Causation in epidemiology confounding and bias Imre Janszky
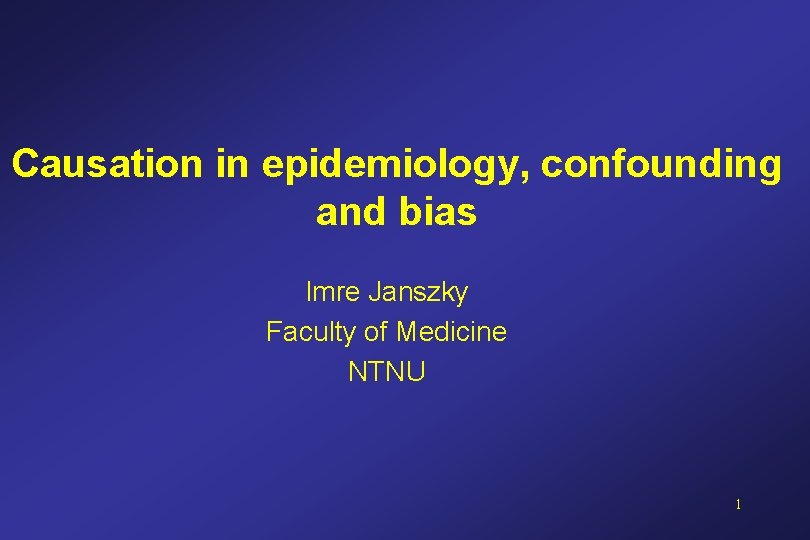
Causation in epidemiology, confounding and bias Imre Janszky Faculty of Medicine NTNU 1
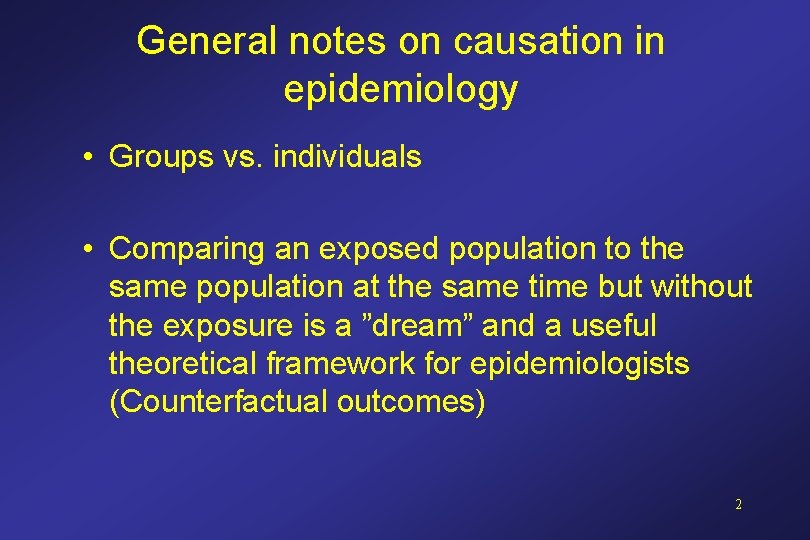
General notes on causation in epidemiology • Groups vs. individuals • Comparing an exposed population to the same population at the same time but without the exposure is a ”dream” and a useful theoretical framework for epidemiologists (Counterfactual outcomes) 2
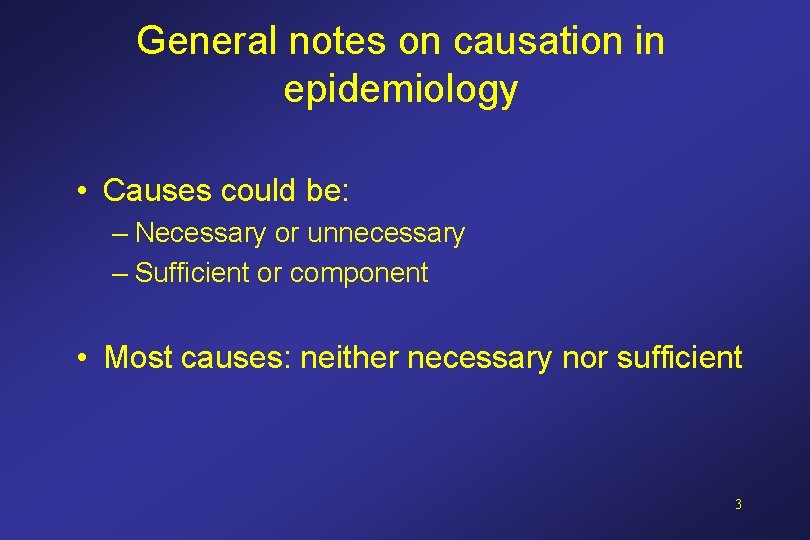
General notes on causation in epidemiology • Causes could be: – Necessary or unnecessary – Sufficient or component • Most causes: neither necessary nor sufficient 3
