ESSNET on Statistical Disclosure Control Daniela Ichim ESSNET
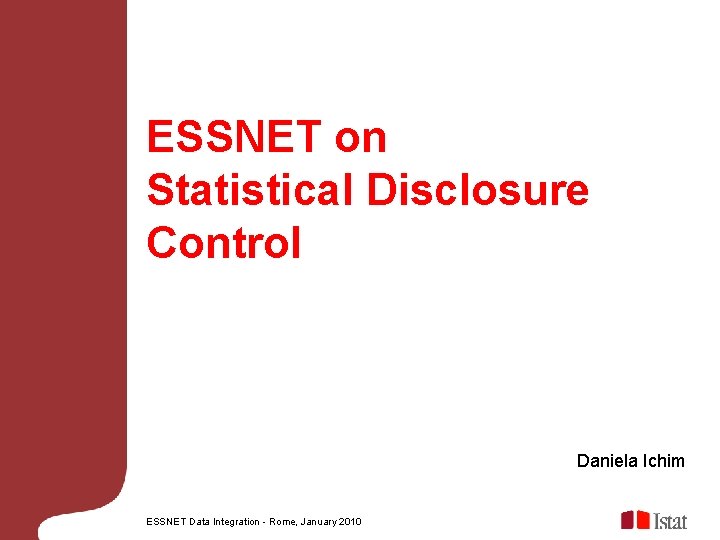
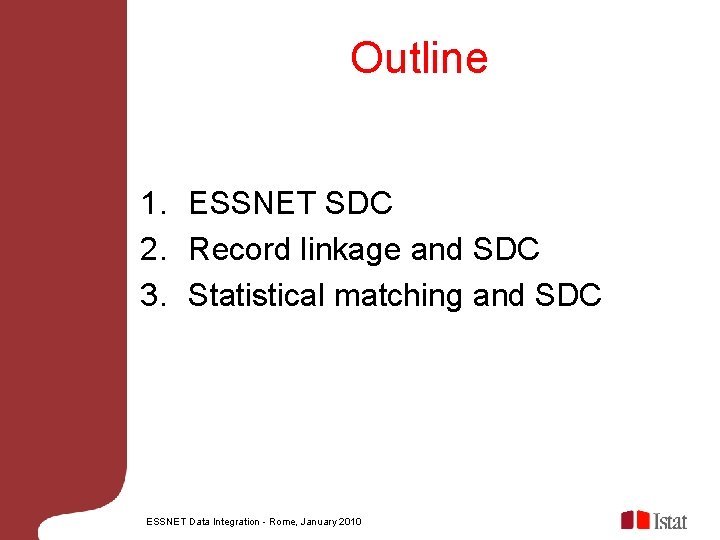
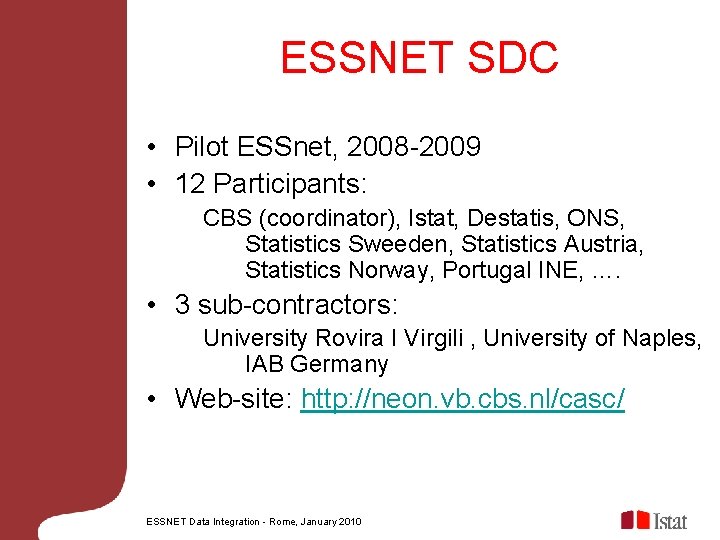
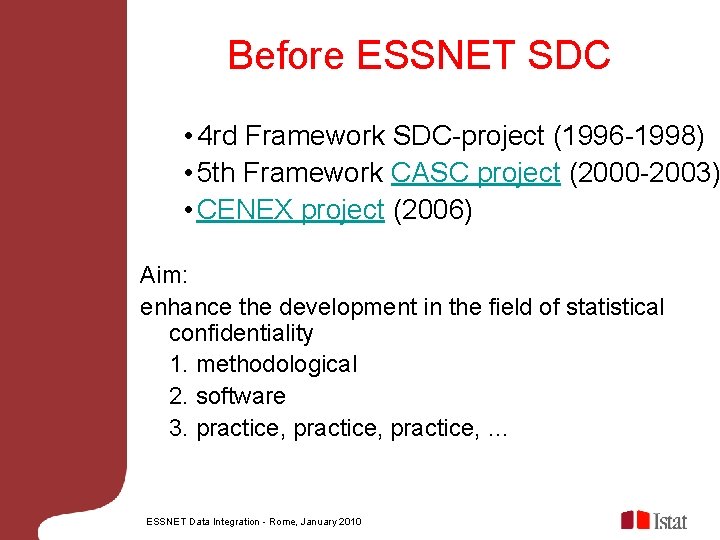
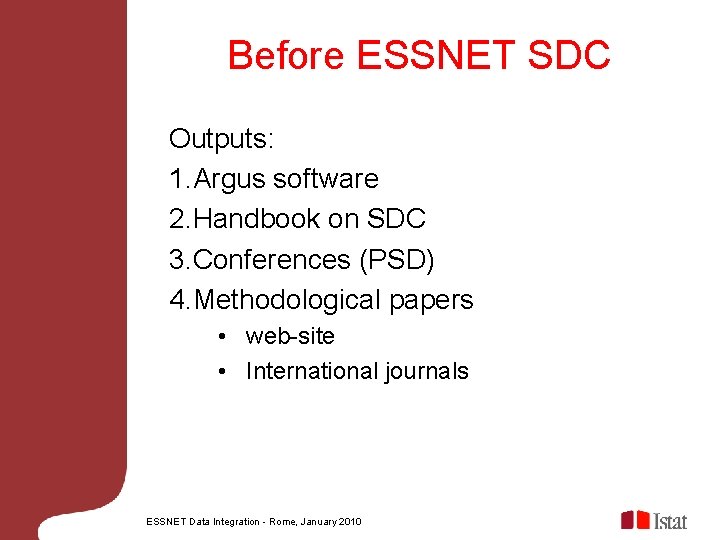
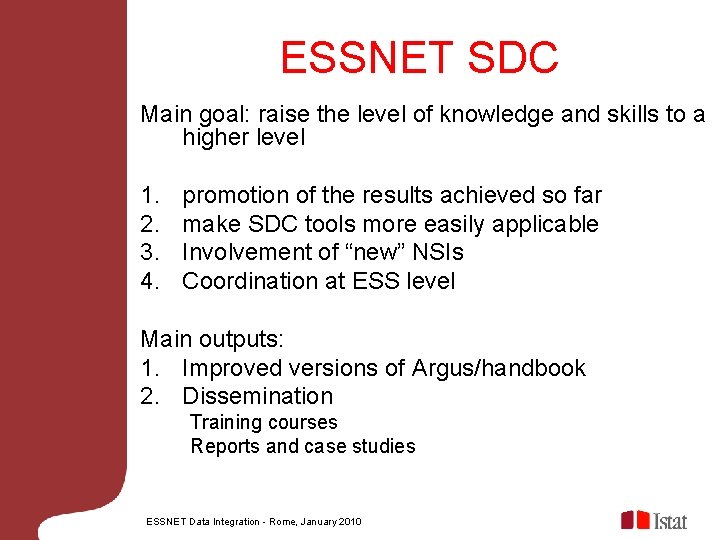
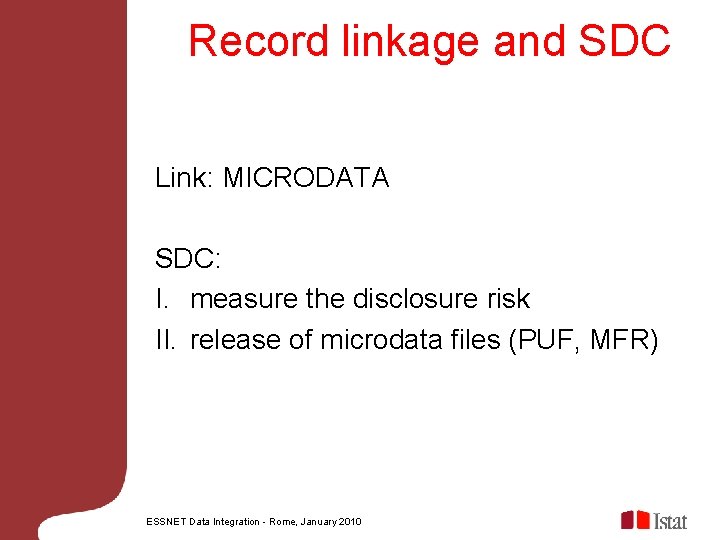
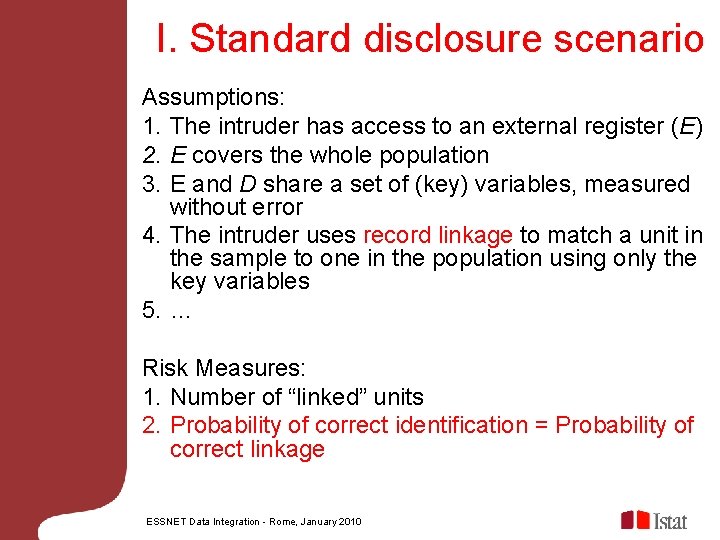
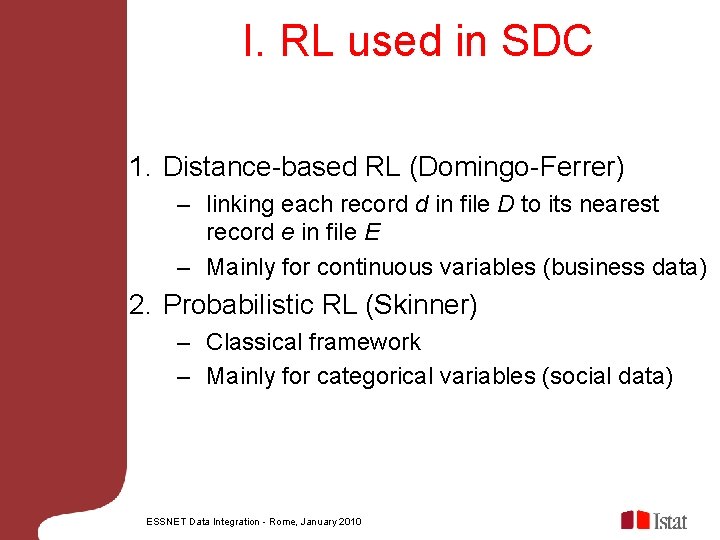
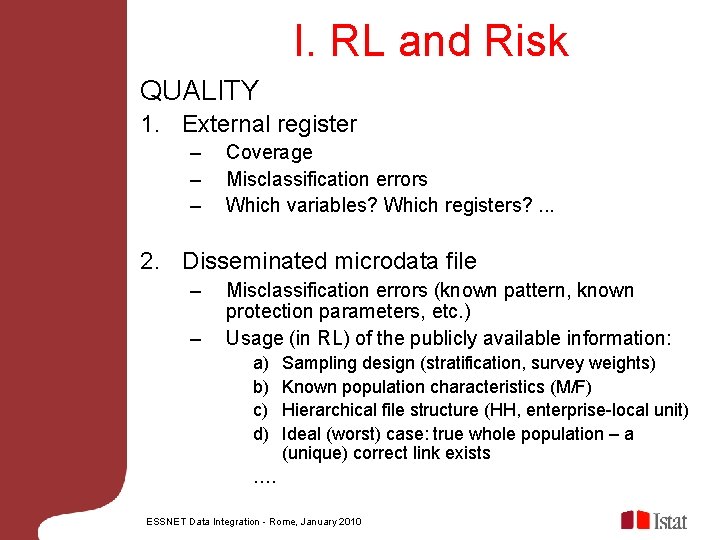
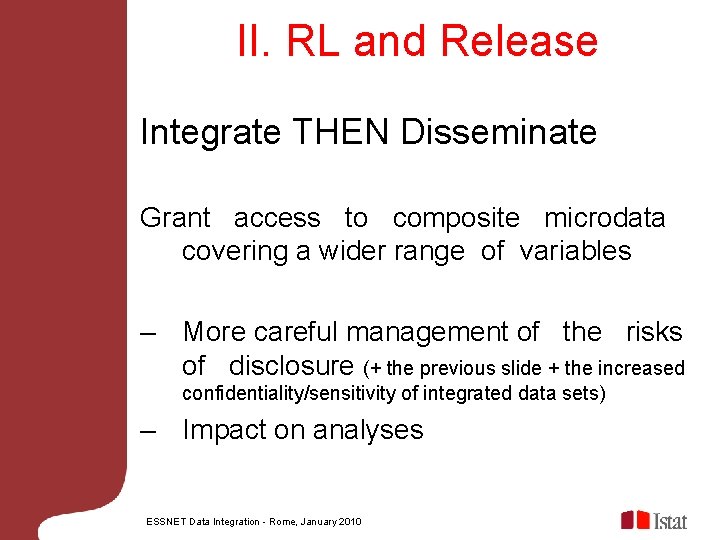
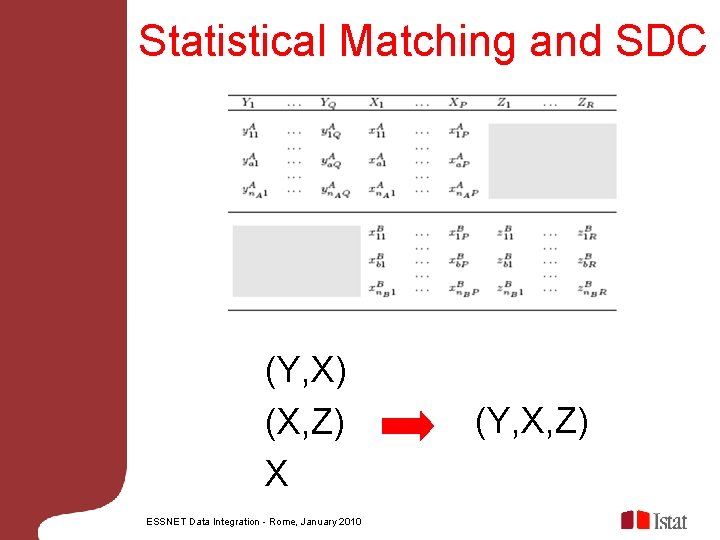
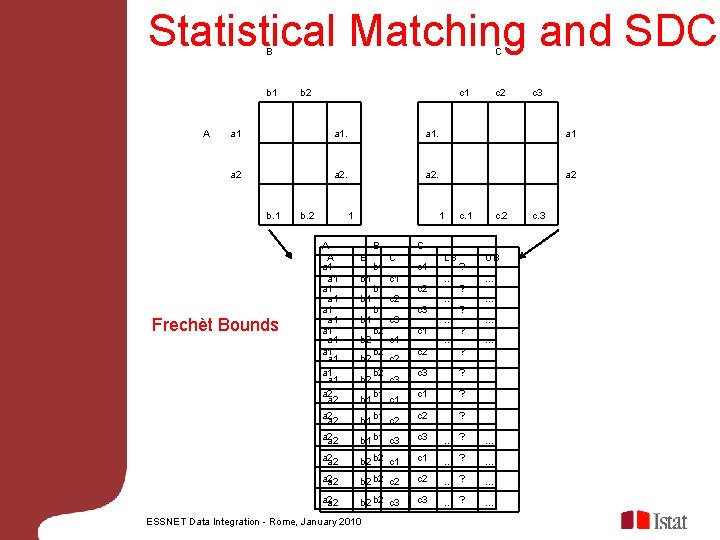
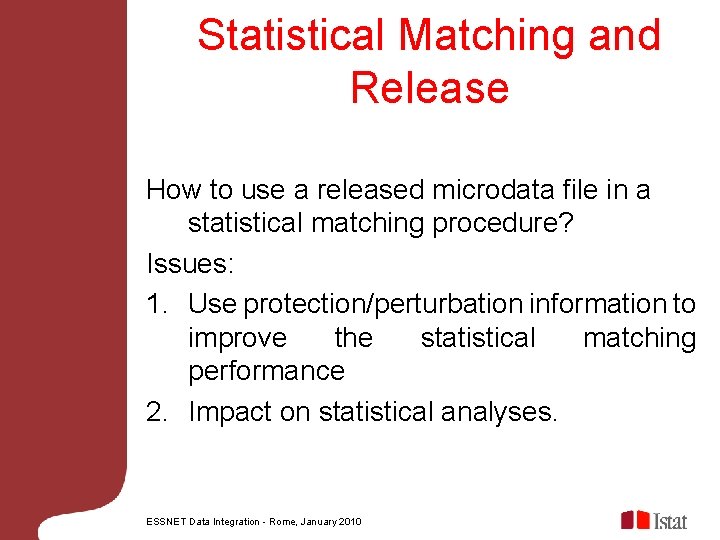
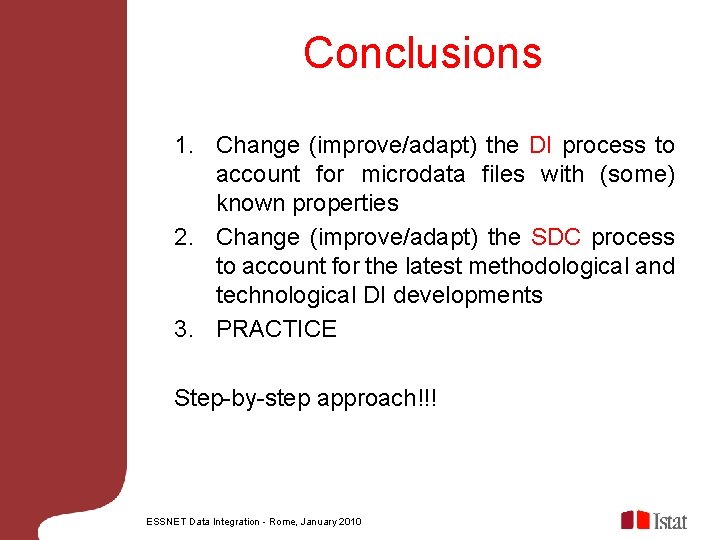
- Slides: 15
