Commodity OriginDestination Provisional Estimates Edward Fekpe Ph D
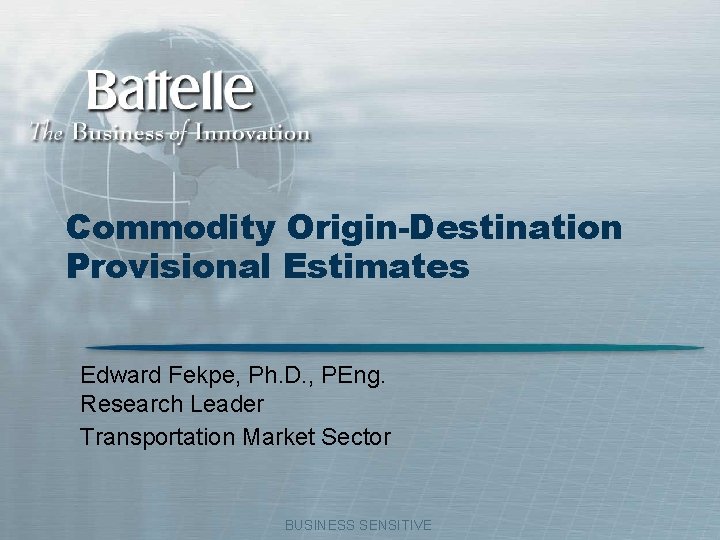
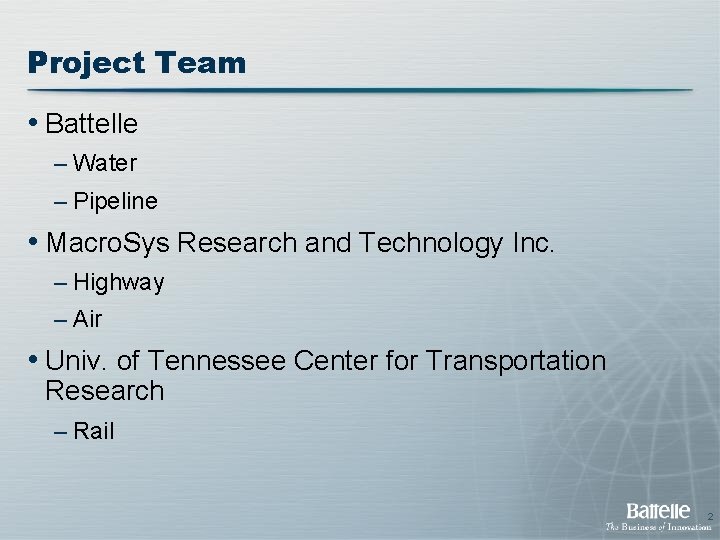
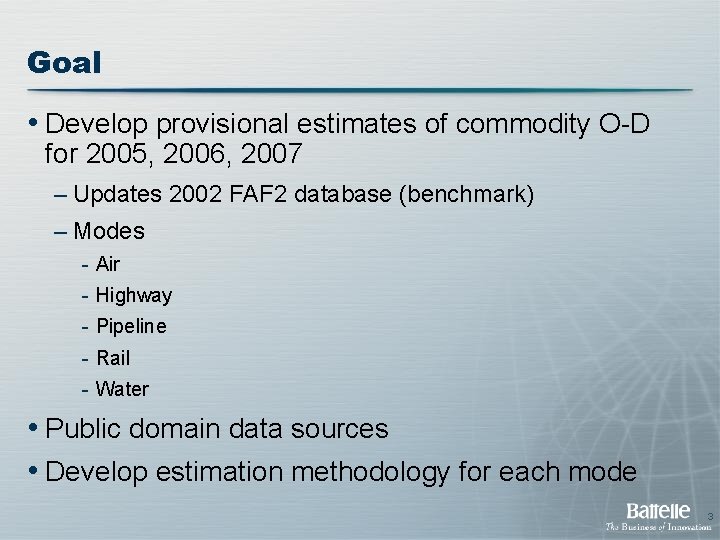
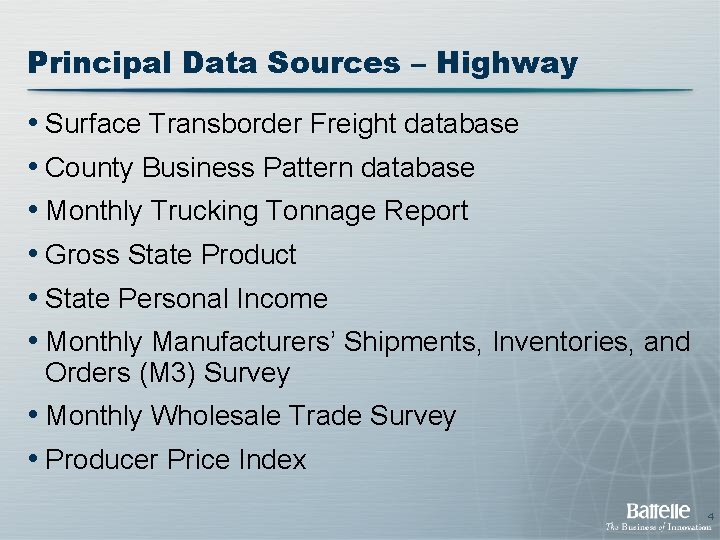
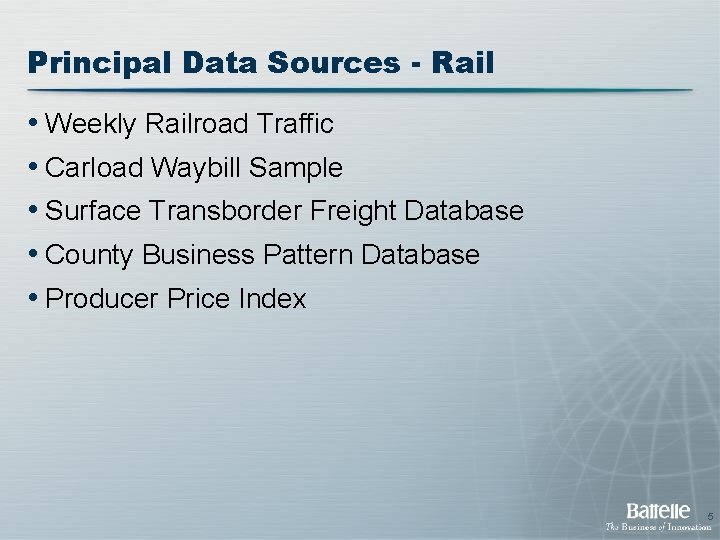
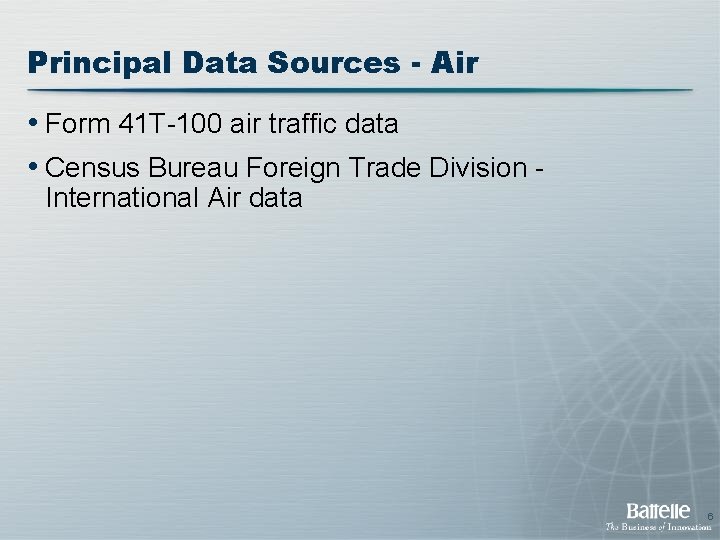
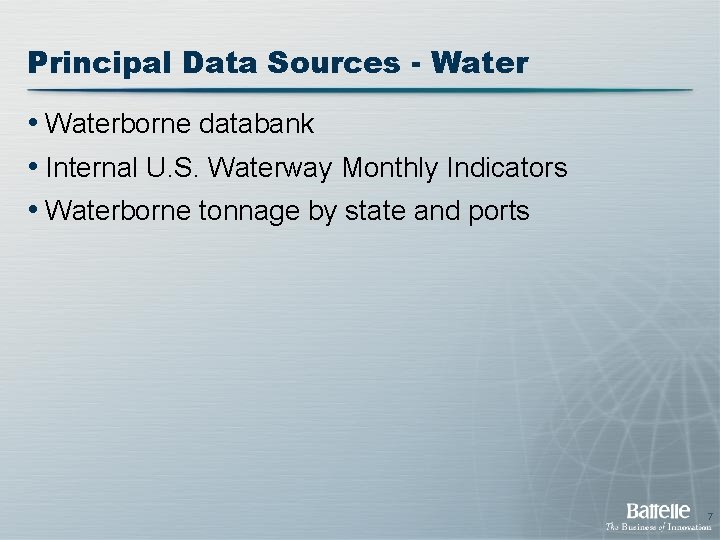
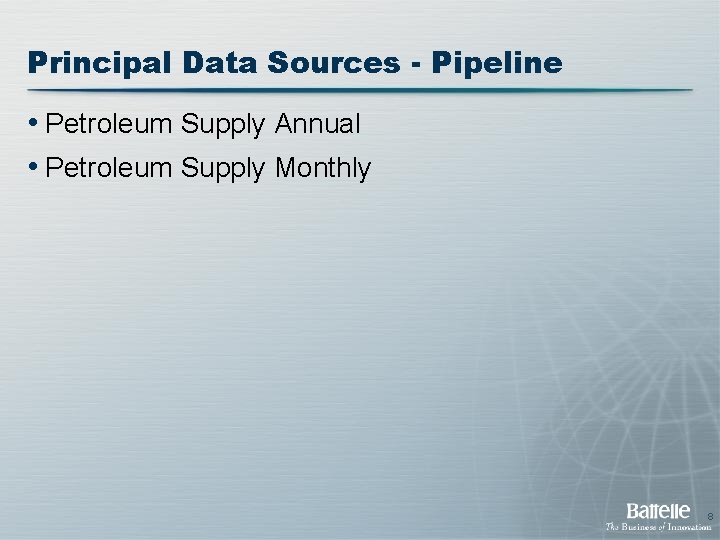
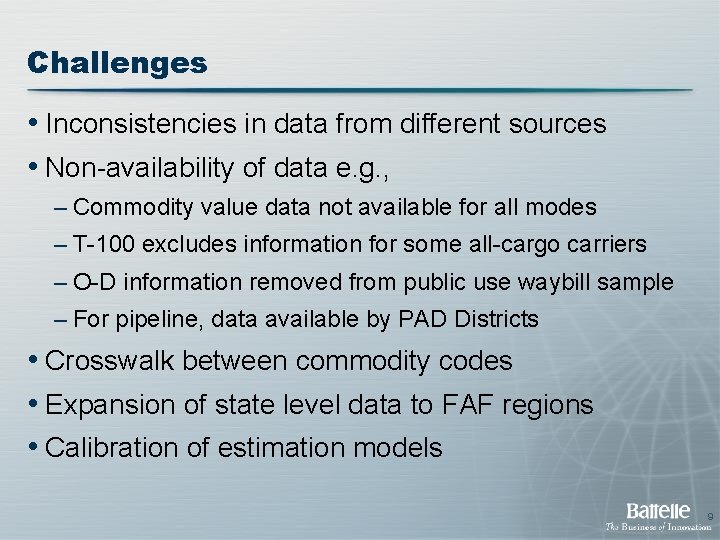
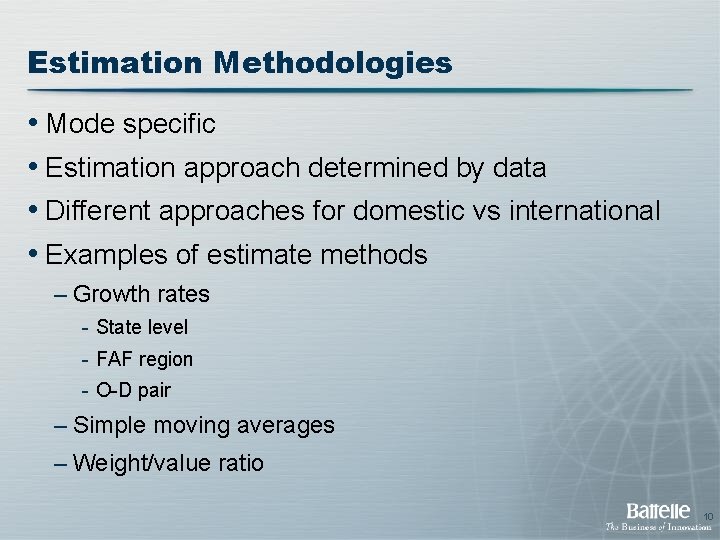
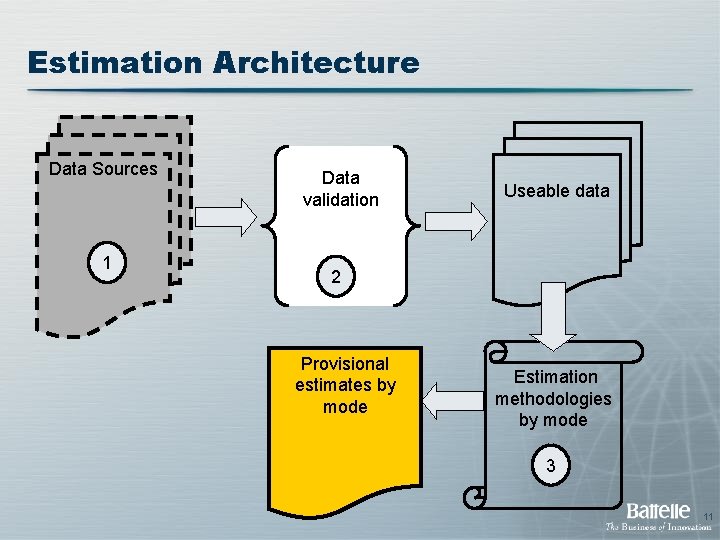
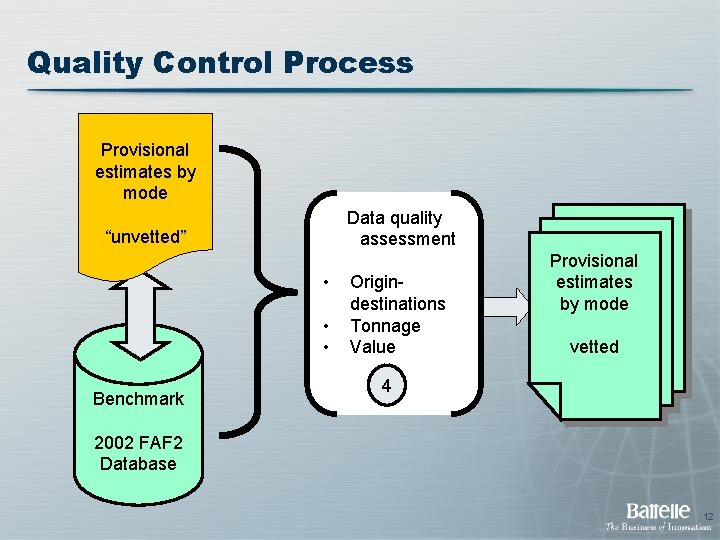
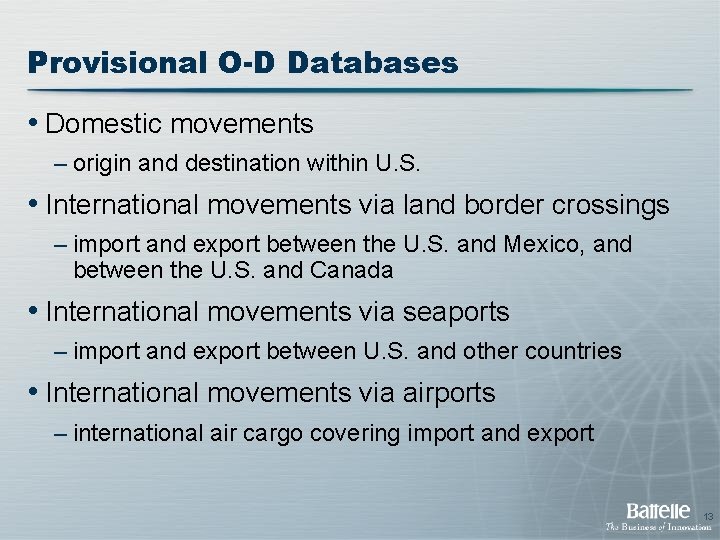
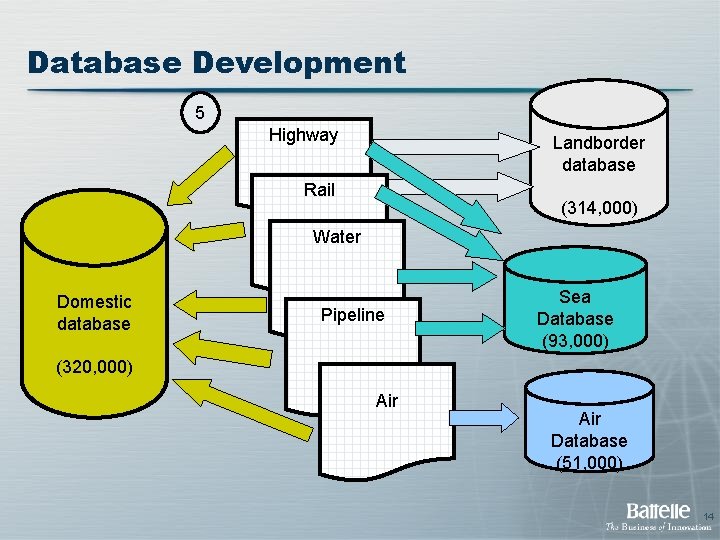
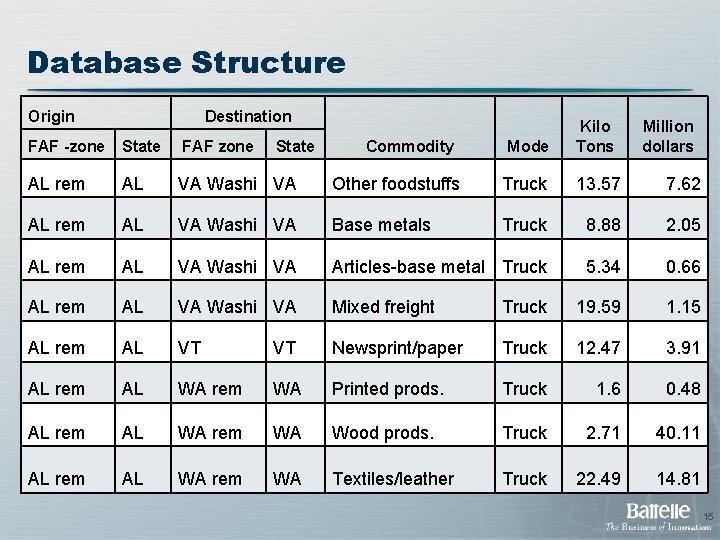
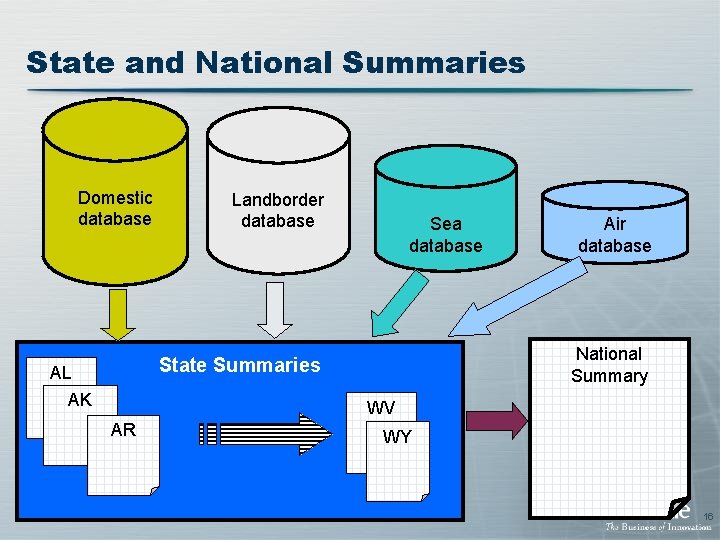
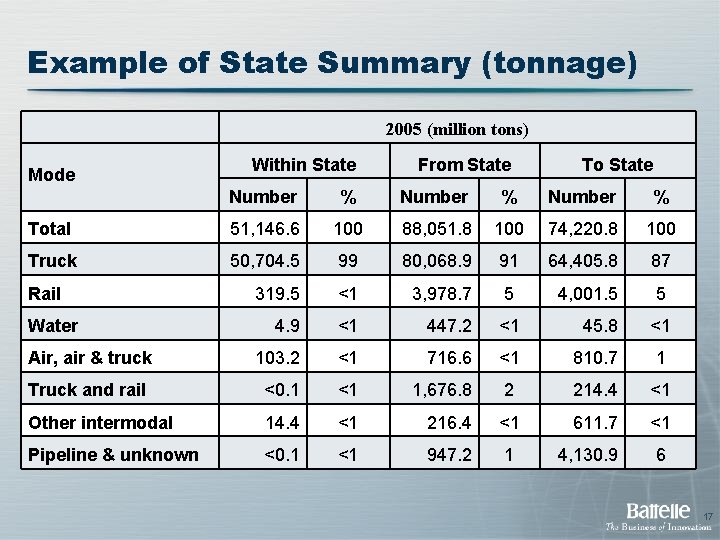
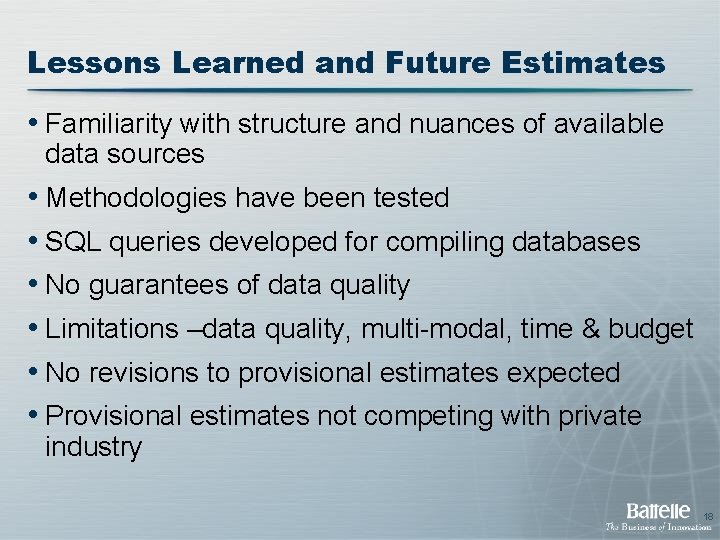
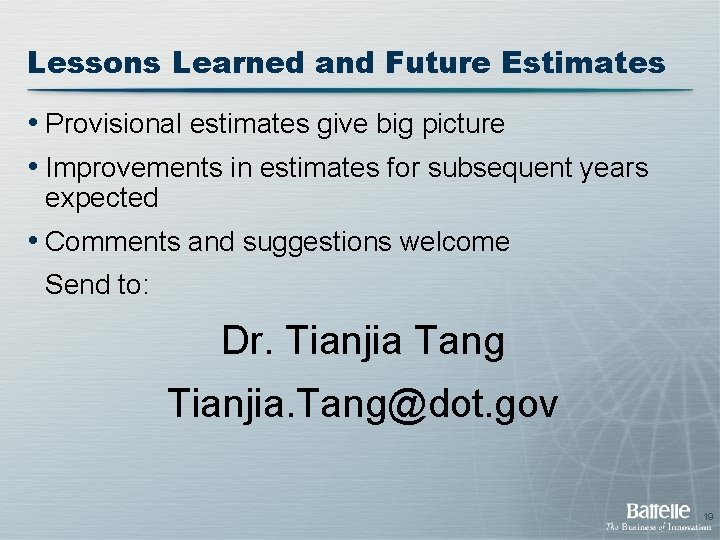
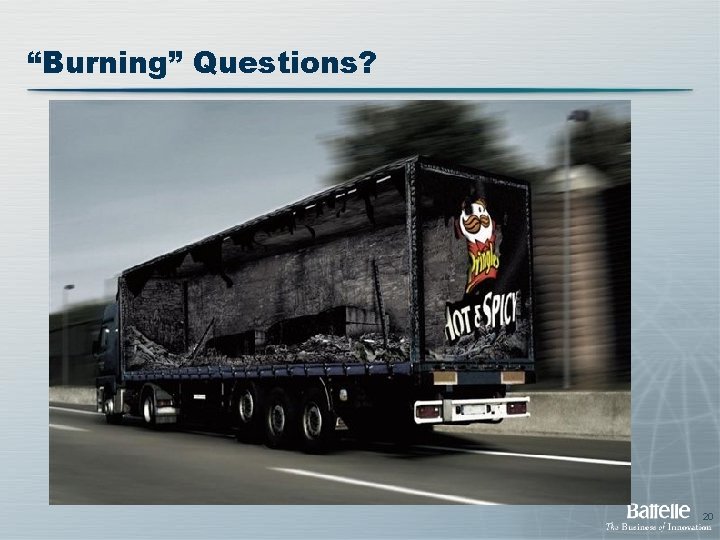
- Slides: 20
