Simple Lectin Identification Using a Chemical Tongue Lucienne
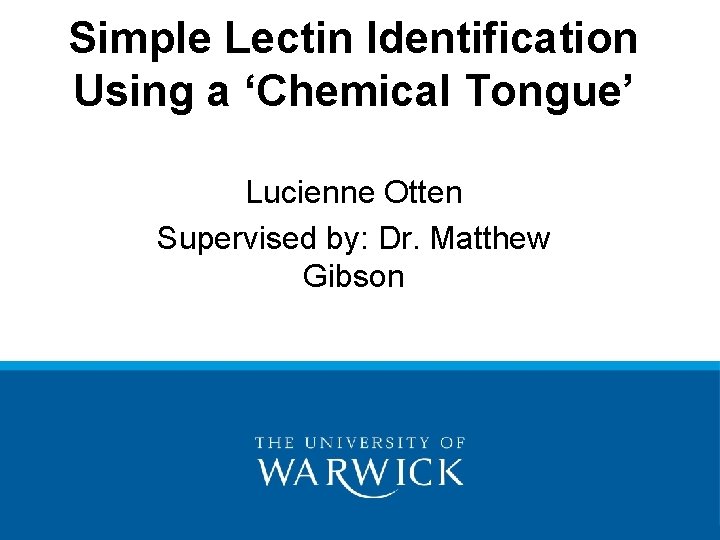
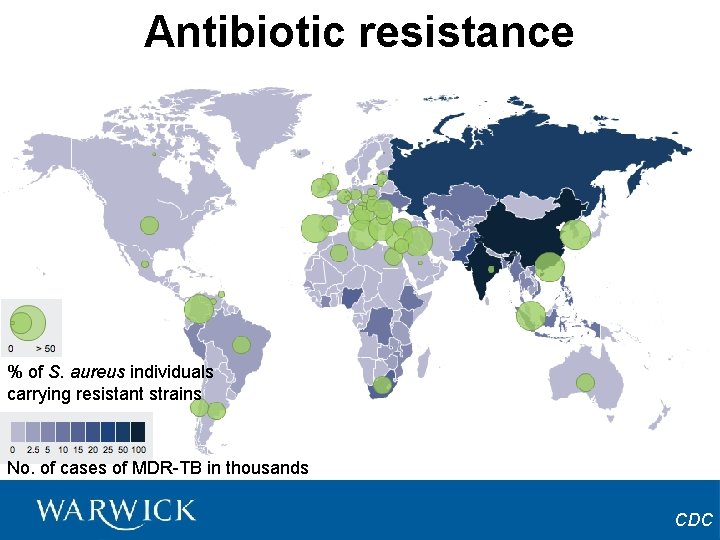
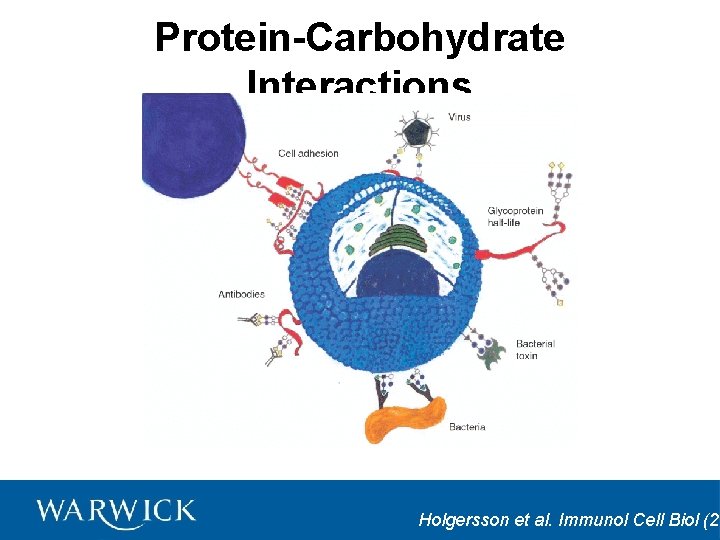
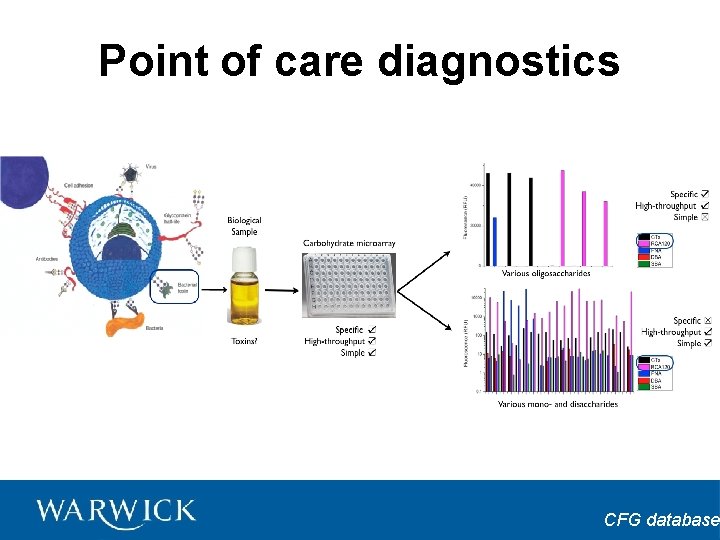
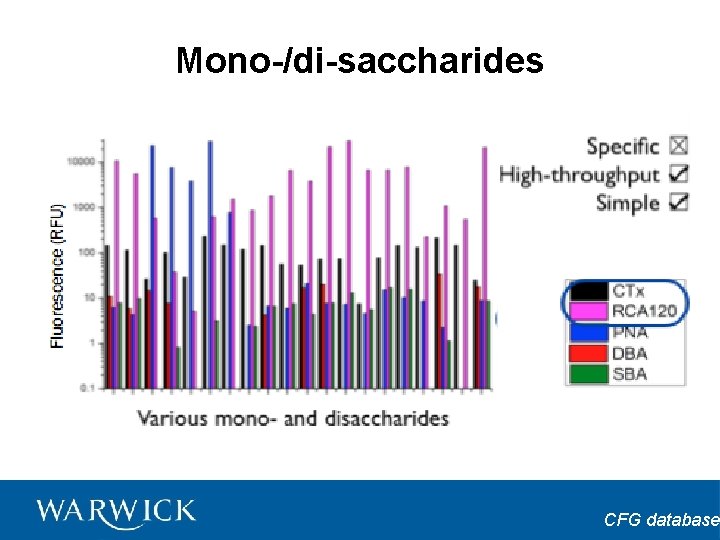
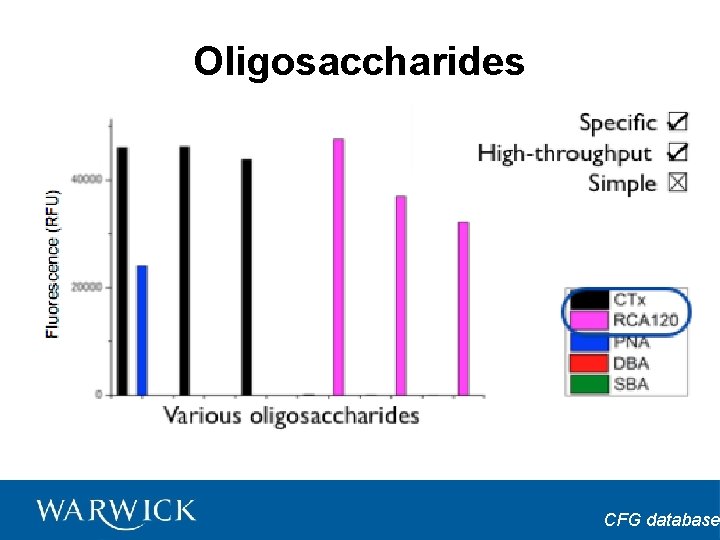
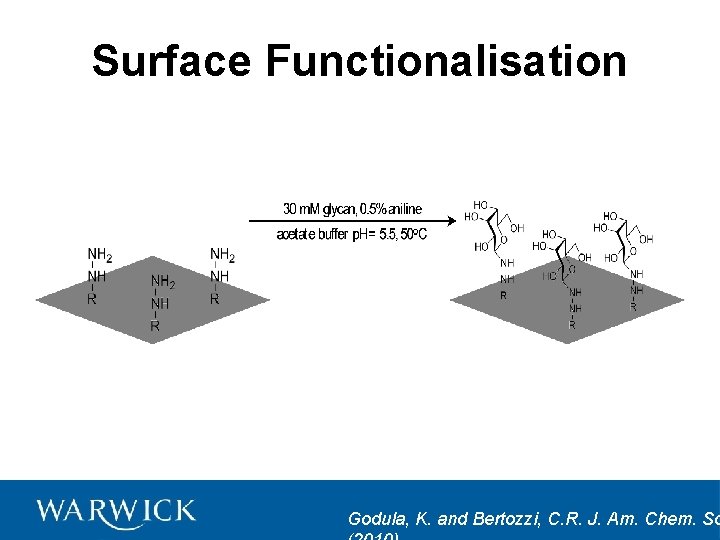
