WP 4 Model Adaptation Georgios Petasis Athens 16
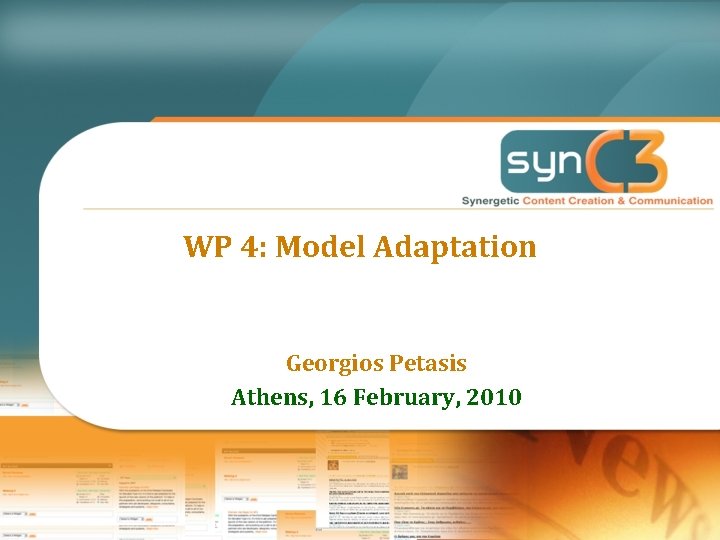
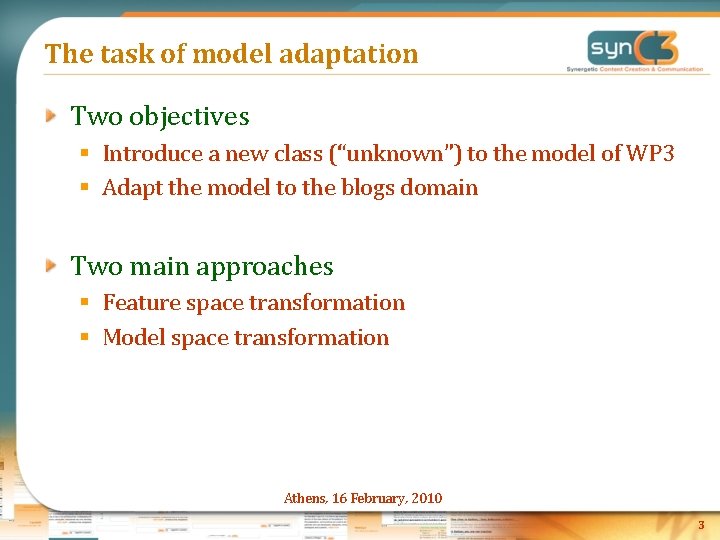
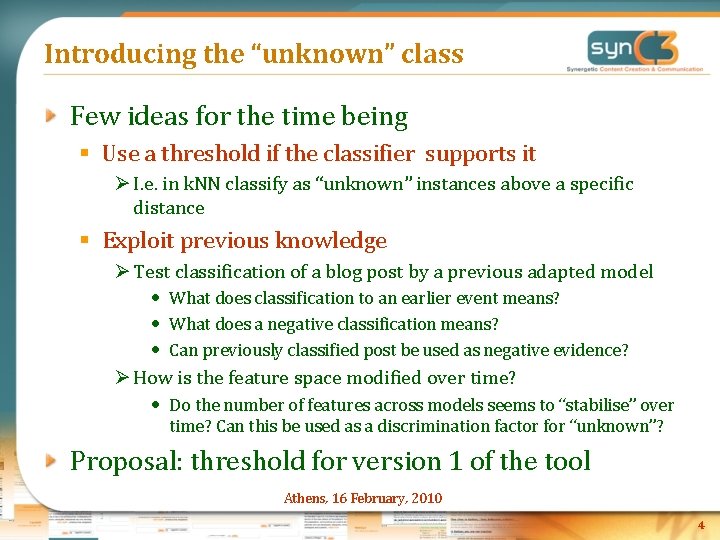
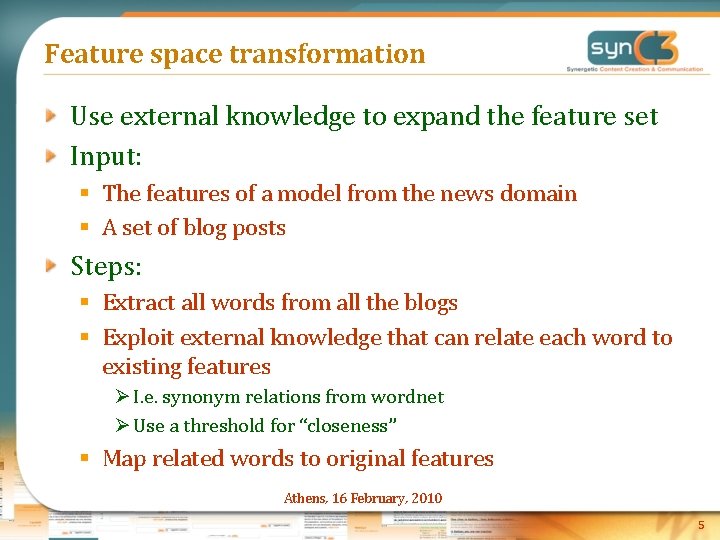
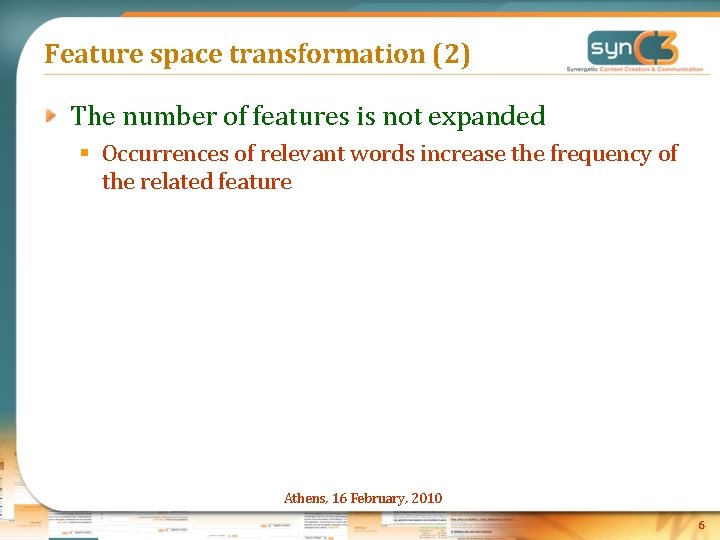
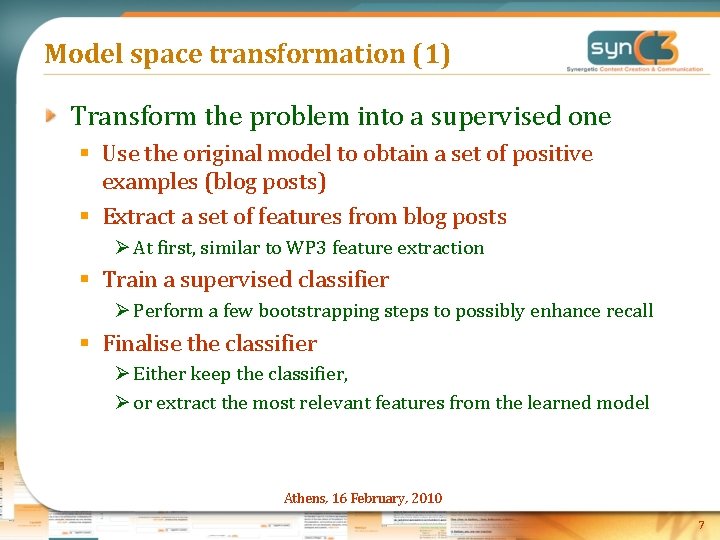
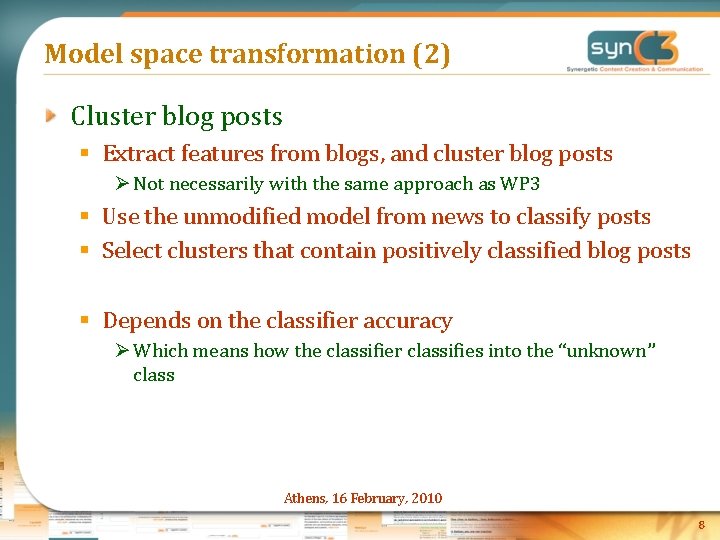
- Slides: 7
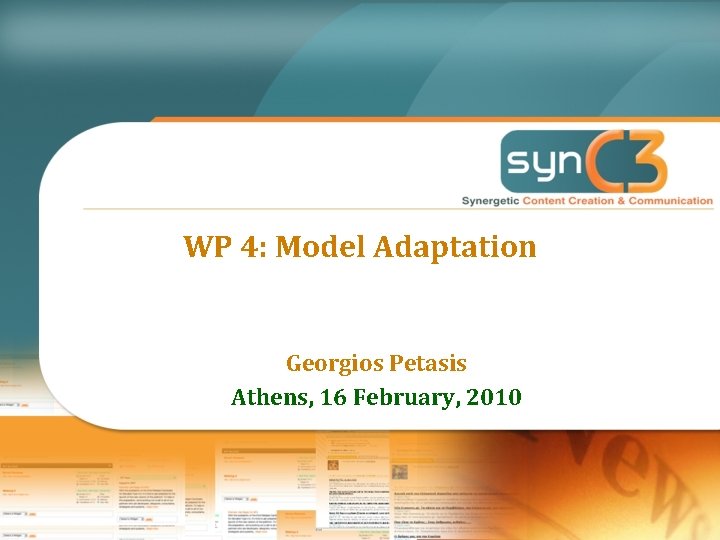
WP 4: Model Adaptation Georgios Petasis Athens, 16 February, 2010
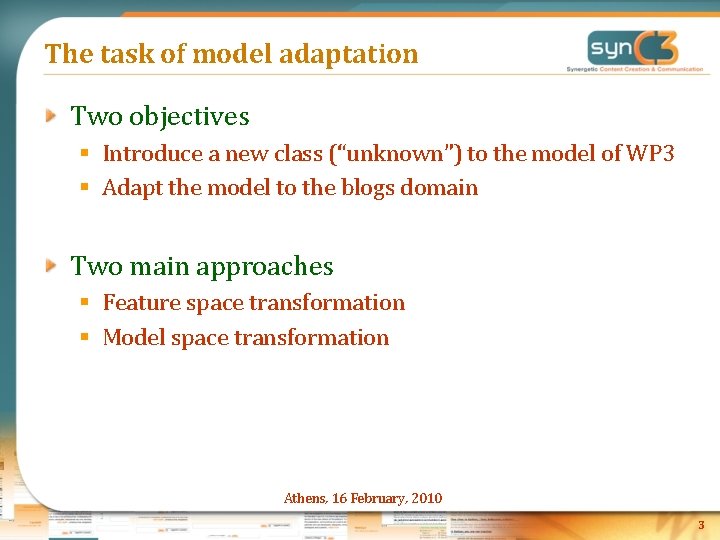
The task of model adaptation Two objectives § Introduce a new class (“unknown”) to the model of WP 3 § Adapt the model to the blogs