Why randomize Experiments Main role of randomization Assign
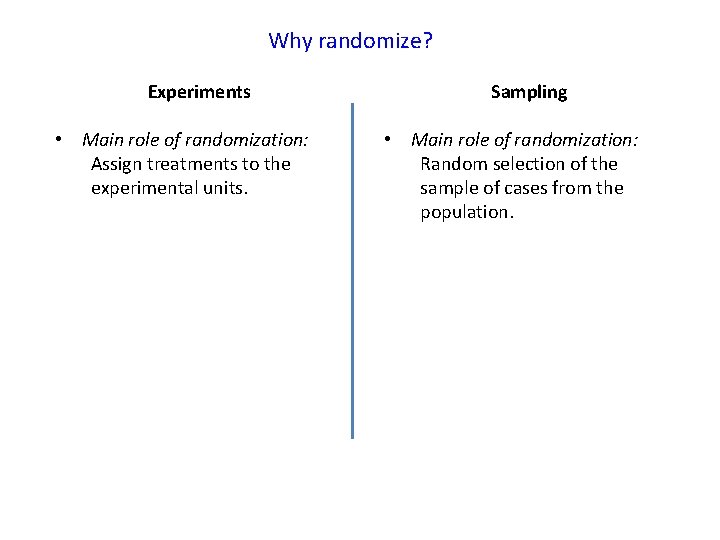
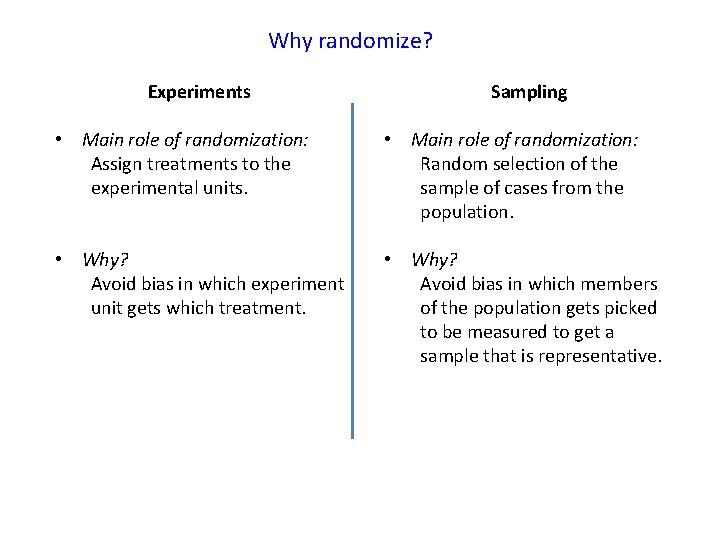
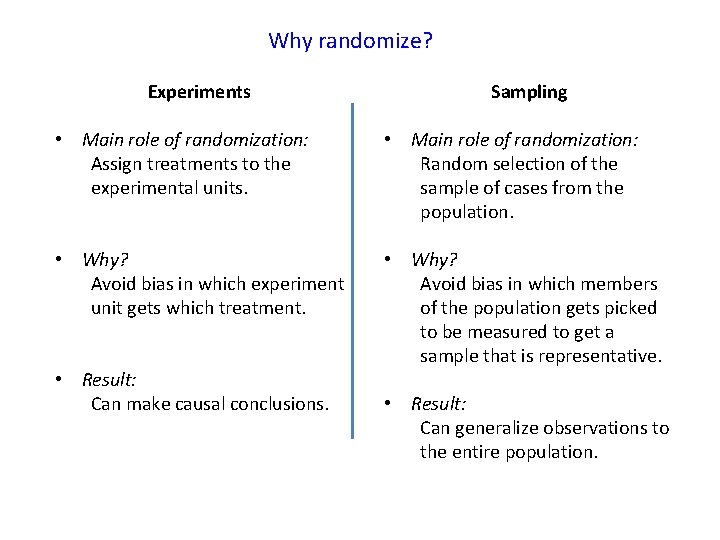
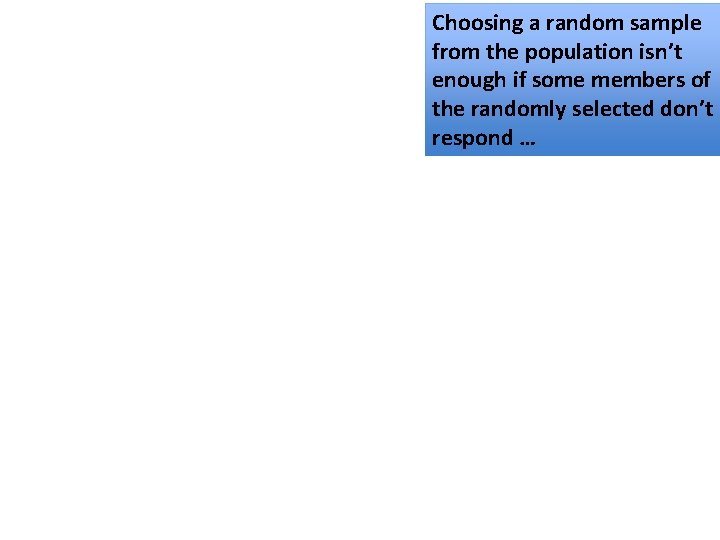