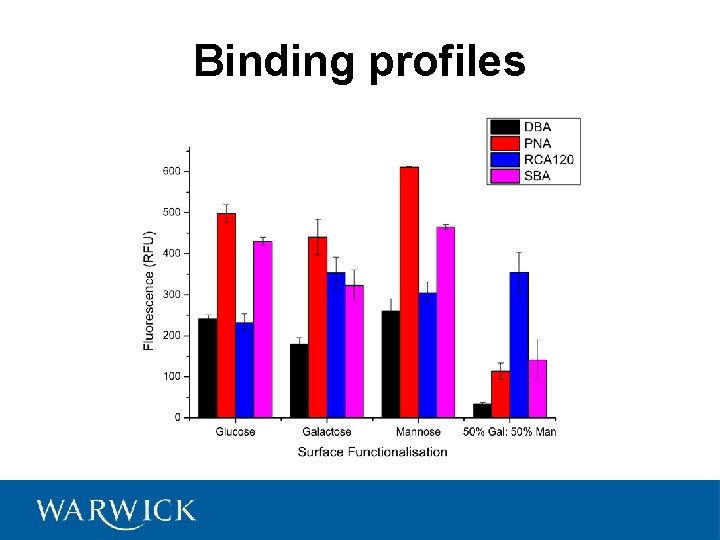
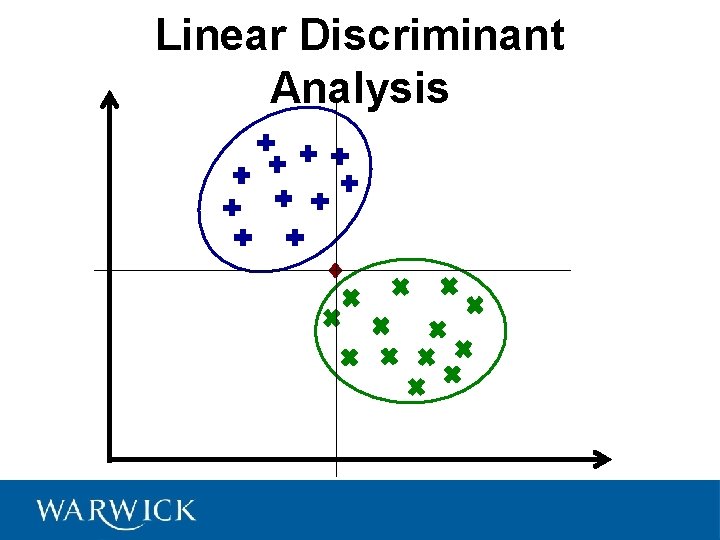
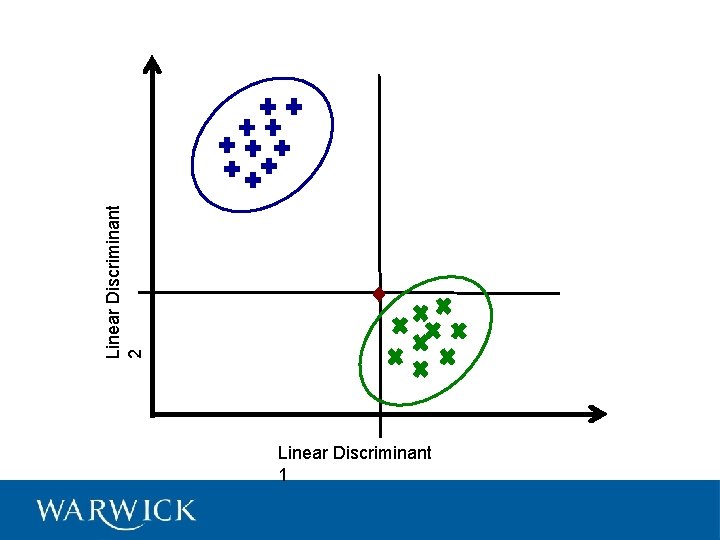
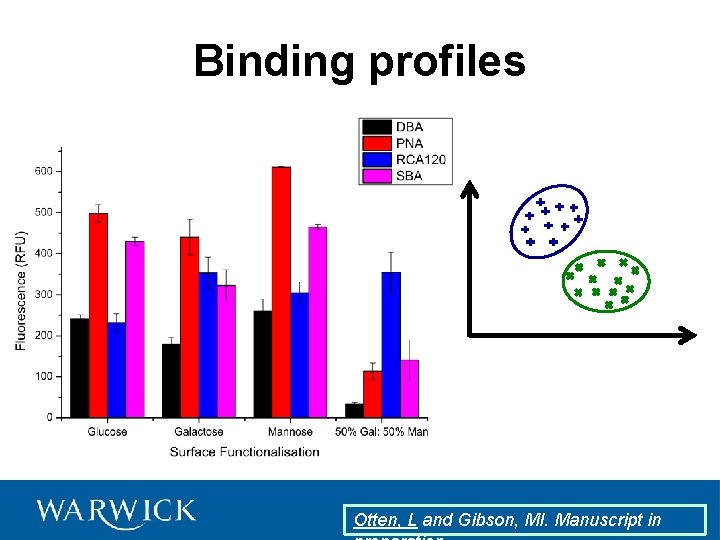
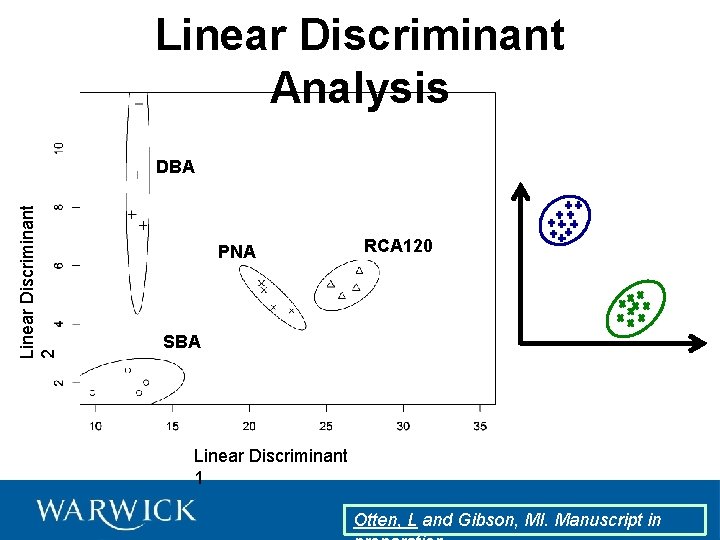
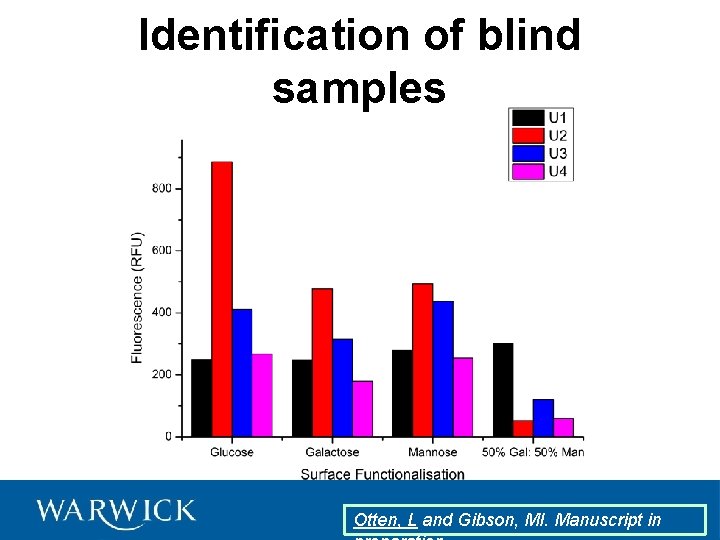
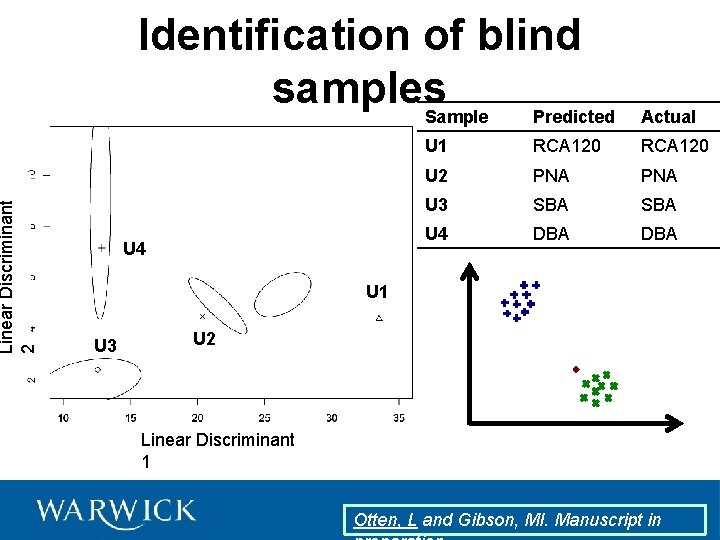
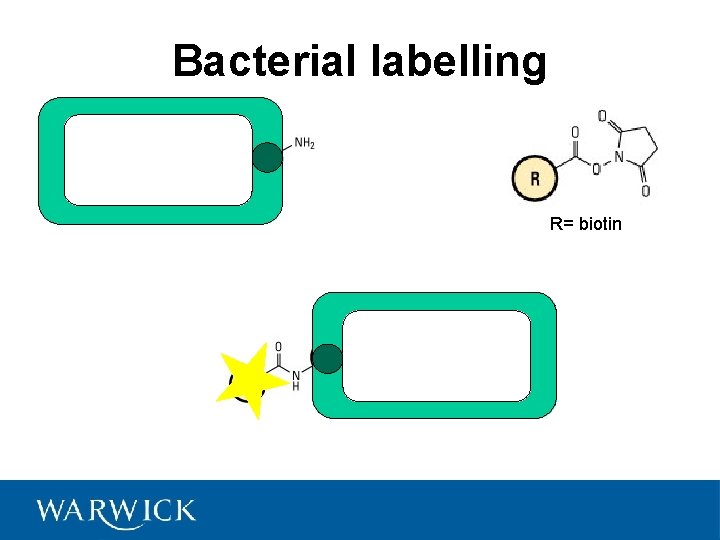
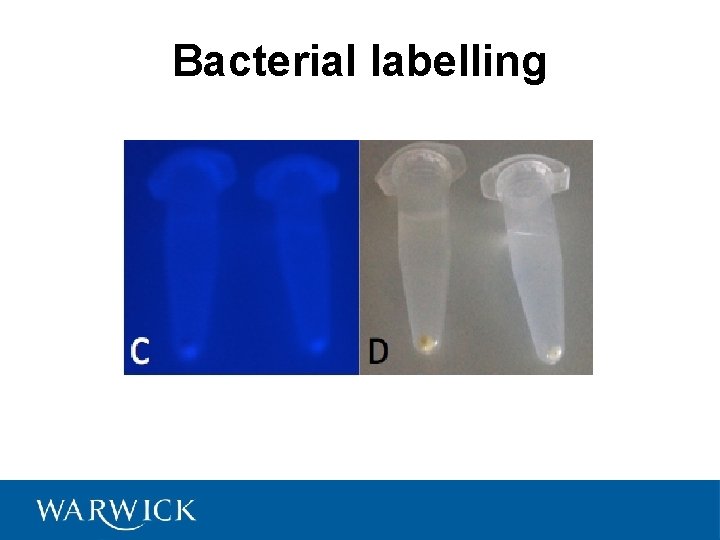