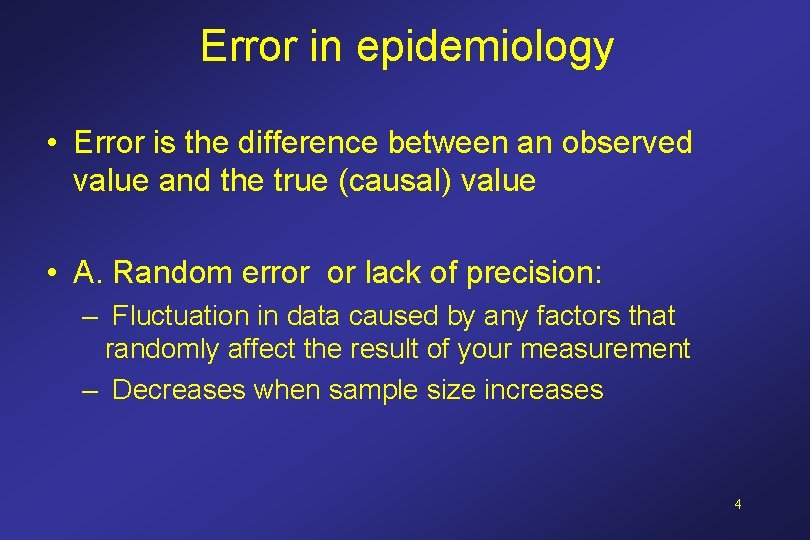
Error in epidemiology • Error is the difference between an observed value and the true (causal) value • A. Random error or lack of precision: – Fluctuation in data caused by any factors that randomly affect the result of your measurement – Decreases when sample size increases 4
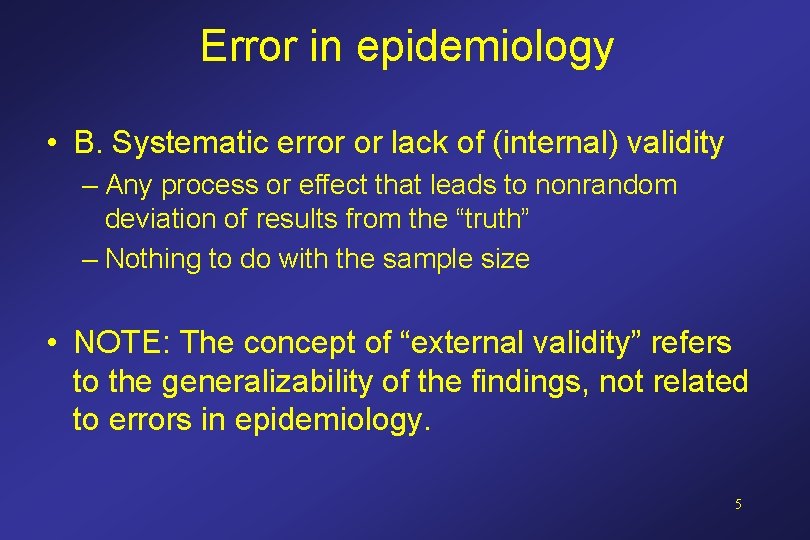
Error in epidemiology • B. Systematic error or lack of (internal) validity – Any process or effect that leads to nonrandom deviation of results from the “truth” – Nothing to do with the sample size • NOTE: The concept of “external validity” refers to the generalizability of the findings, not related to errors in epidemiology. 5
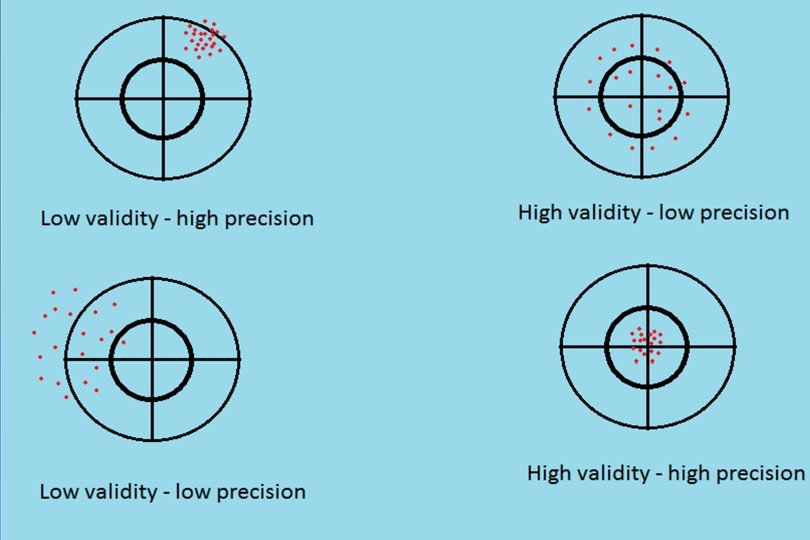
6
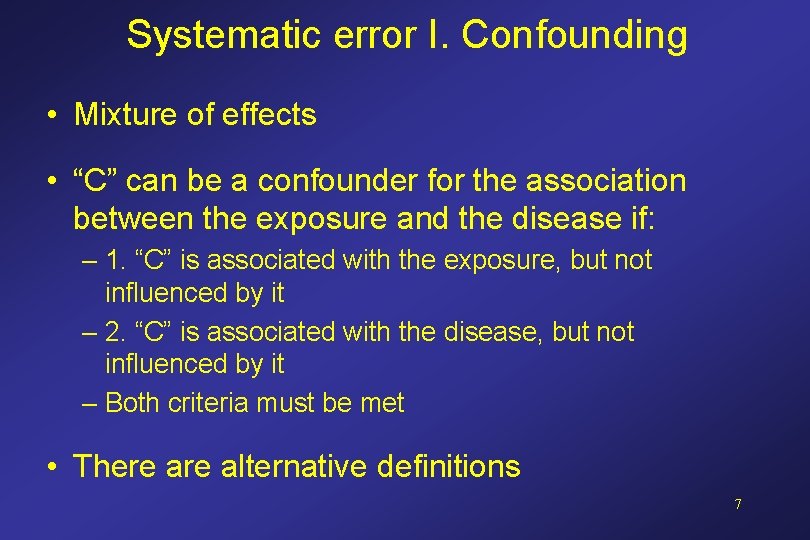
Systematic error I. Confounding • Mixture of effects • “C” can be a confounder for the association between the exposure and the disease if: – 1. “C” is associated with the exposure, but not influenced by it – 2. “C” is associated with the disease, but not influenced by it – Both criteria must be met • There alternative definitions 7
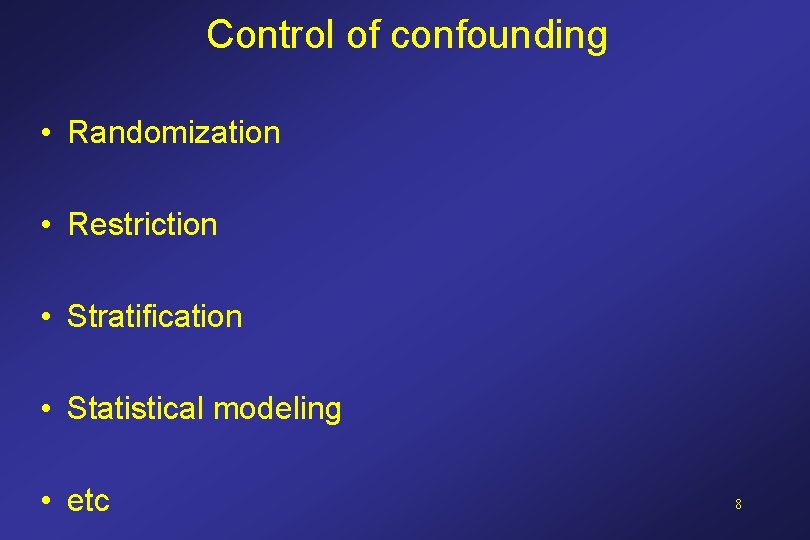