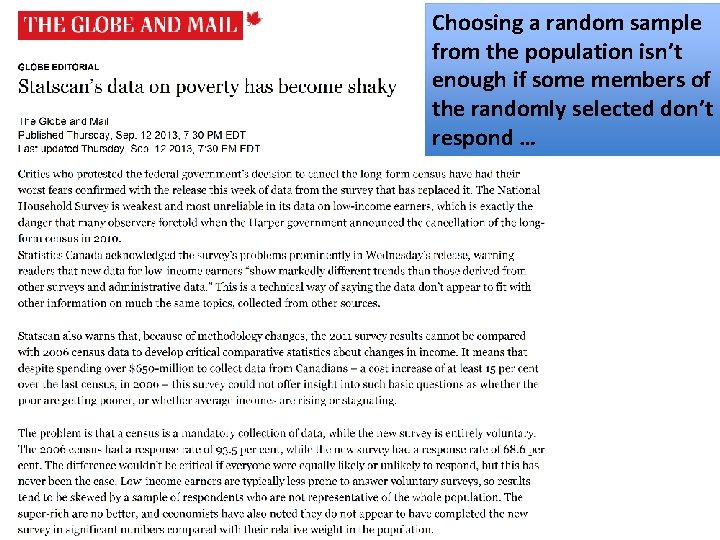
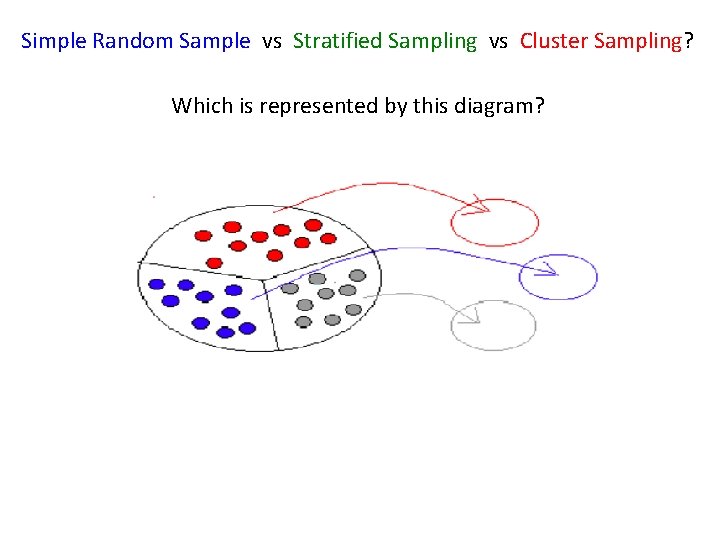
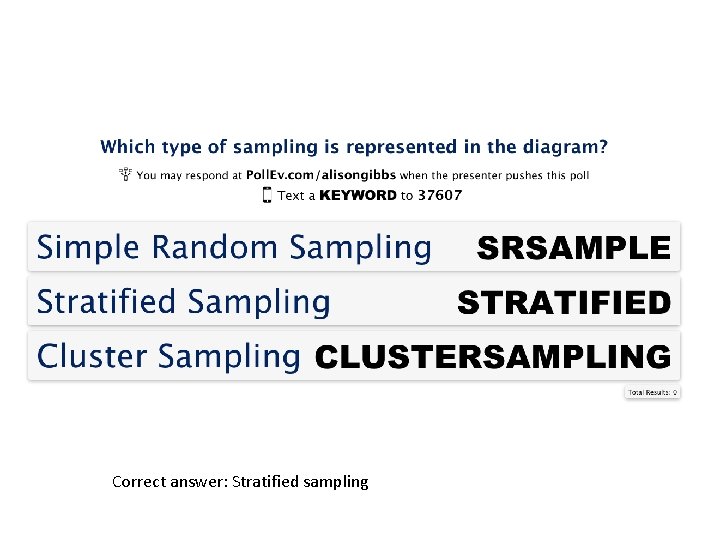
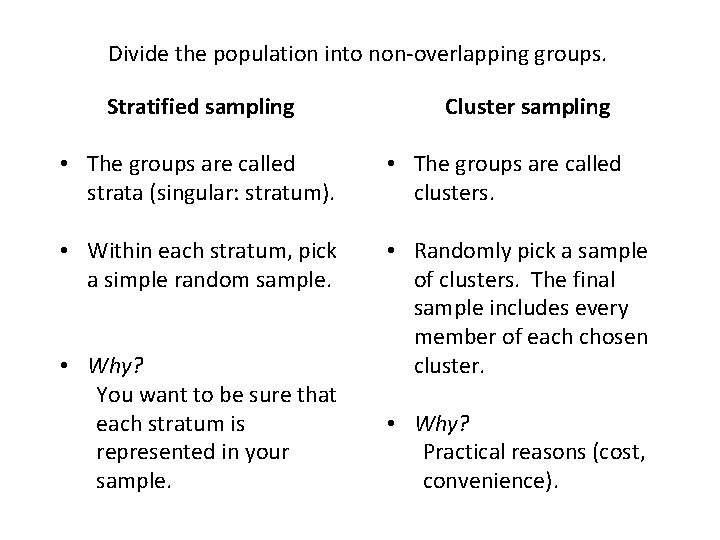
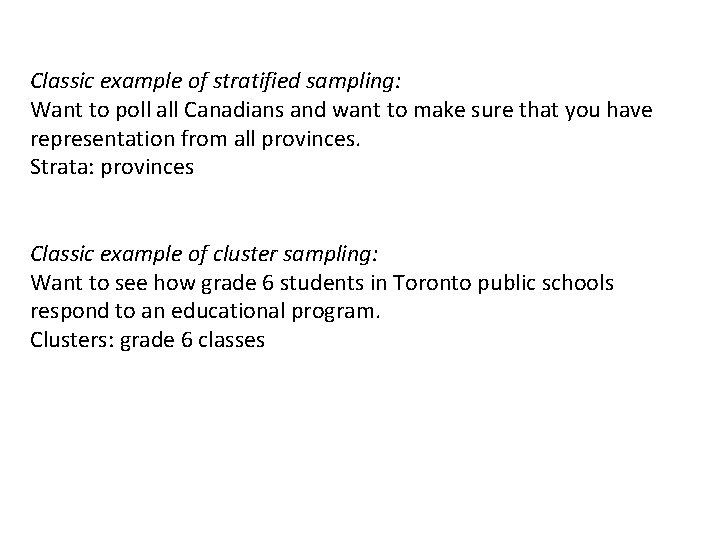
