Design and analysis of animal production experiments Dr
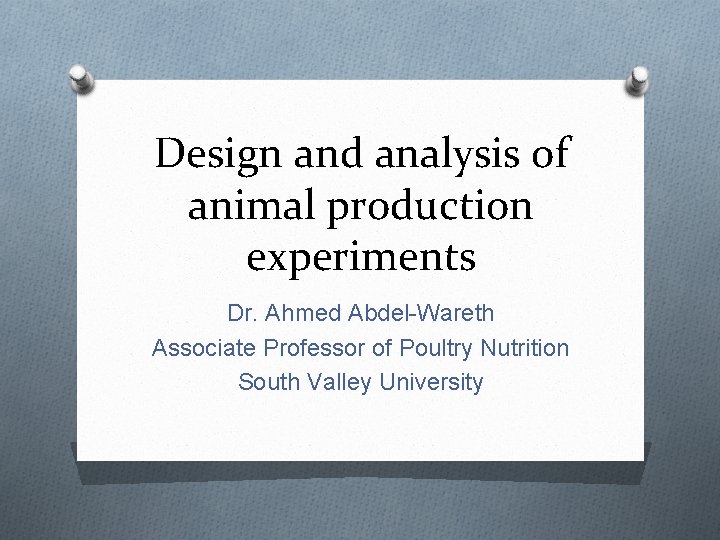
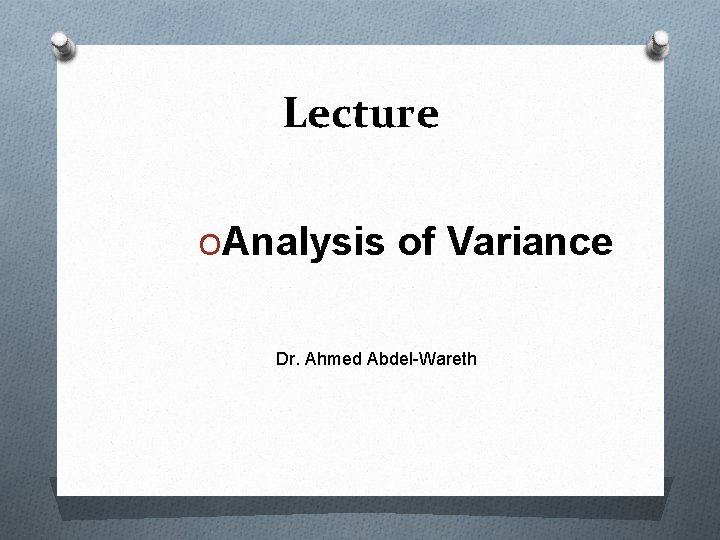
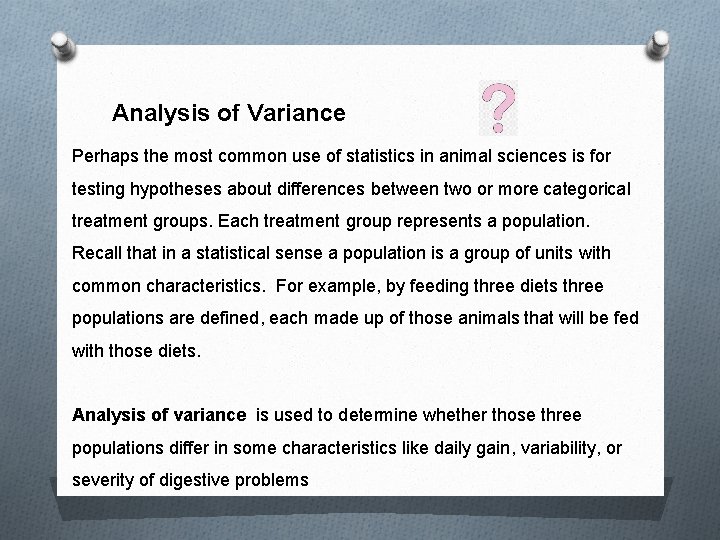
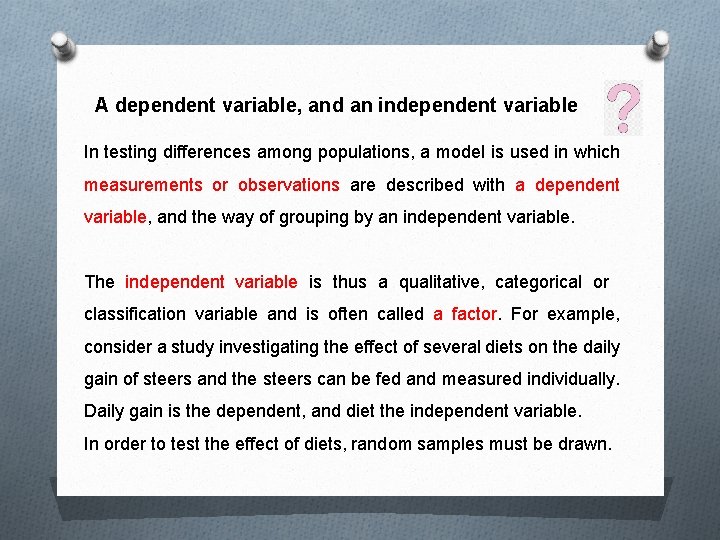
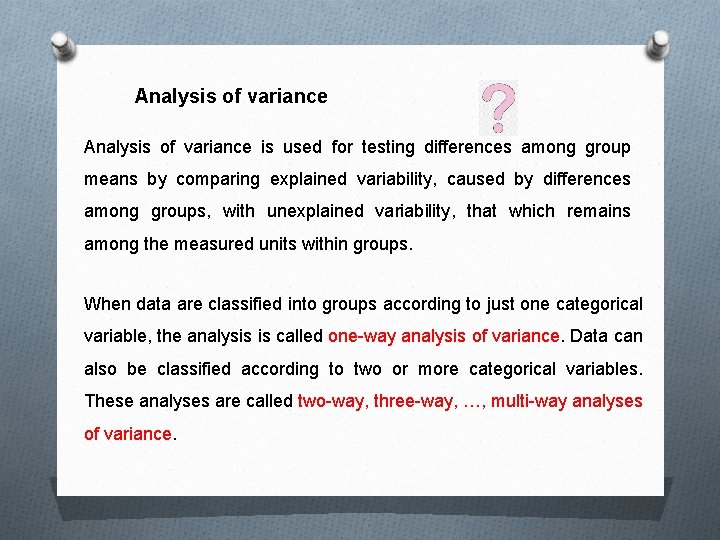
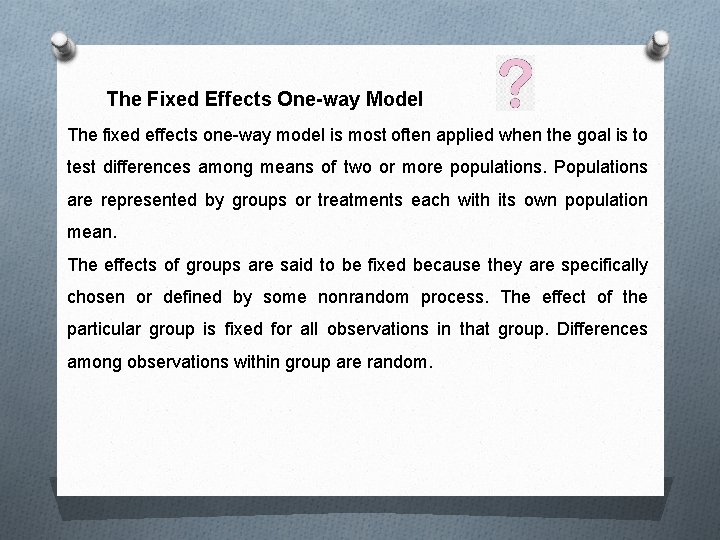
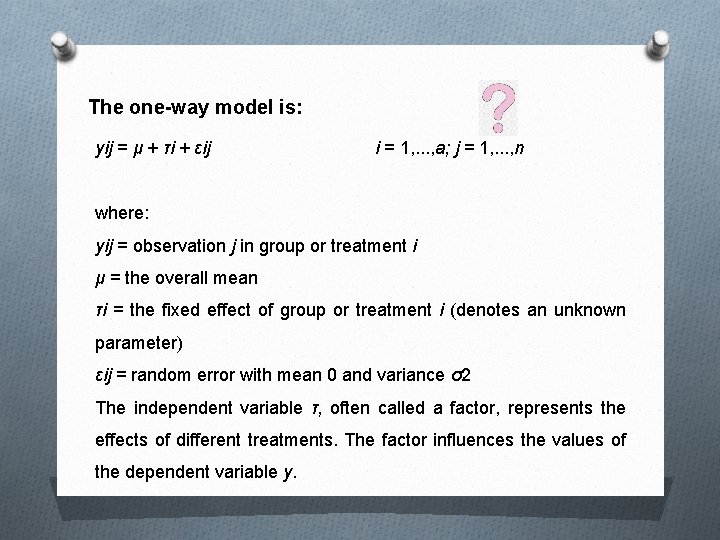
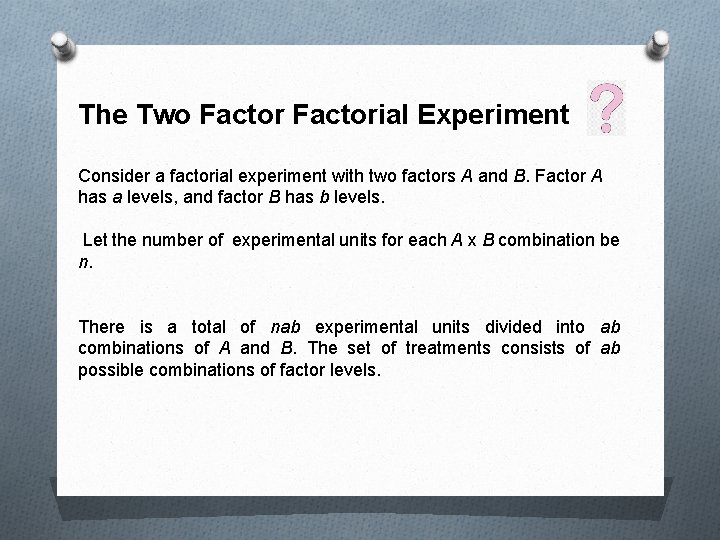
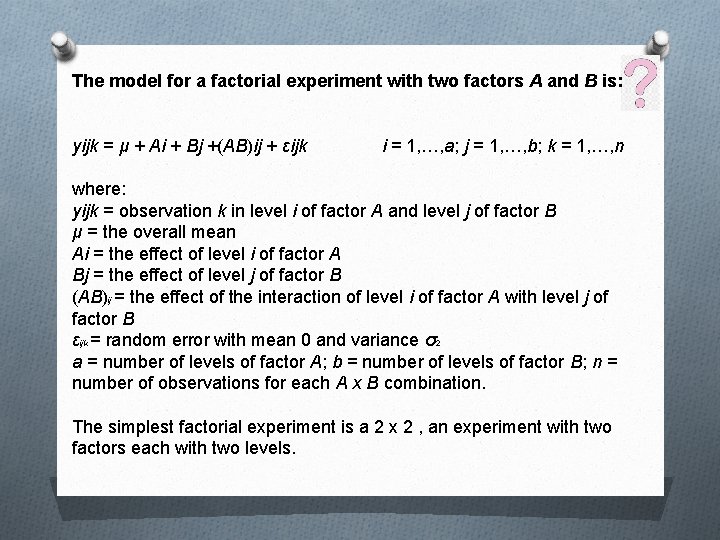