Control of confounding • Randomization • Restriction • Stratification • Statistical modeling • etc 8
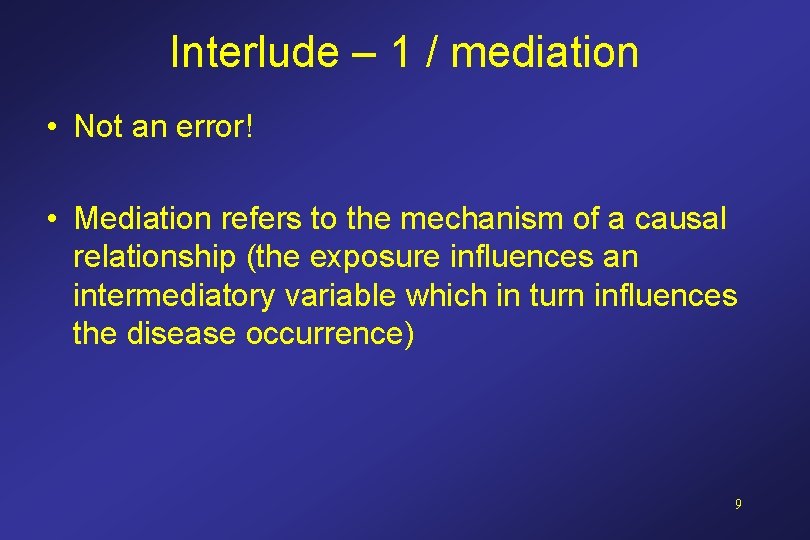
Interlude – 1 / mediation • Not an error! • Mediation refers to the mechanism of a causal relationship (the exposure influences an intermediatory variable which in turn influences the disease occurrence) 9
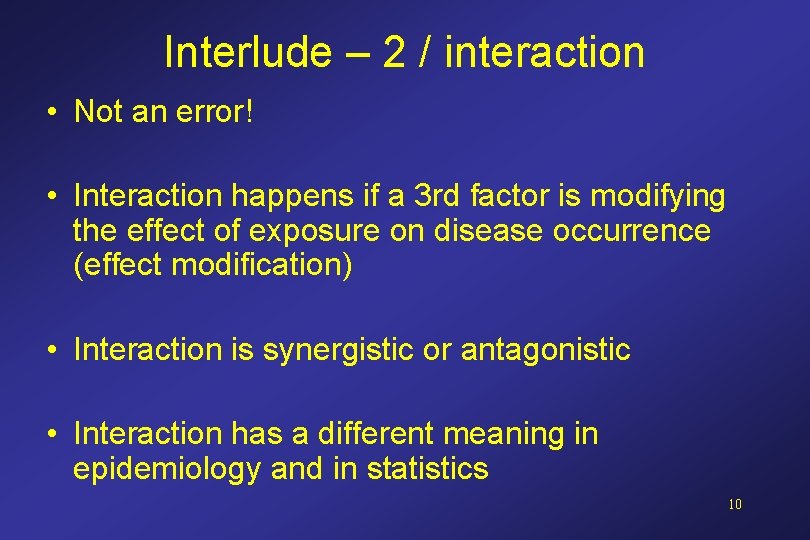
Interlude – 2 / interaction • Not an error! • Interaction happens if a 3 rd factor is modifying the effect of exposure on disease occurrence (effect modification) • Interaction is synergistic or antagonistic • Interaction has a different meaning in epidemiology and in statistics 10