domain Two main approaches § Feature space transformation § Model space transformation Athens, 16 February, 2010 3
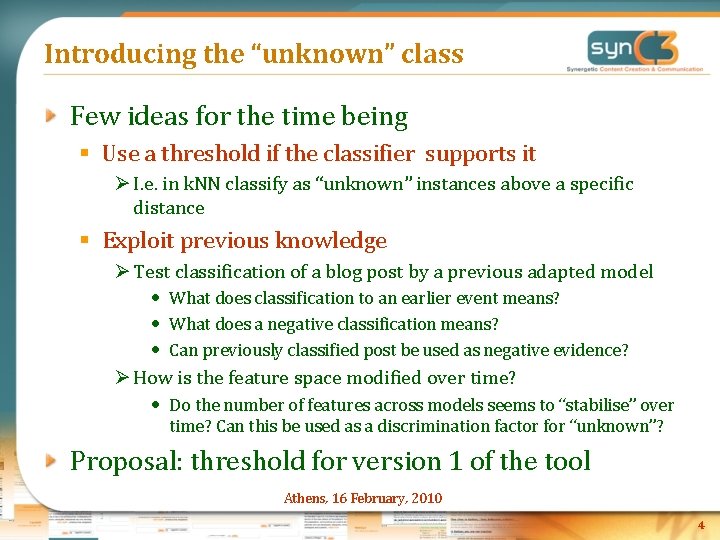
Introducing the “unknown” class Few ideas for the time being § Use a threshold if the classifier supports it Ø I. e. in k. NN classify as “unknown” instances above a specific distance § Exploit previous knowledge Ø Test classification of a blog post by a previous adapted model • What does classification to an earlier event means? • What does a negative classification means? • Can previously classified post be used as negative evidence? Ø How is the feature space