ComputerAssisted Corpus Annotation Xiaofei Lu APLNG 596 D
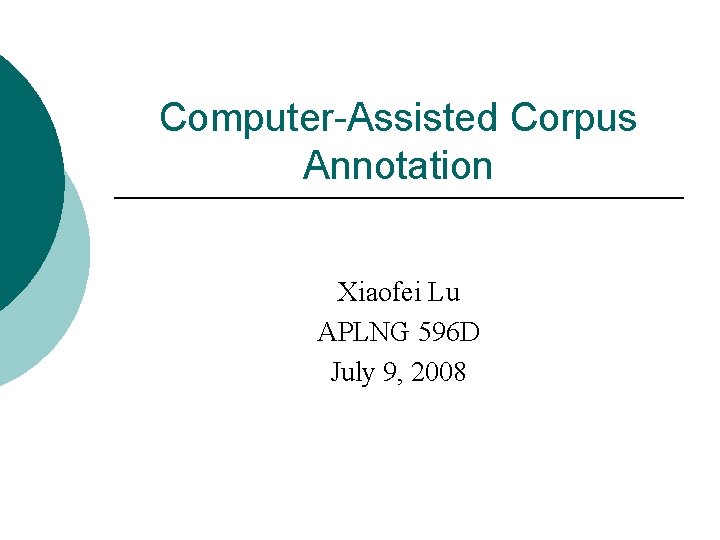
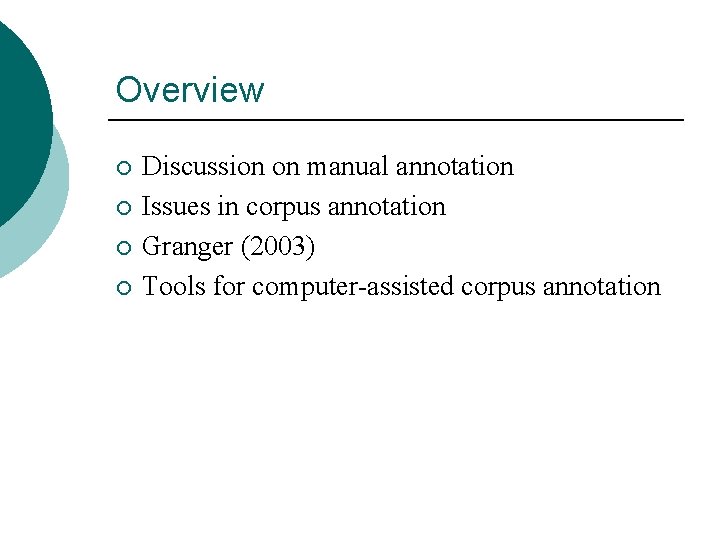
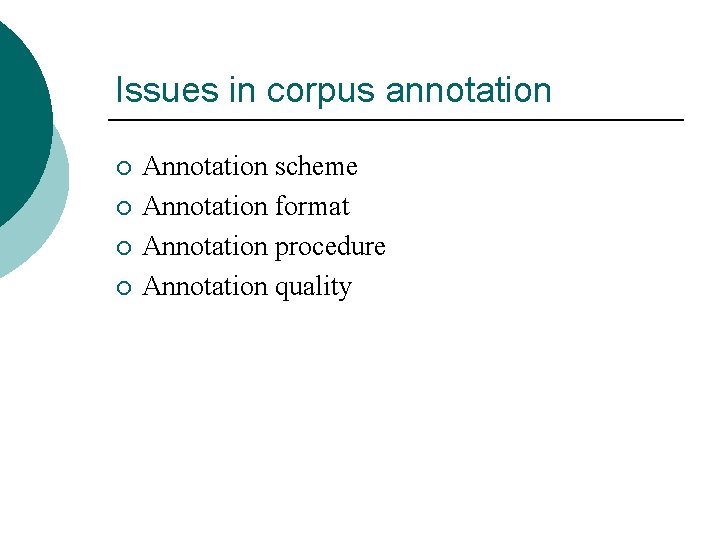
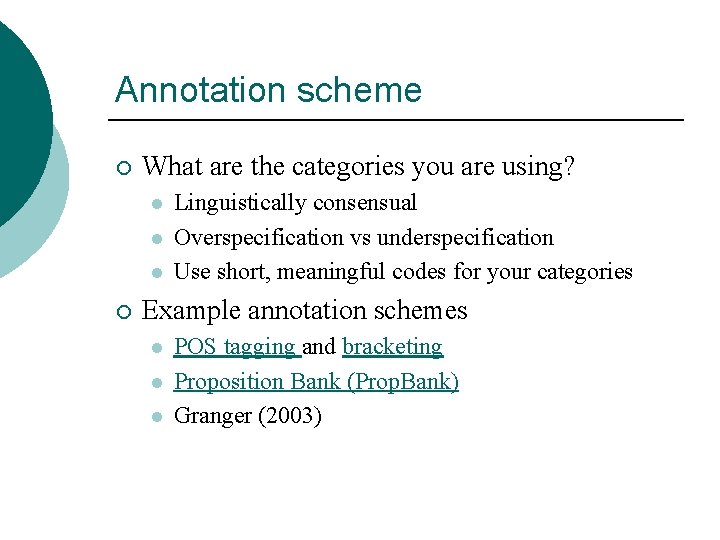
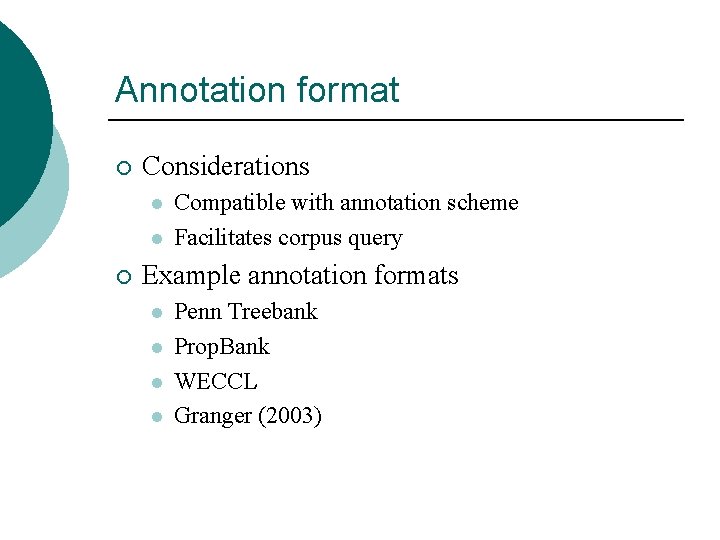
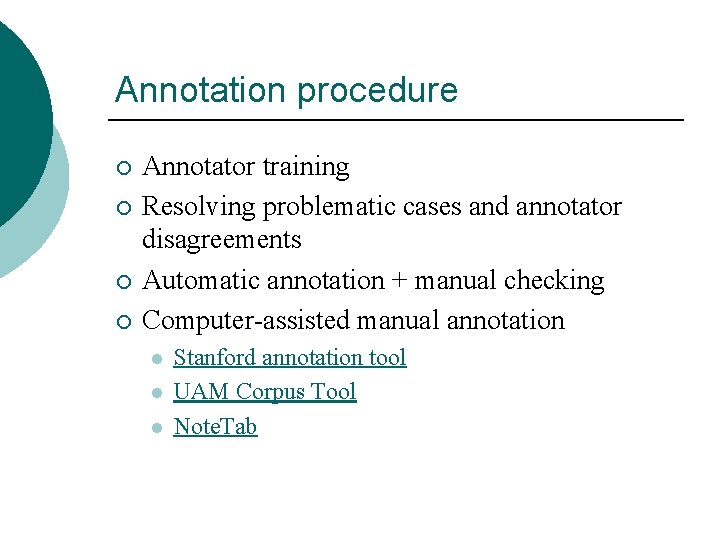
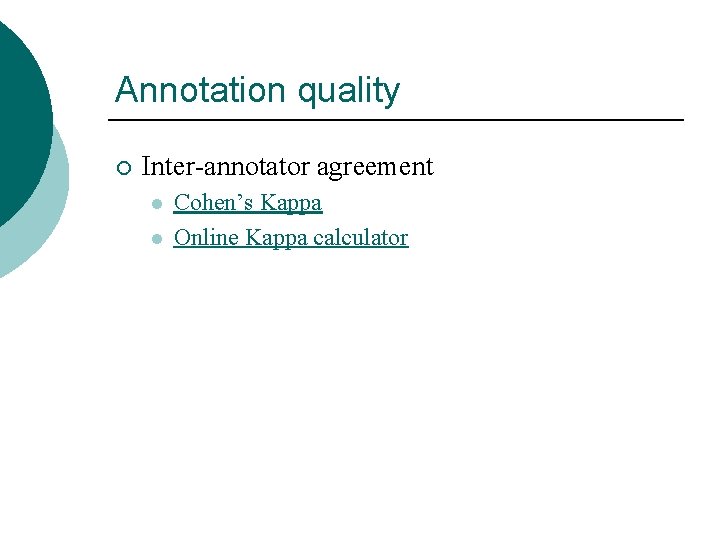
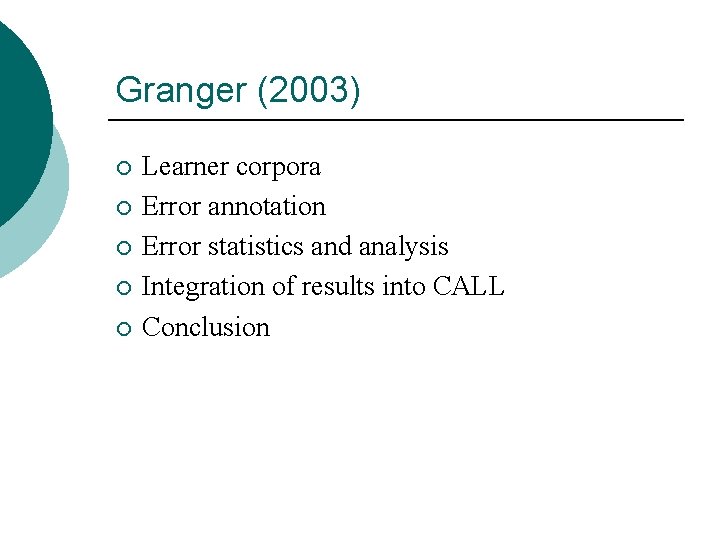
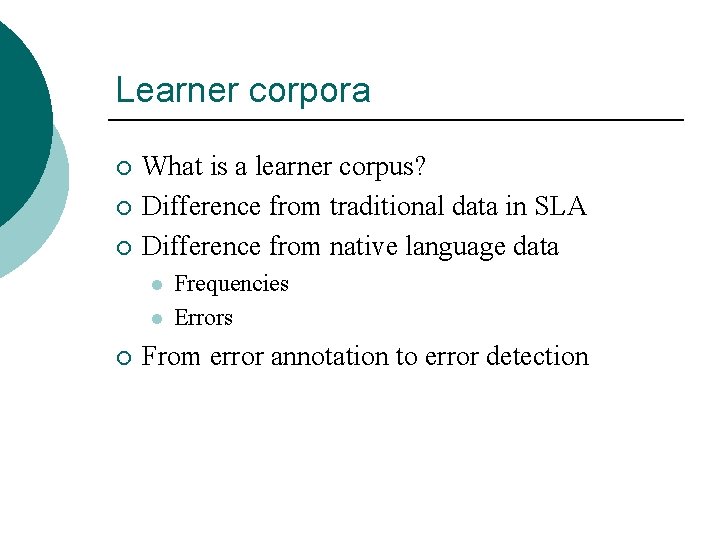
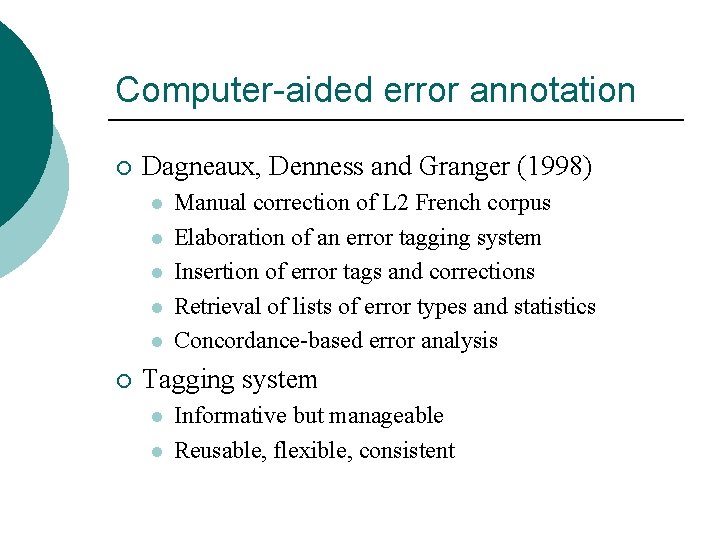
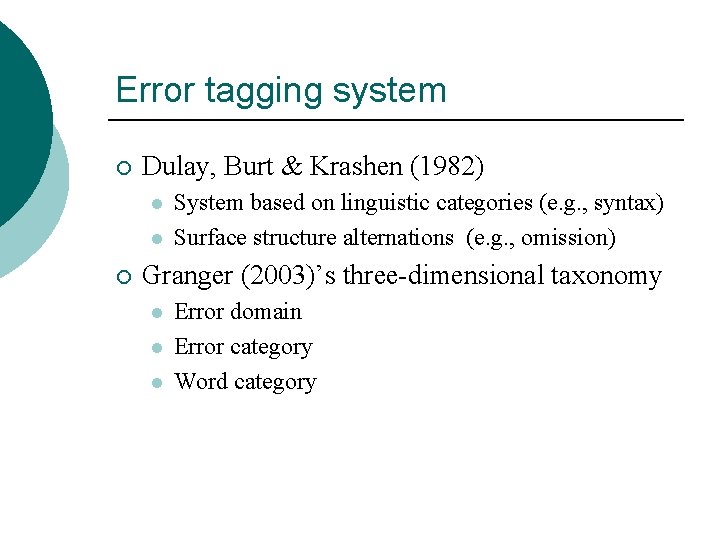
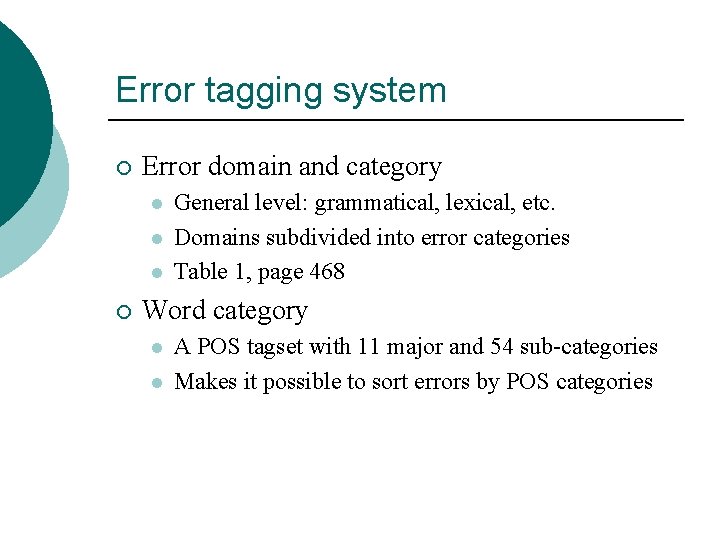
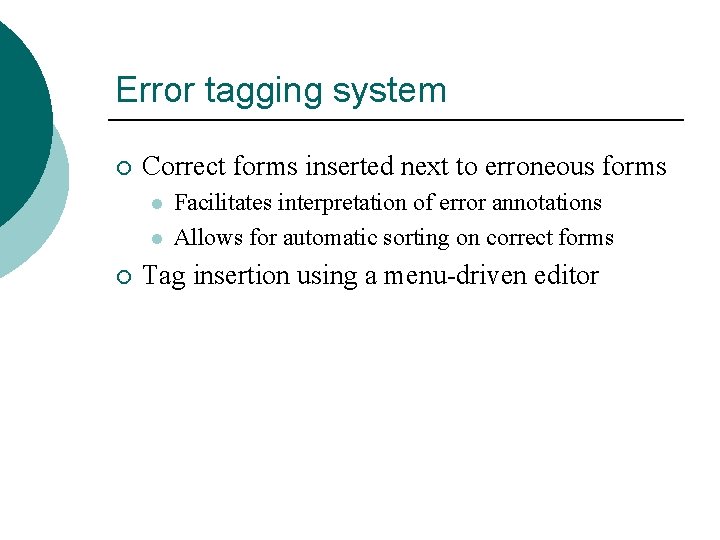
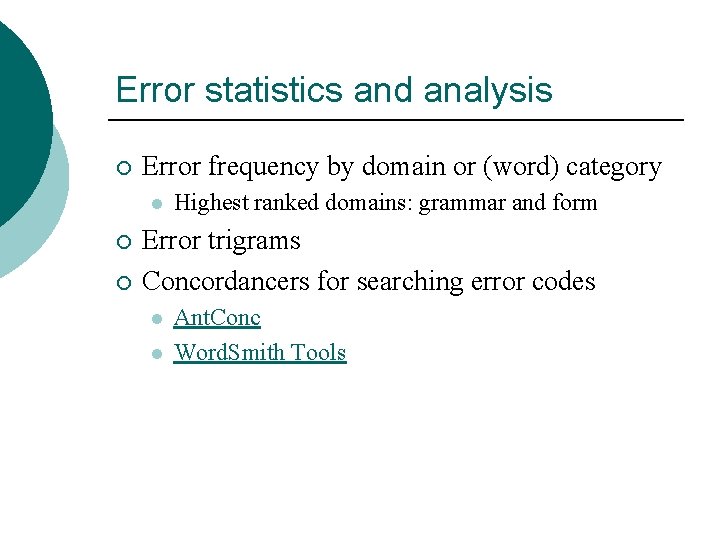
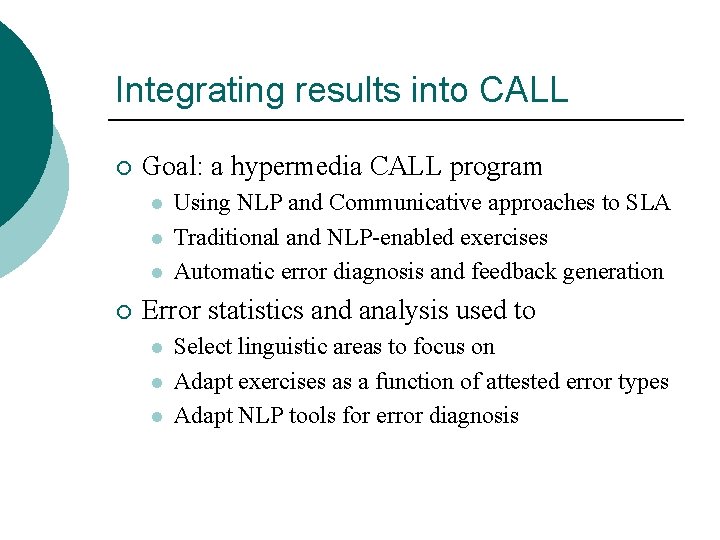
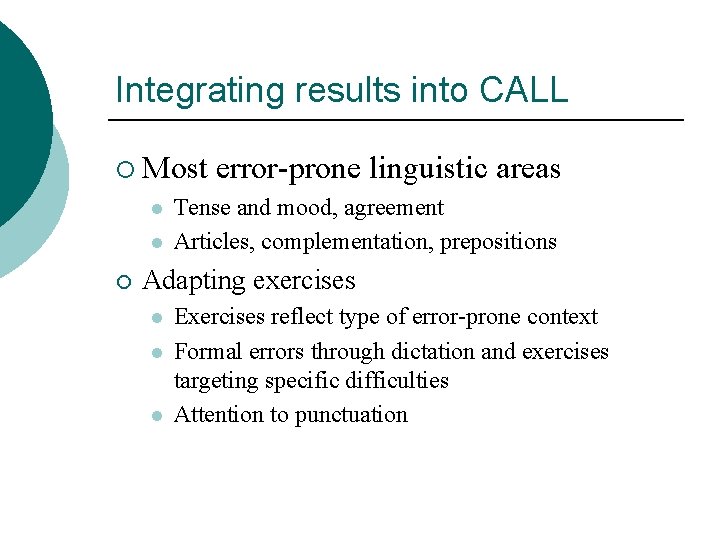
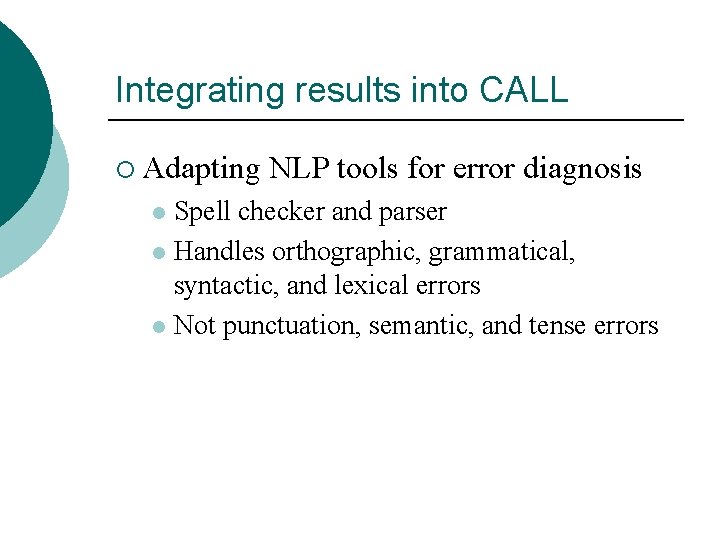
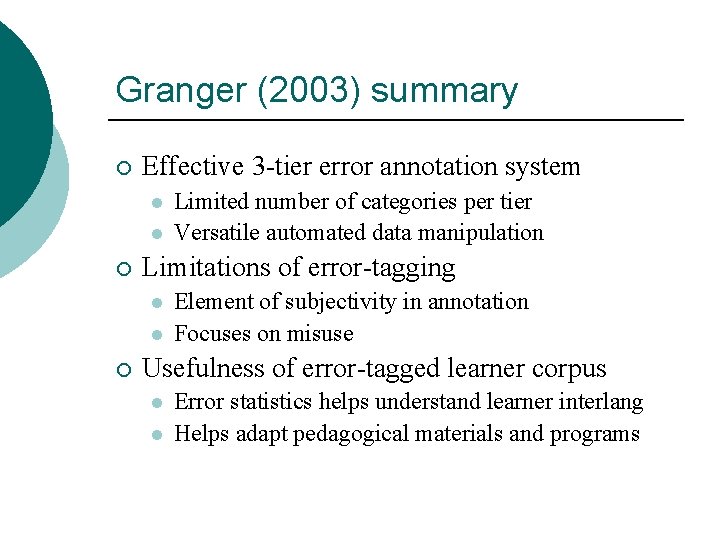