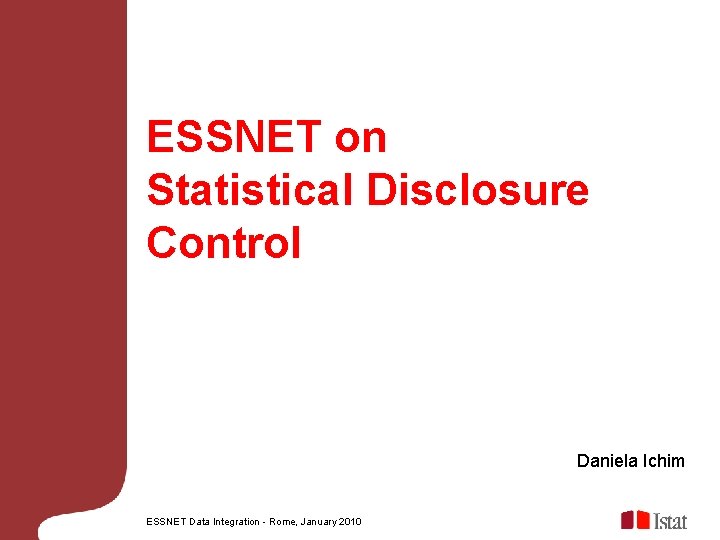
ESSNET on Statistical Disclosure Control Daniela Ichim ESSNET Data Integration - Rome, January 2010
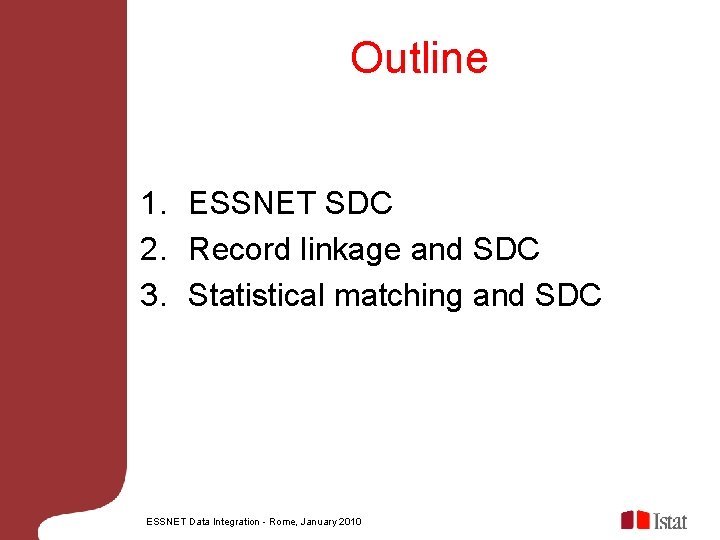
Outline 1. ESSNET SDC 2. Record linkage and SDC 3. Statistical matching and SDC ESSNET Data Integration - Rome, January 2010
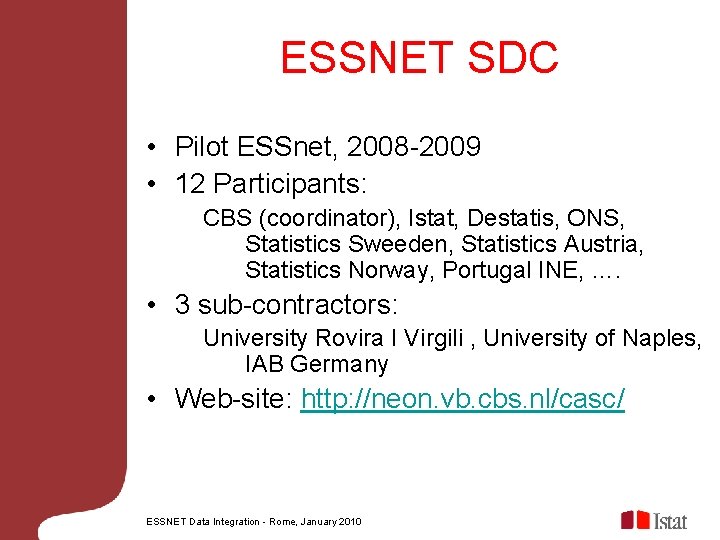
ESSNET SDC • Pilot ESSnet, 2008 -2009 • 12 Participants: CBS (coordinator), Istat, Destatis, ONS, Statistics Sweeden, Statistics Austria, Statistics Norway, Portugal INE, …. • 3 sub-contractors: University Rovira I Virgili , University of Naples, IAB Germany • Web-site: http: //neon. vb. cbs. nl/casc/ ESSNET Data Integration - Rome, January 2010
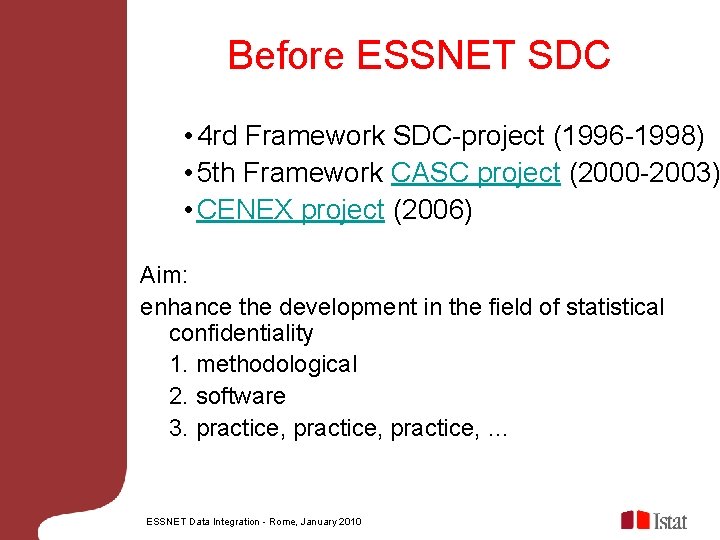