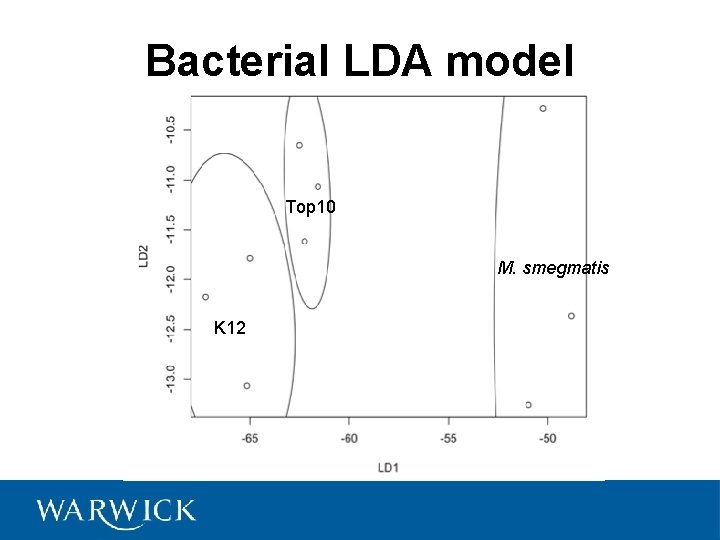
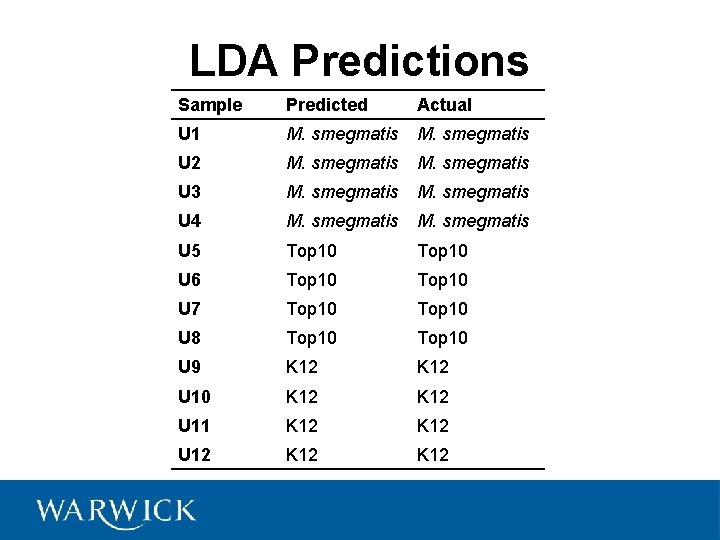
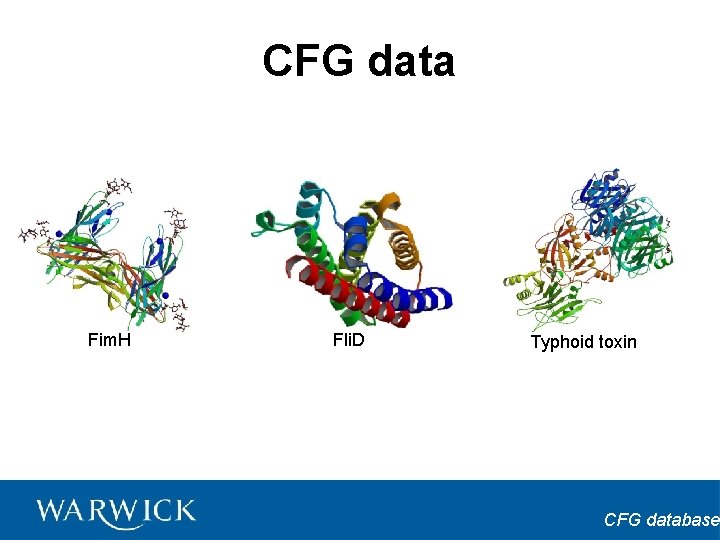
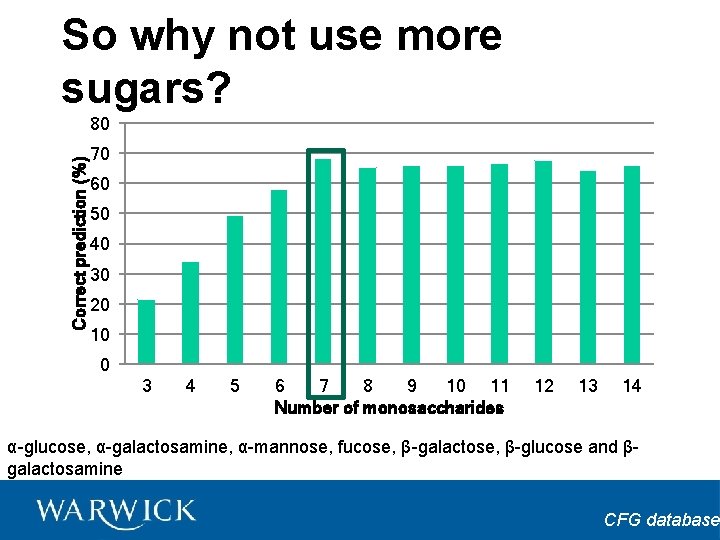
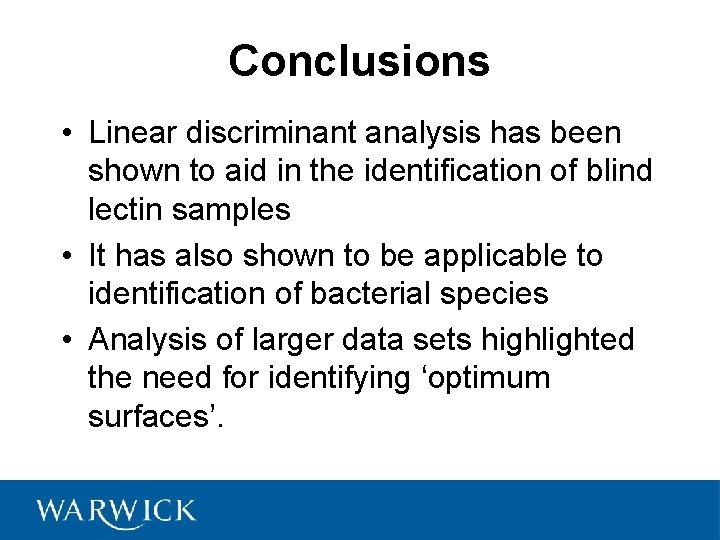
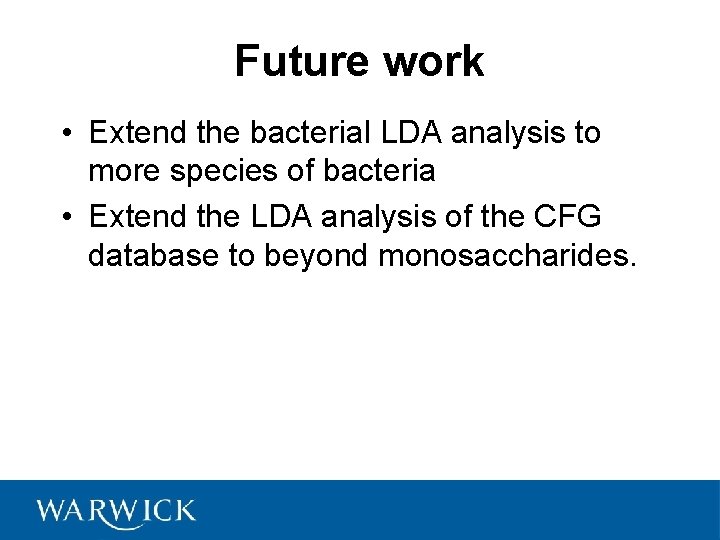
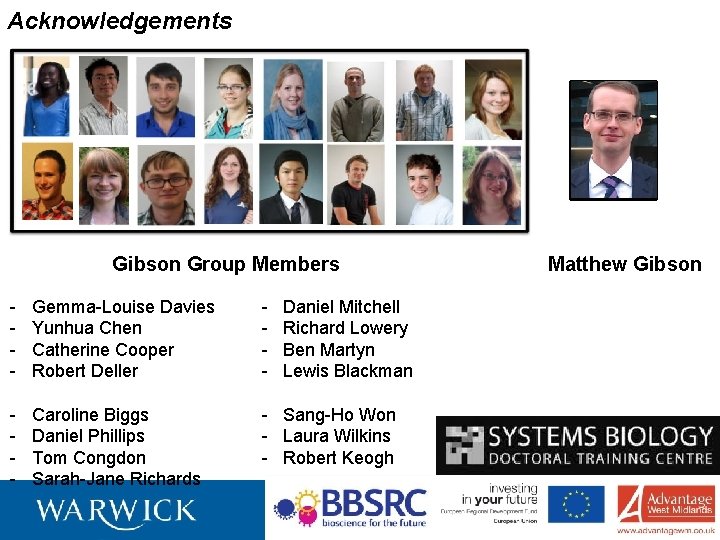
- Slides: 23
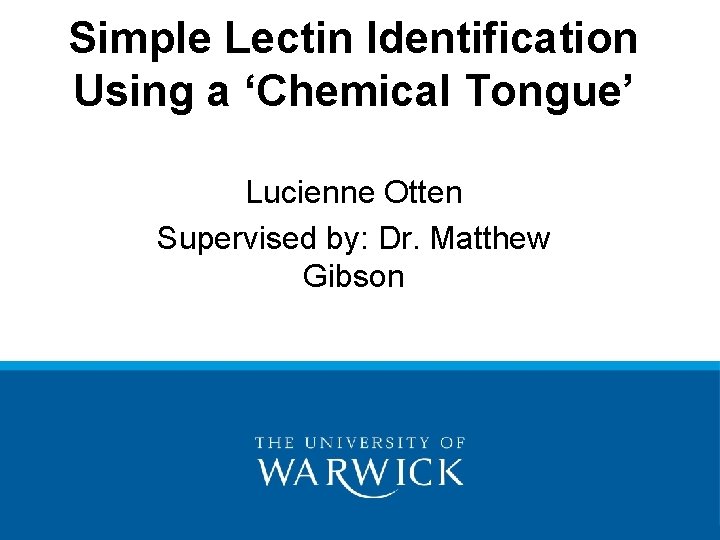