modified over time? • Do the number of features across models seems to “stabilise” over time? Can this be used as a discrimination factor for “unknown”? Proposal: threshold for version 1 of the tool Athens, 16 February, 2010 4
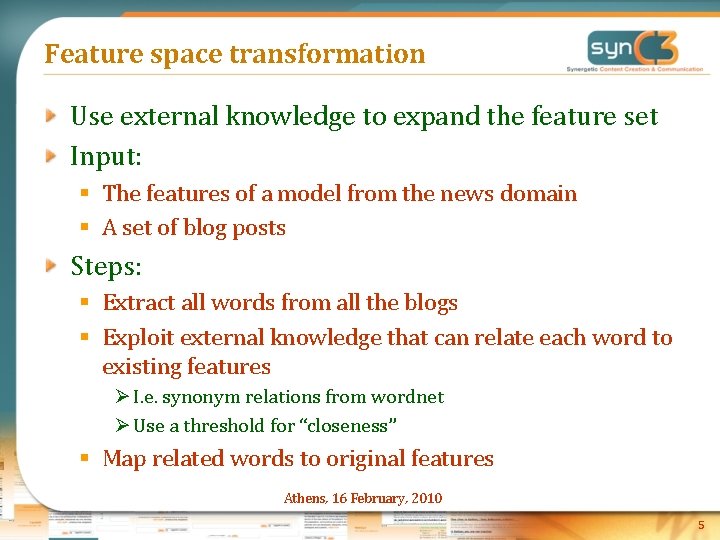
Feature space transformation Use external knowledge to expand the feature set Input: § The features of a model from the news domain § A set of blog posts Steps: § Extract all words from all the blogs § Exploit external knowledge that can relate each word to existing features Ø I. e. synonym relations from wordnet Ø Use a threshold for “closeness” § Map related words to original features Athens, 16 February, 2010 5