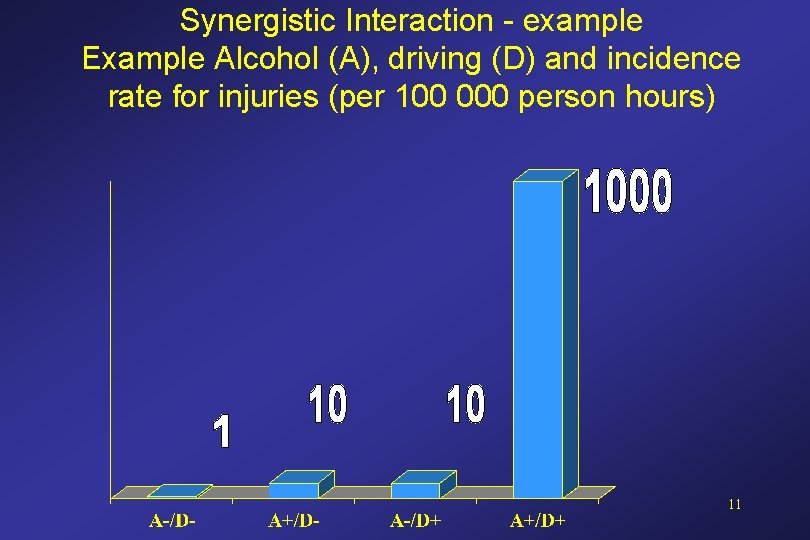
Synergistic Interaction - example Example Alcohol (A), driving (D) and incidence rate for injuries (per 100 000 person hours) 11
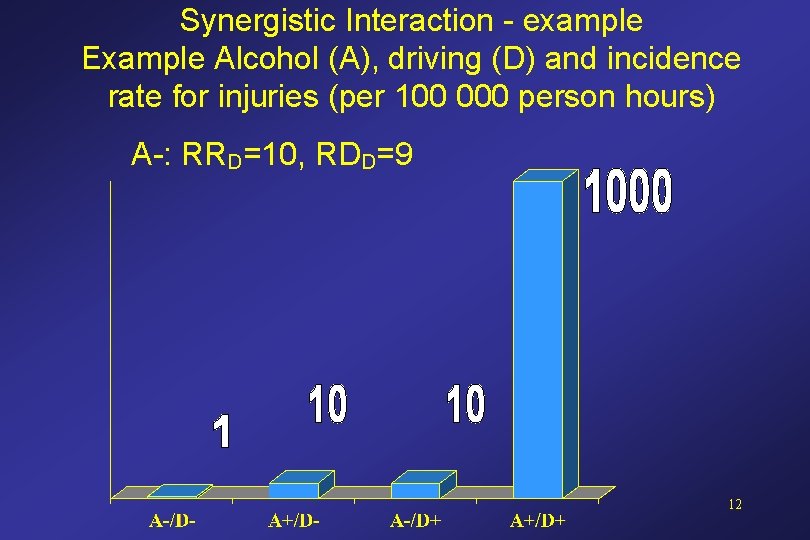
Synergistic Interaction - example Example Alcohol (A), driving (D) and incidence rate for injuries (per 100 000 person hours) A-: RRD=10, RDD=9 12
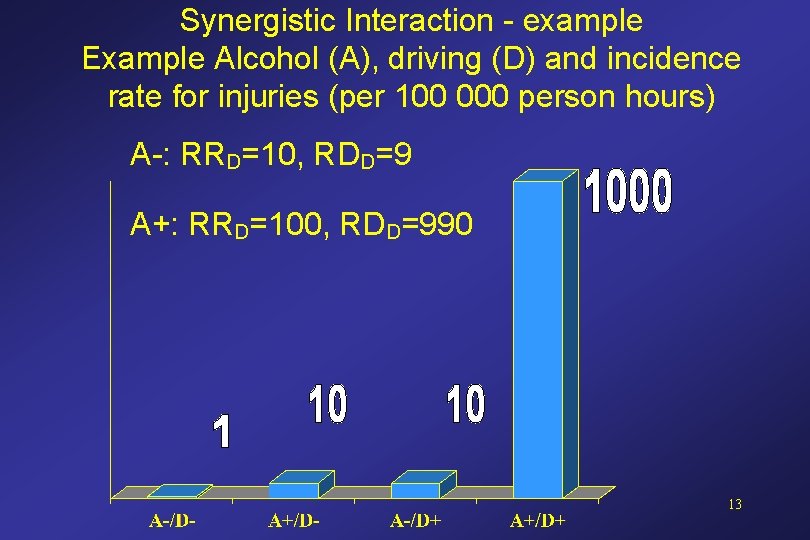
Synergistic Interaction - example Example Alcohol (A), driving (D) and incidence rate for injuries (per 100 000 person hours) A-: RRD=10, RDD=9 A+: RRD=100, RDD=990 13
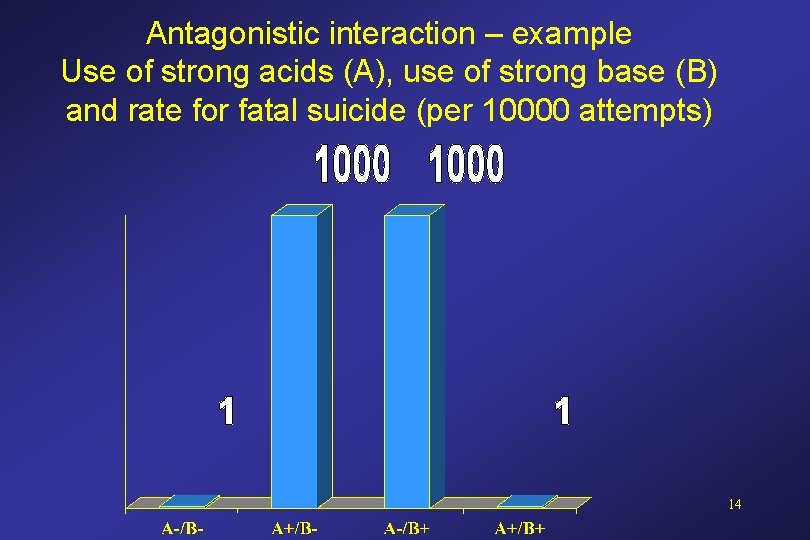