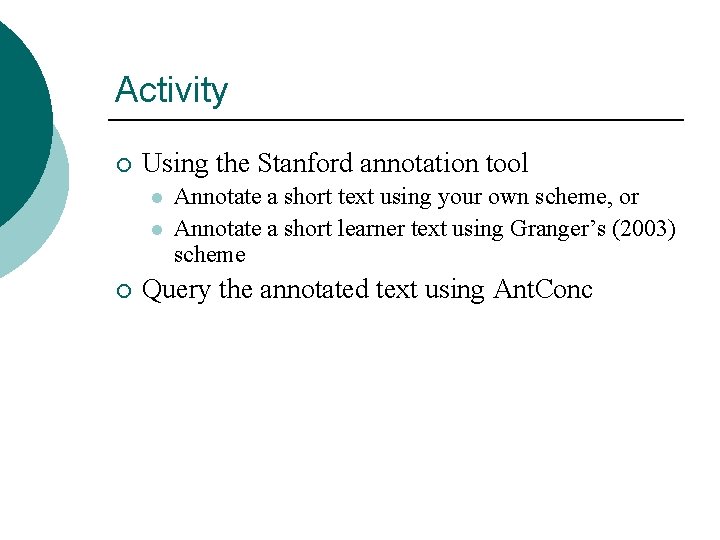
- Slides: 19
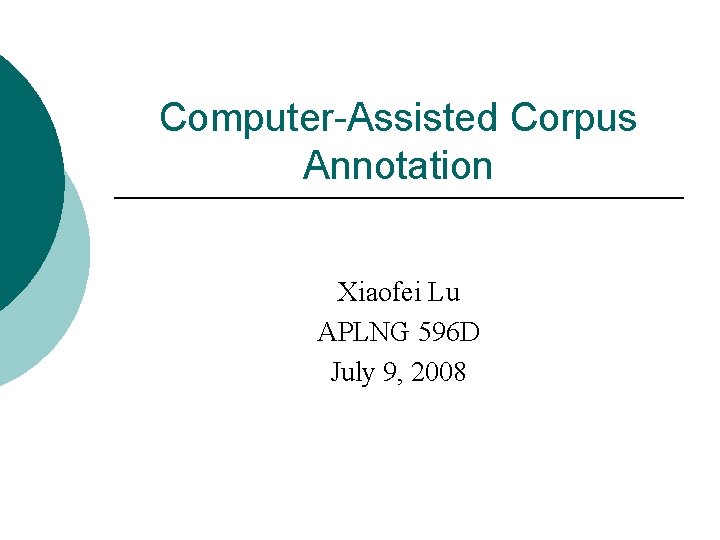
Computer-Assisted Corpus Annotation Xiaofei Lu APLNG 596 D July 9, 2008
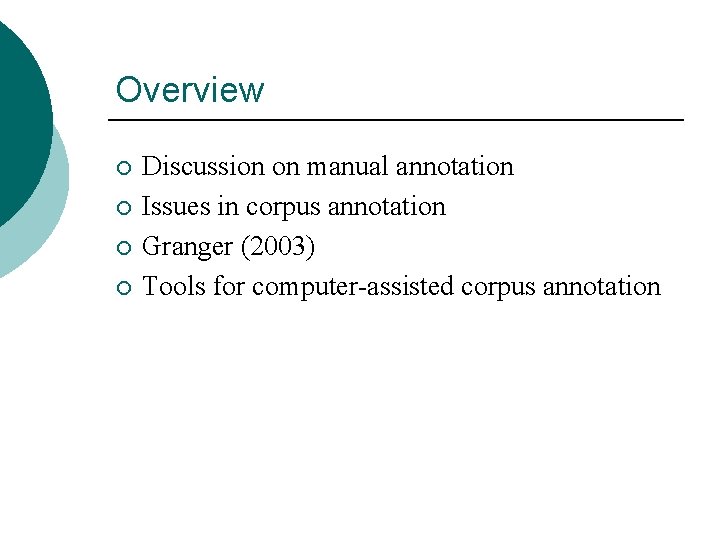
Overview ¡ ¡ Discussion on manual annotation Issues in corpus annotation Granger (2003) Tools for computer-assisted corpus annotation
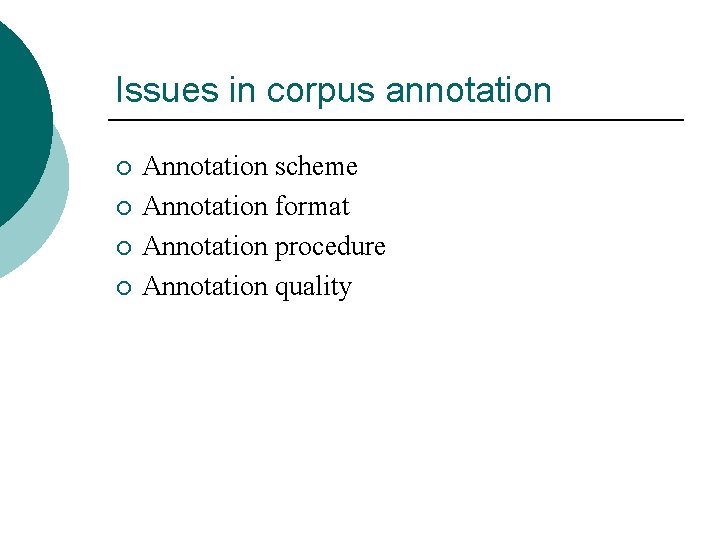
Issues in corpus annotation ¡ ¡ Annotation scheme Annotation format Annotation procedure Annotation quality
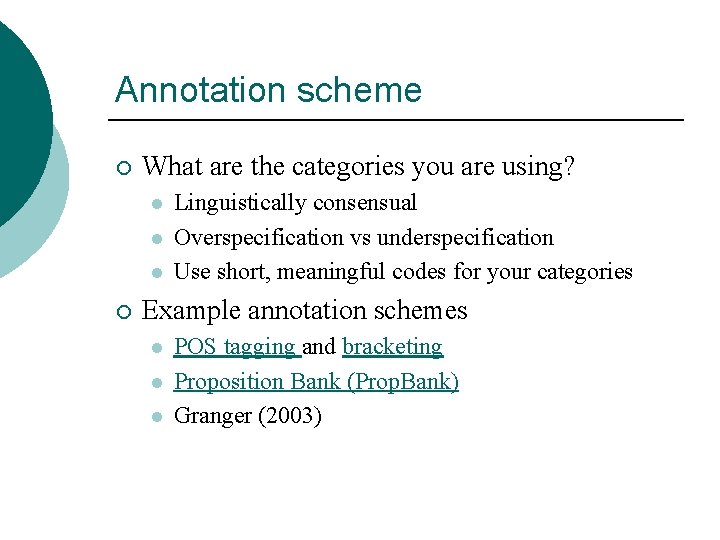
Annotation scheme ¡ What are the categories you are using? l l l ¡ Linguistically consensual Overspecification vs underspecification Use short, meaningful codes for your categories Example annotation schemes l l l POS tagging and bracketing Proposition Bank (Prop. Bank) Granger (2003)