Before ESSNET SDC • 4 rd Framework SDC-project (1996 -1998) • 5 th Framework CASC project (2000 -2003) • CENEX project (2006) Aim: enhance the development in the field of statistical confidentiality 1. methodological 2. software 3. practice, … ESSNET Data Integration - Rome, January 2010
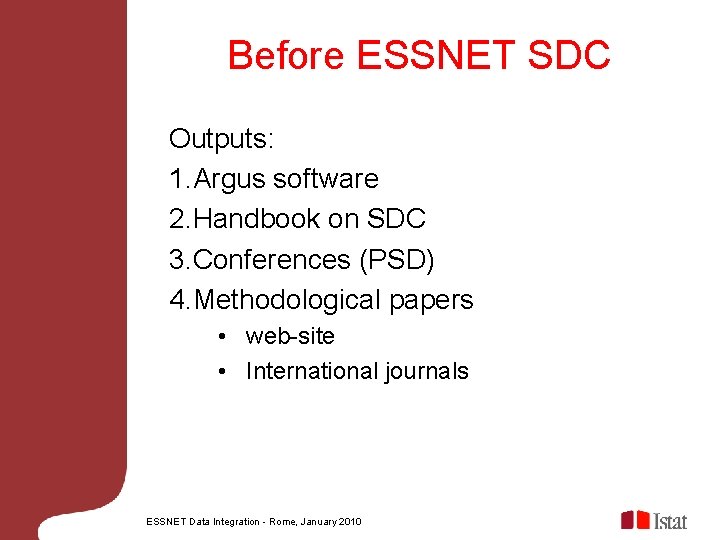
Before ESSNET SDC Outputs: 1. Argus software 2. Handbook on SDC 3. Conferences (PSD) 4. Methodological papers • web-site • International journals ESSNET Data Integration - Rome, January 2010
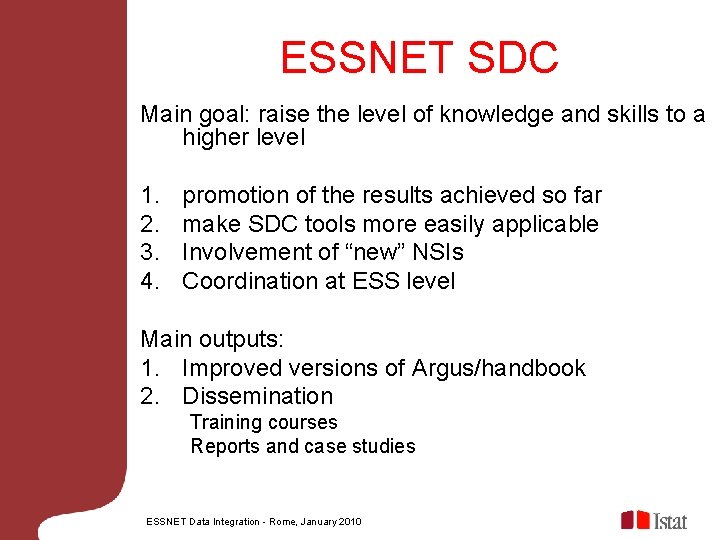
ESSNET SDC Main goal: raise the level of knowledge and skills to a higher level 1. 2. 3. 