Antagonistic interaction – example Use of strong acids (A), use of strong base (B) and rate for fatal suicide (per 10000 attempts) 14
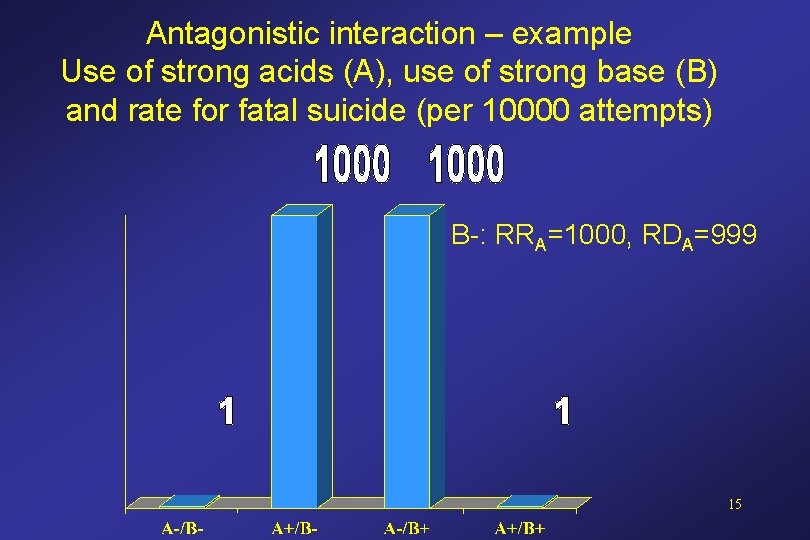
Antagonistic interaction – example Use of strong acids (A), use of strong base (B) and rate for fatal suicide (per 10000 attempts) B-: RRA=1000, RDA=999 15
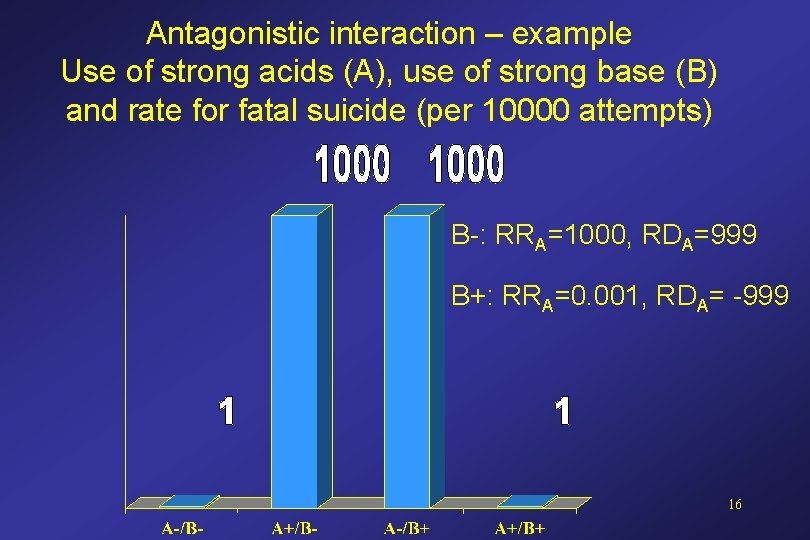
Antagonistic interaction – example Use of strong acids (A), use of strong base (B) and rate for fatal suicide (per 10000 attempts) B-: RRA=1000, RDA=999 B+: RRA=0. 001, RDA= -999 16
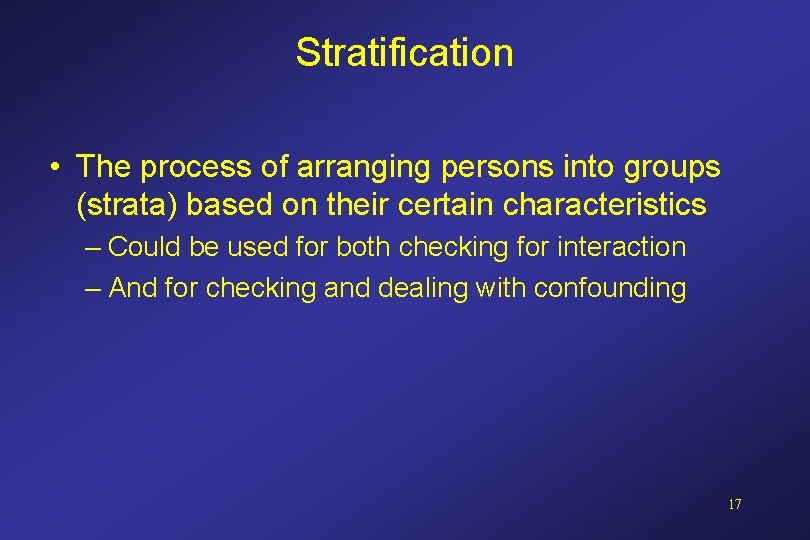