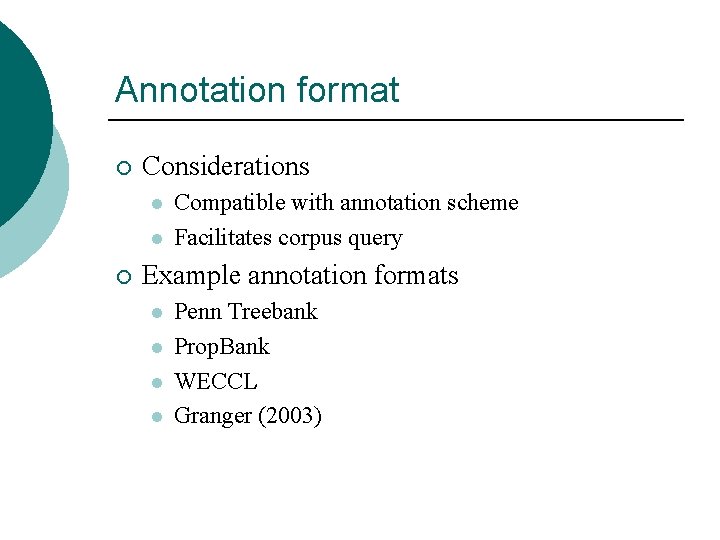
Annotation format ¡ Considerations l l ¡ Compatible with annotation scheme Facilitates corpus query Example annotation formats l l Penn Treebank Prop. Bank WECCL Granger (2003)
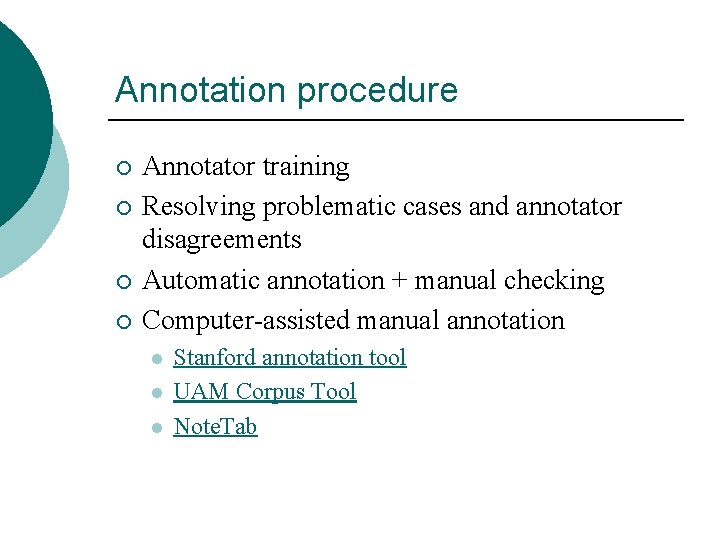
Annotation procedure ¡ ¡ Annotator training Resolving problematic cases and annotator disagreements Automatic annotation + manual checking Computer-assisted manual annotation l l l Stanford annotation tool UAM Corpus Tool Note. Tab
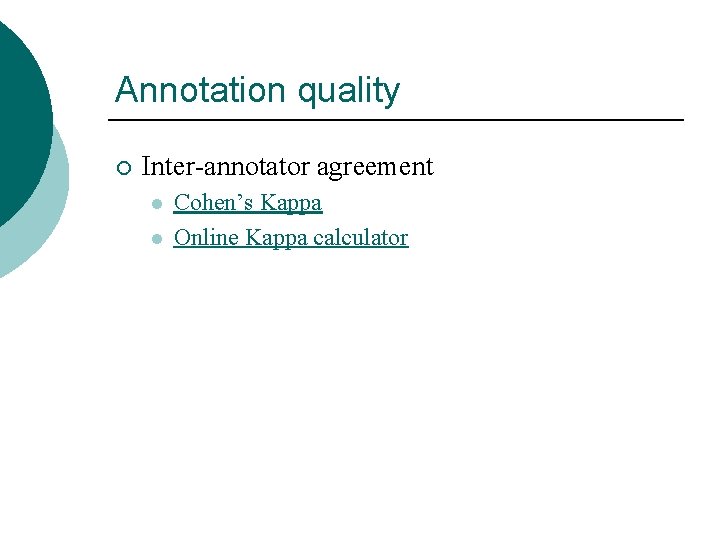
Annotation quality ¡ Inter-annotator agreement l l Cohen’s Kappa Online Kappa calculator
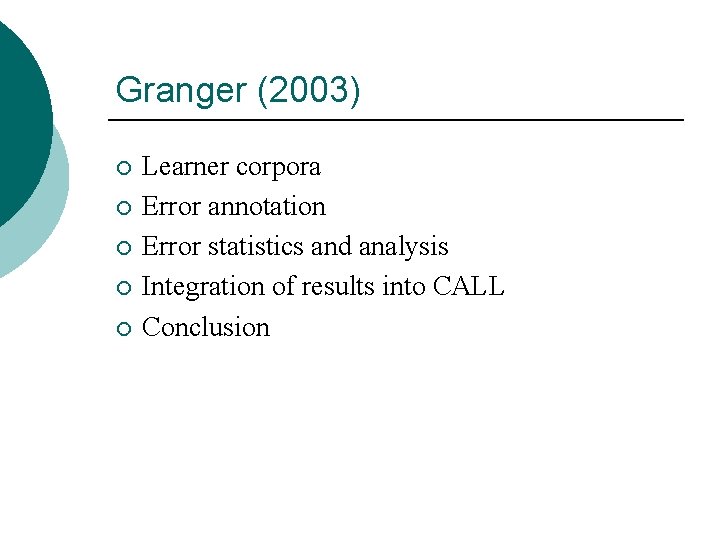
Granger (2003) ¡ ¡ ¡ Learner corpora Error annotation Error statistics and analysis Integration of results into CALL Conclusion
