Sparse Multitask Learning for Detecting Influential Nodes in
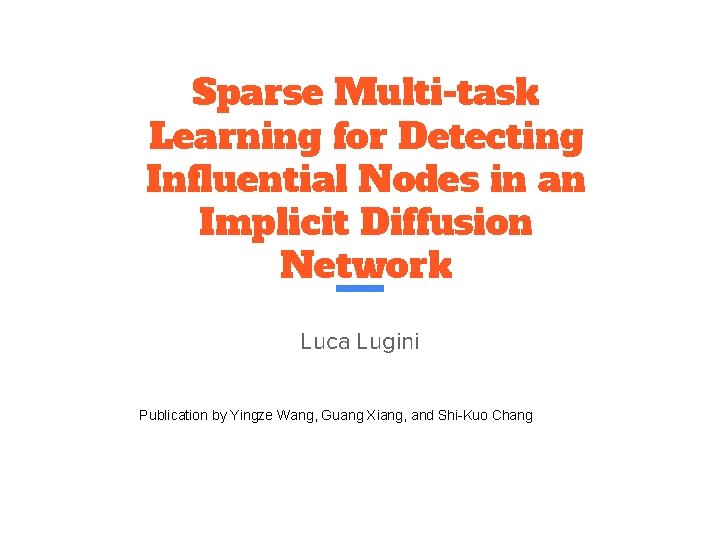
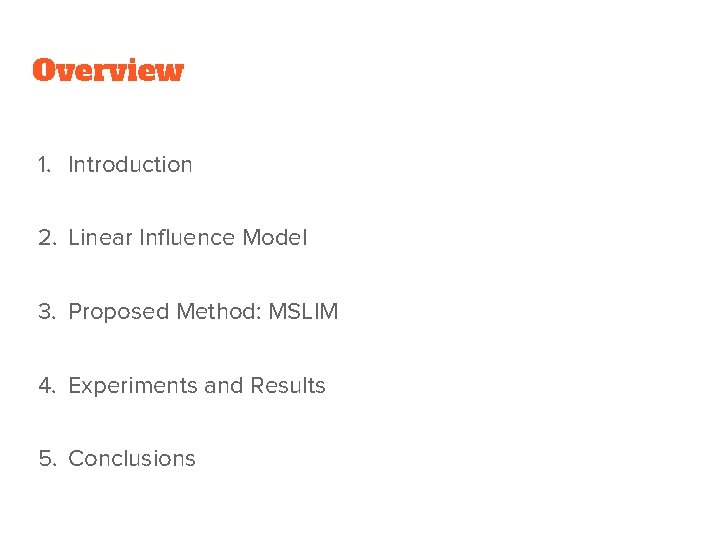
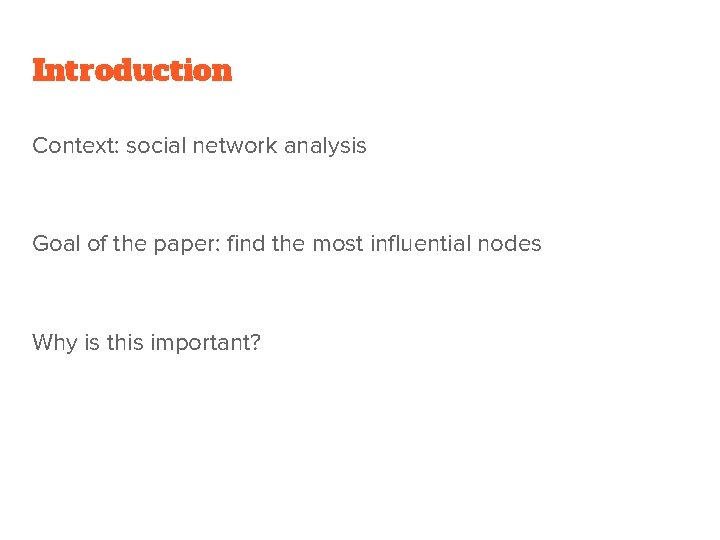
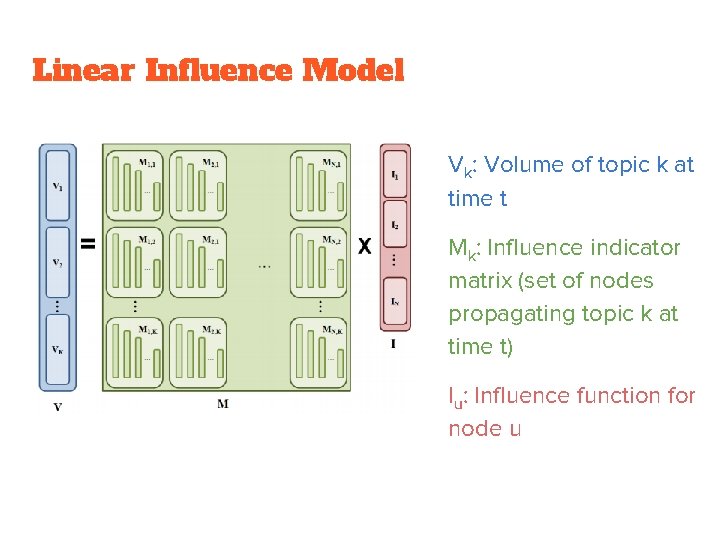