Stratification • The process of arranging persons into groups (strata) based on their certain characteristics – Could be used for both checking for interaction – And for checking and dealing with confounding 17
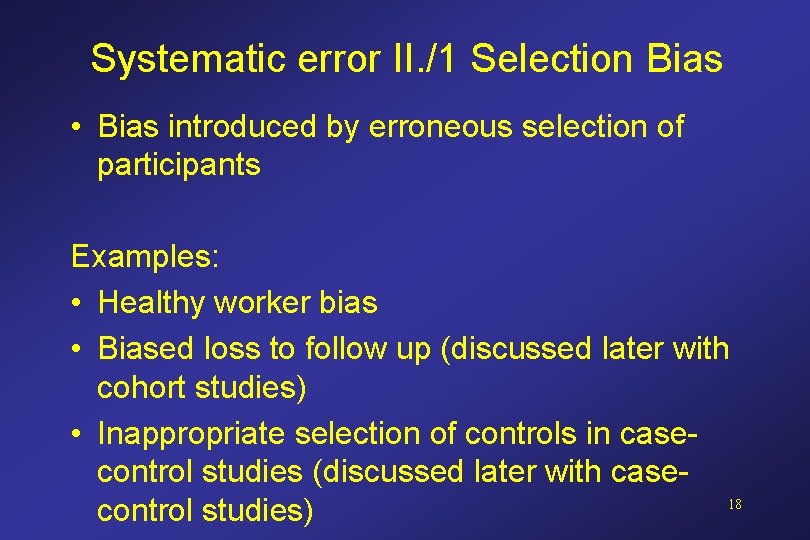
Systematic error II. /1 Selection Bias • Bias introduced by erroneous selection of participants Examples: • Healthy worker bias • Biased loss to follow up (discussed later with cohort studies) • Inappropriate selection of controls in casecontrol studies (discussed later with case 18 control studies)
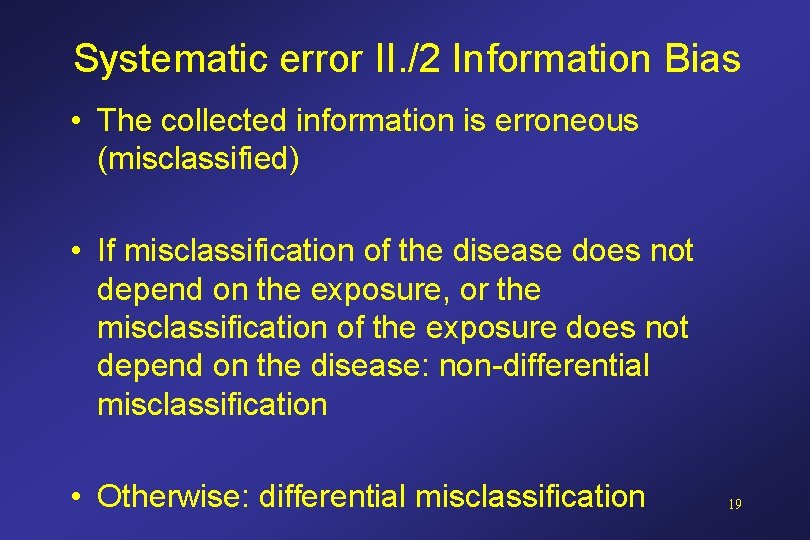
Systematic error II. /2 Information Bias • The collected information is erroneous (misclassified) • If