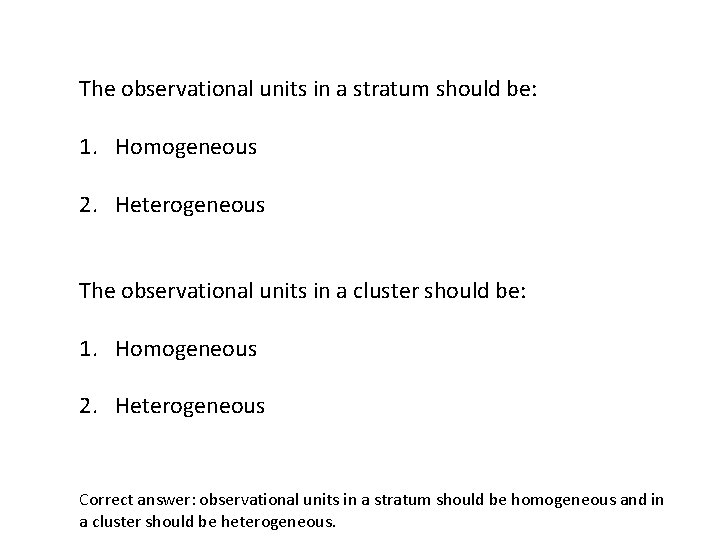
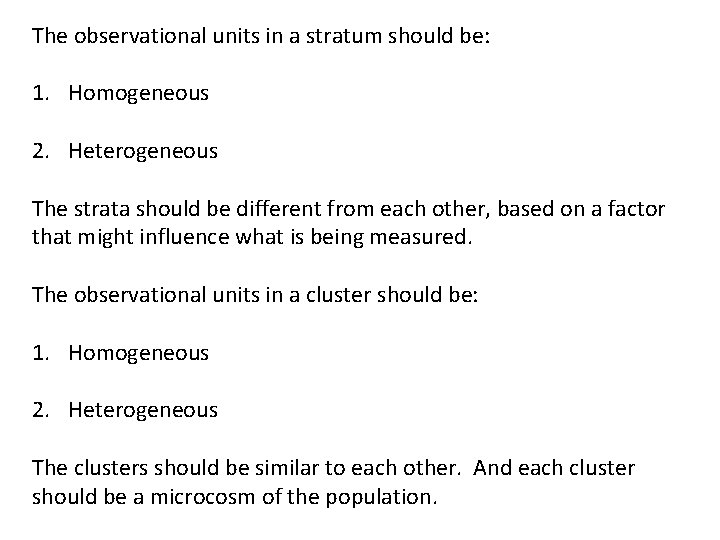
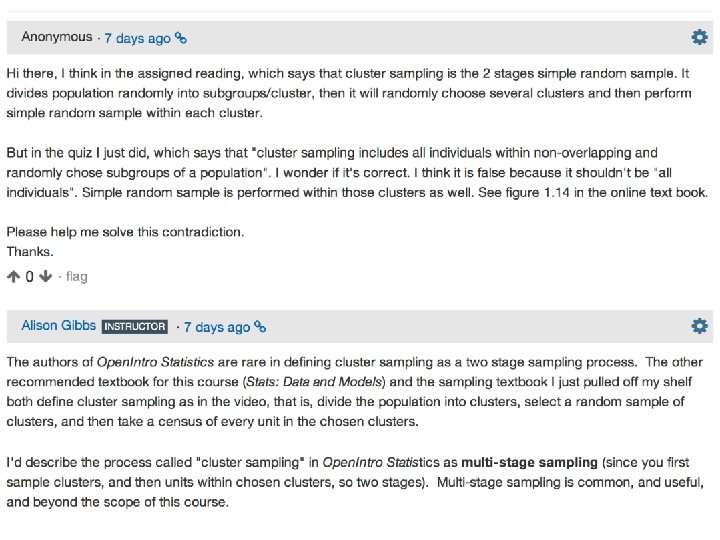
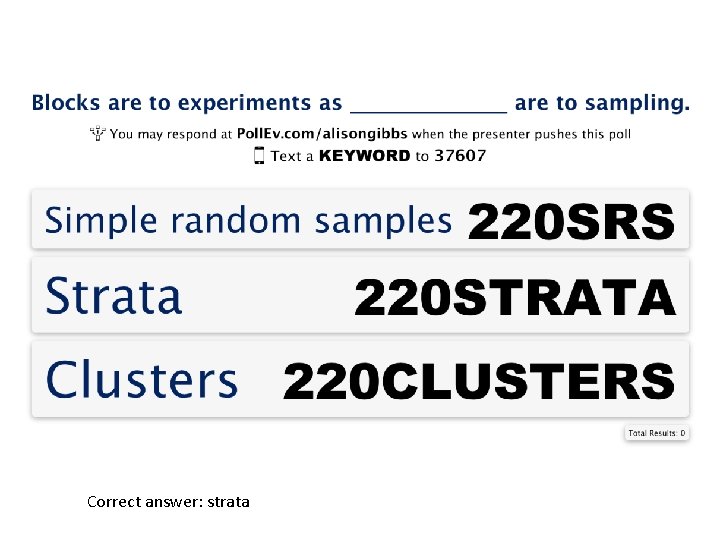
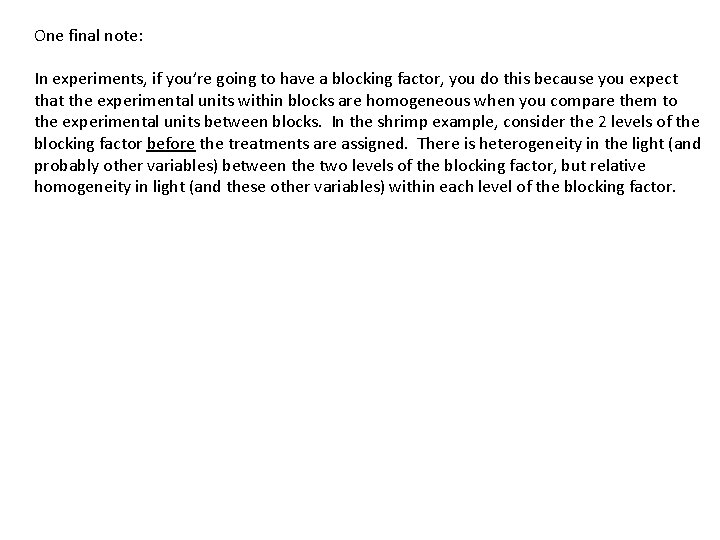
- Slides: 14