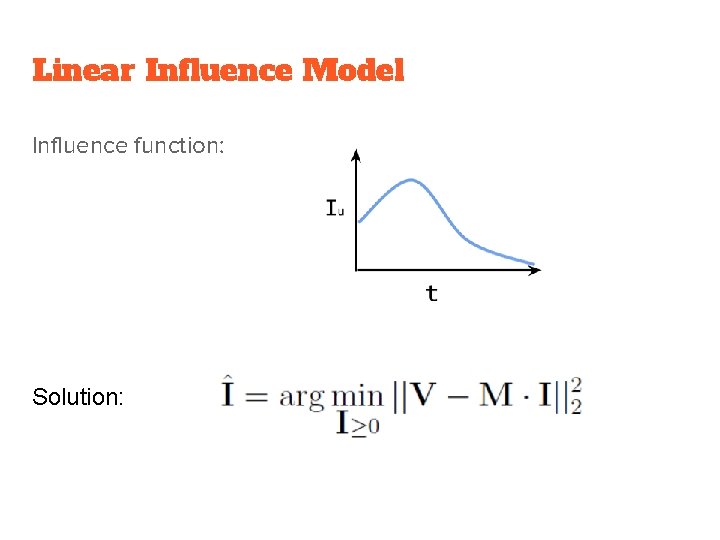
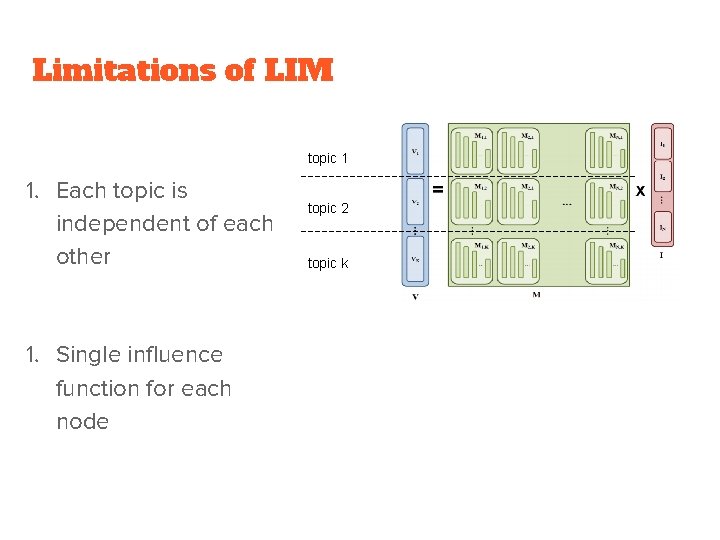
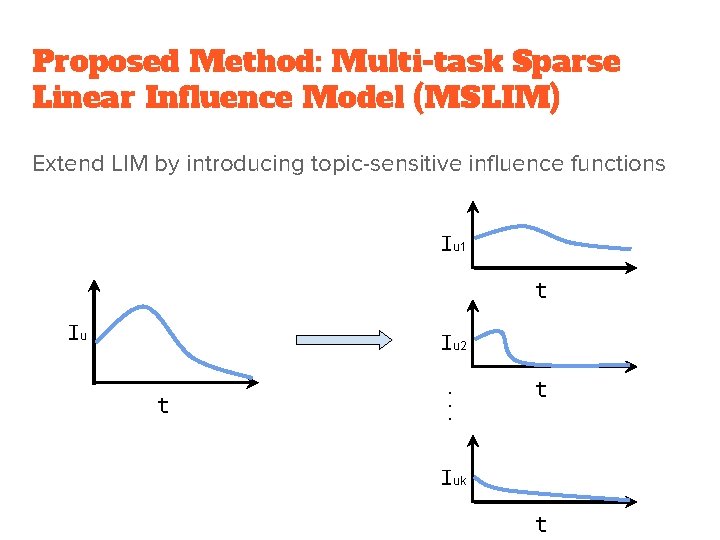
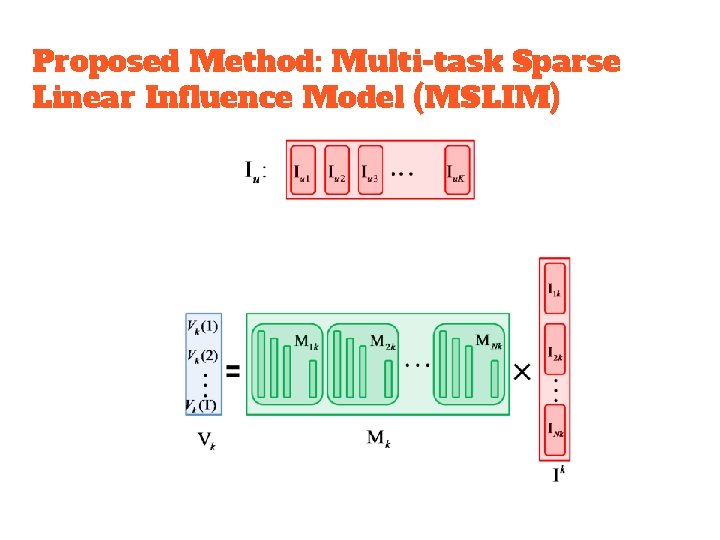
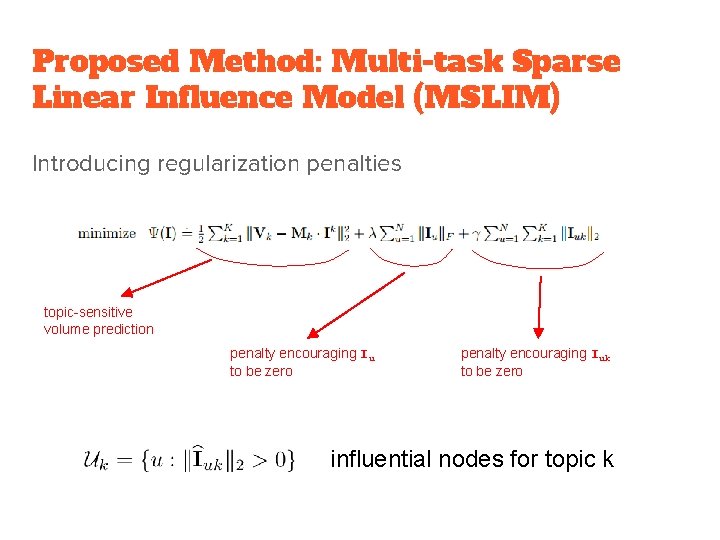
