0 Wisdom of The Crowd Lirong Xia Example
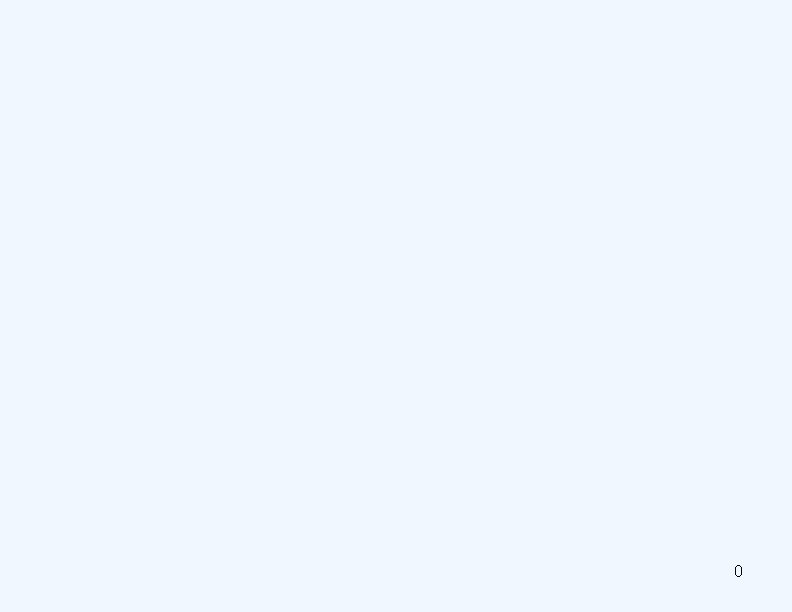
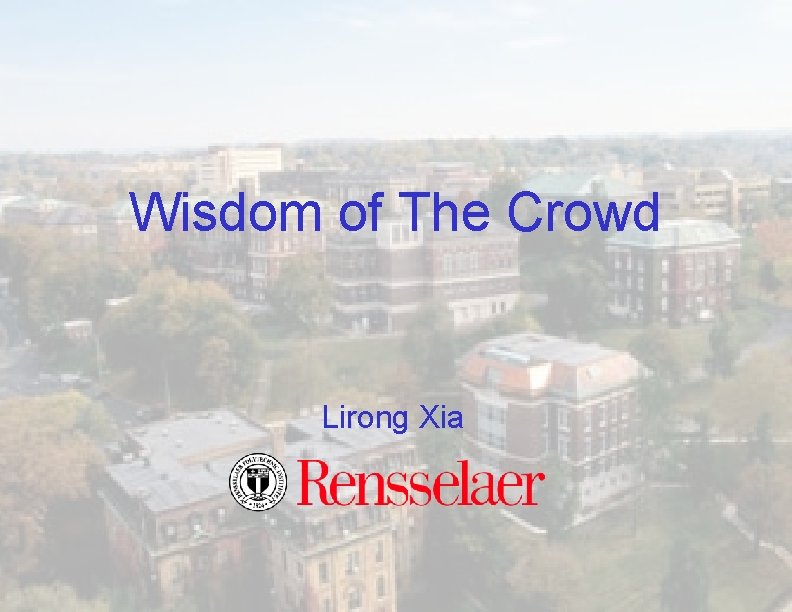
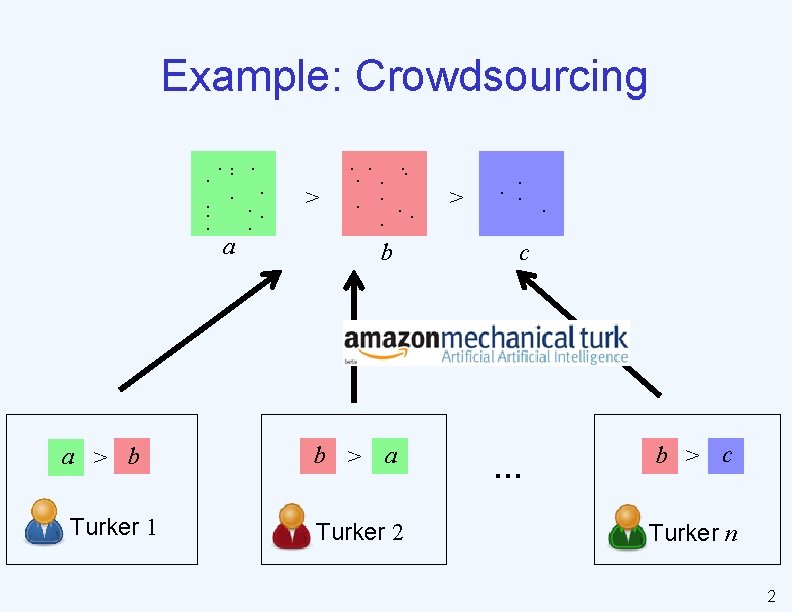
![The Condorcet Jury theorem [Condorcet 1785] The Condorcet Jury theorem. Pr( | ) = The Condorcet Jury theorem [Condorcet 1785] The Condorcet Jury theorem. Pr( | ) =](https://slidetodoc.com/presentation_image_h2/9759136a6dd971c990872d29703bfff2/image-4.jpg)
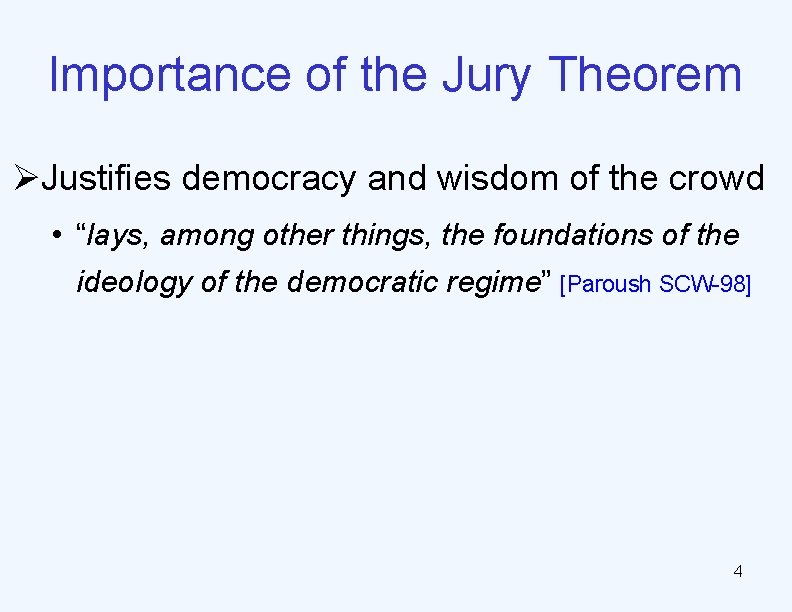
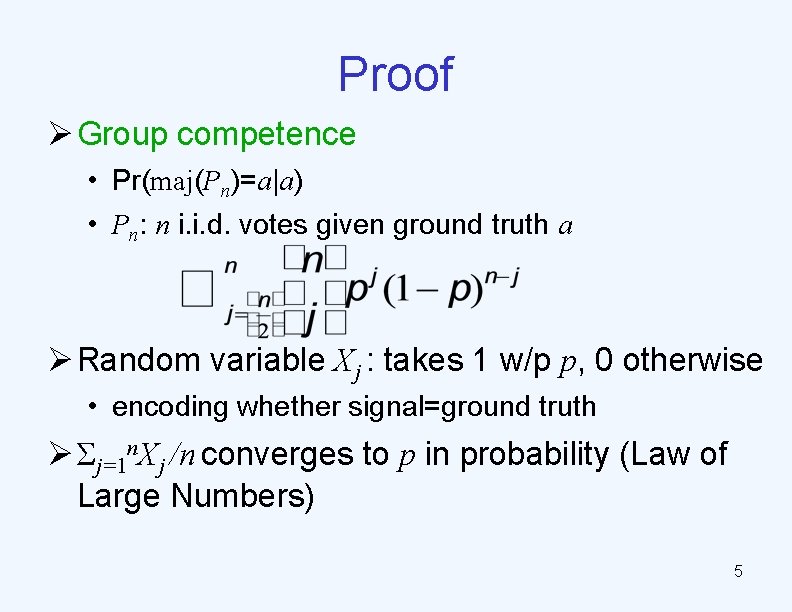
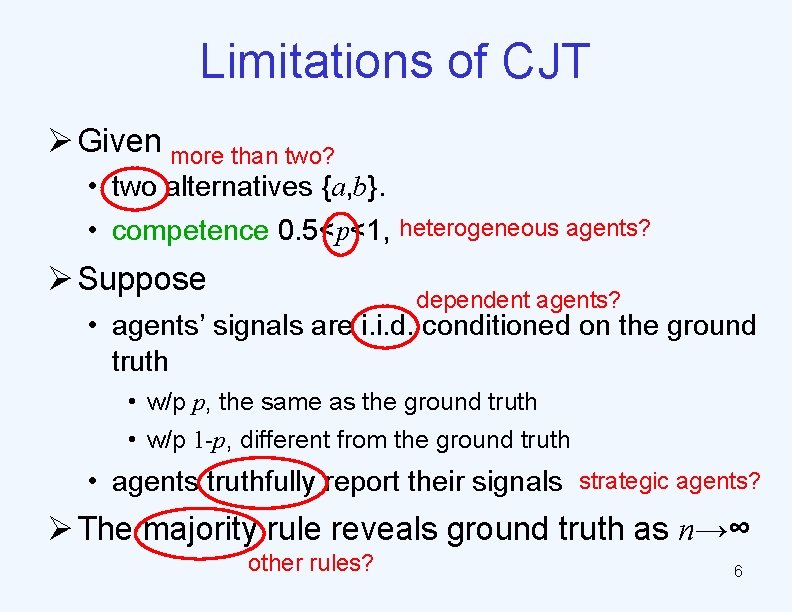
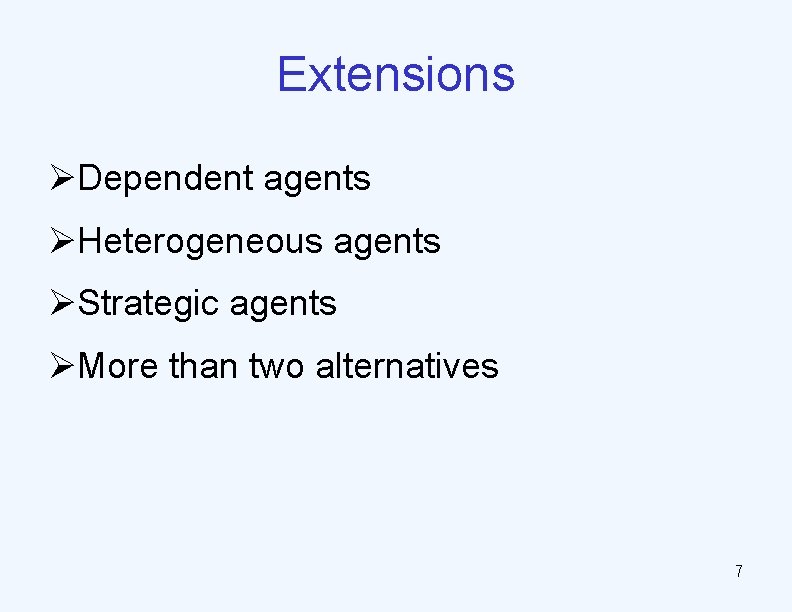
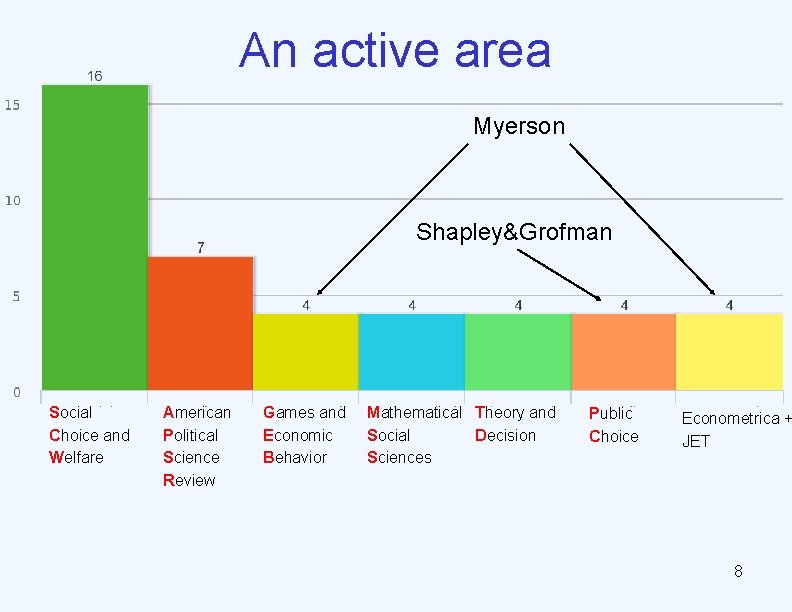
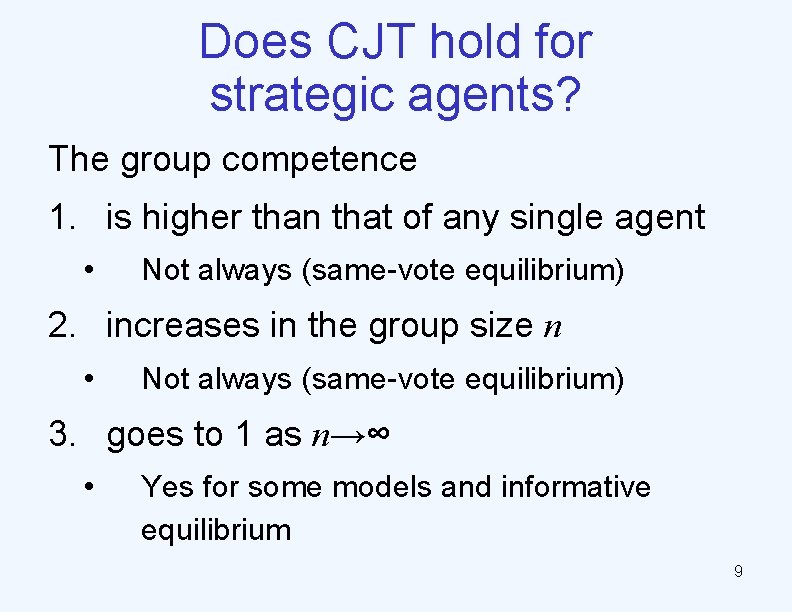
![Strategic voting Ø Common interest Bayesian voting game [Austen. Smith&Banks APSR-96] • two alternatives Strategic voting Ø Common interest Bayesian voting game [Austen. Smith&Banks APSR-96] • two alternatives](https://slidetodoc.com/presentation_image_h2/9759136a6dd971c990872d29703bfff2/image-11.jpg)