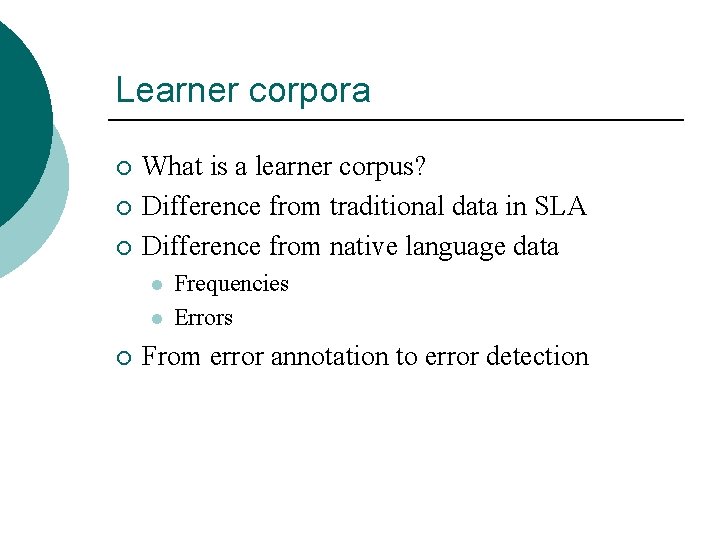
Learner corpora ¡ ¡ ¡ What is a learner corpus? Difference from traditional data in SLA Difference from native language data l l ¡ Frequencies Errors From error annotation to error detection
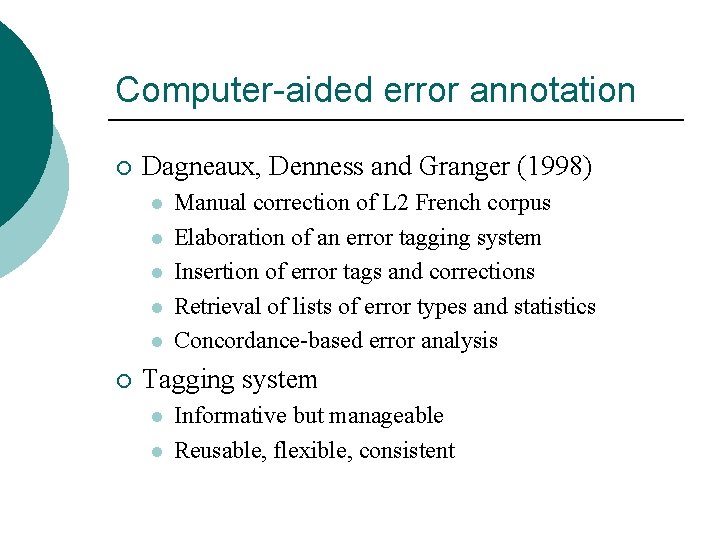
Computer-aided error annotation ¡ Dagneaux, Denness and Granger (1998) l l l ¡ Manual correction of L 2 French corpus Elaboration of an error tagging system Insertion of error tags and corrections Retrieval of lists of error types and statistics Concordance-based error analysis Tagging system l l Informative but manageable Reusable, flexible, consistent
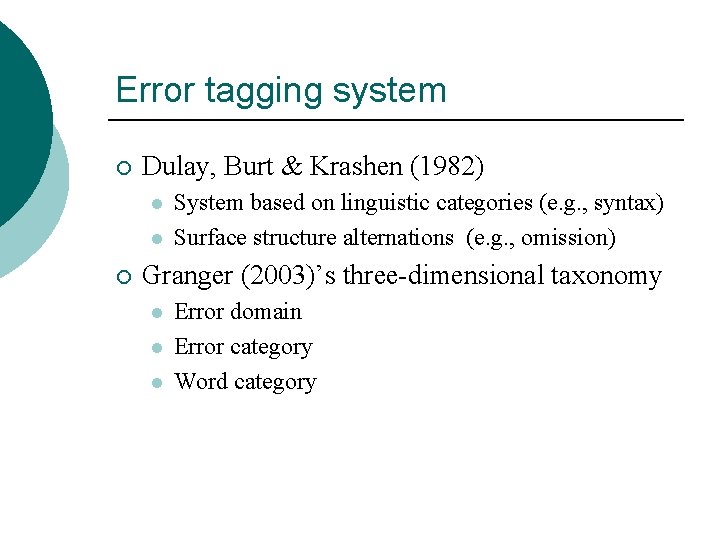