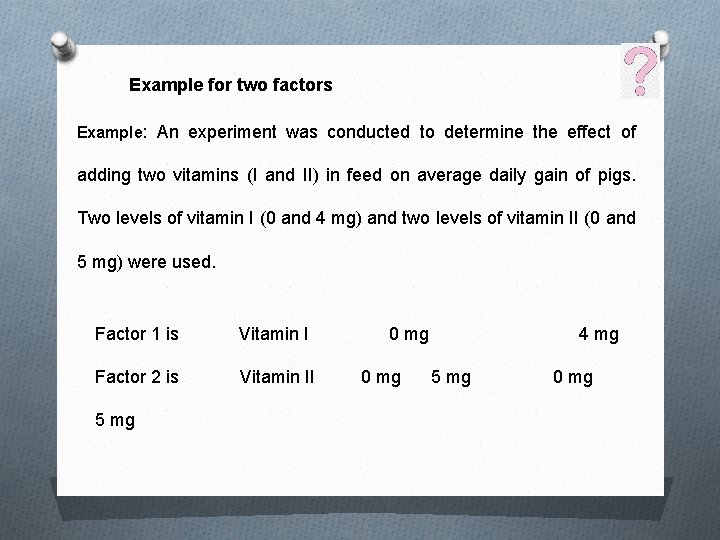
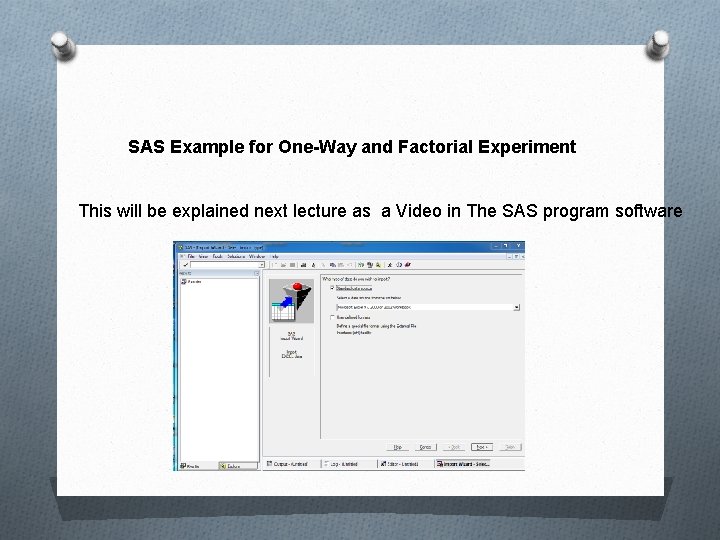
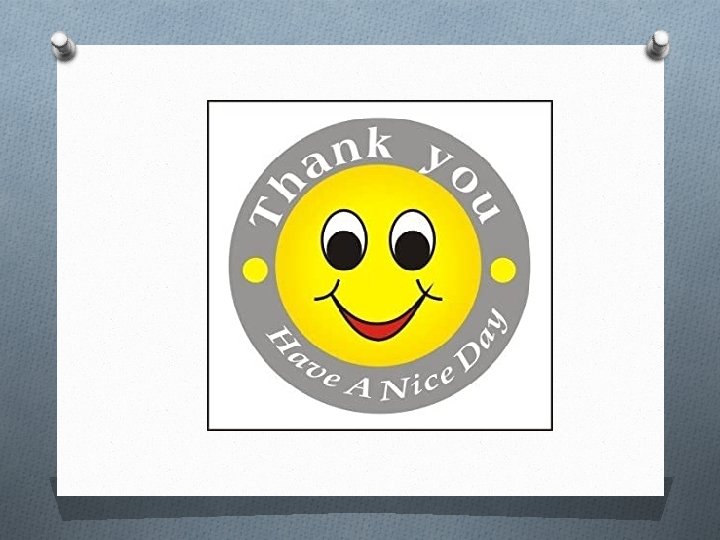
- Slides: 12
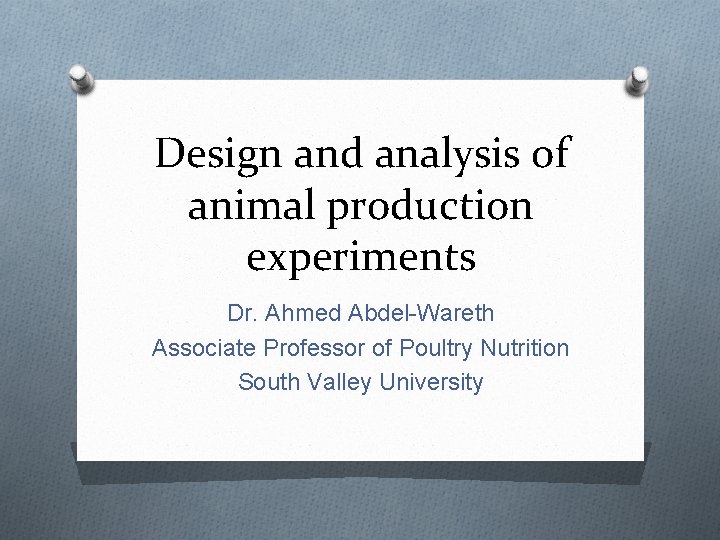
Design and analysis of animal production experiments Dr. Ahmed Abdel-Wareth Associate Professor of Poultry Nutrition South Valley University
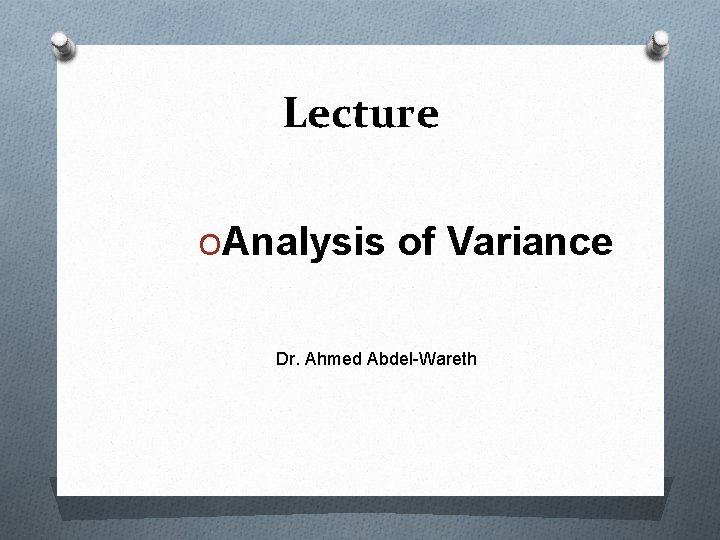
Lecture OAnalysis of Variance Dr. Ahmed Abdel-Wareth
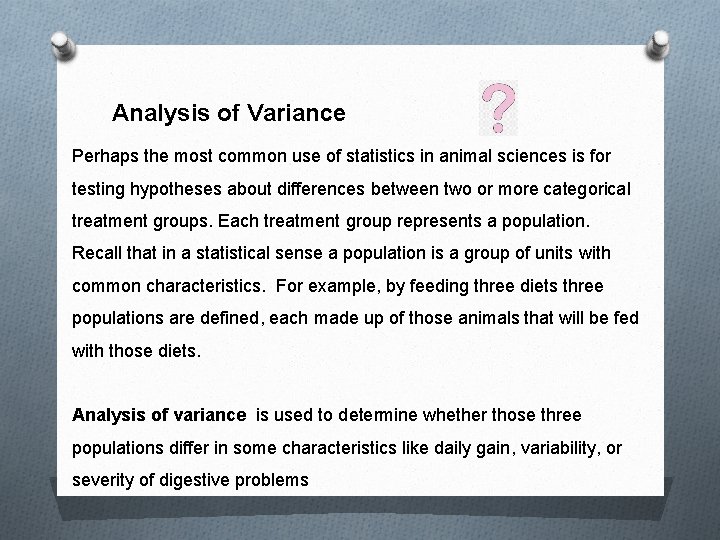