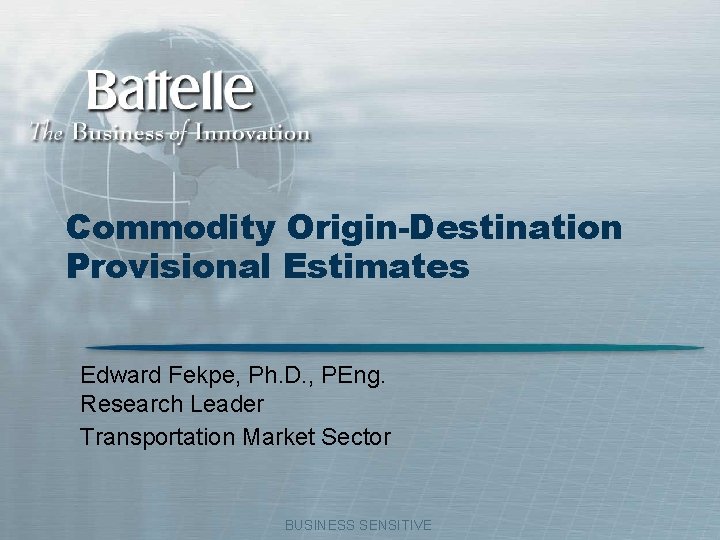
Commodity Origin-Destination Provisional Estimates Edward Fekpe, Ph. D. , PEng. Research Leader Transportation Market Sector BUSINESS SENSITIVE
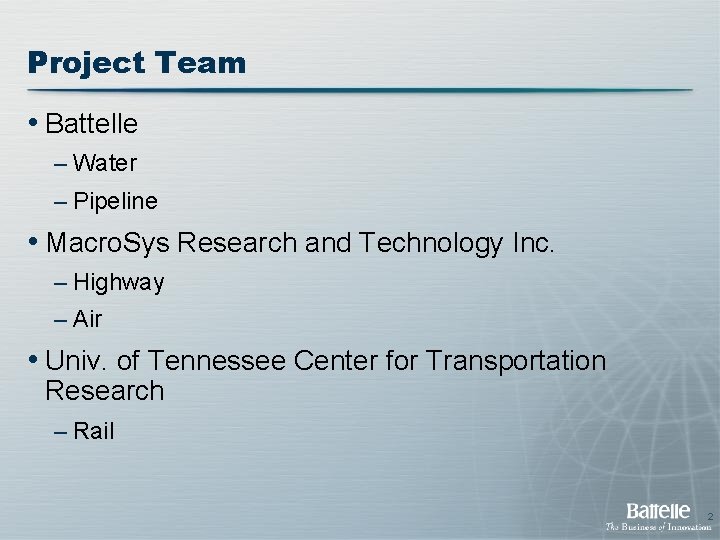
Project Team • Battelle – Water – Pipeline • Macro. Sys Research and Technology Inc. – Highway – Air • Univ. of Tennessee Center for Transportation Research – Rail 2
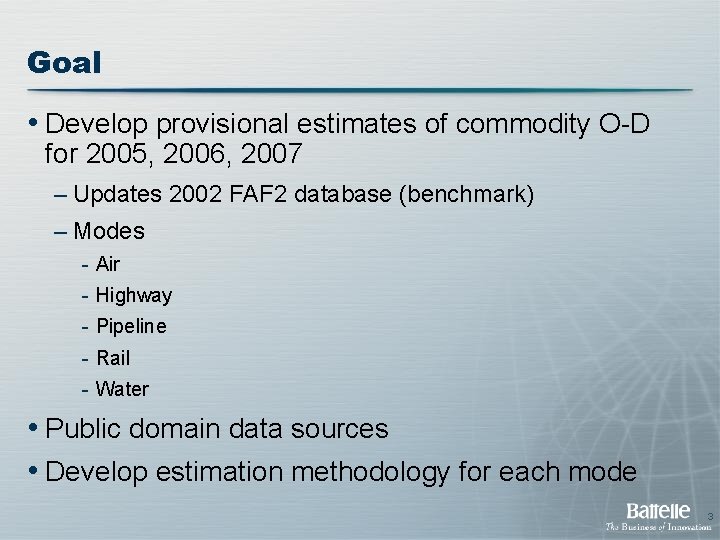
Goal • Develop provisional estimates of commodity O-D for 2005, 2006, 2007 – Updates 2002 FAF 2 database (benchmark) – Modes - Air - Highway - Pipeline - Rail - Water • Public domain data sources • Develop estimation methodology for each mode 3
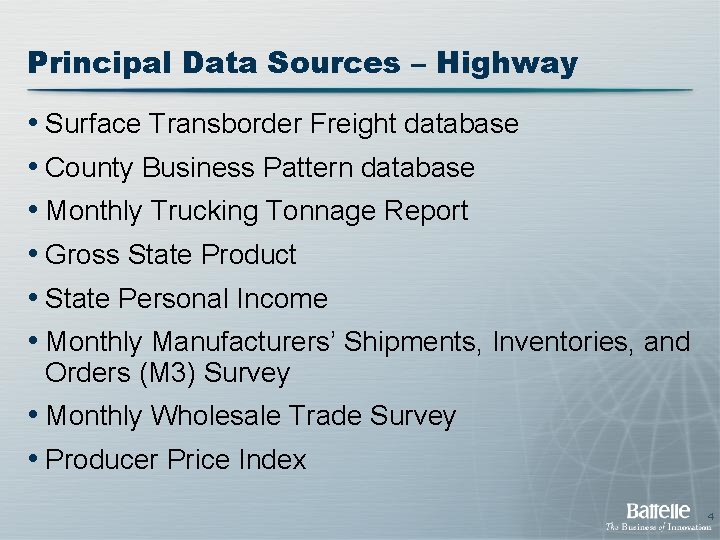
Principal Data Sources – Highway • Surface Transborder Freight database • County Business Pattern database • Monthly Trucking Tonnage Report • Gross State Product • State Personal Income • Monthly Manufacturers’ Shipments, Inventories, and Orders (M 3) Survey • Monthly Wholesale Trade Survey • Producer Price Index 4