misclassification of the disease does not depend on the exposure, or the misclassification of the exposure does not depend on the disease: non-differential misclassification • Otherwise: differential misclassification 19
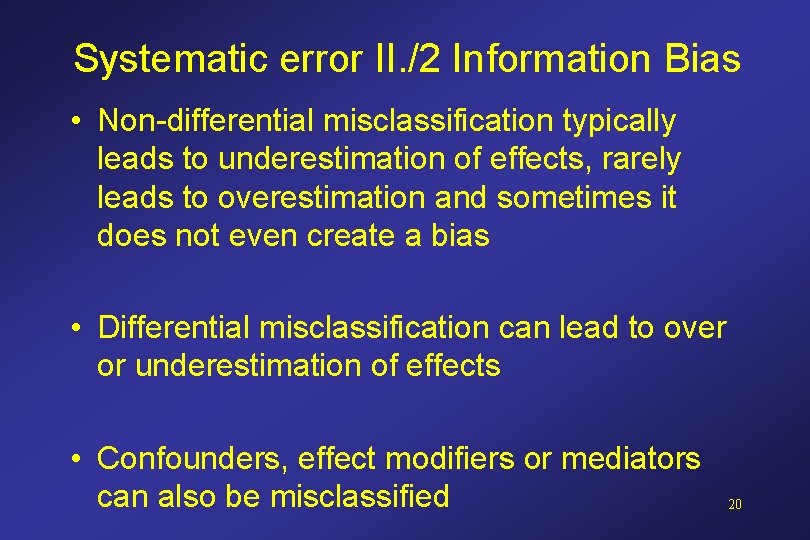
Systematic error II. /2 Information Bias • Non-differential misclassification typically leads to underestimation of effects, rarely leads to overestimation and sometimes it does not even create a bias • Differential misclassification can lead to over or underestimation of effects • Confounders, effect modifiers or mediators can also be misclassified 20
- Slides: 20