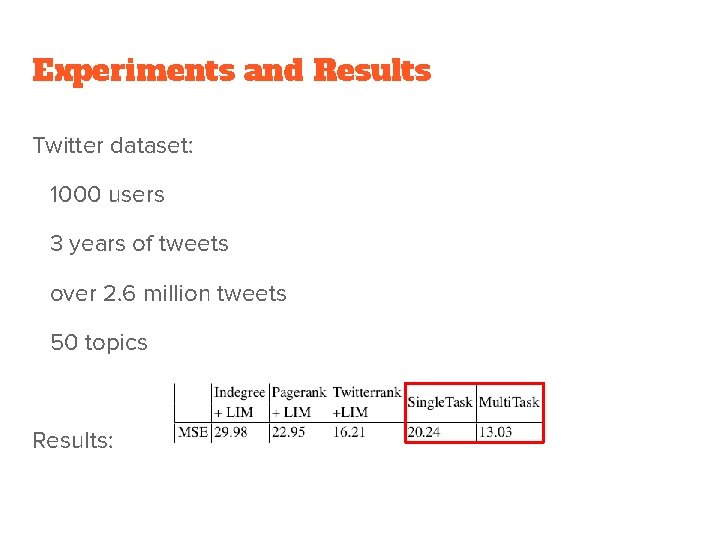
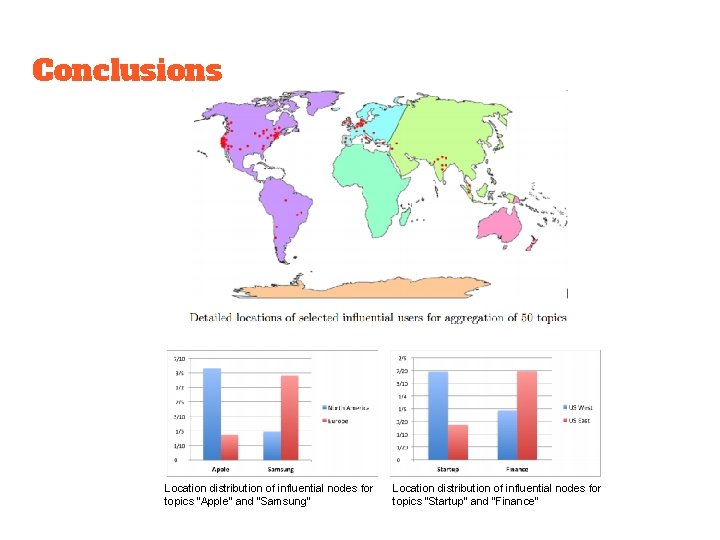
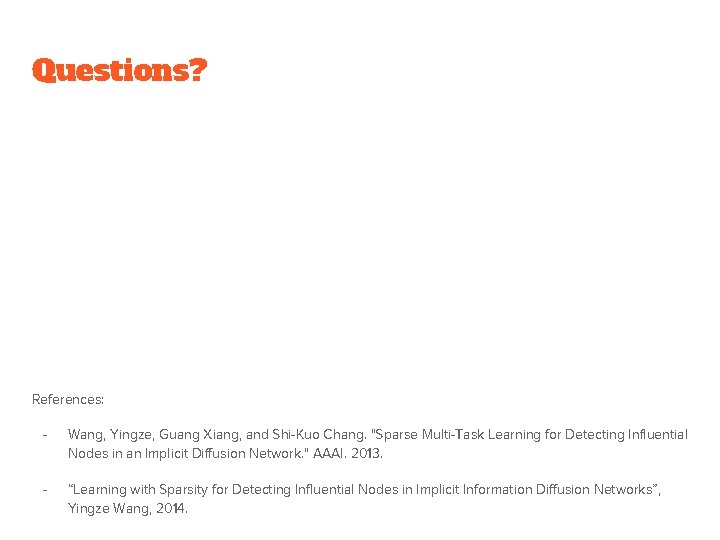
- Slides: 12
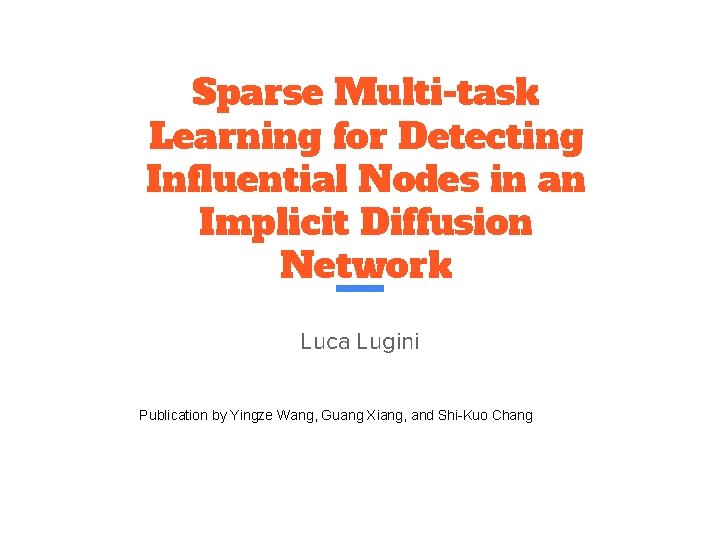
Sparse Multi-task Learning for Detecting Influential