Error tagging system ¡ Dulay, Burt & Krashen (1982) l l ¡ System based on linguistic categories (e. g. , syntax) Surface structure alternations (e. g. , omission) Granger (2003)’s three-dimensional taxonomy l l l Error domain Error category Word category
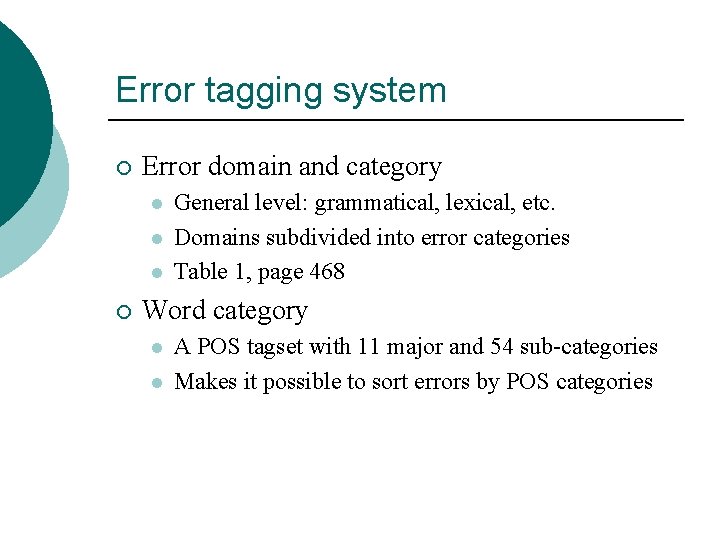
Error tagging system ¡ Error domain and category l l l ¡ General level: grammatical, lexical, etc. Domains subdivided into error categories Table 1, page 468 Word category l l A POS tagset with 11 major and 54 sub-categories Makes it possible to sort errors by POS categories
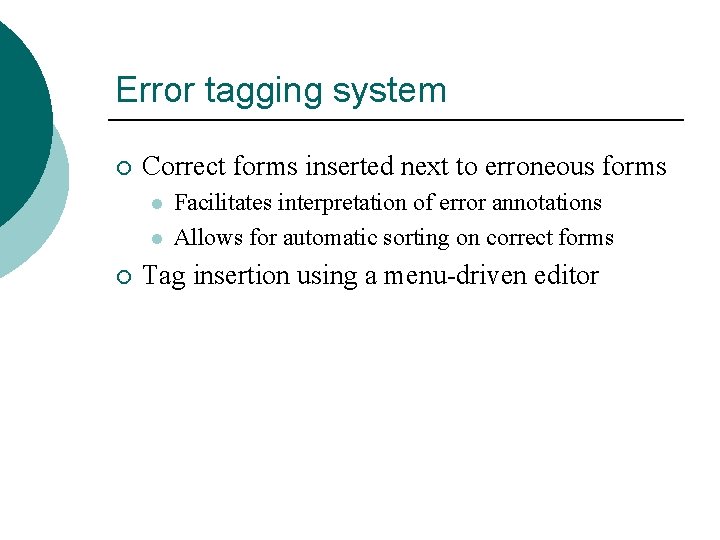
Error tagging system ¡ Correct forms inserted next to erroneous forms l l ¡ Facilitates interpretation of error annotations Allows for automatic sorting on correct forms Tag insertion using a menu-driven editor
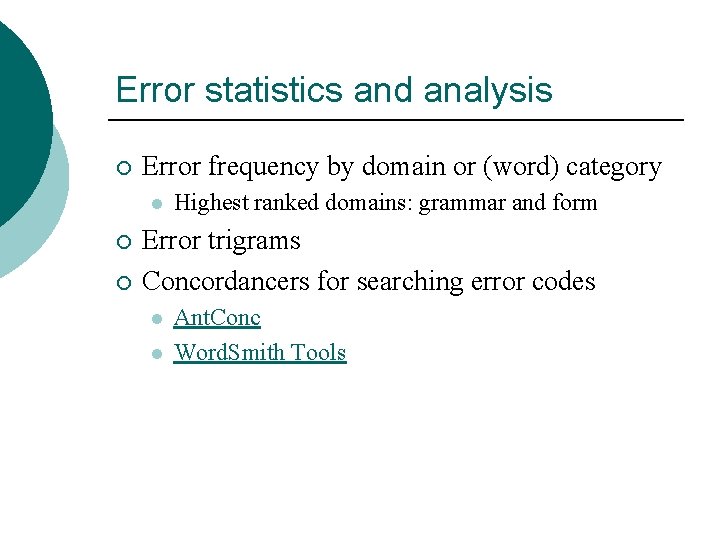