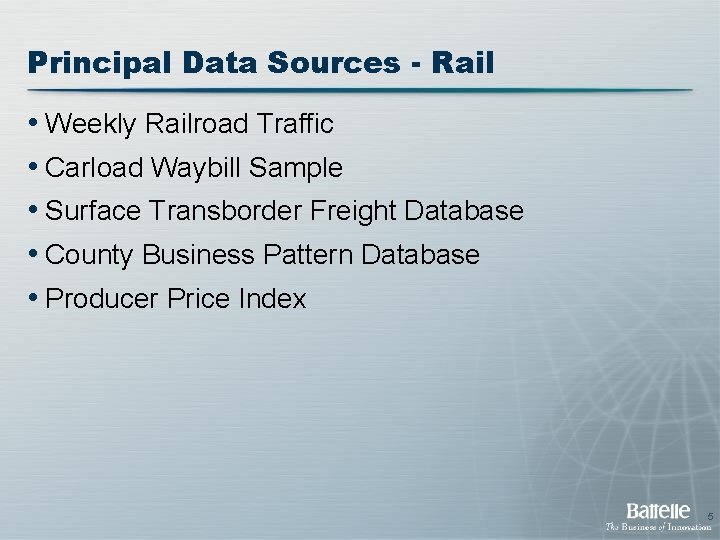
Principal Data Sources - Rail • Weekly Railroad Traffic • Carload Waybill Sample • Surface Transborder Freight Database • County Business Pattern Database • Producer Price Index 5
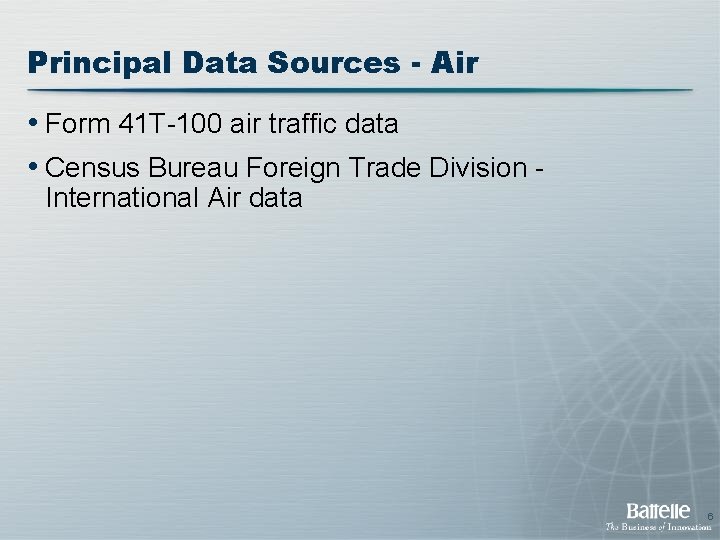
Principal Data Sources - Air • Form 41 T-100 air traffic data • Census Bureau Foreign Trade Division International Air data 6
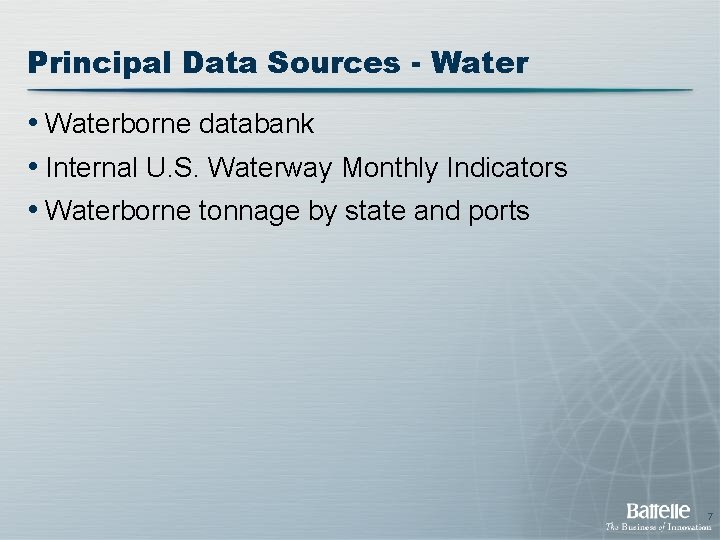
Principal Data Sources - Water • Waterborne databank • Internal U. S. Waterway Monthly Indicators • Waterborne tonnage by state and ports 7
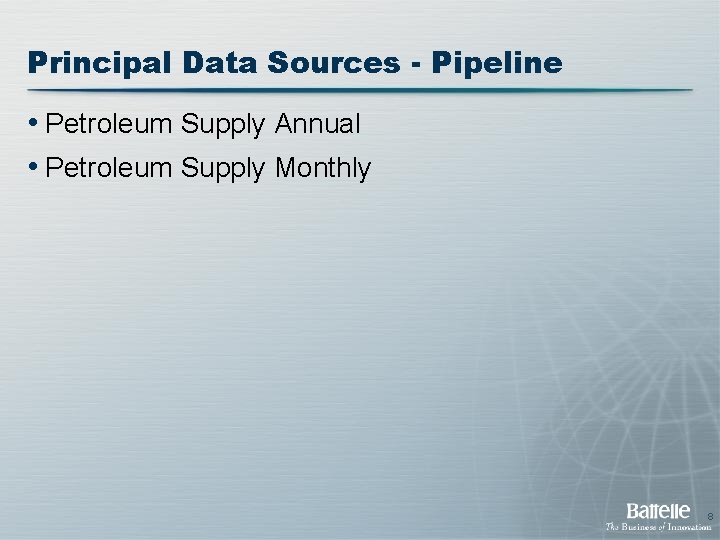
Principal Data Sources - Pipeline • Petroleum Supply Annual • Petroleum Supply Monthly 8
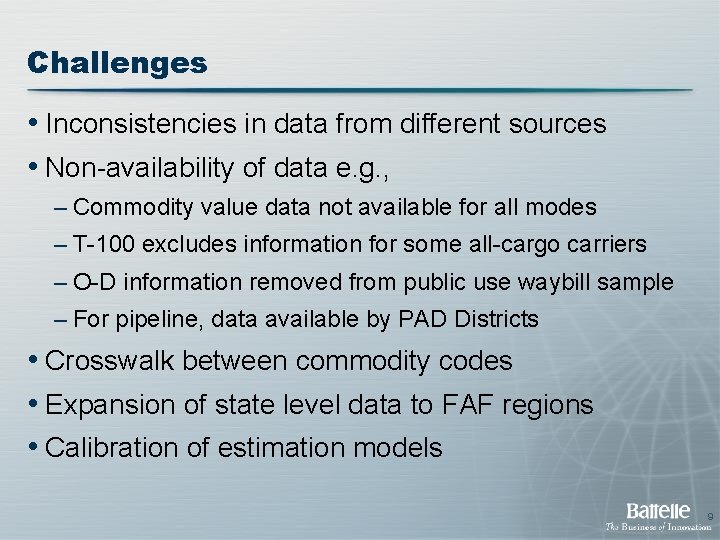
