DifferencesinDifferences Liang Dai Alarm clock again Suppose Alice
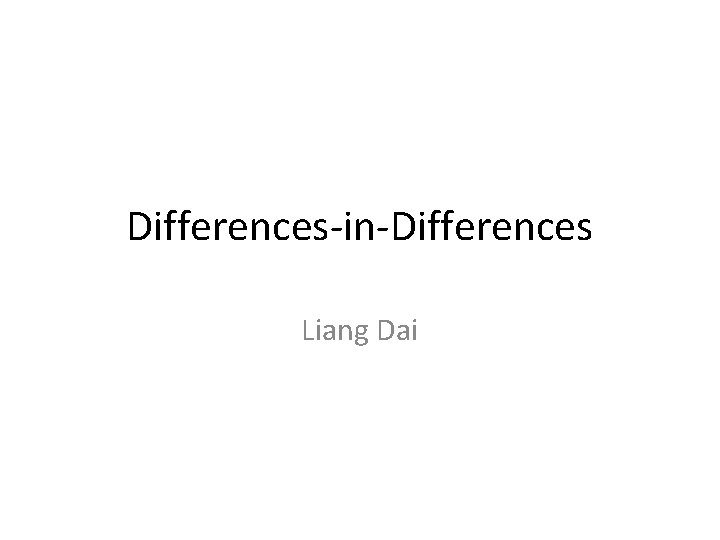
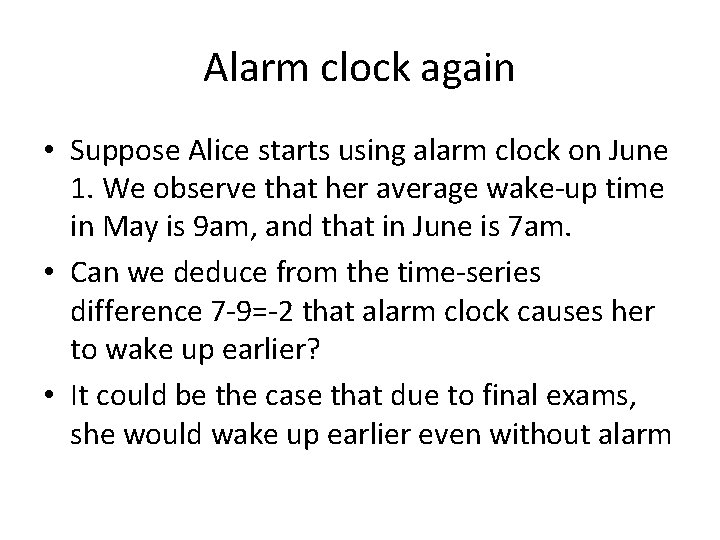
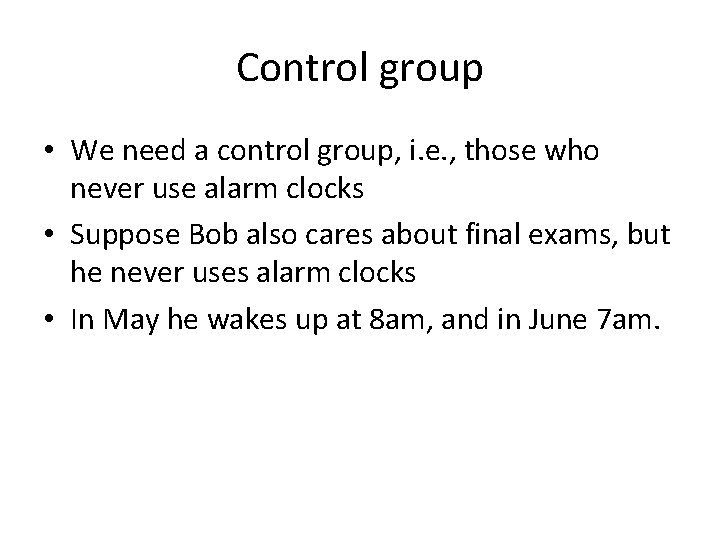
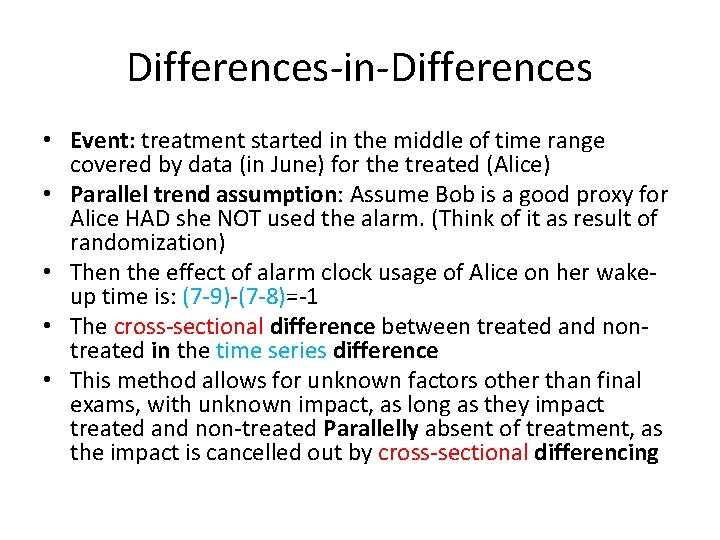
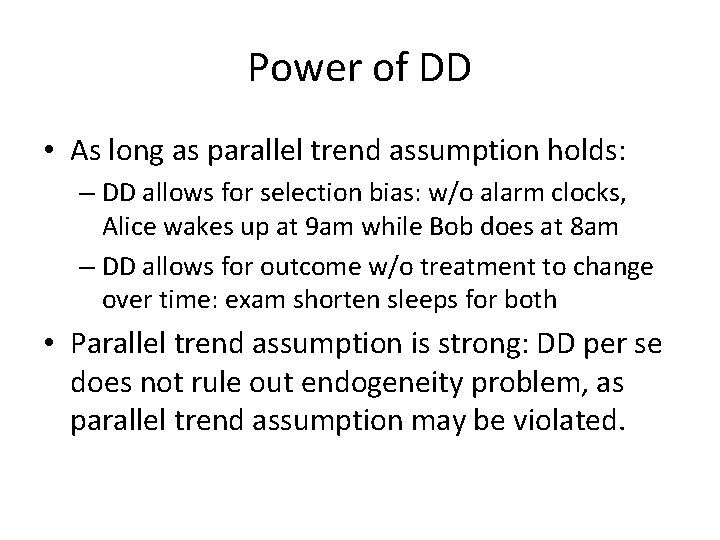
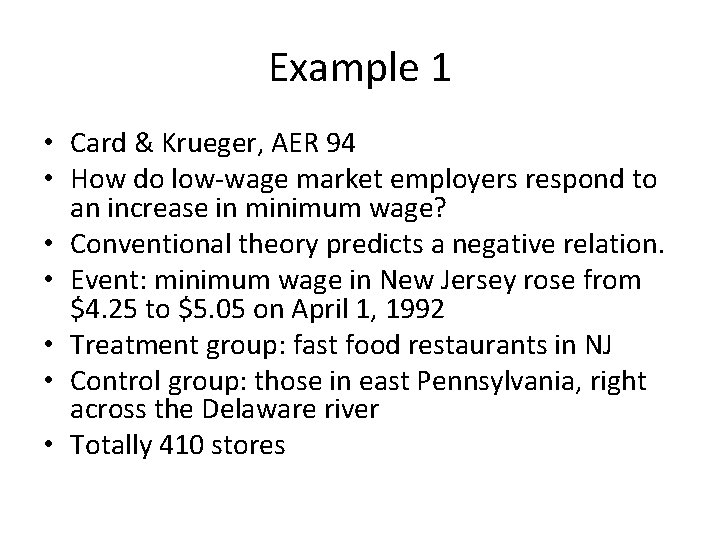
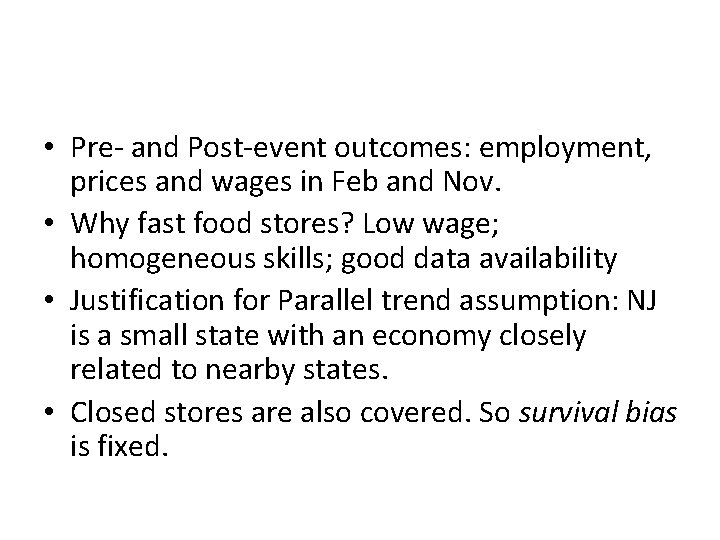
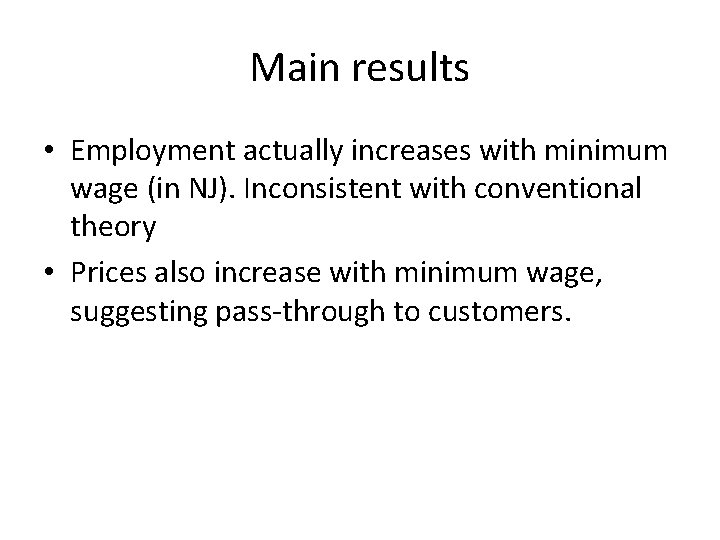
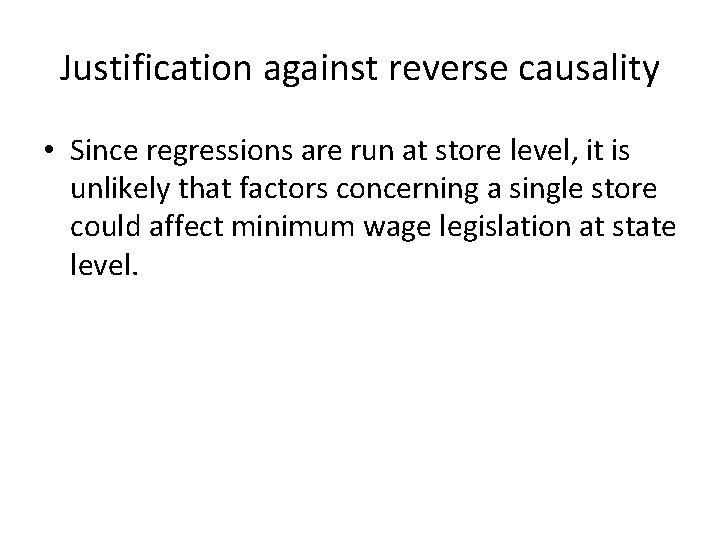
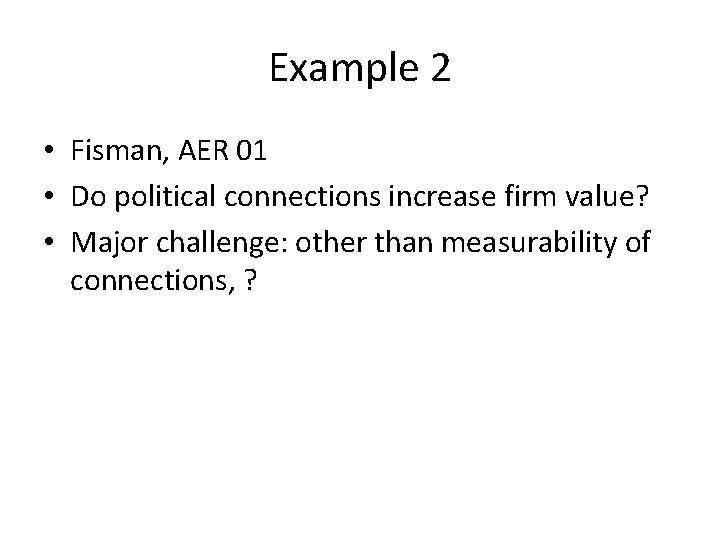
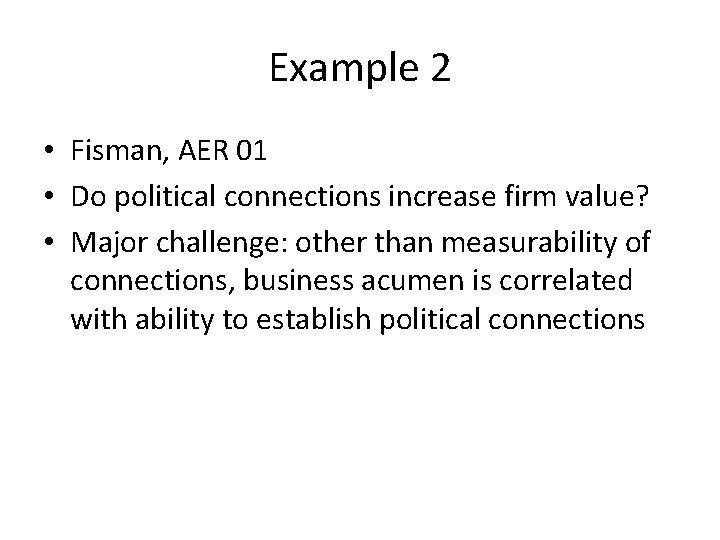