4. promotion of the results achieved so far make SDC tools more easily applicable Involvement of “new” NSIs Coordination at ESS level Main outputs: 1. Improved versions of Argus/handbook 2. Dissemination Training courses Reports and case studies ESSNET Data Integration - Rome, January 2010
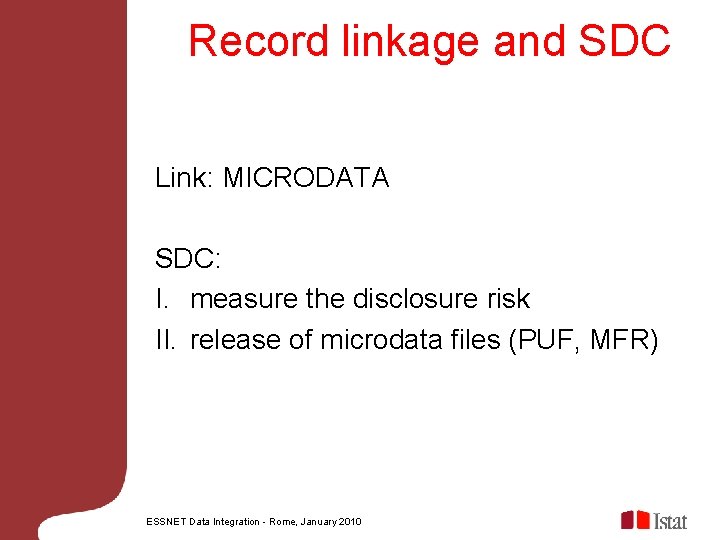
Record linkage and SDC Link: MICRODATA SDC: I. measure the disclosure risk II. release of microdata files (PUF, MFR) ESSNET Data Integration - Rome, January 2010
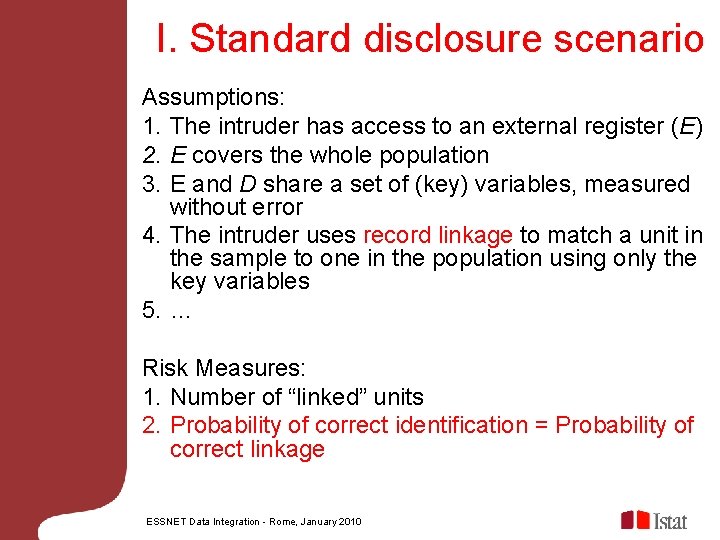
I. Standard disclosure scenario Assumptions: 1. The intruder has access to an external register (E) 2. E covers the whole population 3. E and D share a set of (key) variables, measured without error 4. The