Challenges • Inconsistencies in data from different sources • Non-availability of data e. g. , – Commodity value data not available for all modes – T-100 excludes information for some all-cargo carriers – O-D information removed from public use waybill sample – For pipeline, data available by PAD Districts • Crosswalk between commodity codes • Expansion of state level data to FAF regions • Calibration of estimation models 9
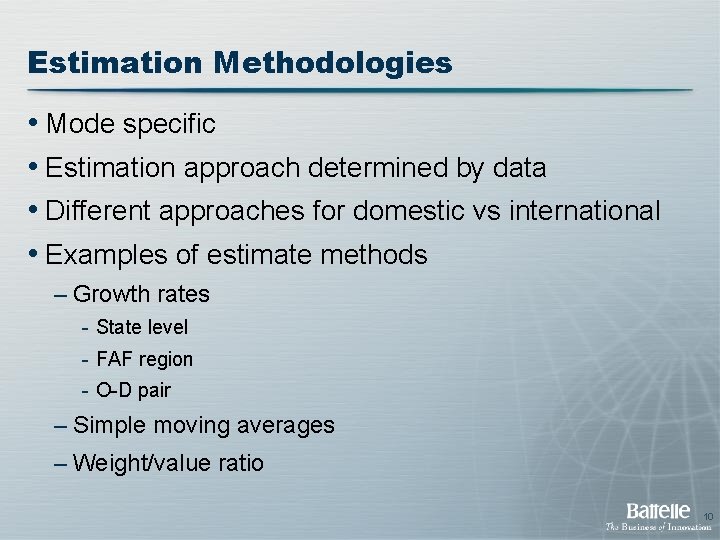
Estimation Methodologies • Mode specific • Estimation approach determined by data • Different approaches for domestic vs international • Examples of estimate methods – Growth rates - State level - FAF region - O-D pair – Simple moving averages – Weight/value ratio 10
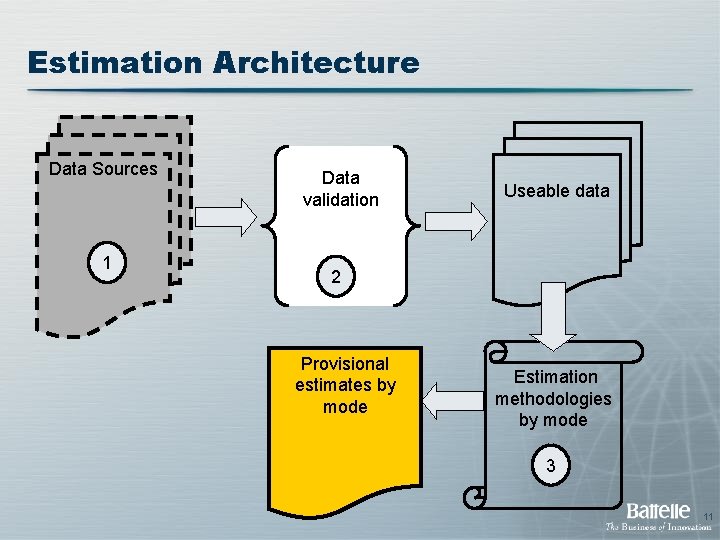
Estimation Architecture Data Sources 1 Data validation Useable data 2 Provisional estimates by mode Estimation methodologies by mode 3 11