Analysis of Variance Perhaps the most common use of statistics in animal sciences is for testing hypotheses about differences between two or more categorical treatment groups. Each treatment group represents a population. Recall that in a statistical sense a population is a group of units with common characteristics. For example, by feeding three diets three populations are defined, each made up of those animals that will be fed with those diets. Analysis of variance is used to determine whether those three populations differ in some characteristics like daily gain, variability, or severity of digestive problems
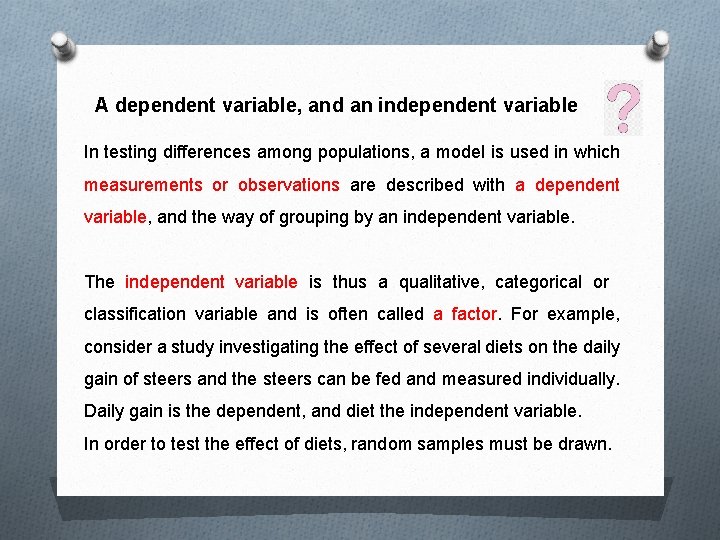
A dependent variable, and an independent variable In testing differences among populations, a model is used in which measurements or observations are described with a dependent variable, and the way of grouping by an independent variable. The independent variable is thus a qualitative, categorical or classification variable and is often called a factor. For example, consider a study investigating the effect of several diets on the daily gain of steers and the steers can be fed and measured individually. Daily gain is the dependent, and diet the independent variable. In order to test the effect of diets, random samples must be drawn.