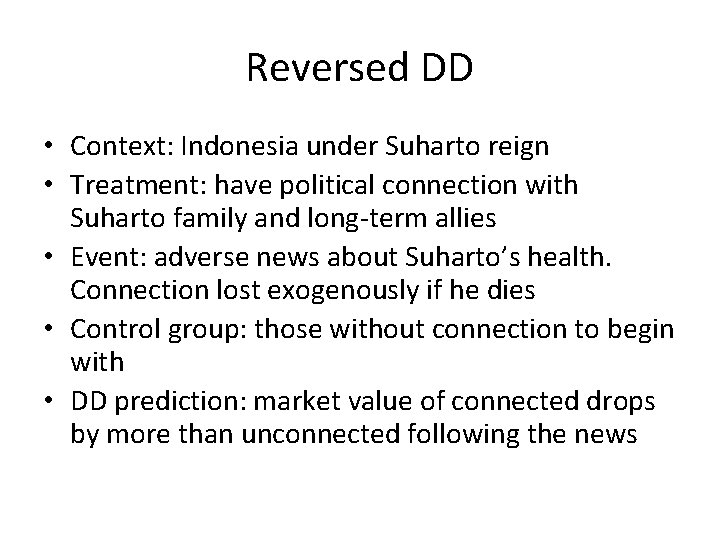
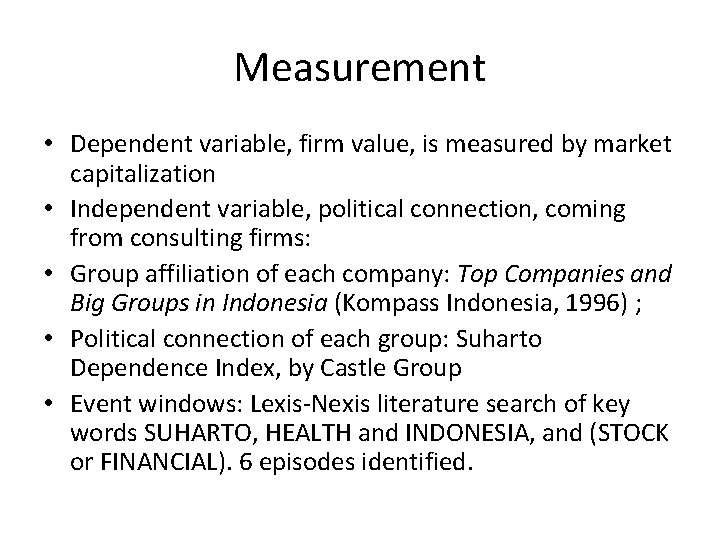
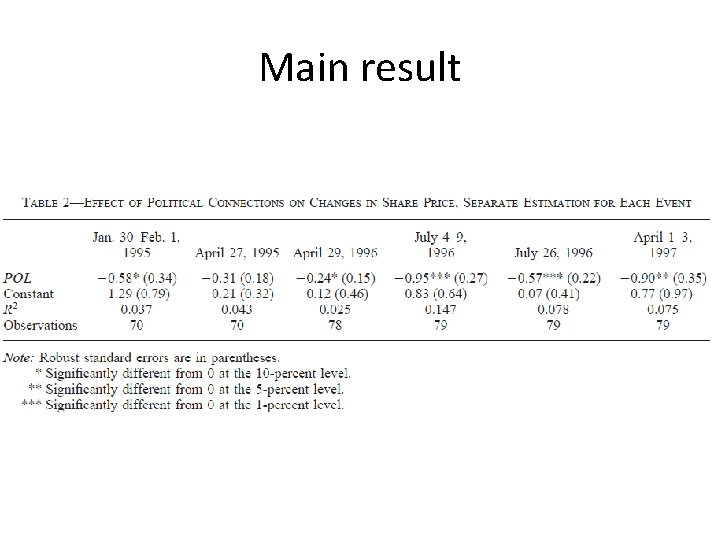
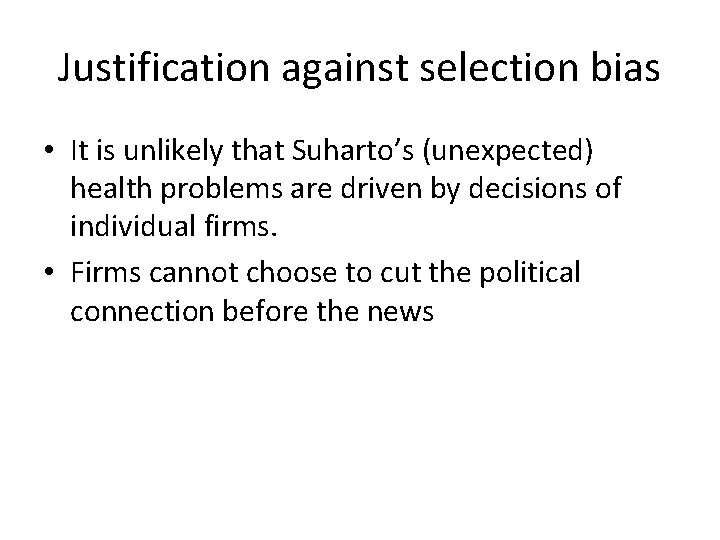
- Slides: 15
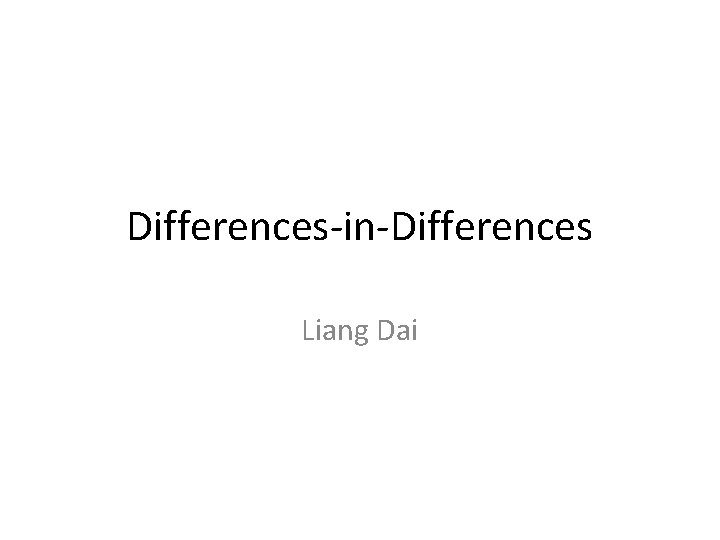
Differences-in-Differences Liang Dai
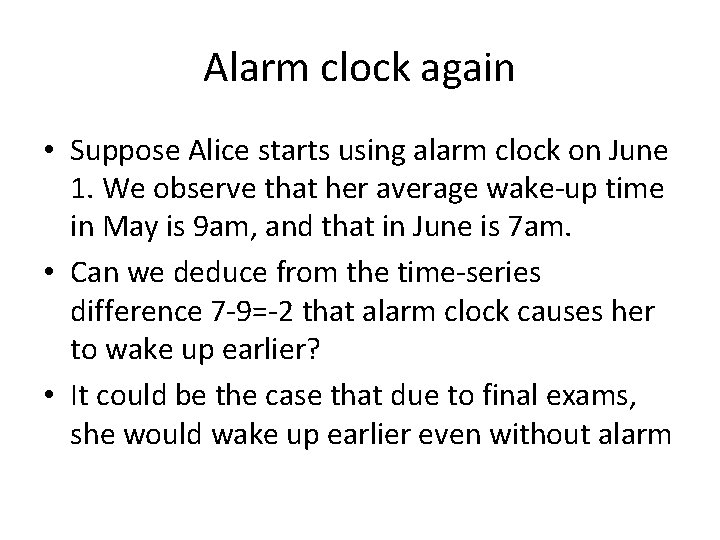
Alarm clock again • Suppose Alice starts using alarm clock on June 1. We observe that her average wake-up time in May is 9 am, and that in June is 7 am. • Can we deduce from the time-series difference 7 -9=-2 that alarm clock causes her to wake up earlier? • It could be the case that due to final exams, she would wake up earlier even without alarm
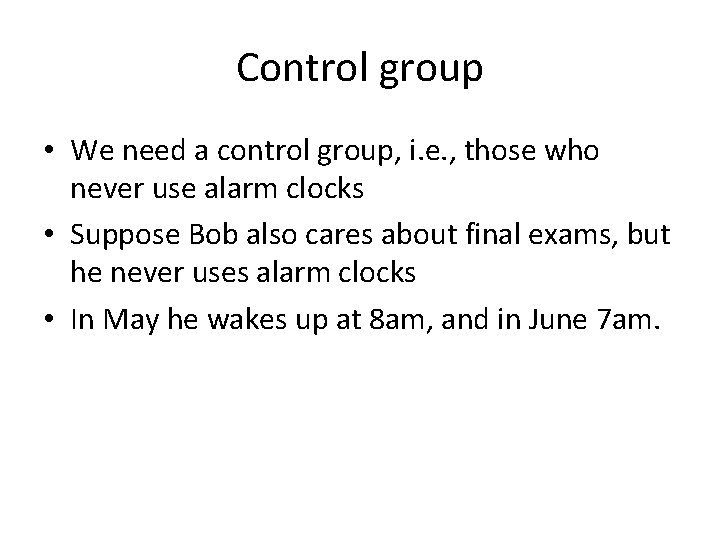
Control group • We need a control group, i. e. , those who never use alarm clocks • Suppose Bob also cares about final exams, but he never uses alarm clocks • In May he wakes up at 8 am, and in June 7 am.
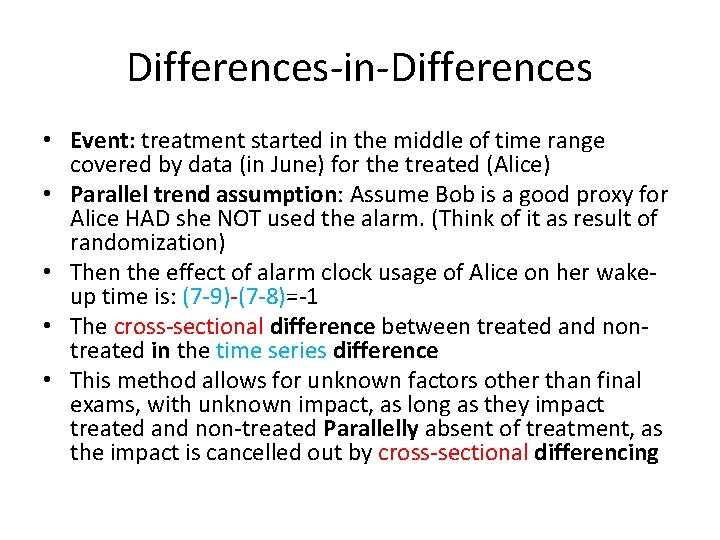