intruder uses record linkage to match a unit in the sample to one in the population using only the key variables 5. … Risk Measures: 1. Number of “linked” units 2. Probability of correct identification = Probability of correct linkage ESSNET Data Integration - Rome, January 2010
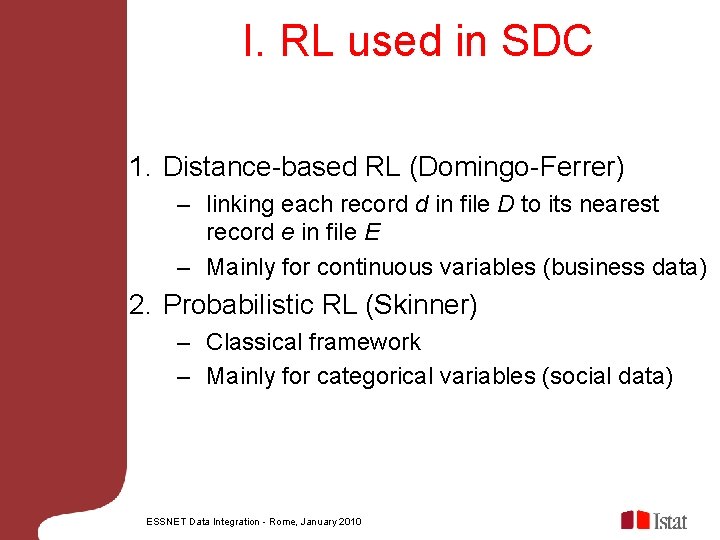
I. RL used in SDC 1. Distance-based RL (Domingo-Ferrer) – linking each record d in file D to its nearest record e in file E – Mainly for continuous variables (business data) 2. Probabilistic RL (Skinner) – Classical framework – Mainly for categorical variables (social data) ESSNET Data Integration - Rome, January 2010
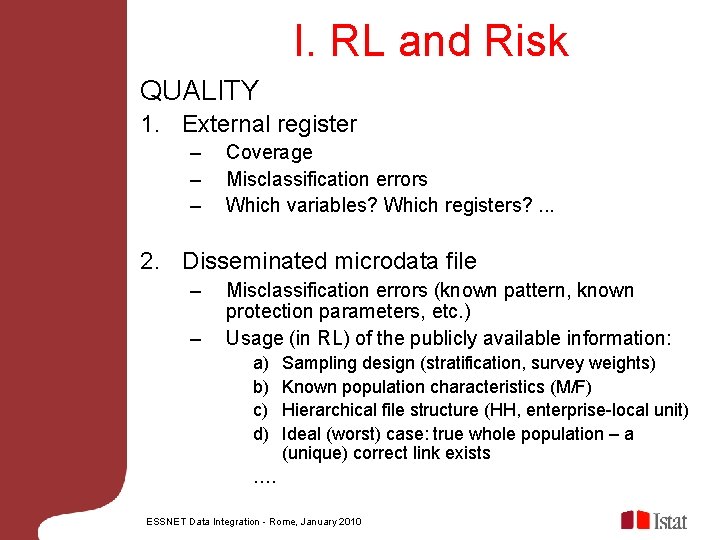