Simple Lectin Identification Using a ‘Chemical Tongue’ Lucienne Otten Supervised by: Dr. Matthew Gibson
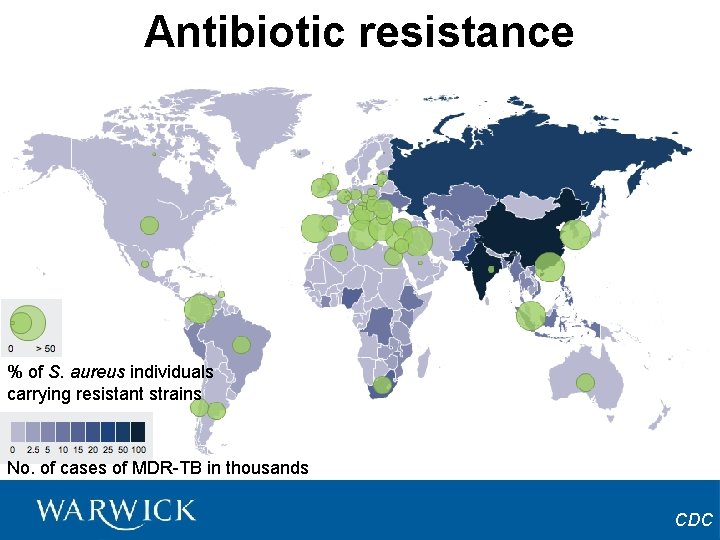
Antibiotic resistance % of S. aureus individuals carrying resistant strains No. of cases of MDR-TB in thousands CDC
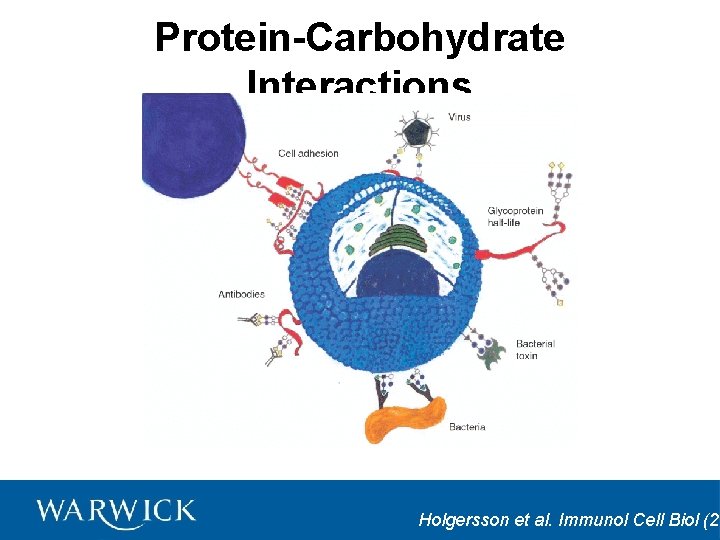
Protein-Carbohydrate Interactions Holgersson et al. Immunol Cell Biol (20