Nodes in an Implicit Diffusion Network Luca Lugini Publication by Yingze Wang, Guang Xiang, and Shi-Kuo Chang
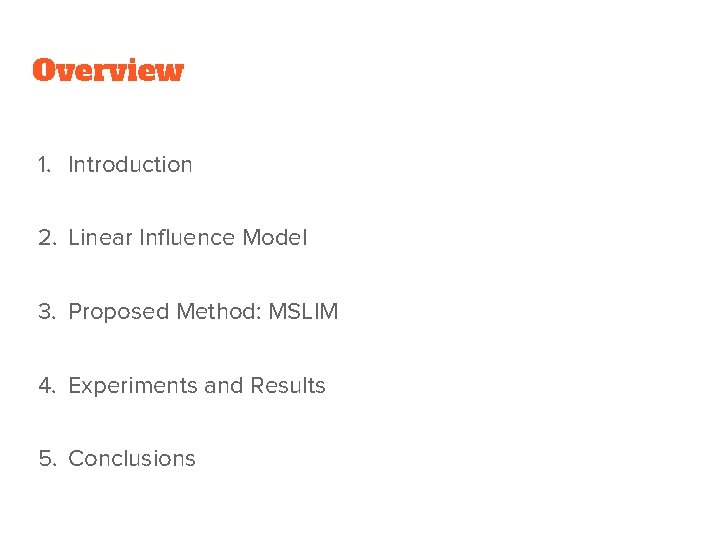
Overview 1. Introduction 2. Linear Influence Model 3. Proposed