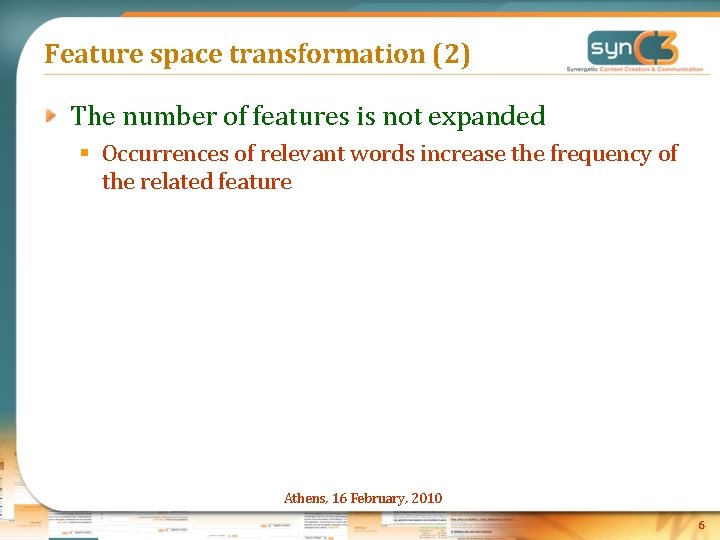
Feature space transformation (2) The number of features is not expanded § Occurrences of relevant words increase the frequency of the related feature Athens, 16 February, 2010 6
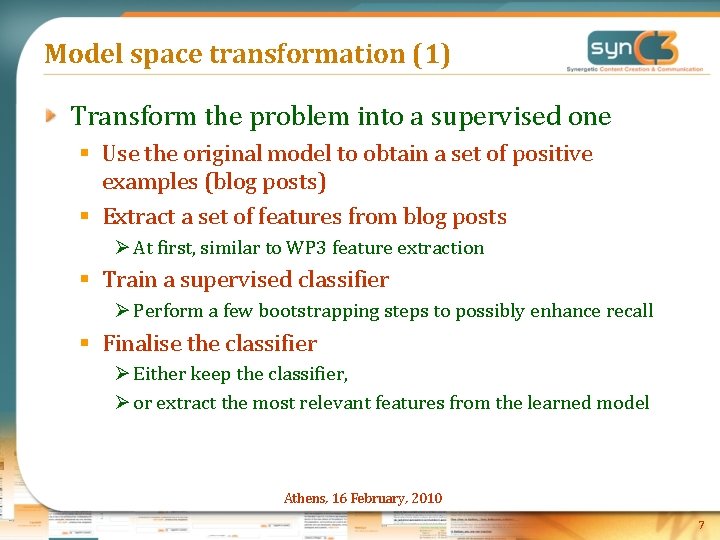
Model space transformation (1) Transform the problem into a supervised one § Use the original model to obtain a set of positive examples (blog posts) § Extract a set of features from blog posts Ø At first, similar to WP 3 feature extraction § Train a supervised classifier Ø Perform a few bootstrapping