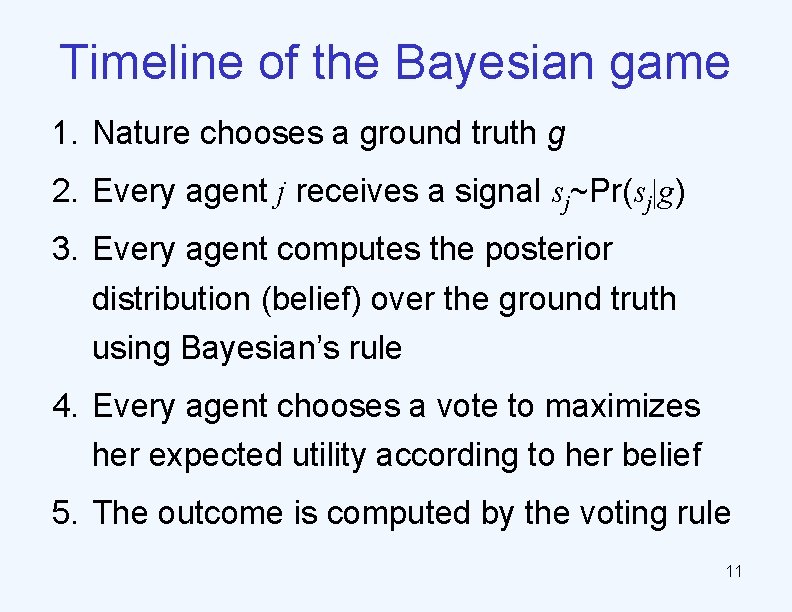
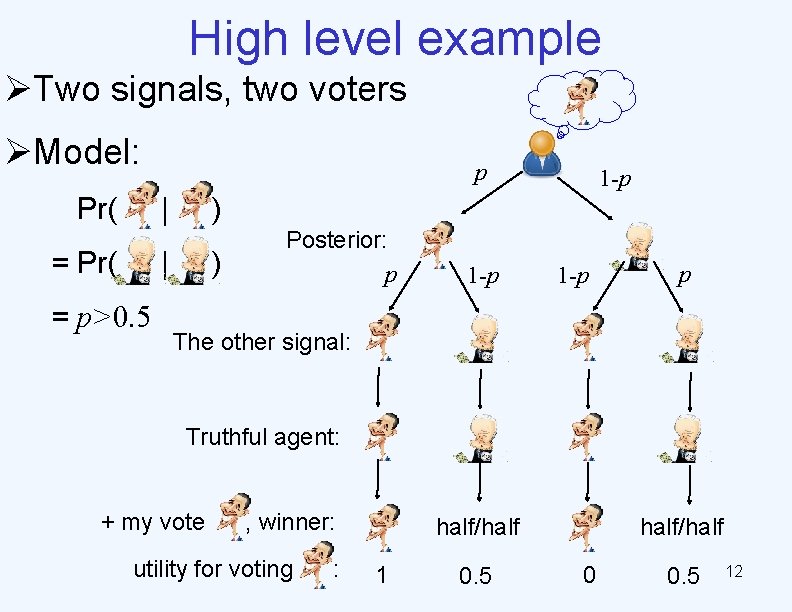
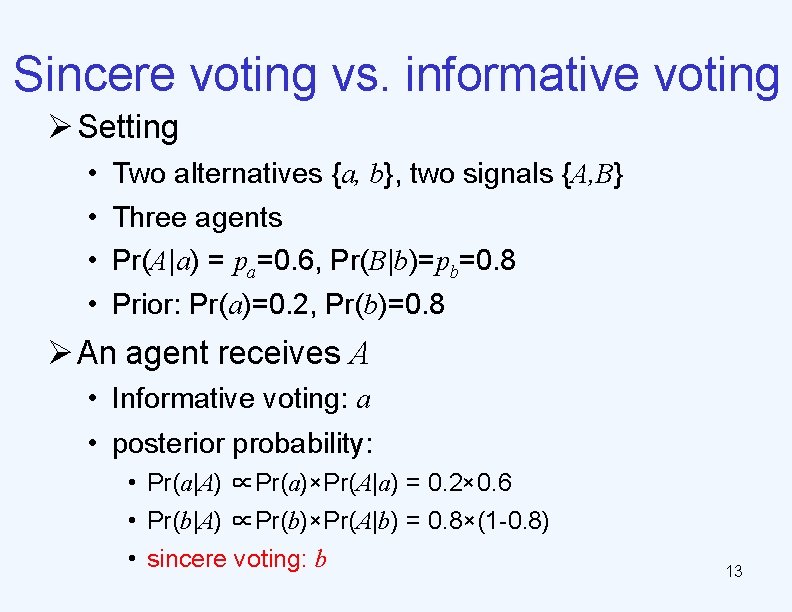
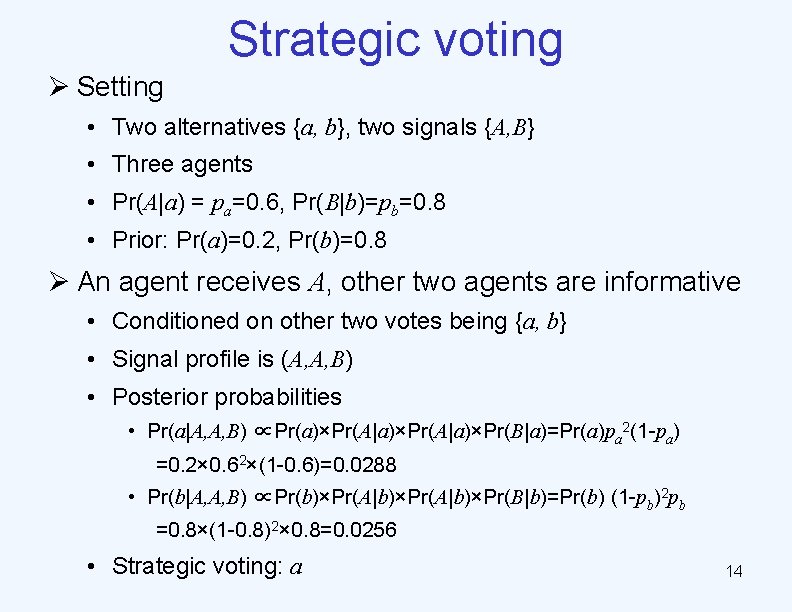
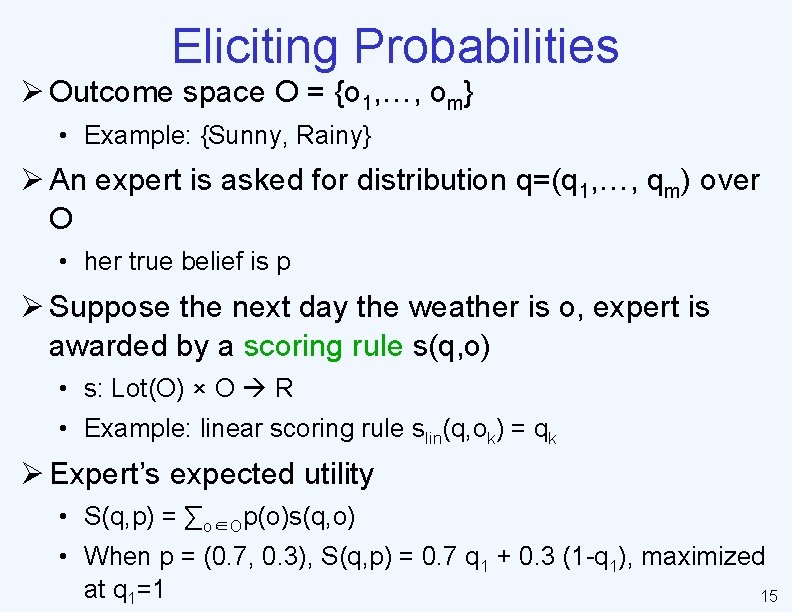
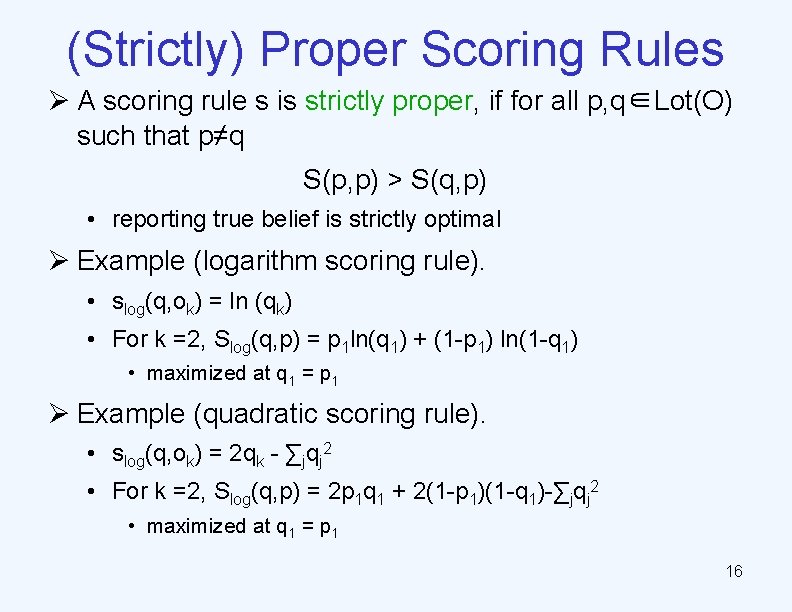
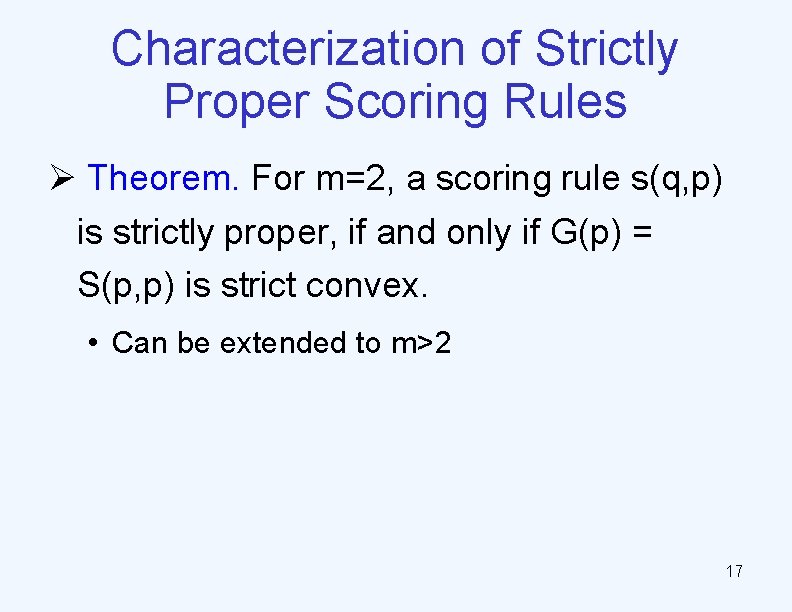
- Slides: 18
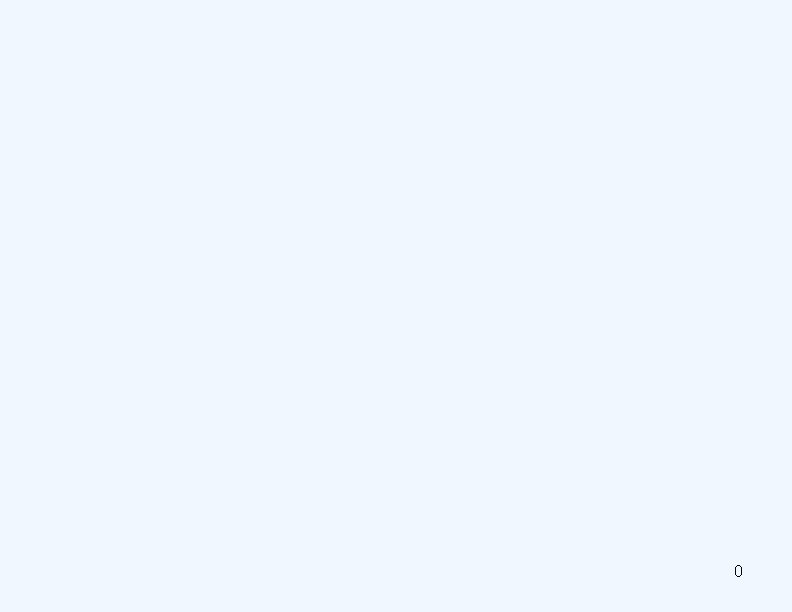
0
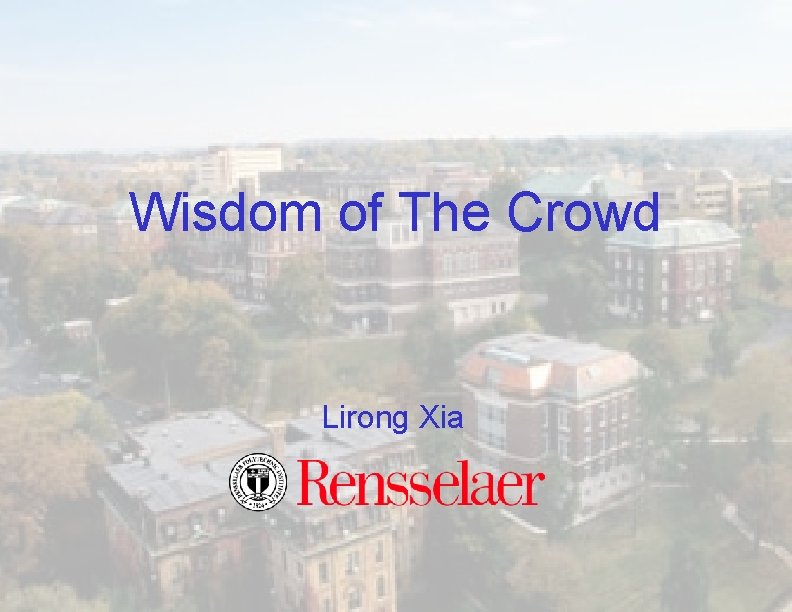
Wisdom of The Crowd Lirong Xia
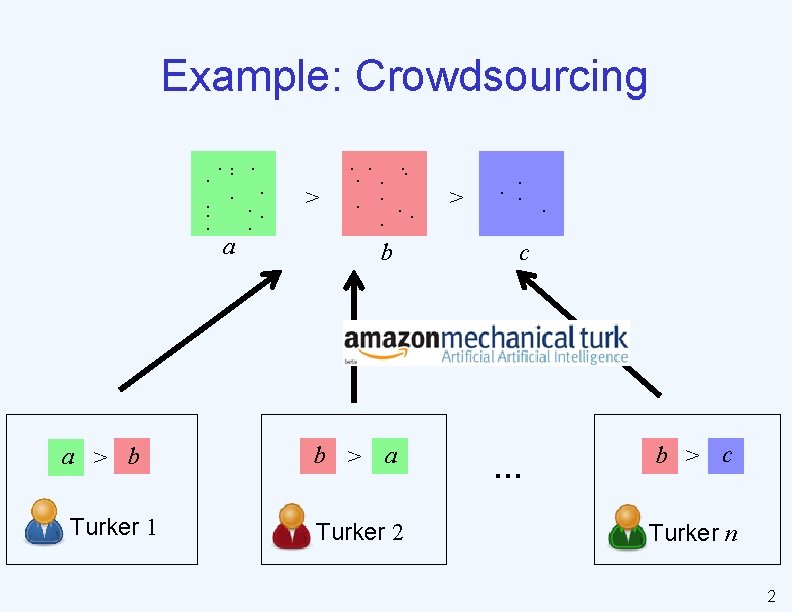
Example: Crowdsourcing. . . . a a > b Turker 1 . . . > . . . b b > a Turker 2 > . . c … b > c Turker n 2
![The Condorcet Jury theorem Condorcet 1785 The Condorcet Jury theorem Pr The Condorcet Jury theorem [Condorcet 1785] The Condorcet Jury theorem. Pr( | ) =](https://slidetodoc.com/presentation_image_h2/9759136a6dd971c990872d29703bfff2/image-4.jpg)
The Condorcet Jury theorem [Condorcet 1785] The Condorcet Jury theorem. Pr( | ) = Pr( | ) Ø Given • two alternatives {O, M}. • 0. 5<p<1, Ø Suppose = p>0. 