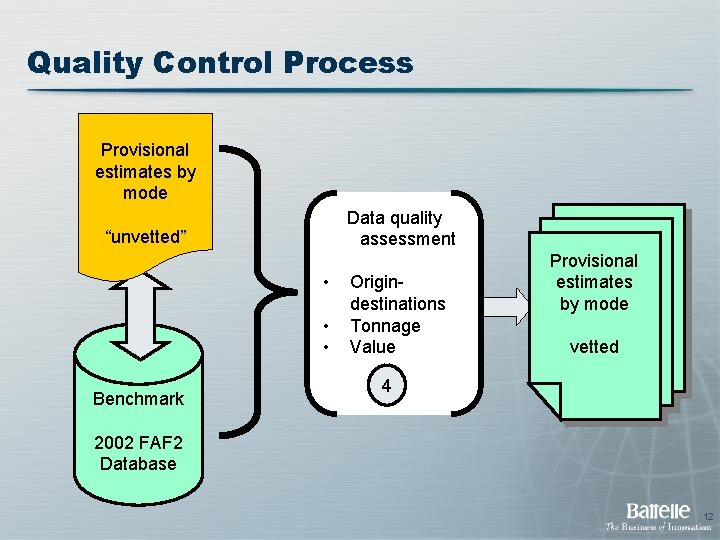
Quality Control Process Provisional estimates by mode Data quality assessment “unvetted” • • • Benchmark Origindestinations Tonnage Value Provisional estimates by mode vetted 4 2002 FAF 2 Database 12
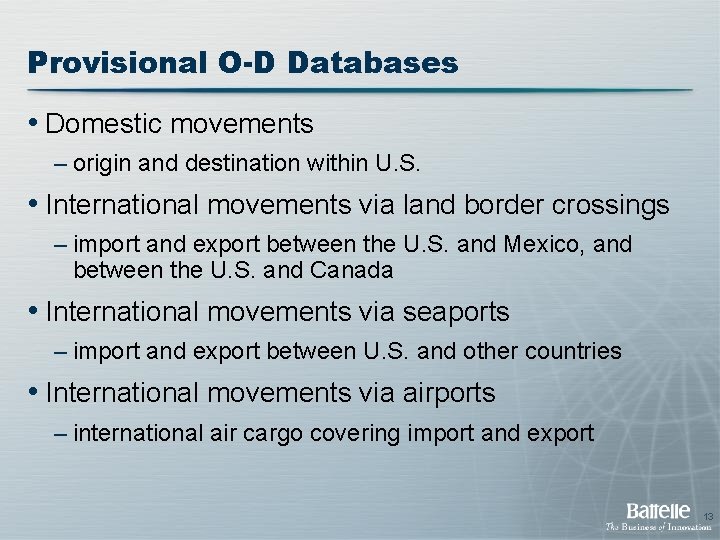
Provisional O-D Databases • Domestic movements – origin and destination within U. S. • International movements via land border crossings – import and export between the U. S. and Mexico, and between the U. S. and Canada • International movements via seaports – import and export between U. S. and other countries • International movements via airports – international air cargo covering import and export 13
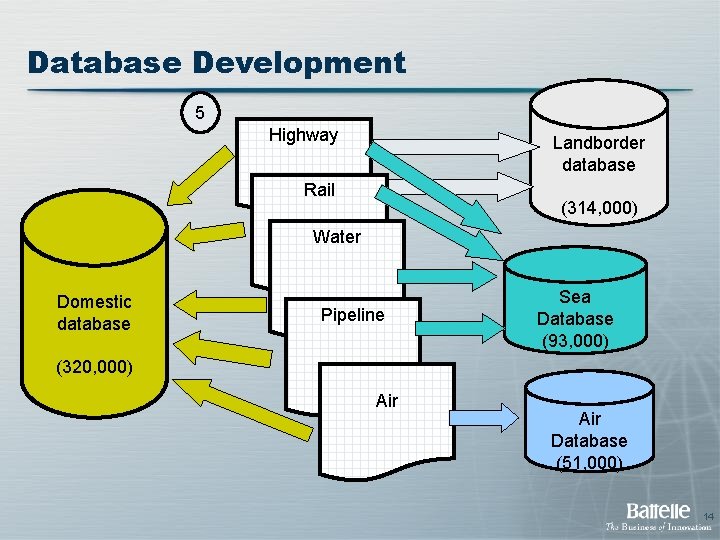
Database Development 5 Highway Landborder database Rail (314, 000) Water Domestic database Pipeline Sea Database (93, 000) (320, 000) Air Database (51, 000) 14
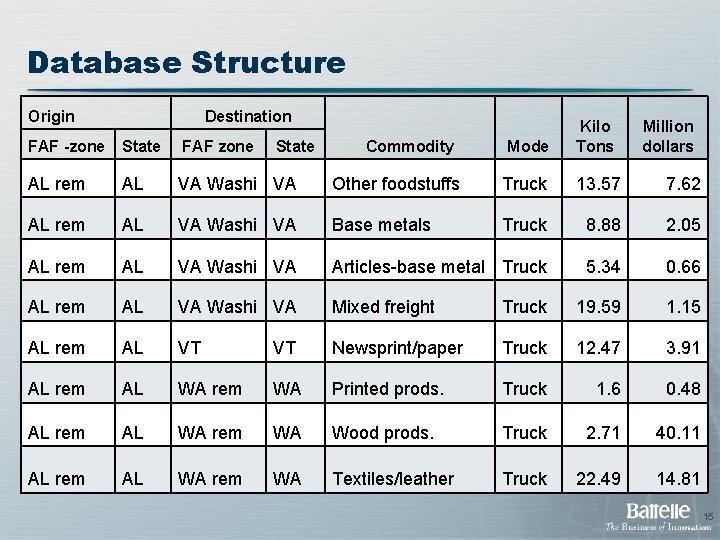