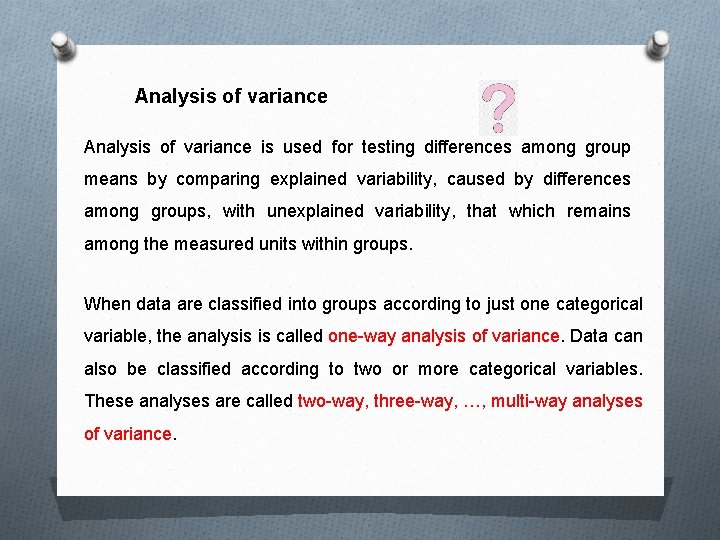
Analysis of variance is used for testing differences among group means by comparing explained variability, caused by differences among groups, with unexplained variability, that which remains among the measured units within groups. When data are classified into groups according to just one categorical variable, the analysis is called one-way analysis of variance. Data can also be classified according to two or more categorical variables. These analyses are called two-way, three-way, …, multi-way analyses of variance.
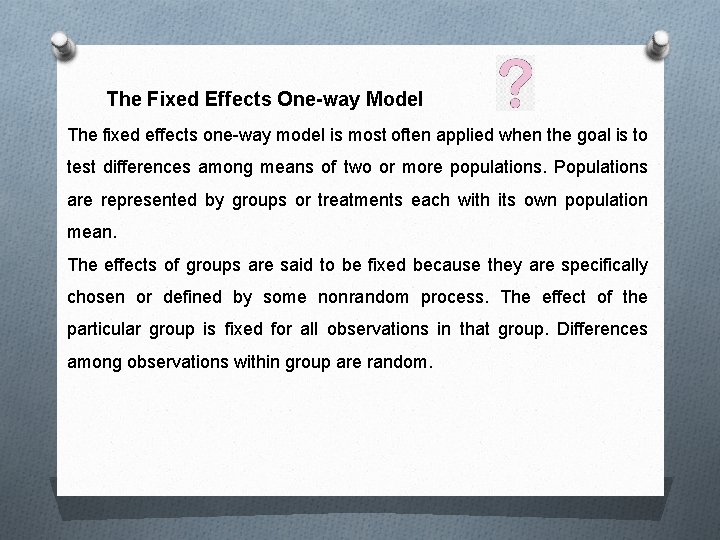
The Fixed Effects One-way Model The fixed effects one-way model is most often applied when the goal is to test differences among means of two or more populations. Populations are represented by groups or treatments each with its own population mean. The effects of groups are said to be fixed because they are specifically chosen or defined by some nonrandom process. The effect of the particular group is fixed for all observations in that group. Differences among observations within group are random.
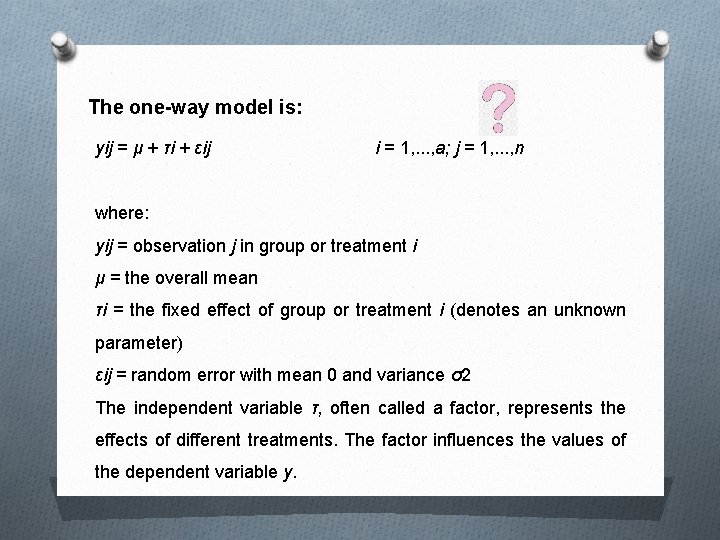
The one-way model is: yij = μ + τi + εij i = 1, . . . , a; j = 1, . . . , n where: yij = observation j in group or treatment i μ = the overall mean τi = the fixed effect of group or treatment i (denotes an unknown parameter) εij = random error with mean 0 and variance σ2 The independent variable τ, often called a factor, represents the effects of different treatments. The factor influences the values of the dependent variable y.