5 • each agent’s preferences is generated i. i. d. , such that • w/p p, the same as the ground truth • w/p 1 -p, different from the ground truth Ø Then, as n→∞, the majority of agents’ preferences converges in probability to the ground truth 3
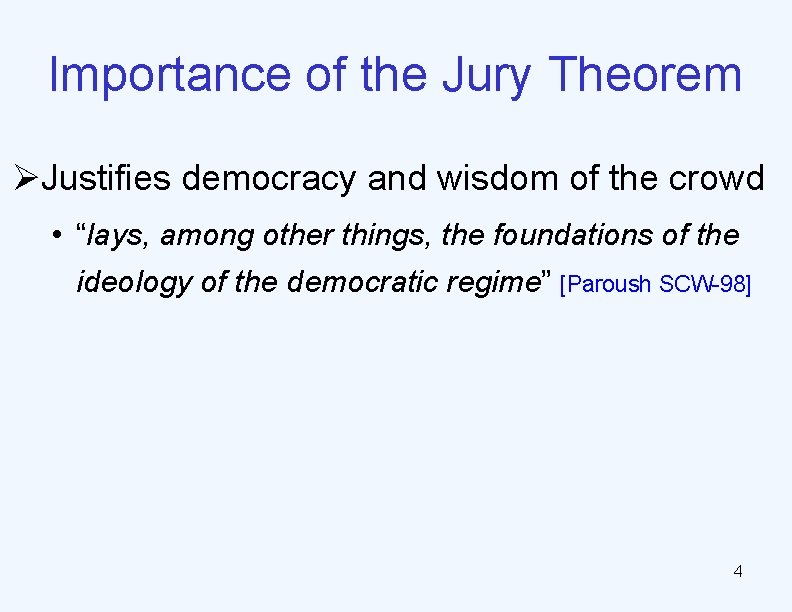
Importance of the Jury Theorem ØJustifies democracy and wisdom of the crowd • “lays, among other things, the foundations of the ideology of the democratic regime” [Paroush SCW-98] 4
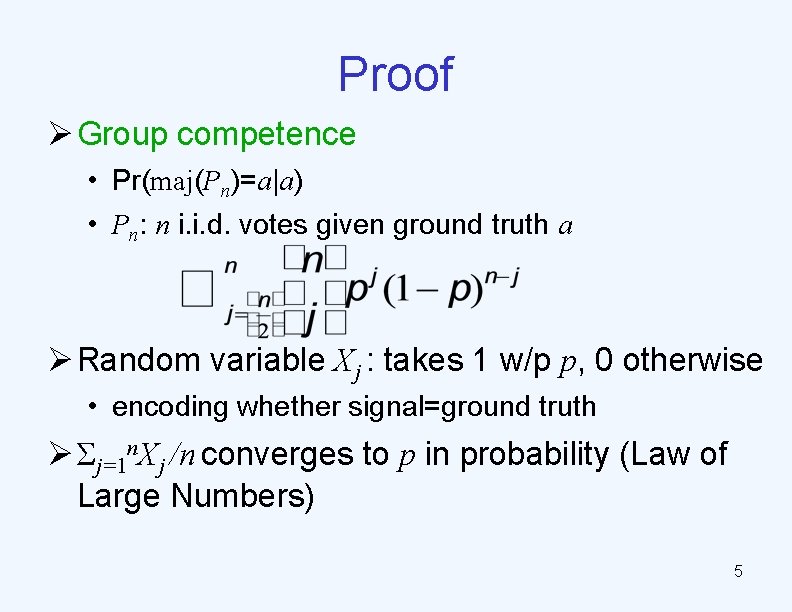
Proof Ø Group competence • Pr(maj(Pn)=a|a) • Pn: n i. i. d. votes given ground truth a Ø Random variable Xj : takes 1 w/p p, 0 otherwise • encoding whether signal=ground truth Ø Σj=1 n. Xj /n converges to p in probability (Law of Large Numbers) 5
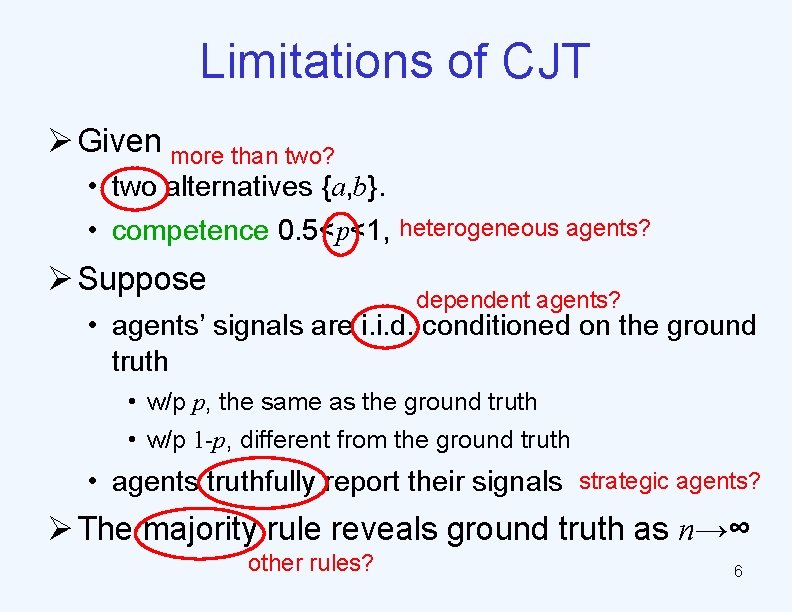
Limitations of CJT Ø Given more than two? • two alternatives {a, b}. • competence 0. 