steps to possibly enhance recall § Finalise the classifier Ø Either keep the classifier, Ø or extract the most relevant features from the learned model Athens, 16 February, 2010 7
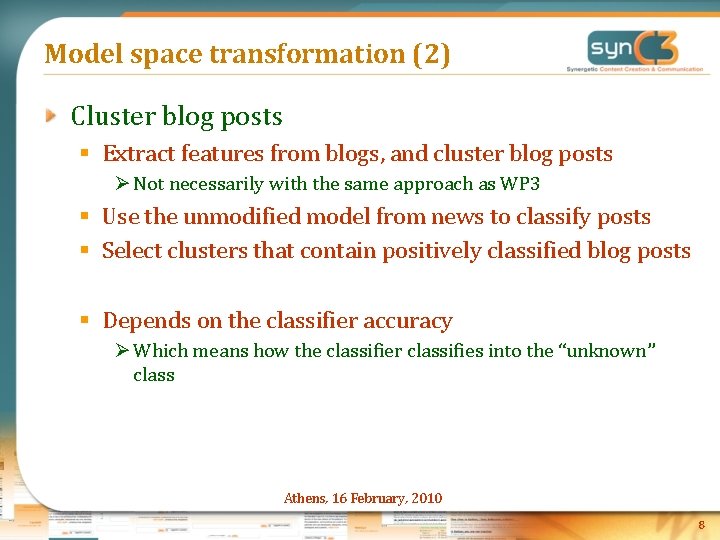
Model space transformation (2) Cluster blog posts § Extract features from blogs, and cluster blog posts Ø Not necessarily with the same approach as WP 3 § Use the unmodified model from news to classify posts § Select clusters that contain positively classified blog posts § Depends on the classifier accuracy Ø Which means how the classifier classifies into the “unknown” class Athens, 16 February, 2010 8