Method: MSLIM 4. Experiments and Results 5. Conclusions
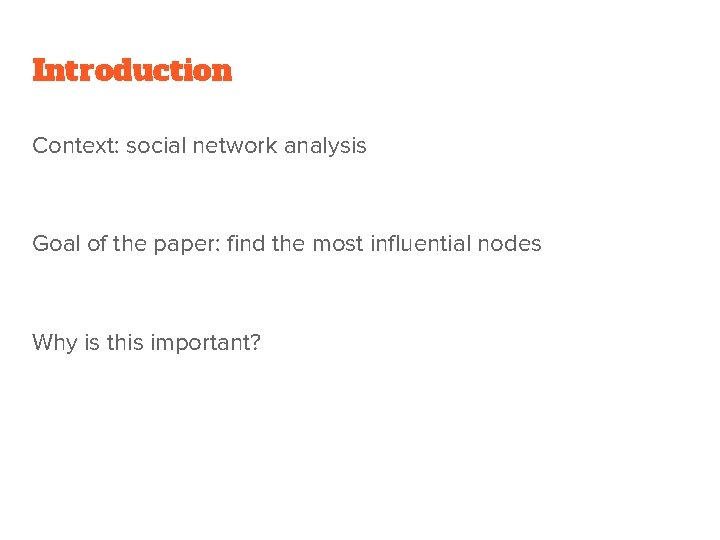
Introduction Context: social network analysis Goal of the paper: find the most influential nodes Why is this important?