I. RL and Risk QUALITY 1. External register – – – Coverage Misclassification errors Which variables? Which registers? . . . 2. Disseminated microdata file – – Misclassification errors (known pattern, known protection parameters, etc. ) Usage (in RL) of the publicly available information: a) b) c) d) Sampling design (stratification, survey weights) Known population characteristics (M/F) Hierarchical file structure (HH, enterprise-local unit) Ideal (worst) case: true whole population – a (unique) correct link exists …. ESSNET Data Integration - Rome, January 2010
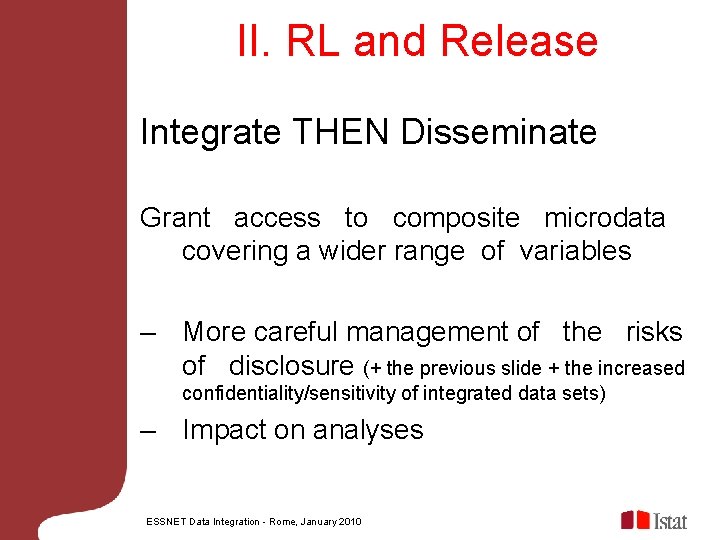
II. RL and Release Integrate THEN Disseminate Grant access to composite microdata covering a wider range of variables – More