5<p<1, heterogeneous agents? Ø Suppose dependent agents? • agents’ signals are i. i. d. conditioned on the ground truth • w/p p, the same as the ground truth • w/p 1 -p, different from the ground truth • agents truthfully report their signals strategic agents? Ø The majority rule reveals ground truth as n→∞ other rules? 6
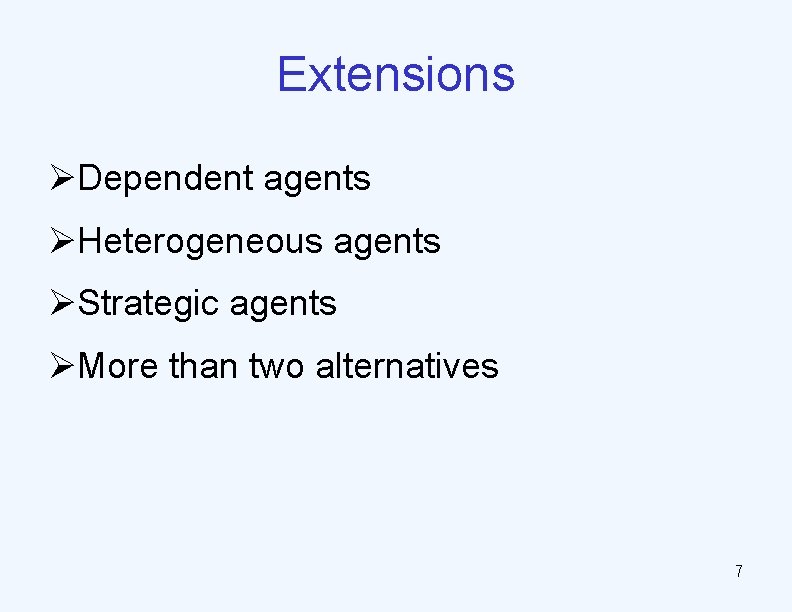
Extensions ØDependent agents ØHeterogeneous agents ØStrategic agents ØMore than two alternatives 7
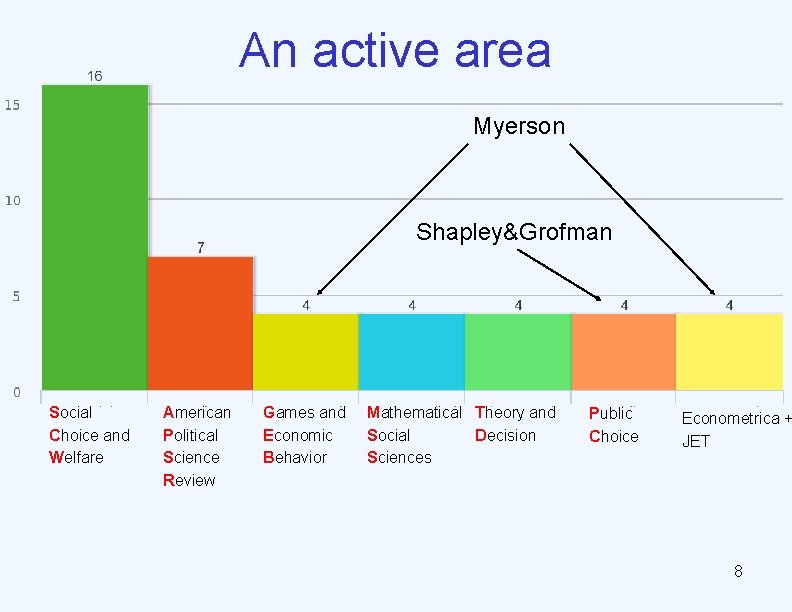
An active area Myerson Shapley&Grofman Social Choice and Welfare American Political Science Review Games and Economic Behavior Mathematical Theory and Social Decision Sciences Public Choice Econometrica + JET 8
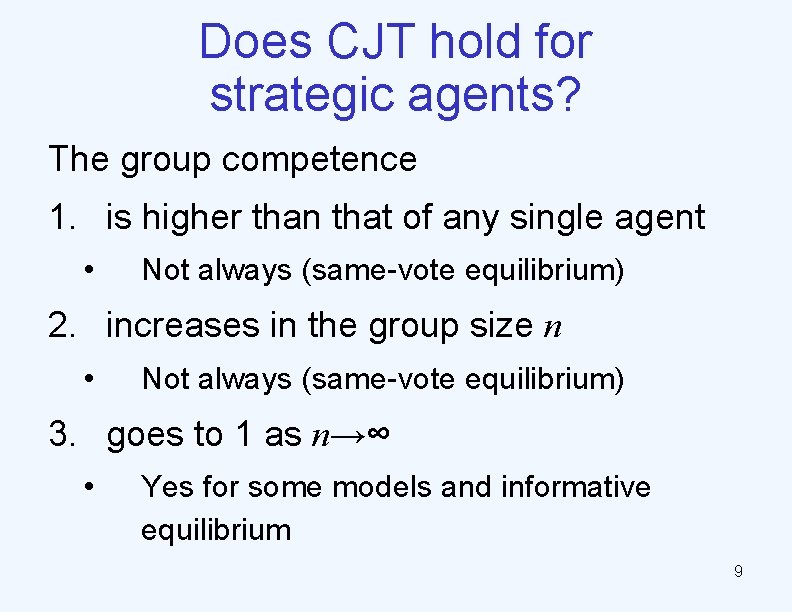
Does CJT hold for strategic agents? The group competence 1. is higher than that of any single agent • Not always (same-vote equilibrium) 2. increases in the group size n • Not always (same-vote equilibrium) 3. goes to 1 as n→∞ • Yes for some models and informative equilibrium 9