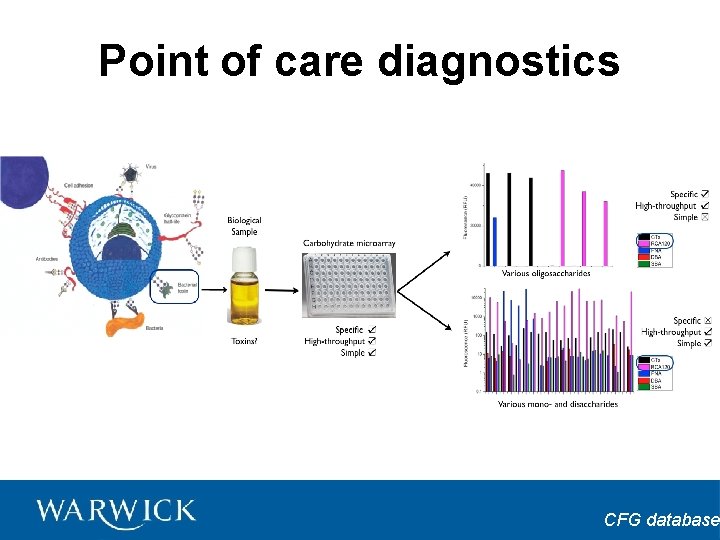
Point of care diagnostics CFG database
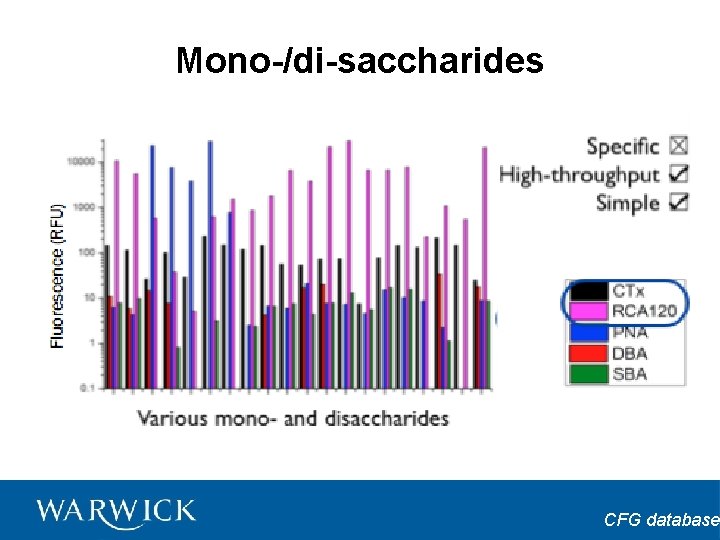
Mono-/di-saccharides CFG database
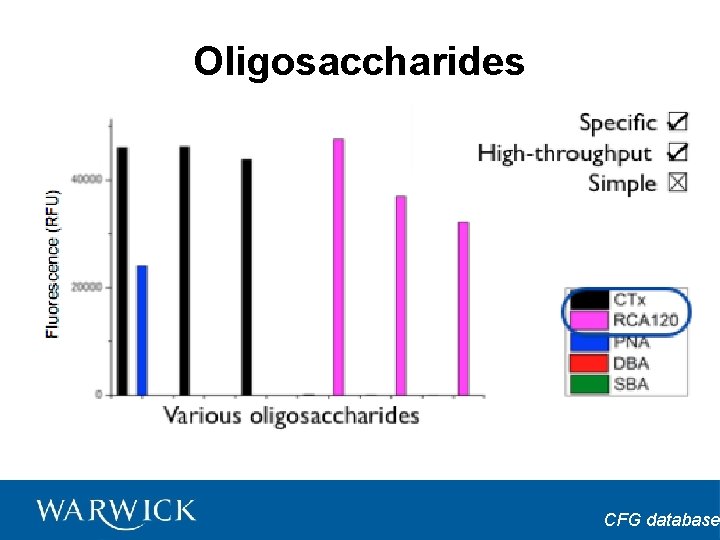
Oligosaccharides CFG database
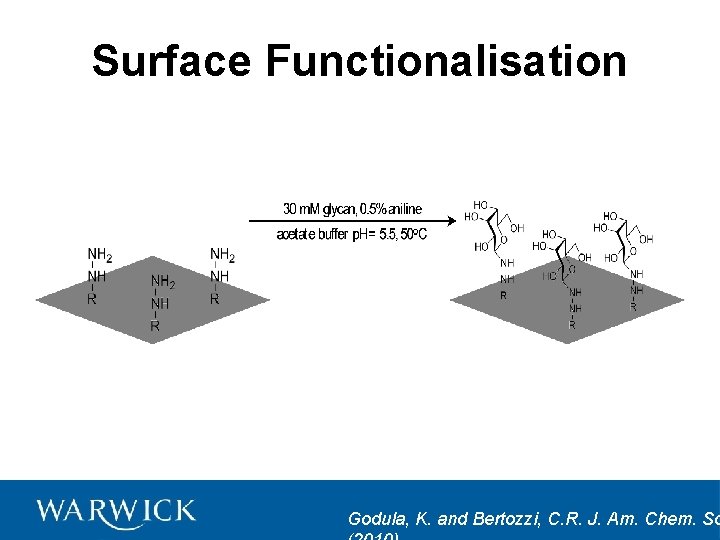
Surface Functionalisation Godula, K. and Bertozzi, C. R. J. Am. Chem. So