Database Structure Origin Destination Mode Kilo Tons Other foodstuffs Truck 13. 57 7. 62 VA Washi VA Base metals Truck 8. 88 2. 05 AL VA Washi VA Articles-base metal Truck 5. 34 0. 66 AL rem AL VA Washi VA Mixed freight Truck 19. 59 1. 15 AL rem AL VT VT Newsprint/paper Truck 12. 47 3. 91 AL rem AL WA rem WA Printed prods. Truck 1. 6 0. 48 AL rem AL WA rem WA Wood prods. Truck 2. 71 40. 11 AL rem AL WA rem WA Textiles/leather Truck 22. 49 14. 81 FAF -zone State FAF zone State AL rem AL VA Washi VA AL rem AL AL rem Commodity Million dollars 15
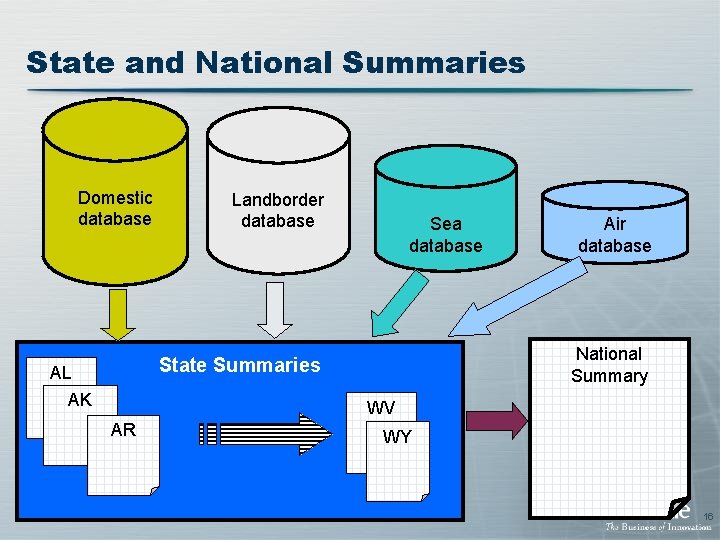
State and National Summaries Domestic database Landborder database Sea database National Summary State Summaries AL AK Air database WV AR WY 16
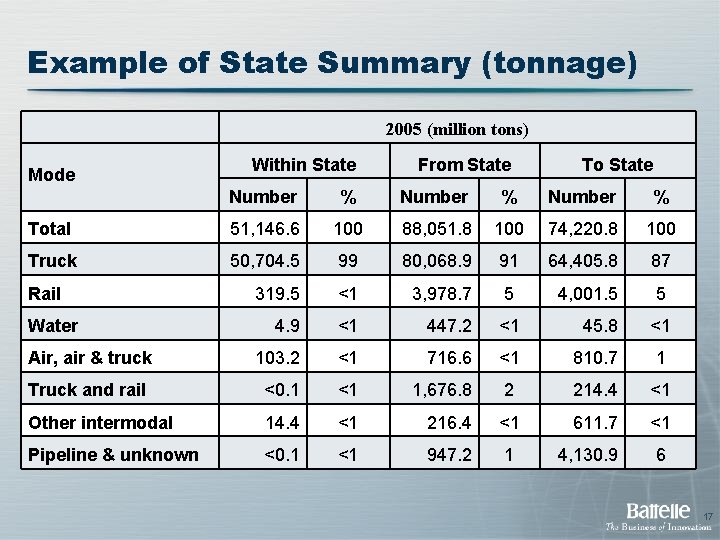