Differences-in-Differences • Event: treatment started in the middle of time range covered by data (in June) for the treated (Alice) • Parallel trend assumption: Assume Bob is a good proxy for Alice HAD she NOT used the alarm. (Think of it as result of randomization) • Then the effect of alarm clock usage of Alice on her wakeup time is: (7 -9)-(7 -8)=-1 • The cross-sectional difference between treated and nontreated in the time series difference • This method allows for unknown factors other than final exams, with unknown impact, as long as they impact treated and non-treated Parallelly absent of treatment, as the impact is cancelled out by cross-sectional differencing
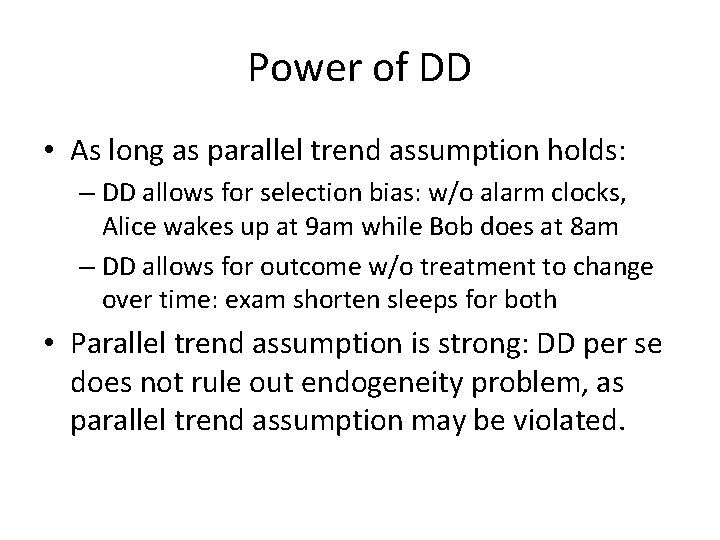
Power of DD • As long as parallel trend assumption holds: – DD allows for selection bias: w/o alarm clocks, Alice wakes up at 9 am while Bob does at 8 am – DD allows for outcome w/o treatment to change over time: exam shorten sleeps for both • Parallel trend assumption is strong: DD per se does not rule out endogeneity problem, as parallel trend assumption may be violated.
