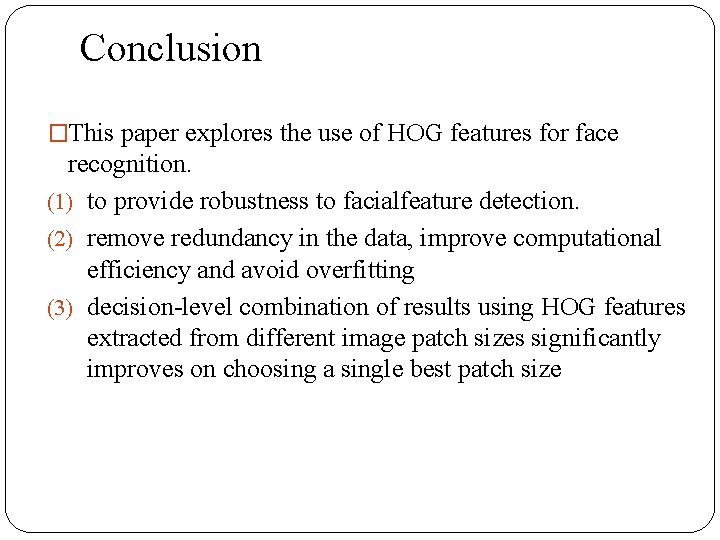
Conclusion �This paper explores the use of HOG features for face recognition. (1) to provide robustness to facialfeature detection. (2) remove redundancy in the data, improve computational efficiency and avoid overfitting (3) decision-level combination of results using HOG features extracted from different image patch sizes significantly improves on choosing a single best patch size
Histograms of oriented gradients for human detection
Histograms of oriented gradients for human detection
Pressure gradients in the heart
Authority gradient
Trade oriented sales promotion
What is a column row db
Face recognition
Facial expression recognition
Face recognition
Demystifying face recognition
Old woman young woman illusion
Face recognition a literature survey
Omkar parkhi
Clova face recognition
Conclusion of face recognition
Face recognition
Face recognition
Face recognition literature review
California association of school psychologists
Face recognition
Face recognition