Example of State Summary (tonnage) 2005 (million tons) Mode Within State From State To State Number % Total 51, 146. 6 100 88, 051. 8 100 74, 220. 8 100 Truck 50, 704. 5 99 80, 068. 9 91 64, 405. 8 87 319. 5 <1 3, 978. 7 5 4, 001. 5 5 4. 9 <1 447. 2 <1 45. 8 <1 Air, air & truck 103. 2 <1 716. 6 <1 810. 7 1 Truck and rail <0. 1 <1 1, 676. 8 2 214. 4 <1 Other intermodal 14. 4 <1 216. 4 <1 611. 7 <1 Pipeline & unknown <0. 1 <1 947. 2 1 4, 130. 9 6 Rail Water 17
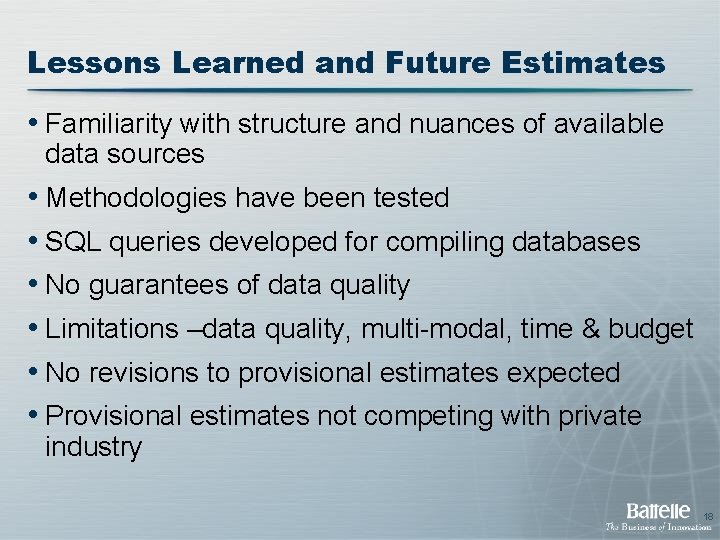
Lessons Learned and Future Estimates • Familiarity with structure and nuances of available data sources • Methodologies have been tested • SQL queries developed for compiling databases • No guarantees of data quality • Limitations –data quality, multi-modal, time & budget • No revisions to provisional estimates expected • Provisional estimates not competing with private industry 18
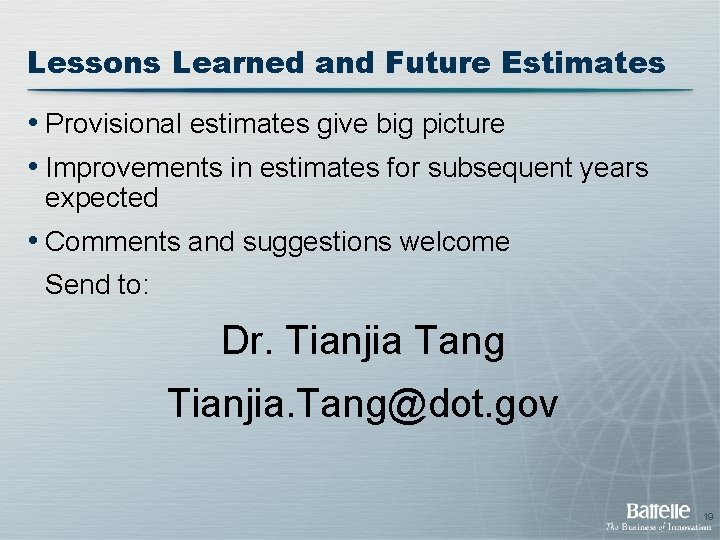