![Strategic voting Ø Common interest Bayesian voting game Austen SmithBanks APSR96 two alternatives Strategic voting Ø Common interest Bayesian voting game [Austen. Smith&Banks APSR-96] • two alternatives](https://slidetodoc.com/presentation_image_h2/9759136a6dd971c990872d29703bfff2/image-11.jpg)
Strategic voting Ø Common interest Bayesian voting game [Austen. Smith&Banks APSR-96] • two alternatives {a, b}, two signals {A, B}, a prior, Pr(signal|truth), • pa=Pr(signal=A|truth=a) • pb=Pr(signal=B|truth=b) • agents have the same utility function U(outcome, ground truth) =1 iff outcome = ground truth • sincere voting: vote for the alternative with the highest posterior probability • informative voting: vote for the signal • strategic voting: vote for the alternative with the highest expected utility 10
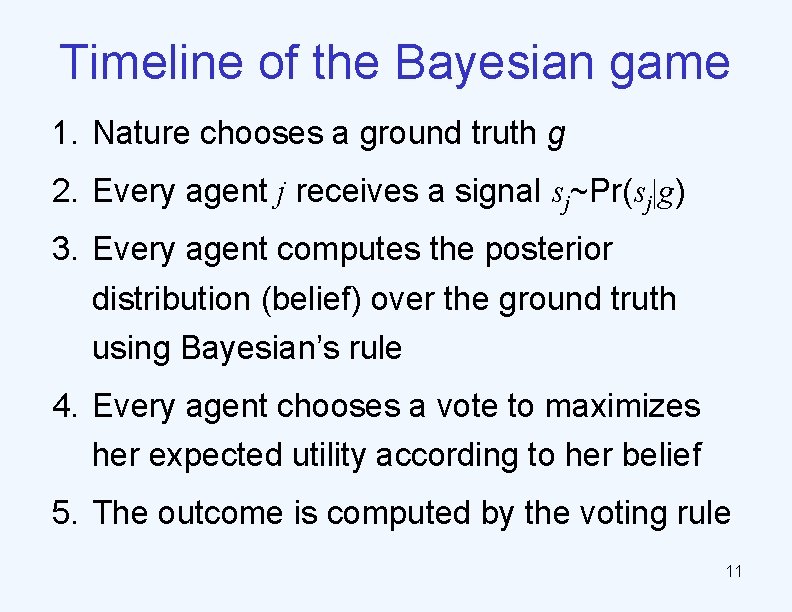
Timeline of the Bayesian game 1. Nature chooses a ground truth g 2. Every agent j receives a signal sj~Pr(sj|g) 3. Every agent computes the posterior distribution (belief) over the ground truth using Bayesian’s rule 4. Every agent chooses a vote to maximizes her expected utility according to her belief 5. The outcome is computed by the voting rule 11