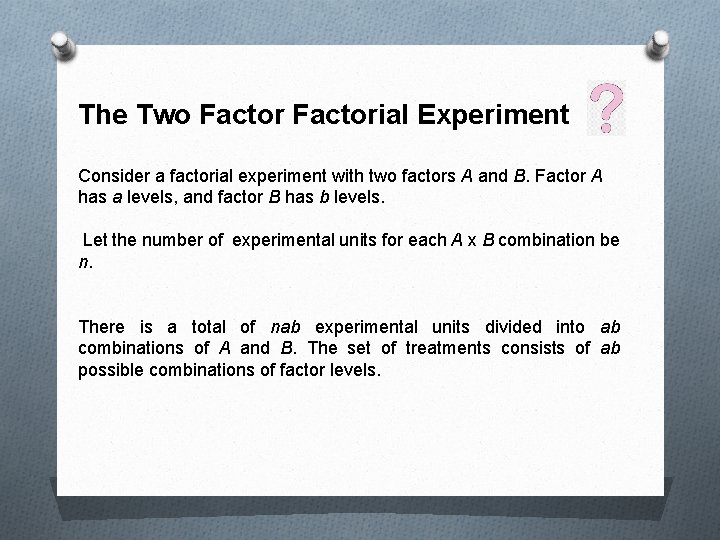
The Two Factorial Experiment Consider a factorial experiment with two factors A and B. Factor A has a levels, and factor B has b levels. Let the number of experimental units for each A x B combination be n. There is a total of nab experimental units divided into ab combinations of A and B. The set of treatments consists of ab possible combinations of factor levels.
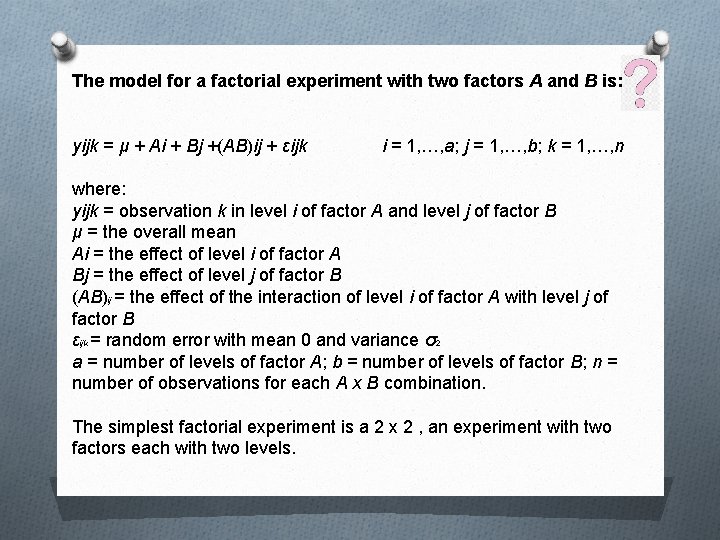
The model for a factorial experiment with two factors A and B is: yijk = μ + Ai + Bj +(AB)ij + εijk i = 1, …, a; j = 1, …, b; k = 1, …, n where: yijk = observation k in level i of factor A and level j of factor B μ = the overall mean Ai = the effect of level i of factor A Bj = the effect of level j of factor B (AB)ij = the effect of the interaction of level i of factor A with level j of factor B εijk = random error with mean 0 and variance σ2 a = number of levels of factor A; b = number of levels of factor B; n = number of observations for each A x B combination. The simplest factorial experiment is a 2 x 2 , an experiment with two factors each with two levels.