Error statistics and analysis ¡ Error frequency by domain or (word) category l ¡ ¡ Highest ranked domains: grammar and form Error trigrams Concordancers for searching error codes l l Ant. Conc Word. Smith Tools
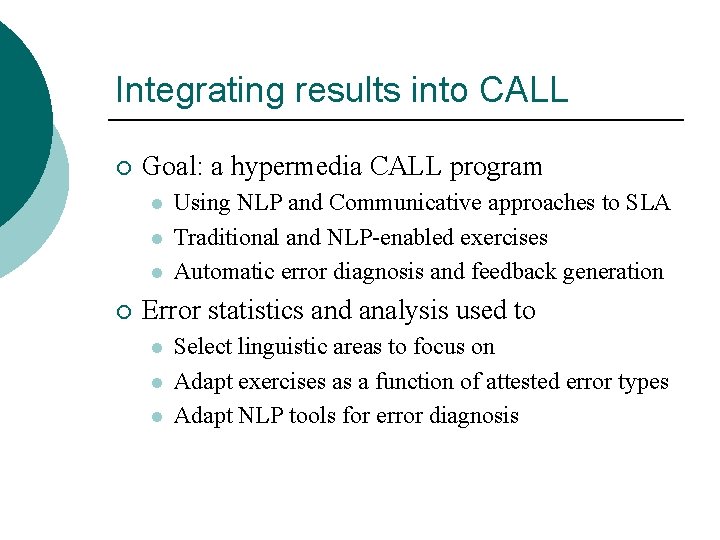
Integrating results into CALL ¡ Goal: a hypermedia CALL program l l l ¡ Using NLP and Communicative approaches to SLA Traditional and NLP-enabled exercises Automatic error diagnosis and feedback generation Error statistics and analysis used to l l l Select linguistic areas to focus on Adapt exercises as a function of attested error types Adapt NLP tools for error diagnosis
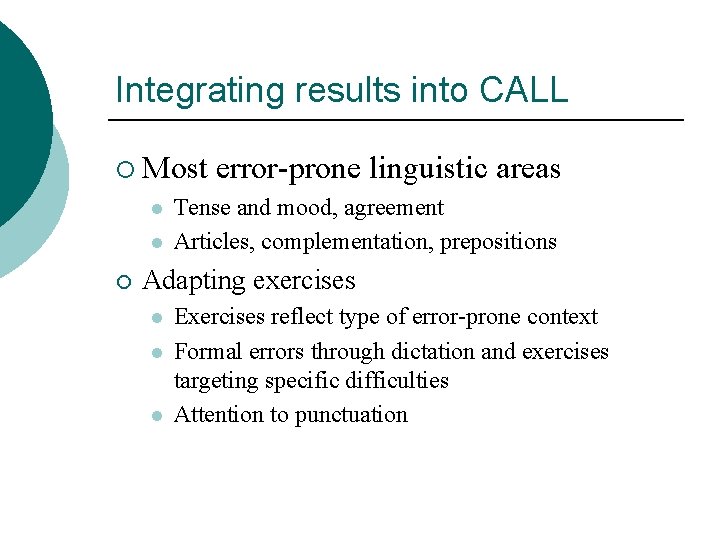
Integrating results into CALL ¡ Most l l ¡ error-prone linguistic areas Tense and mood, agreement Articles, complementation, prepositions