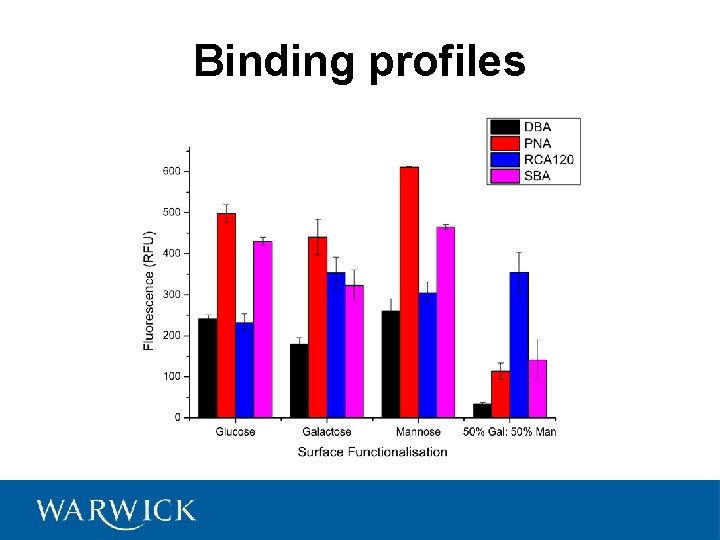
Binding profiles
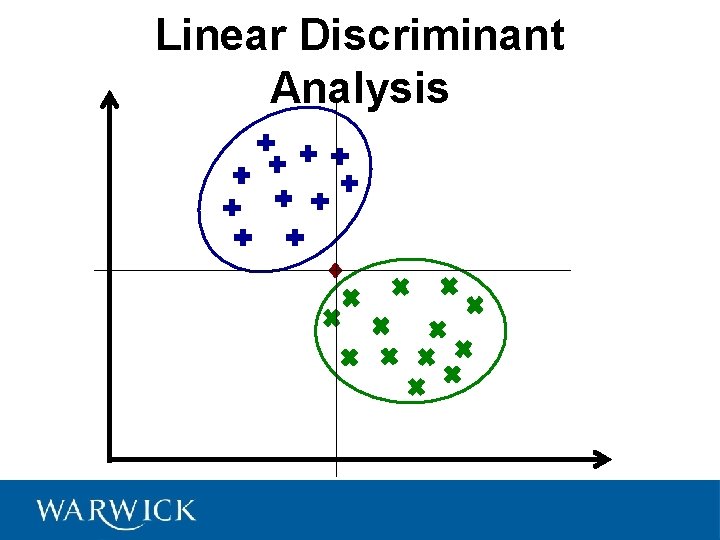
Linear Discriminant Analysis
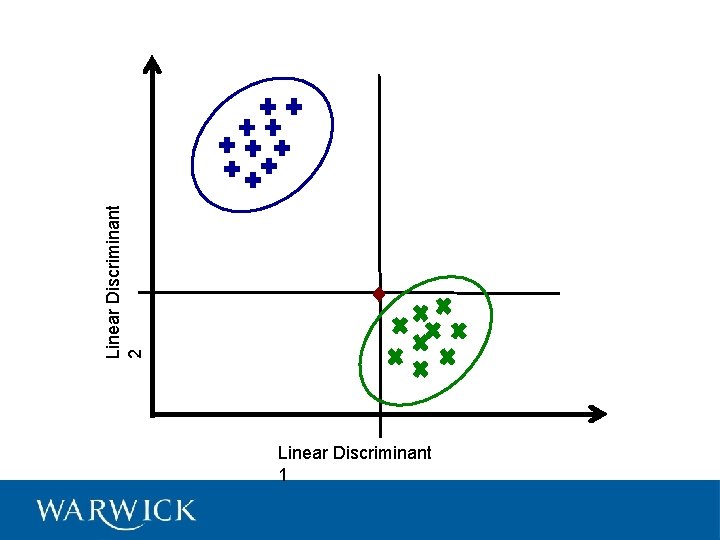
Linear Discriminant 2 Linear Discriminant 1
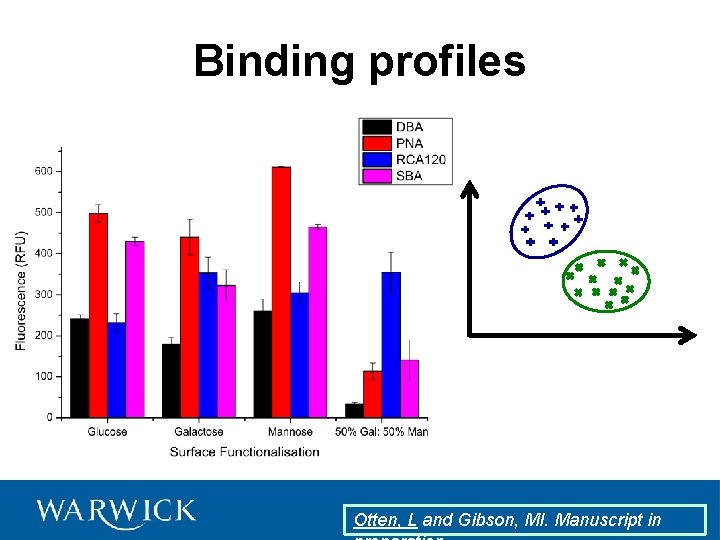
Binding profiles Otten, L and Gibson, MI. Manuscript in
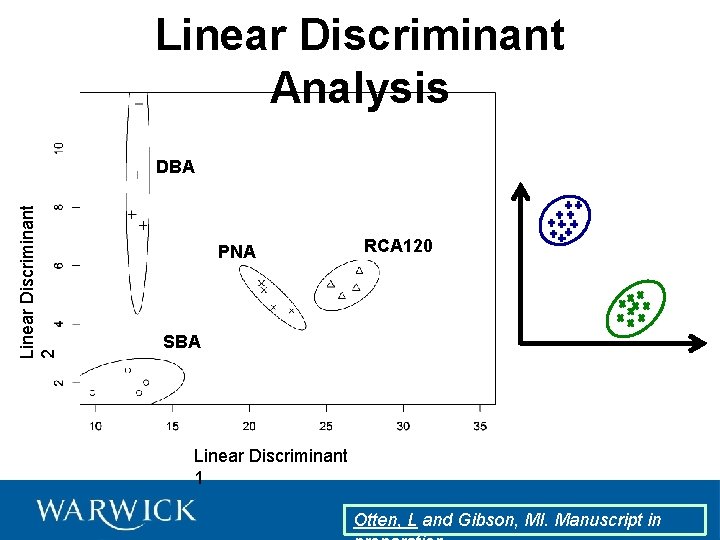