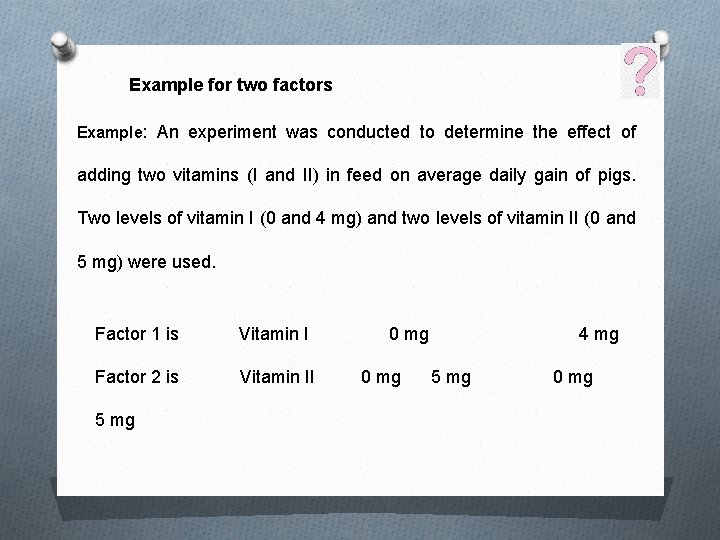
Example for two factors Example: An experiment was conducted to determine the effect of adding two vitamins (I and II) in feed on average daily gain of pigs. Two levels of vitamin I (0 and 4 mg) and two levels of vitamin II (0 and 5 mg) were used. Factor 1 is Vitamin I Factor 2 is Vitamin II 5 mg 0 mg 4 mg 5 mg 0 mg
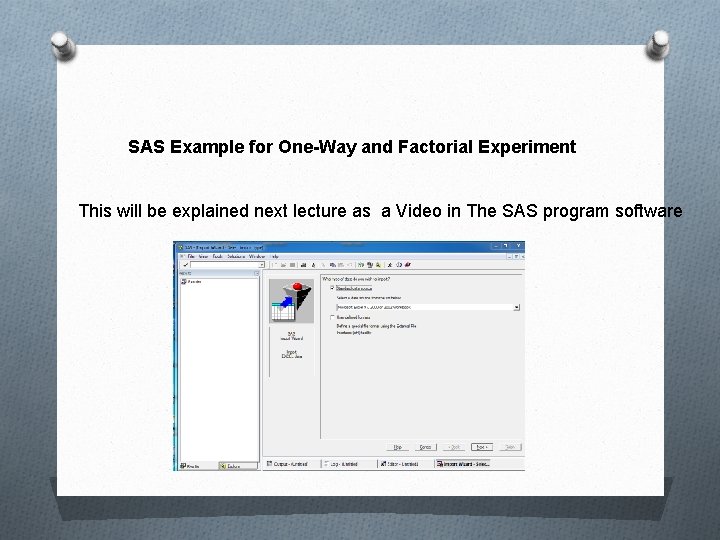
SAS Example for One-Way and Factorial Experiment This will be explained next lecture as a Video in The SAS program software
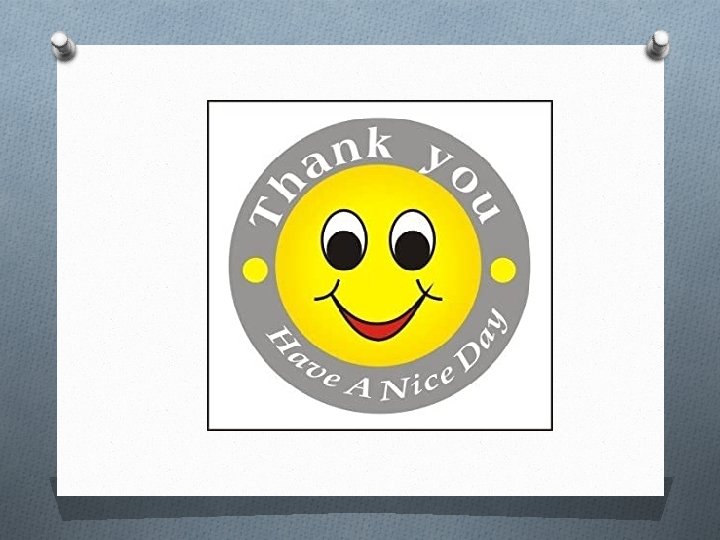
Diagram proses produksi
Torrent qq
Design of experiments doe
Jmp design of experiments
Full factorial design
Design of experiments quality management pmp
Design of experiments presentation
Worked out
Design of experiments pmp
Plackett burman method
Six sigma betekenis
Design of experiments
General directorate of animal health and production