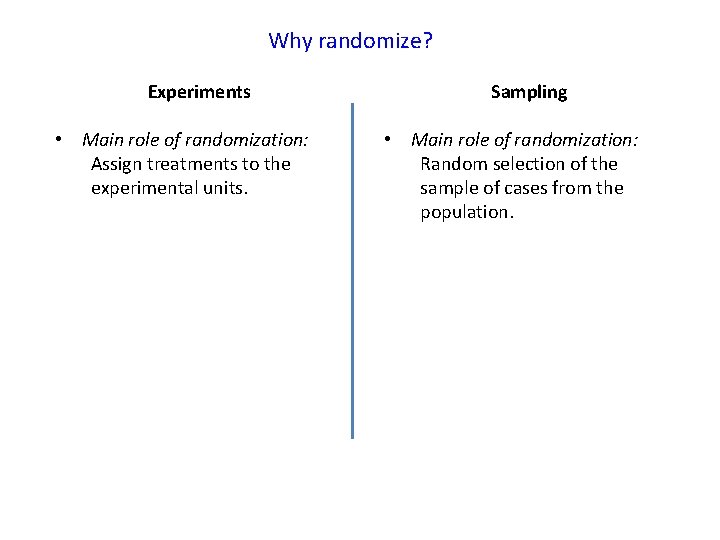
Why randomize? Experiments • Main role of randomization: Assign treatments to the experimental units. Sampling • Main role of randomization: Random selection of the sample of cases from the population.