careful management of the risks of disclosure (+ the previous slide + the increased confidentiality/sensitivity of integrated data sets) – Impact on analyses ESSNET Data Integration - Rome, January 2010
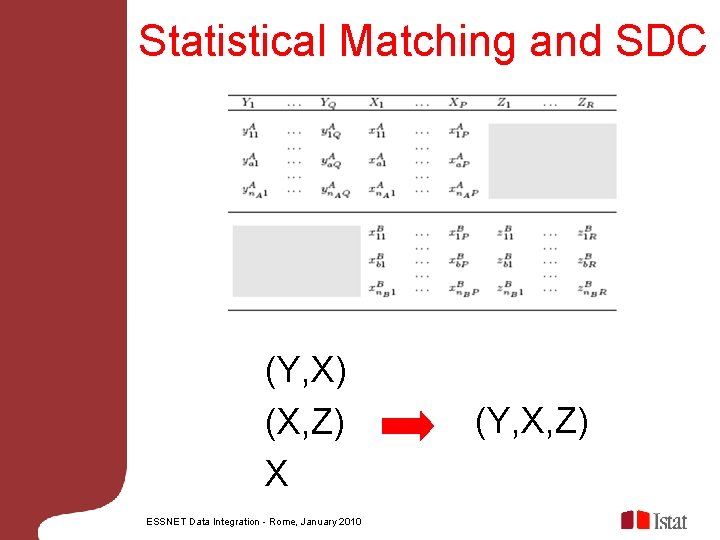
Statistical Matching and SDC (Y, X) (X, Z) X ESSNET Data Integration - Rome, January 2010 (Y, X, Z)
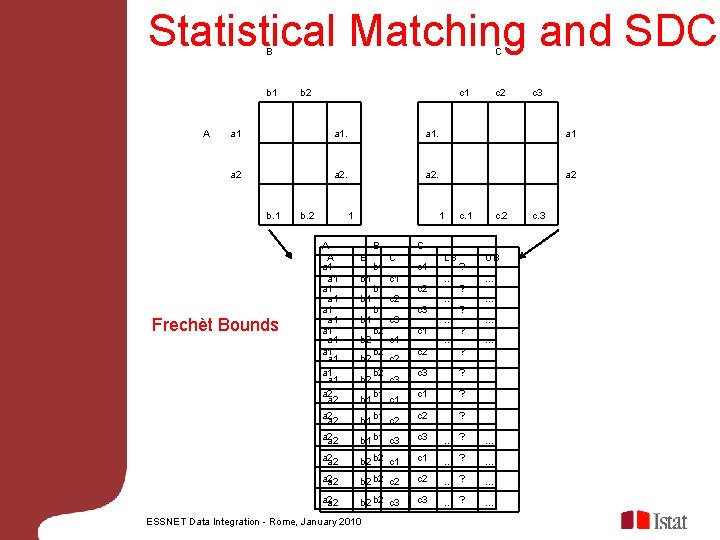
Statistical Matching and SDC B b 1 A C b 2 c 1 c 2 c 3 a 1 a 2 b. 1 Frechèt Bounds b. 2 1 A A a 1 a 1 a 1 a 2 1 B B b 1 b 1 b 1 b 2 b 2 b 2 b 1 c. 2 C C c 1 c 2 c 3 c 1 … … … … ? ? ? ? … … … … a 2 b 1 c 3 … ? … a 2 b 2 c 1 … ? … a 2 b 2 c 2 … ? … a 2 b 2 c 3 … ? … … ? UB b 1 c 2 LB a 2 ESSNET Data Integration - Rome, January 2010 b 1 c. 1 … c. 3