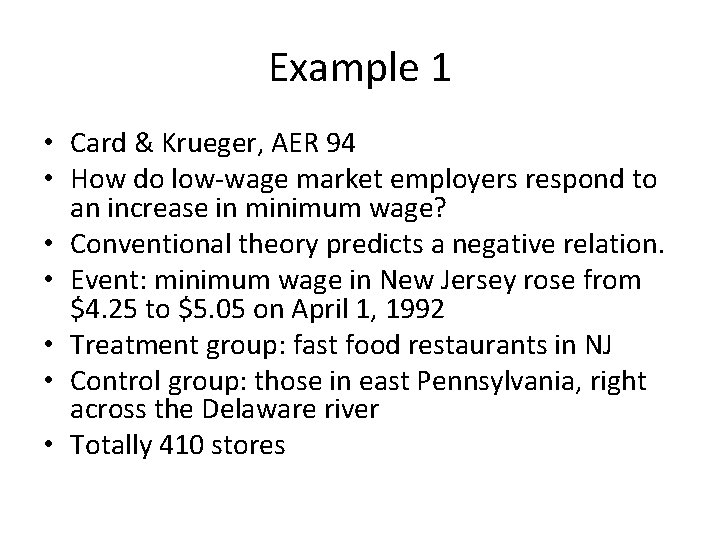
Example 1 • Card & Krueger, AER 94 • How do low-wage market employers respond to an increase in minimum wage? • Conventional theory predicts a negative relation. • Event: minimum wage in New Jersey rose from $4. 25 to $5. 05 on April 1, 1992 • Treatment group: fast food restaurants in NJ • Control group: those in east Pennsylvania, right across the Delaware river • Totally 410 stores
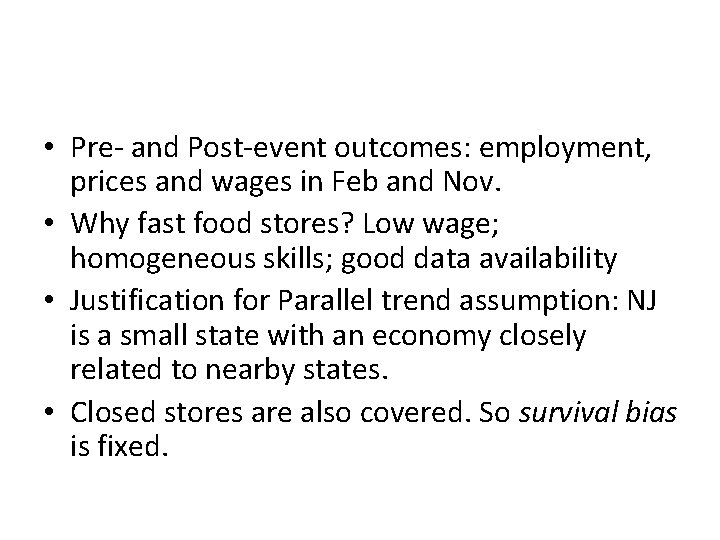
• Pre- and Post-event outcomes: employment, prices and wages in Feb and Nov. • Why fast food stores? Low wage; homogeneous skills; good data availability • Justification for Parallel trend assumption: NJ is a small state with an economy closely related to nearby states. • Closed stores are also covered. So survival bias is fixed.
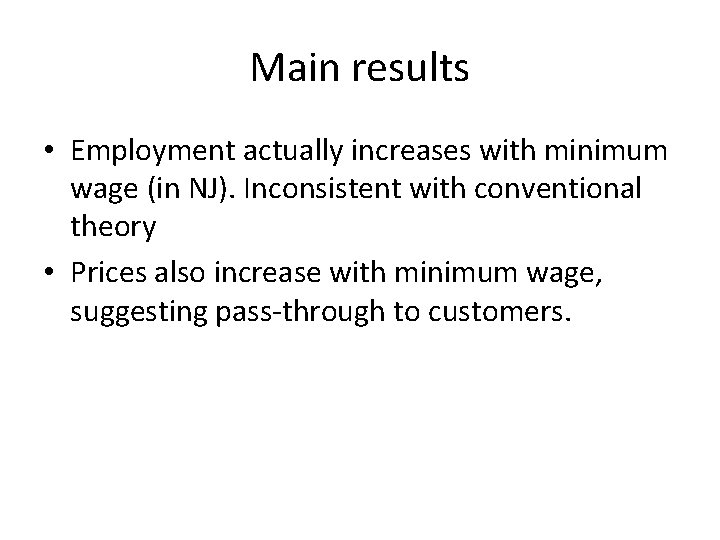
Main results • Employment actually increases with minimum wage (in NJ). Inconsistent with conventional theory • Prices also increase with minimum wage, suggesting pass-through to customers.