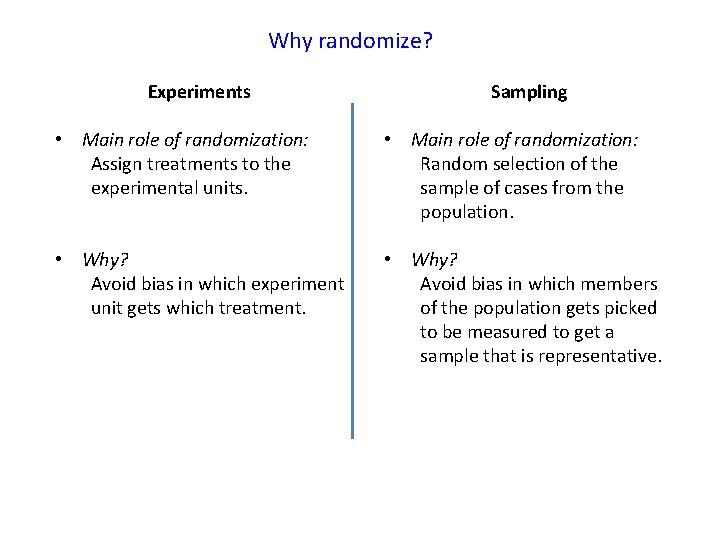
Why randomize? Experiments Sampling • Main role of randomization: Assign treatments to the experimental units. • Main role of randomization: Random selection of the sample of cases from the population. • Why? Avoid bias in which experiment unit gets which treatment. • Why? Avoid bias in which members of the population gets picked to be measured to get a sample that is representative.
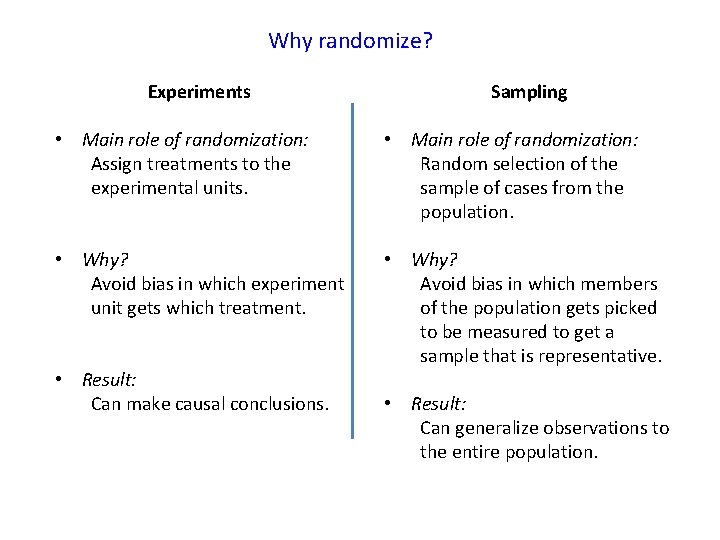
Why randomize? Experiments Sampling • Main role of randomization: Assign treatments to the experimental units. • Main role of randomization: Random selection of the sample of cases from the population. • Why? Avoid bias in which experiment unit gets which treatment. • Why? Avoid bias in which members of the population gets picked to be measured to get a sample that is representative. • Result: Can make causal conclusions. • Result: Can generalize observations to the entire population.
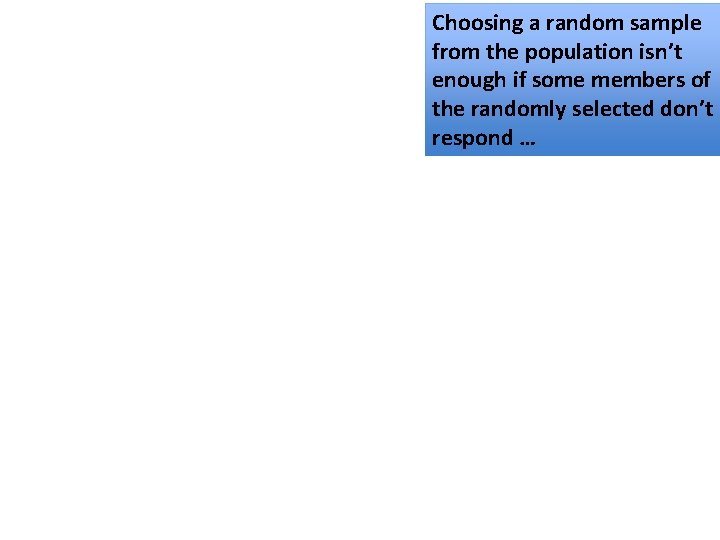