Adapting exercises l l l Exercises reflect type of error-prone context Formal errors through dictation and exercises targeting specific difficulties Attention to punctuation
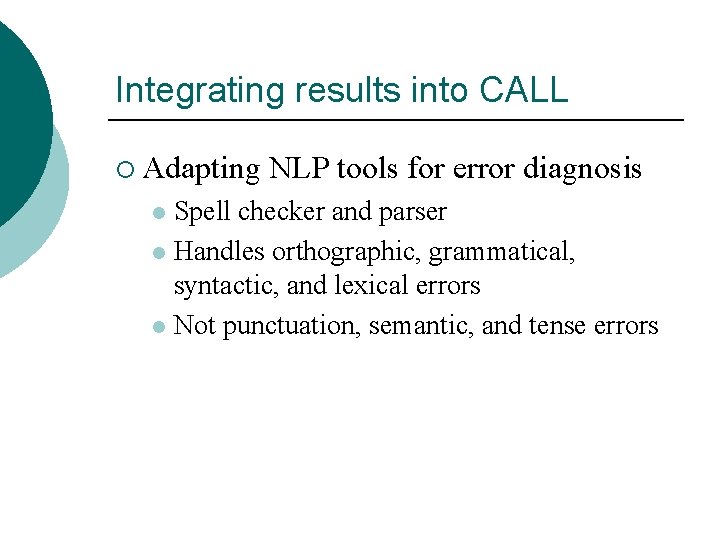
Integrating results into CALL ¡ Adapting NLP tools for error diagnosis Spell checker and parser l Handles orthographic, grammatical, syntactic, and lexical errors l Not punctuation, semantic, and tense errors l
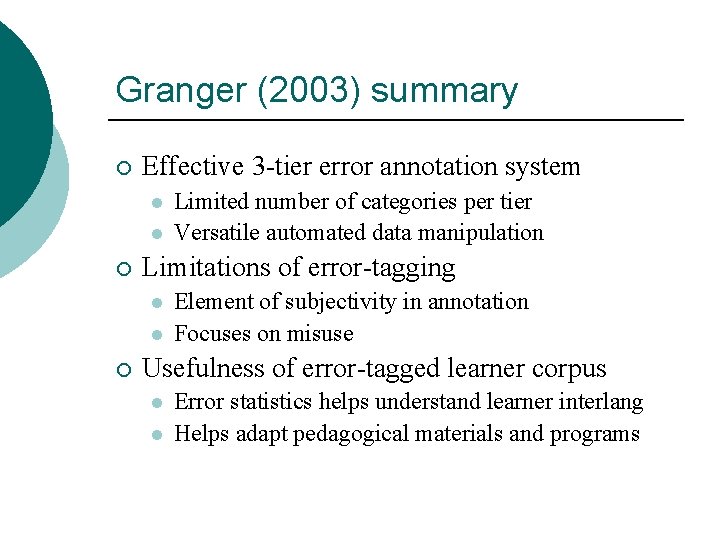
Granger (2003) summary ¡ Effective 3 -tier error annotation system l l ¡ Limitations of error-tagging l l ¡ Limited number of categories per tier Versatile automated data manipulation Element of subjectivity in annotation Focuses on misuse Usefulness of error-tagged learner corpus l l Error statistics helps understand learner interlang Helps adapt pedagogical materials and programs