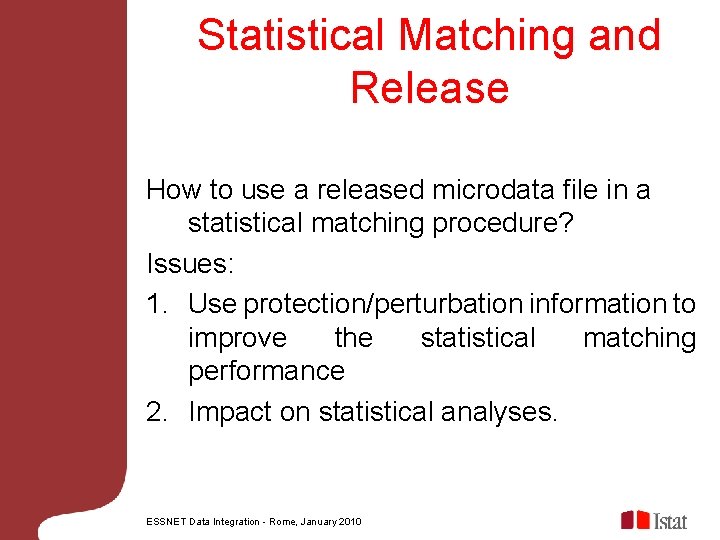
Statistical Matching and Release How to use a released microdata file in a statistical matching procedure? Issues: 1. Use protection/perturbation information to improve the statistical matching performance 2. Impact on statistical analyses. ESSNET Data Integration - Rome, January 2010
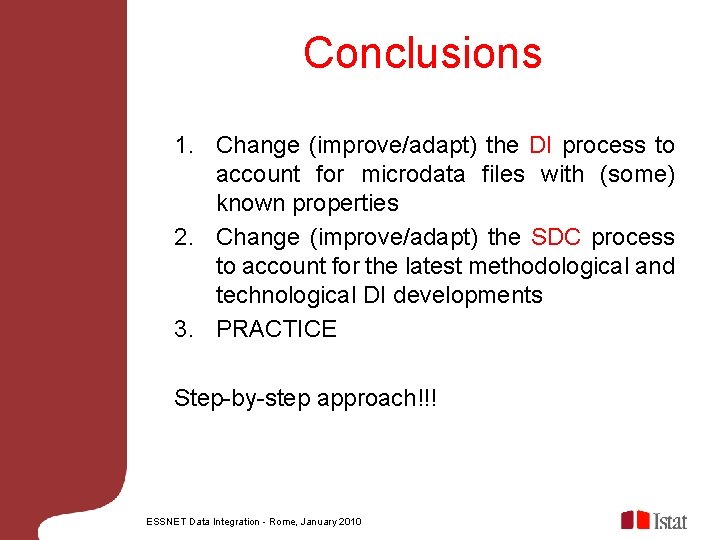
Conclusions 1. Change (improve/adapt) the DI process to account for microdata files with (some) known properties 2. Change (improve/adapt) the SDC process to account for the latest methodological and technological DI developments 3. PRACTICE Step-by-step approach!!! ESSNET Data Integration - Rome, January 2010