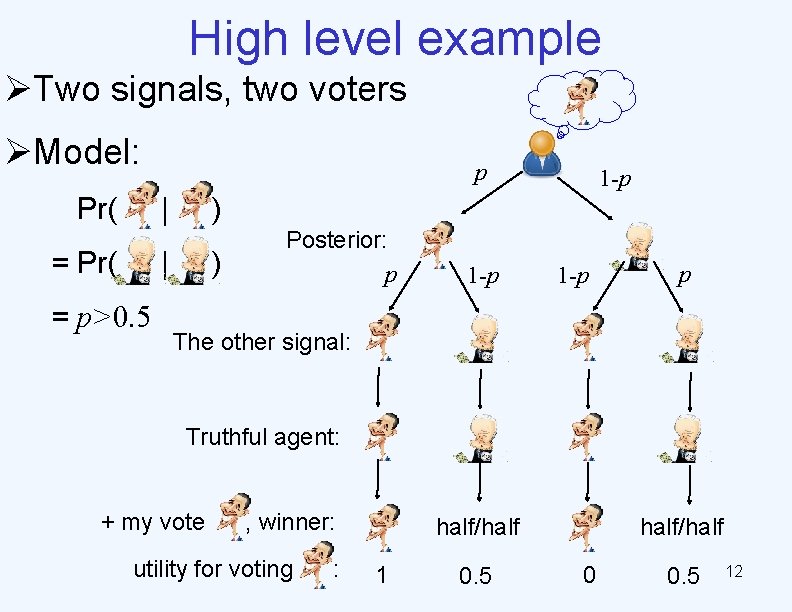
High level example ØTwo signals, two voters ØModel: Pr( p | = Pr( ) | = p>0. 5 1 -p ) Posterior: p 1 -p p The other signal: Truthful agent: + my vote , winner: utility for voting : half/half 1 0. 5 half/half 0 0. 5 12
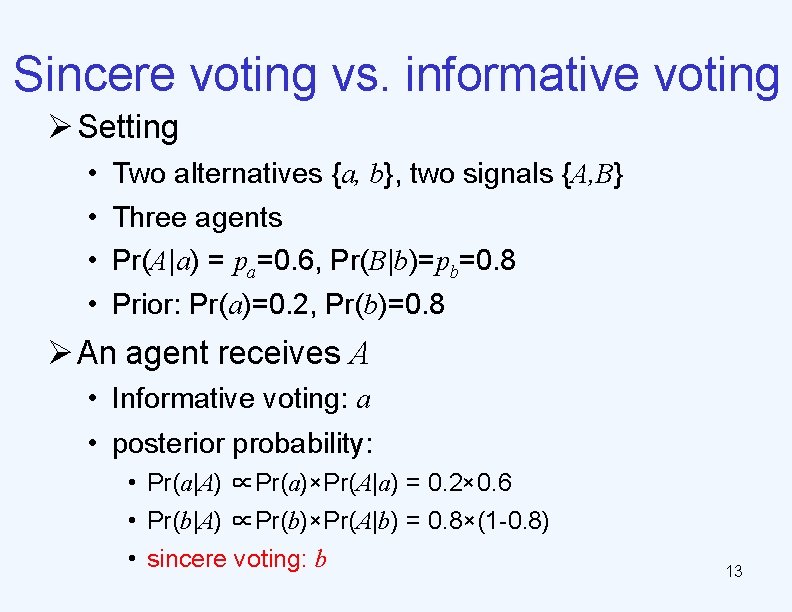
Sincere voting vs. informative voting Ø Setting • • Two alternatives {a, b}, two signals {A, B} Three agents Pr(A|a) = pa=0. 6, Pr(B|b)=pb=0. 8 Prior: Pr(a)=0. 2, Pr(b)=0. 8 Ø An agent receives A • Informative voting: a • posterior probability: • Pr(a|A) ∝Pr(a)×Pr(A|a) = 0. 2× 0. 6 • Pr(b|A) ∝Pr(b)×Pr(A|b) = 0. 8×(1 -0. 8) • sincere voting: b 13
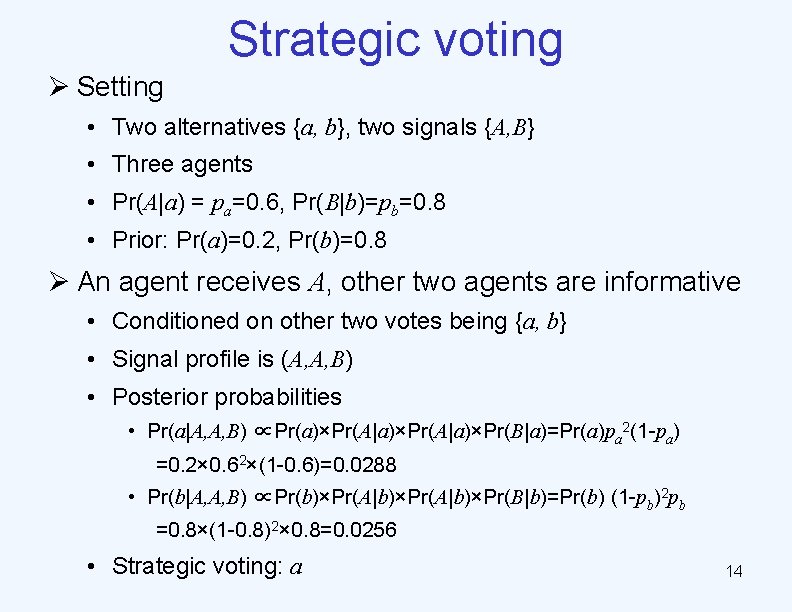
Strategic voting Ø Setting • Two alternatives {a, b}, two signals {A, B} • Three agents • Pr(A|a) = pa=0. 6, Pr(B|b)=pb=0. 8 • Prior: Pr(a)=0. 2, Pr(b)=0. 