Choosing a random sample from the population isn’t enough if some members of the randomly selected don’t respond …
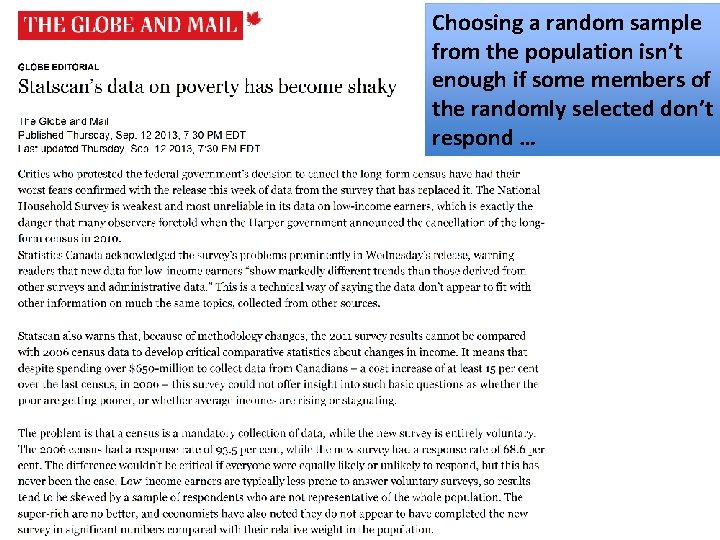
Choosing a random sample from the population isn’t enough if some members of the randomly selected don’t respond …
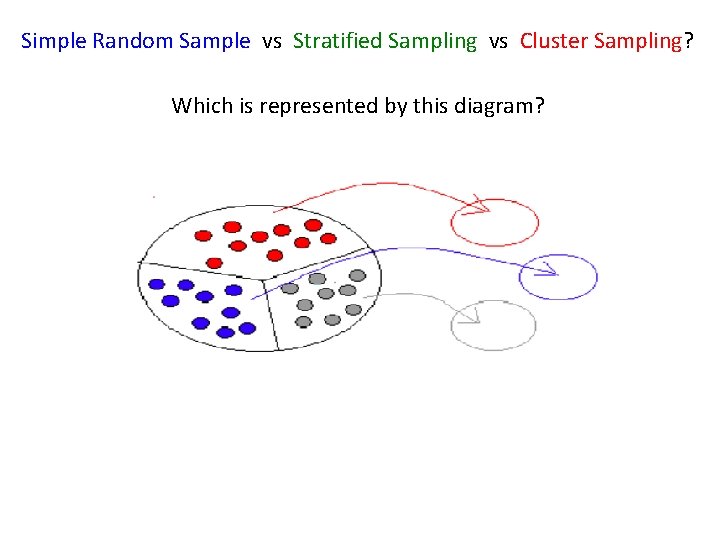
Simple Random Sample vs Stratified Sampling vs Cluster Sampling? Which is represented by this diagram?
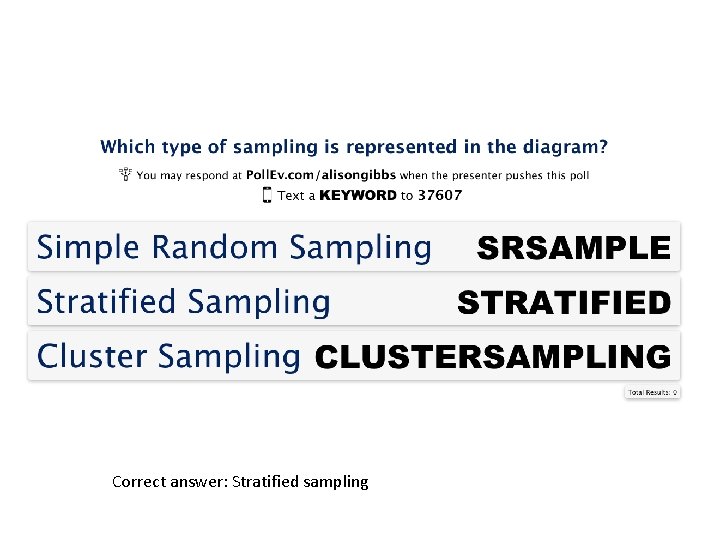
Correct answer: Stratified sampling
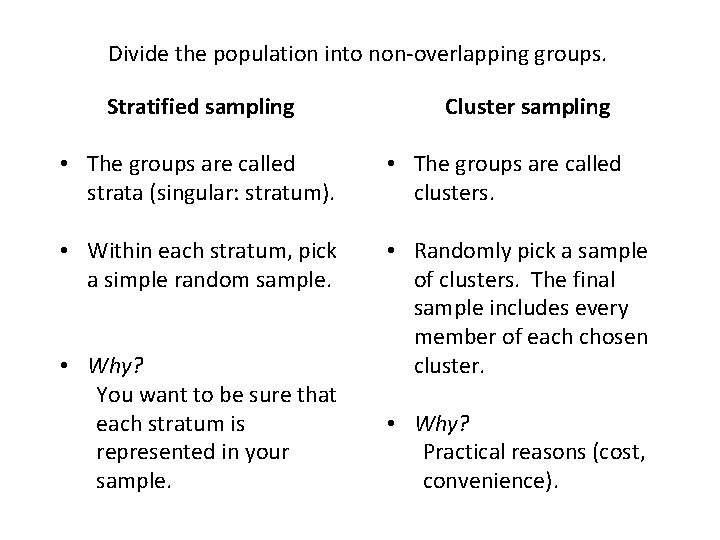
Divide the population into non-overlapping groups. Stratified sampling Cluster sampling • The groups are called strata (singular: stratum). • The groups are called clusters. • Within each stratum, pick a simple random sample. • Randomly pick a sample of clusters. The final sample includes every member of each chosen cluster. • Why? You want to be sure that each stratum is represented in your sample. • Why? Practical reasons (cost, convenience).