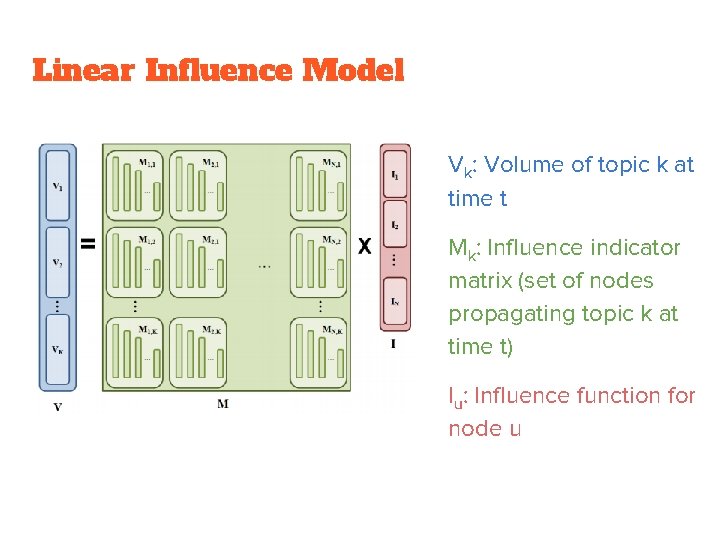
Linear Influence Model Vk: Volume of topic k at time t Mk: Influence indicator matrix (set of nodes propagating topic k at time t) Iu: Influence function for node u
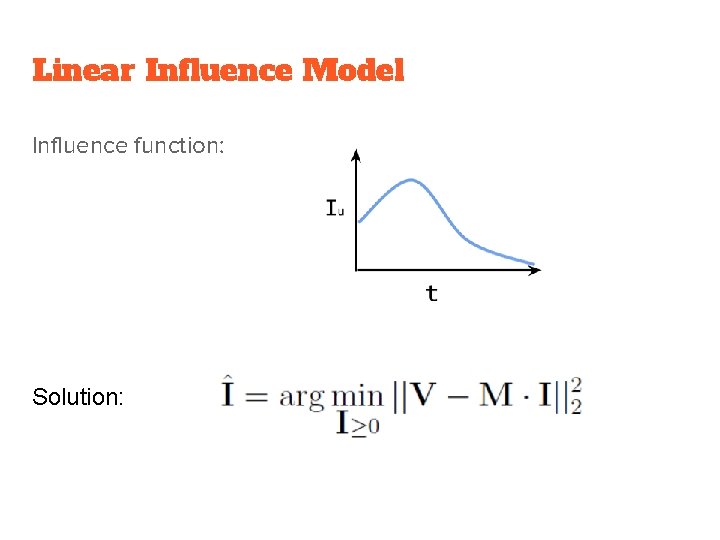
Linear Influence Model Influence function: Solution:
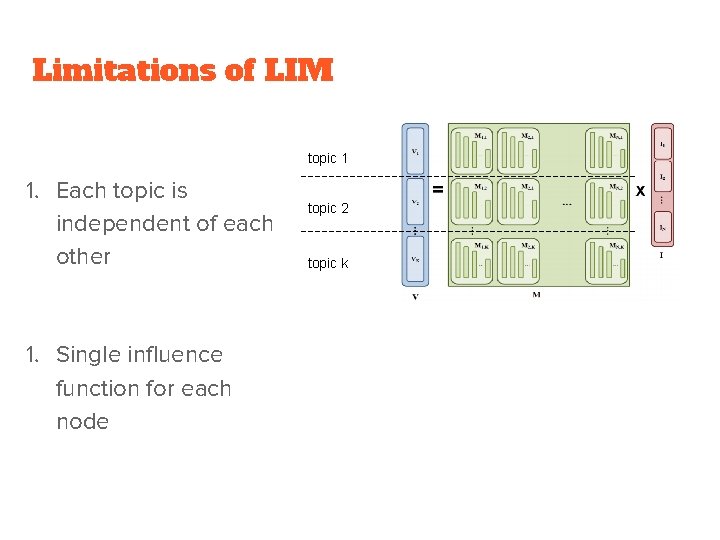
Limitations of LIM topic 1 1. Each topic is independent of each other 1. Single influence function for each node topic 2 topic k
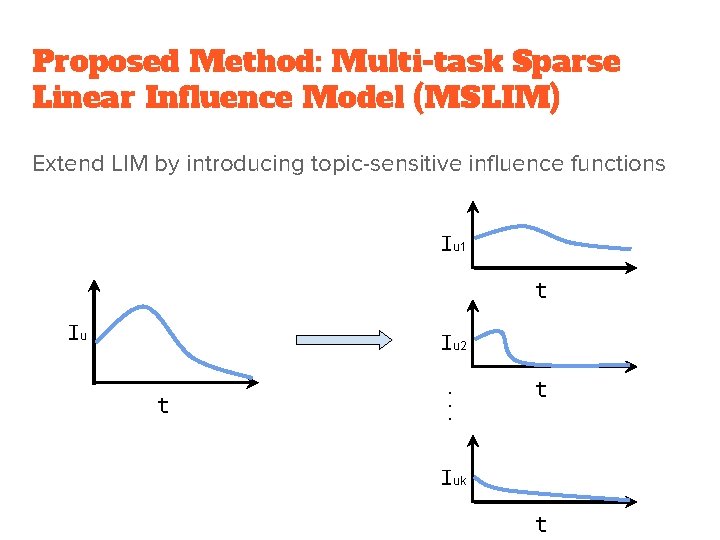
Proposed Method: Multi-task Sparse Linear Influence Model (MSLIM) Extend LIM by introducing topic-sensitive influence functions Iu 1 t Iu t . . . Iu 2 t Iuk t
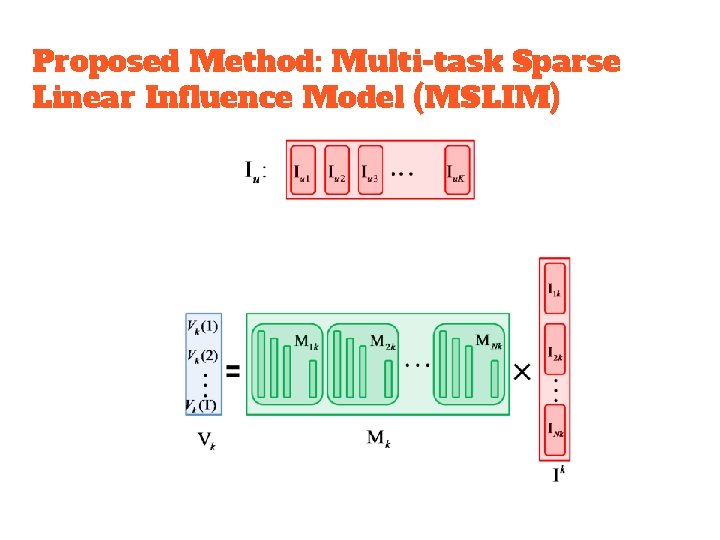