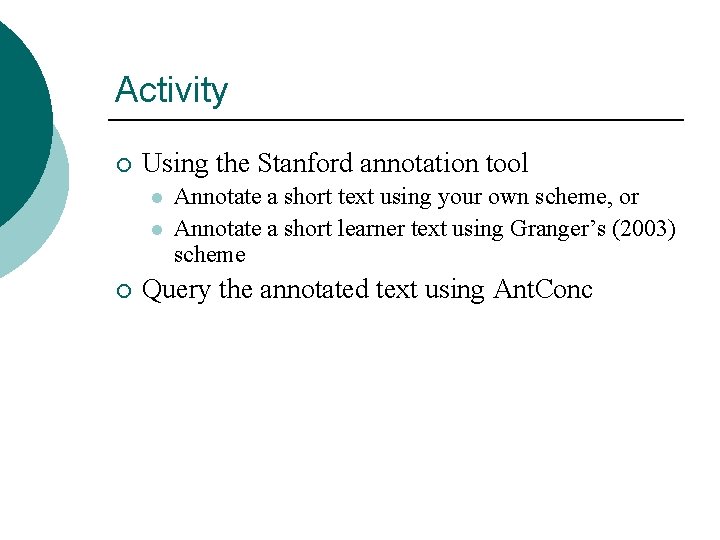
Activity ¡ Using the Stanford annotation tool l l ¡ Annotate a short text using your own scheme, or Annotate a short learner text using Granger’s (2003) scheme Query the annotated text using Ant. Conc
Xiaofei lu
Maxims of annotation in corpus linguistics
Follicle stage
Uterus layers histology
596
Cit 596
9 596 960
Cit 596
Superficie cina
Hw 596
Annotation slows down the reader to deepen understanding
Amazon data annotation
What is annotations
Text symbols for annotating text
How to make a hole in autocad
Rainsford comes to the island because he
Amazon data annotation
Yesterday by patricia pogson
Bacteriophage annotation
What does o valiant cousin worthy gentleman mean