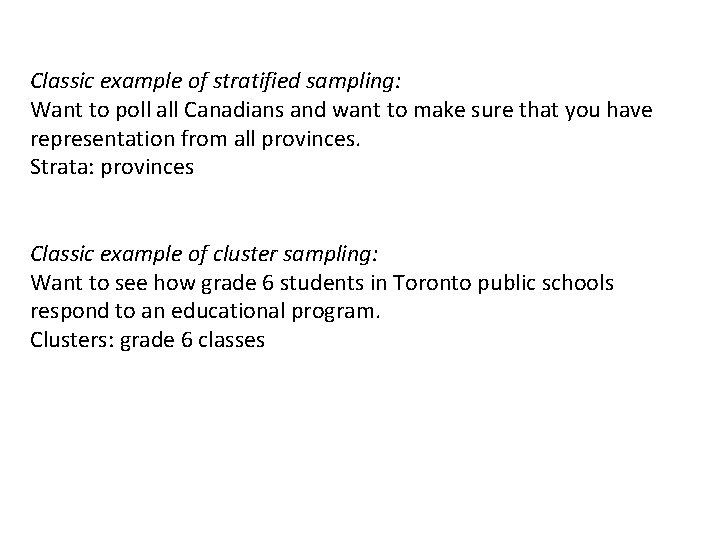
Classic example of stratified sampling: Want to poll all Canadians and want to make sure that you have representation from all provinces. Strata: provinces Classic example of cluster sampling: Want to see how grade 6 students in Toronto public schools respond to an educational program. Clusters: grade 6 classes
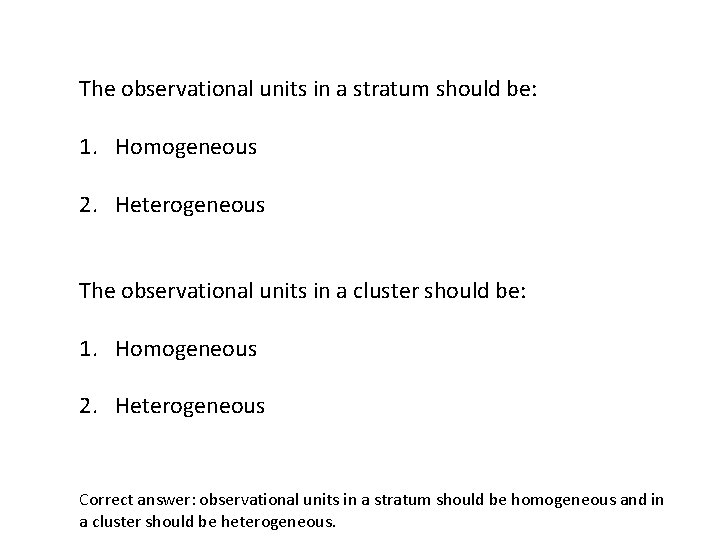
The observational units in a stratum should be: 1. Homogeneous 2. Heterogeneous The observational units in a cluster should be: 1. Homogeneous 2. Heterogeneous Correct answer: observational units in a stratum should be homogeneous and in a cluster should be heterogeneous.
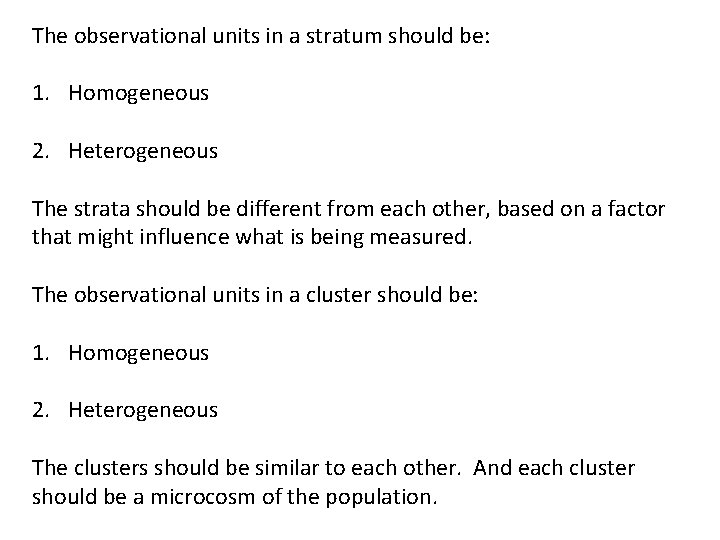
The observational units in a stratum should be: 1. Homogeneous 2. Heterogeneous The strata should be different from each other, based on a factor that might influence what is being measured. The observational units in a cluster should be: 1. Homogeneous 2. Heterogeneous The clusters should be similar to each other. And each cluster should be a microcosm of the population.