Lessons Learned and Future Estimates • Provisional estimates give big picture • Improvements in estimates for subsequent years expected • Comments and suggestions welcome Send to: Dr. Tianjia Tang Tianjia. Tang@dot. gov 19
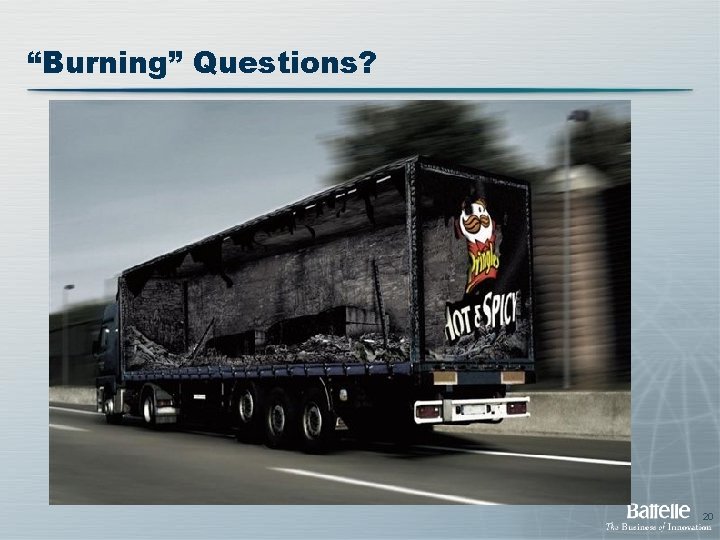
“Burning” Questions? 20
Building maintenance cost estimates
The account analysis method estimates cost functions
Jessie estimates the weight of her cat
Job order cost system
Marquis company estimates that annual manufacturing
State the properties of least square estimators.
Fermi estimate
Who global estimates on prevalence of hearing loss 2020
Creative industries economic estimates
フェルミ推定
Who global estimates on prevalence of hearing loss 2020
Eec brc provisionally approved
Din no means
Preformed polymer crowns
Arcon provisional registration
Inverted provisional stenting
Assessment of custom duty
Preformed polycarbonate crowns
Nurse verification bccnp
Harmonized commodity description and coding systems
Public opinion in pr