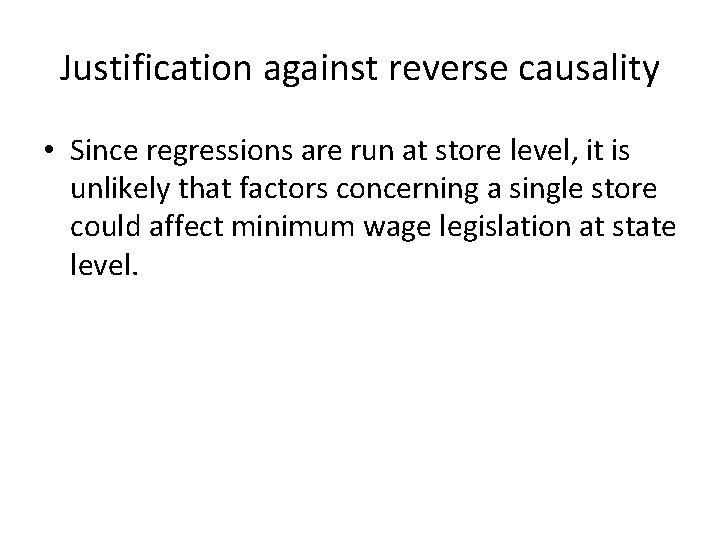
Justification against reverse causality • Since regressions are run at store level, it is unlikely that factors concerning a single store could affect minimum wage legislation at state level.
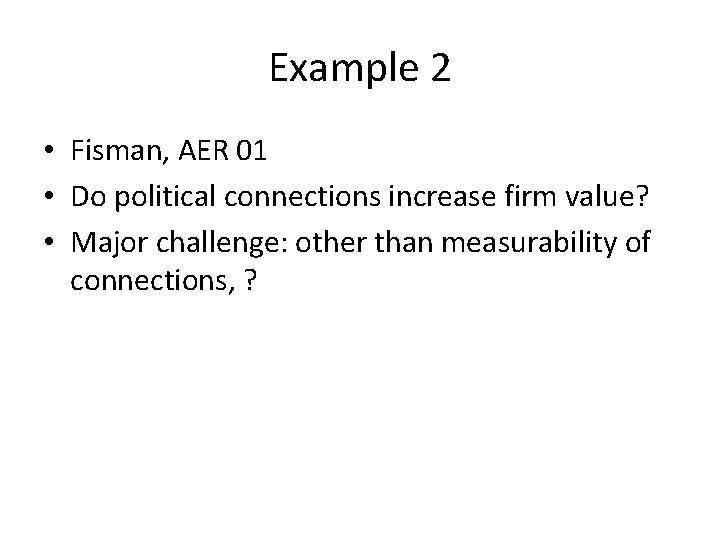
Example 2 • Fisman, AER 01 • Do political connections increase firm value? • Major challenge: other than measurability of connections, ?
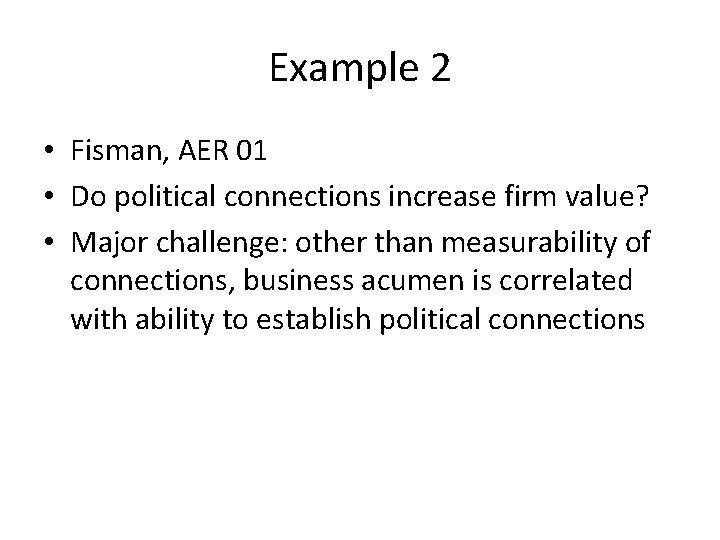
Example 2 • Fisman, AER 01 • Do political connections increase firm value? • Major challenge: other than measurability of connections, business acumen is correlated with ability to establish political connections
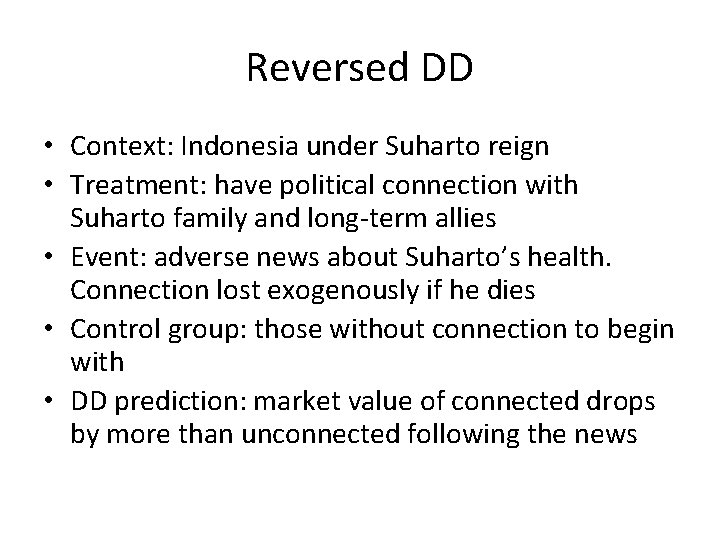
Reversed DD • Context: Indonesia under Suharto reign • Treatment: have political connection with Suharto family and long-term allies • Event: adverse news about Suharto’s health. Connection lost exogenously if he dies • Control group: those without connection to begin with • DD prediction: market value of connected drops by more than unconnected following the news
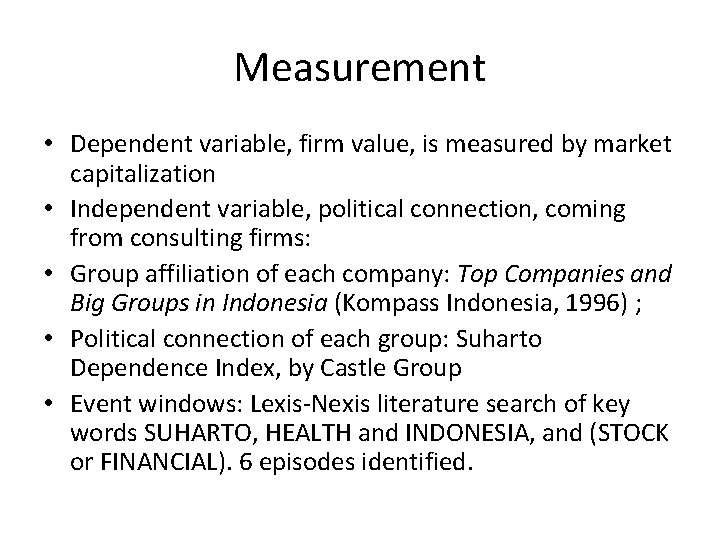