Proposed Method: Multi-task Sparse Linear Influence Model (MSLIM)
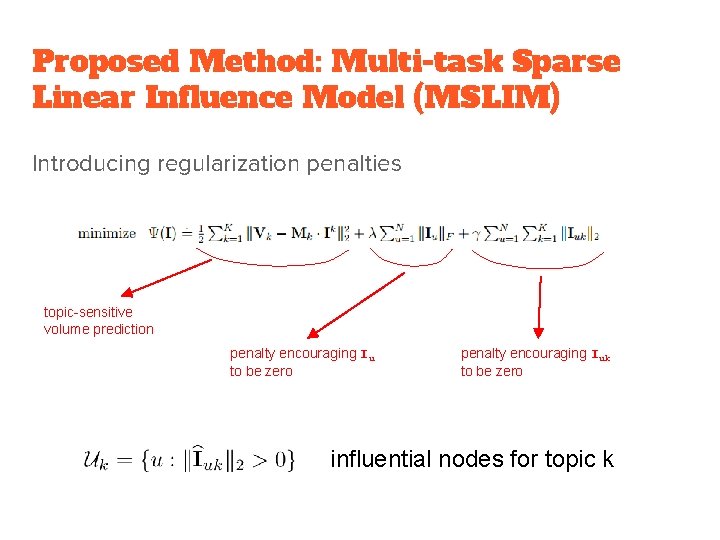
Proposed Method: Multi-task Sparse Linear Influence Model (MSLIM) Introducing regularization penalties topic-sensitive volume prediction penalty encouraging Iu to be zero penalty encouraging Iuk to be zero influential nodes for topic k
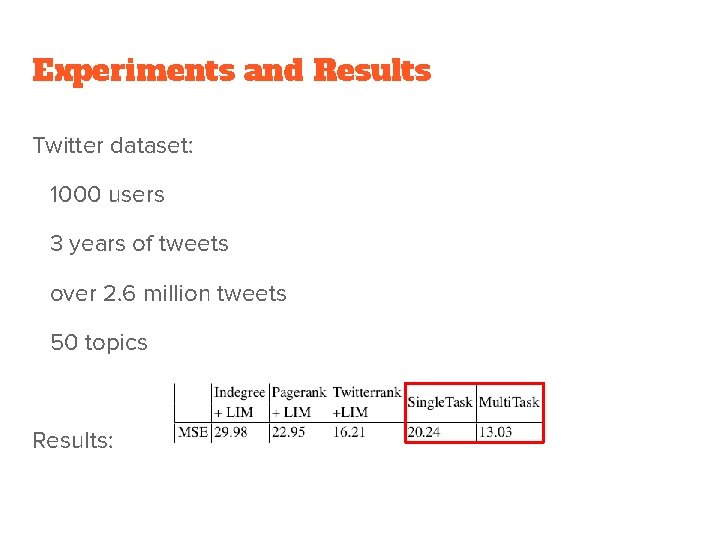
Experiments and Results Twitter dataset: 1000 users 3 years of tweets over 2. 6 million tweets 50 topics Results:
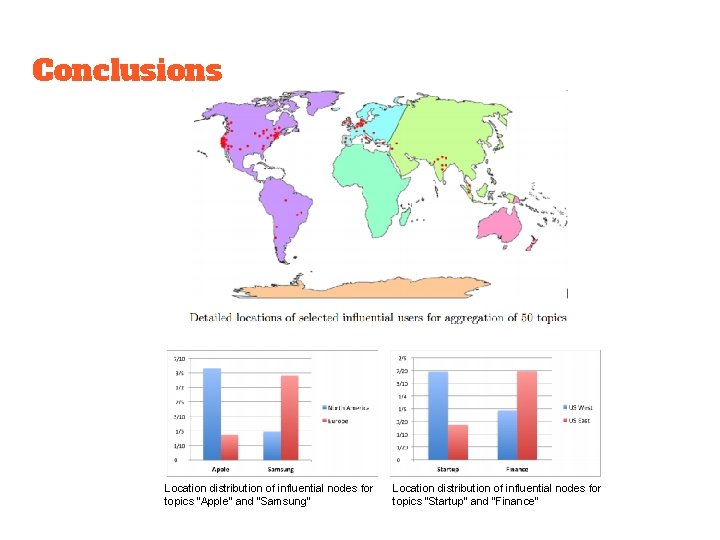
Conclusions Location distribution of influential nodes for topics “Apple” and “Samsung” Location distribution of influential nodes for topics “Startup” and “Finance”
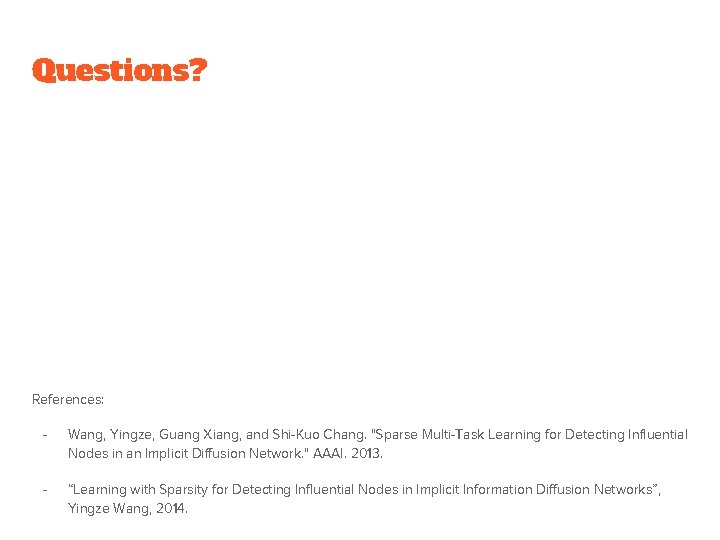
Questions? References: - Wang, Yingze, Guang Xiang, and Shi-Kuo Chang. "Sparse Multi-Task Learning for Detecting Influential Nodes in an Implicit Diffusion Network. " AAAI. 2013. - “Learning with Sparsity for Detecting Influential Nodes in Implicit Information Diffusion Networks”, Yingze Wang, 2014.
European physical characteristics
Outliers and influential points
Instrumental power examples
Christian louboutin louis benech
Sniffer for detecting lost mobiles
Detecting evolutionary forces in language change
Detecting golden ticket attack
How do fraud symptoms help in detecting fraud
Havex
Cuadro comparativo e-learning b-learning m-learning
Sparse conditional constant propagation
Suatu array dikatakan lower triangular jika
Sparse matrix operator kernel emissions