Linear Discriminant Analysis Linear Discriminant 2 DBA PNA RCA 120 SBA Linear Discriminant 1 Otten, L and Gibson, MI. Manuscript in
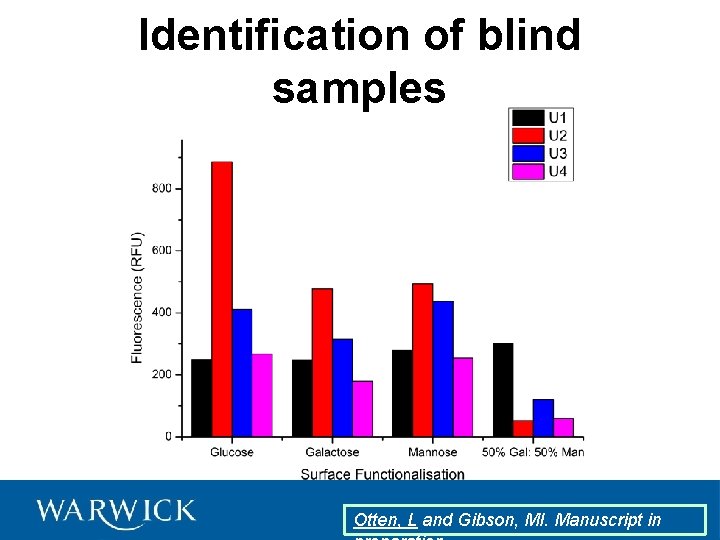
Identification of blind samples Otten, L and Gibson, MI. Manuscript in
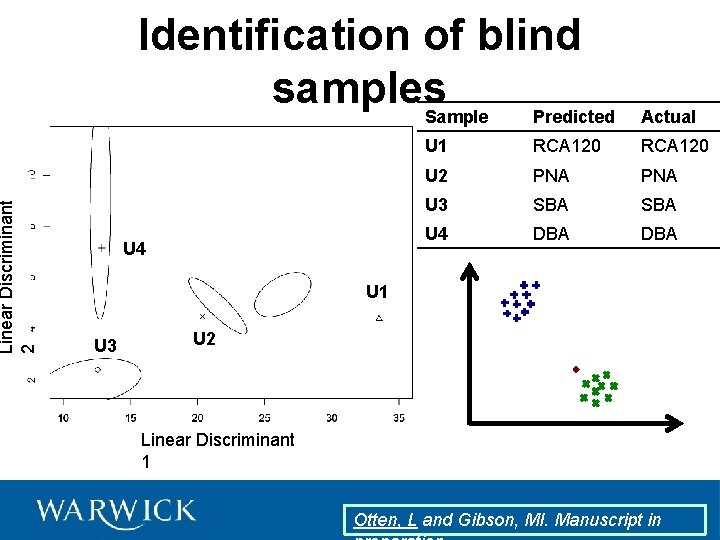
Linear Discriminant 2 Identification of blind samples