Measurement • Dependent variable, firm value, is measured by market capitalization • Independent variable, political connection, coming from consulting firms: • Group affiliation of each company: Top Companies and Big Groups in Indonesia (Kompass Indonesia, 1996) ; • Political connection of each group: Suharto Dependence Index, by Castle Group • Event windows: Lexis-Nexis literature search of key words SUHARTO, HEALTH and INDONESIA, and (STOCK or FINANCIAL). 6 episodes identified.
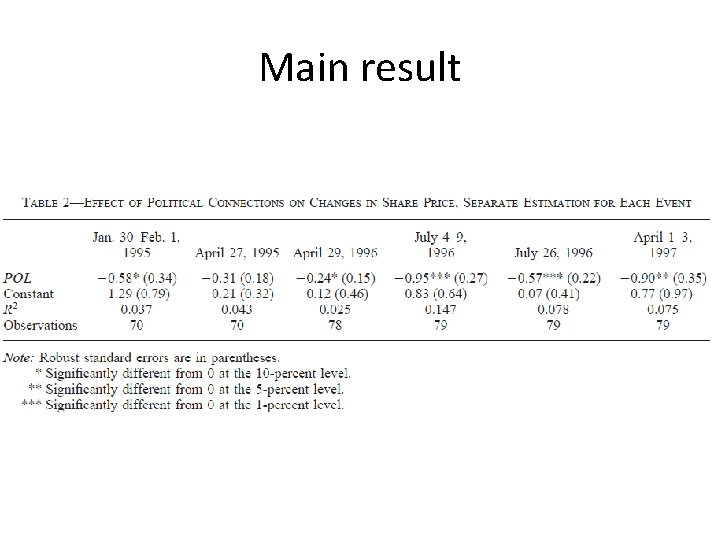
Main result
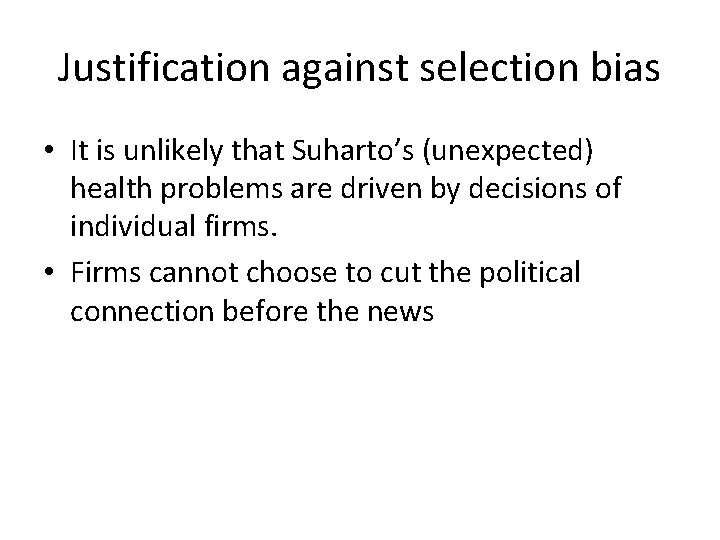
Justification against selection bias • It is unlikely that Suharto’s (unexpected) health problems are driven by decisions of individual firms. • Firms cannot choose to cut the political connection before the news
Use case diagram for alarm clock
Raise and rise again until lambs become lions
The outsiders signposts
Again and again signpost picture books
Alliteration song lyrics
My alarm clock yells at me every morning
Ano ang gamit ng tunog ng alarm clock
Raspberry pi smart alarm clock
Alarm clock energy transformation
Personification in sentence
Energy transformation of clock
Digital alarm clock class diagram
Alarm clock class diagram
Dharma initiative alarm clock
Whats a quater pass 8
Pintos manual