8 Ø An agent receives A, other two agents are informative • Conditioned on other two votes being {a, b} • Signal profile is (A, A, B) • Posterior probabilities • Pr(a|A, A, B) ∝Pr(a)×Pr(A|a)×Pr(B|a)=Pr(a)pa 2(1 -pa) =0. 2× 0. 62×(1 -0. 6)=0. 0288 • Pr(b|A, A, B) ∝Pr(b)×Pr(A|b)×Pr(B|b)=Pr(b) (1 -pb)2 pb =0. 8×(1 -0. 8)2× 0. 8=0. 0256 • Strategic voting: a 14
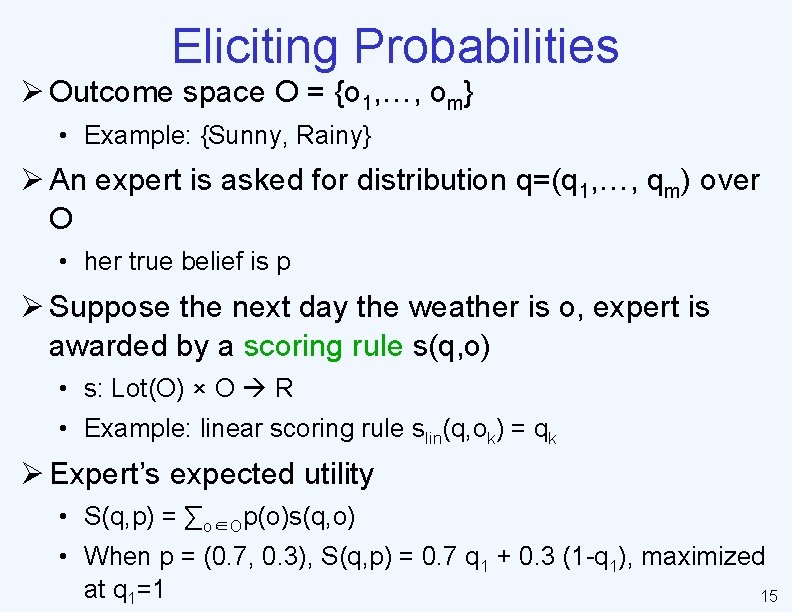
Eliciting Probabilities Ø Outcome space O = {o 1, …, om} • Example: {Sunny, Rainy} Ø An expert is asked for distribution q=(q 1, …, qm) over O • her true belief is p Ø Suppose the next day the weather is o, expert is awarded by a scoring rule s(q, o) • s: Lot(O) × O R • Example: linear scoring rule slin(q, ok) = qk Ø Expert’s expected utility • S(q, p) = ∑o∈Op(o)s(q, o) • When p = (0. 7, 0. 3), S(q, p) = 0. 7 q 1 + 0. 3 (1 -q 1), maximized at q 1=1 15
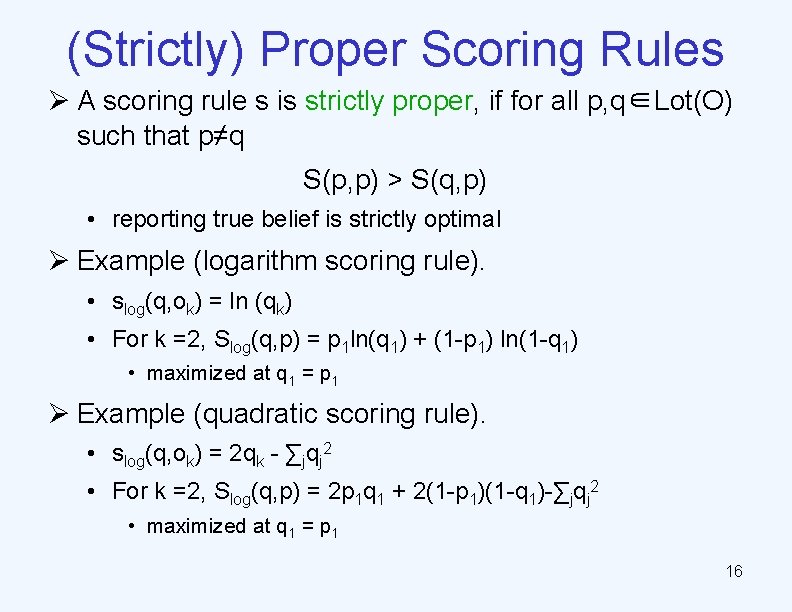