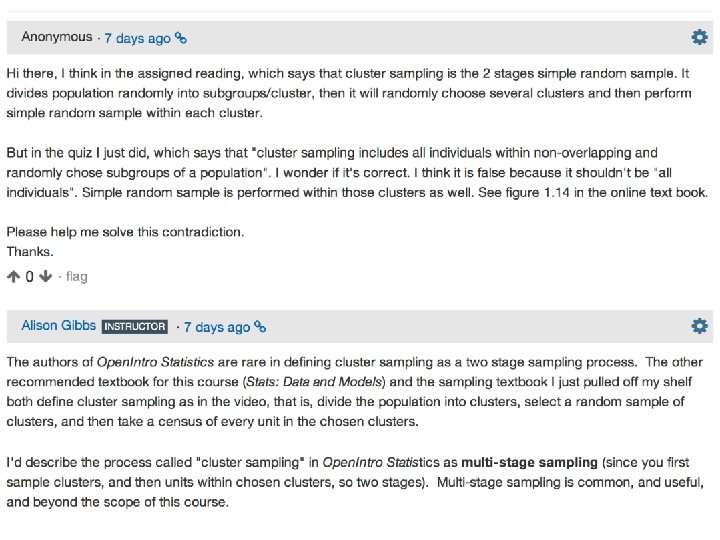
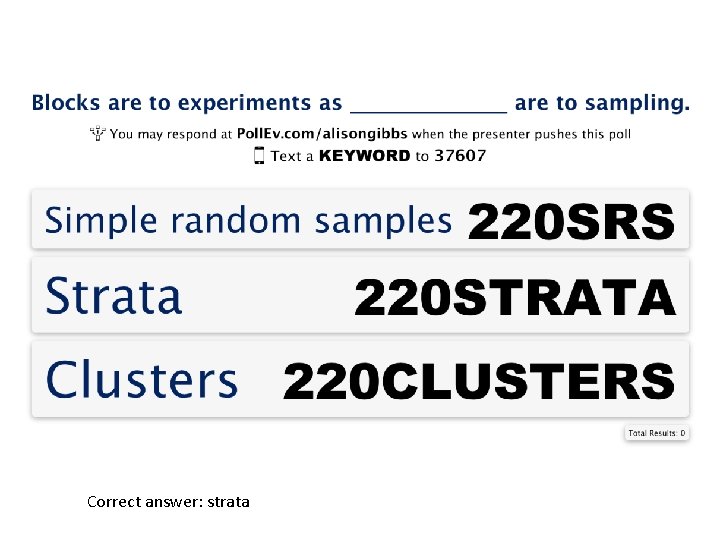
Correct answer: strata
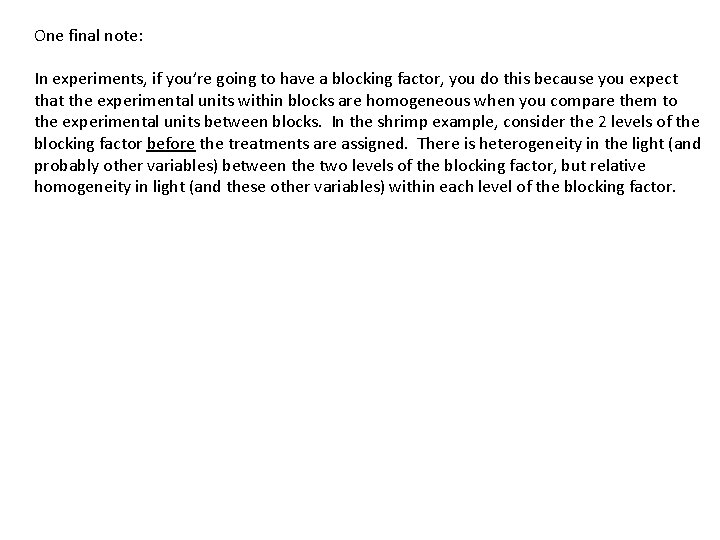
One final note: In experiments, if you’re going to have a blocking factor, you do this because you expect that the experimental units within blocks are homogeneous when you compare them to the experimental units between blocks. In the shrimp example, consider the 2 levels of the blocking factor before the treatments are assigned. There is heterogeneity in the light (and probably other variables) between the two levels of the blocking factor, but relative homogeneity in light (and these other variables) within each level of the blocking factor.
Qualtrics block randomization
Randomize timer
Salnda
Why why why why
Why is “replication key” psychology experiments?
Why are controlled experiments sometimes impossible
Interactive voice response system randomization
Stratified randomization
Whats a redcap
Poonam naik
Stratified randomization
Block randomization example
Randomization
Randomization based on remainder
Redcap randomization module