(Strictly) Proper Scoring Rules Ø A scoring rule s is strictly proper, if for all p, q∈Lot(O) such that p≠q S(p, p) > S(q, p) • reporting true belief is strictly optimal Ø Example (logarithm scoring rule). • slog(q, ok) = ln (qk) • For k =2, Slog(q, p) = p 1 ln(q 1) + (1 -p 1) ln(1 -q 1) • maximized at q 1 = p 1 Ø Example (quadratic scoring rule). • slog(q, ok) = 2 qk - ∑jqj 2 • For k =2, Slog(q, p) = 2 p 1 q 1 + 2(1 -p 1)(1 -q 1)-∑jqj 2 • maximized at q 1 = p 1 16
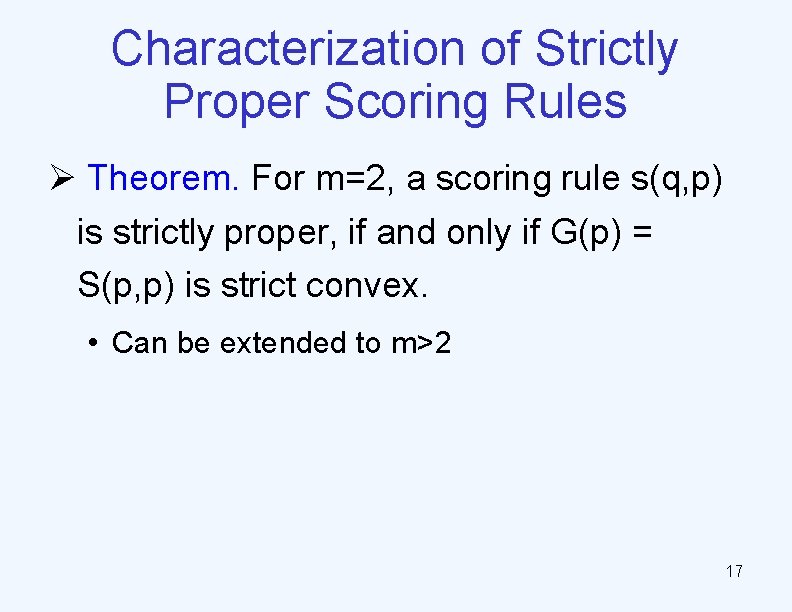
Characterization of Strictly Proper Scoring Rules Ø Theorem. For m=2, a scoring rule s(q, p) is strictly proper, if and only if G(p) = S(p, p) is strict convex. • Can be extended to m>2 17
Lirong xia
Lirong xia rpi
James 3 earthly wisdom
Godly wisdom vs worldly wisdom
Heavenly wisdom
Hsia dynasty
Guoxing xia
Jennifer xia
Dr xia wang
Bejing opera mask
Unang dinastiya sa china
Longest chinese dynasty
Albert xia
Tian shang tian xia wei wo du zun
Svitlana vyetrenko
Contributions of shang dynasty
Guoxing xia
Ningxia red testimonios
Xuhua xia rate my prof