Sample Predicted U 4 Actual U 1 RCA 120 U 2 PNA U 3 SBA U 4 DBA U 1 U 3 U 2 Linear Discriminant 1 Otten, L and Gibson, MI. Manuscript in
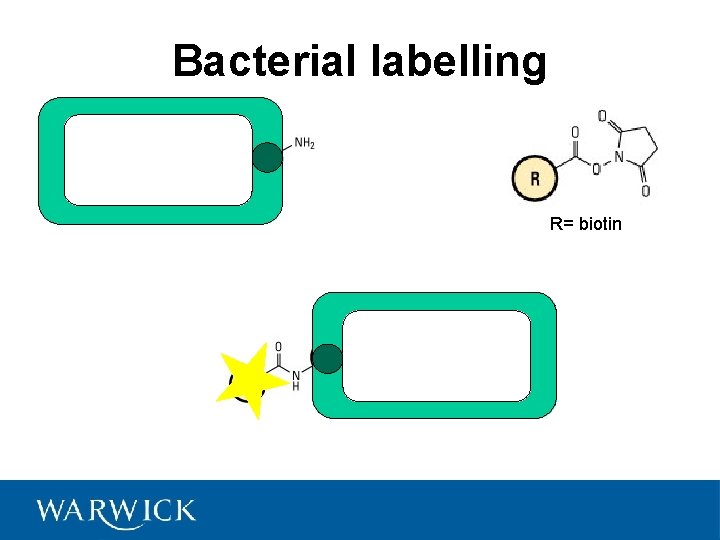
Bacterial labelling R= biotin
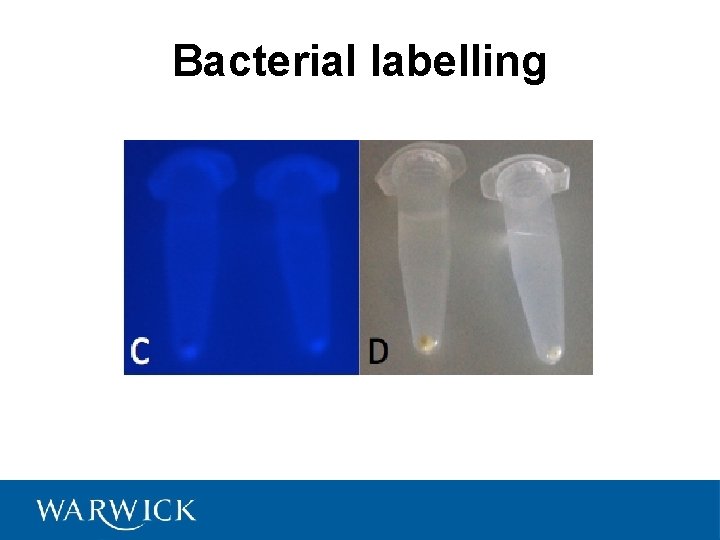
Bacterial labelling
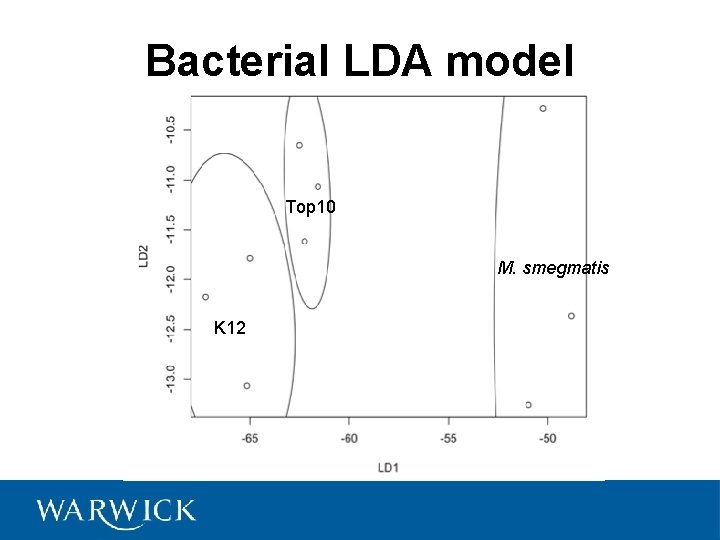
Bacterial LDA model Top 10 M. smegmatis K 12
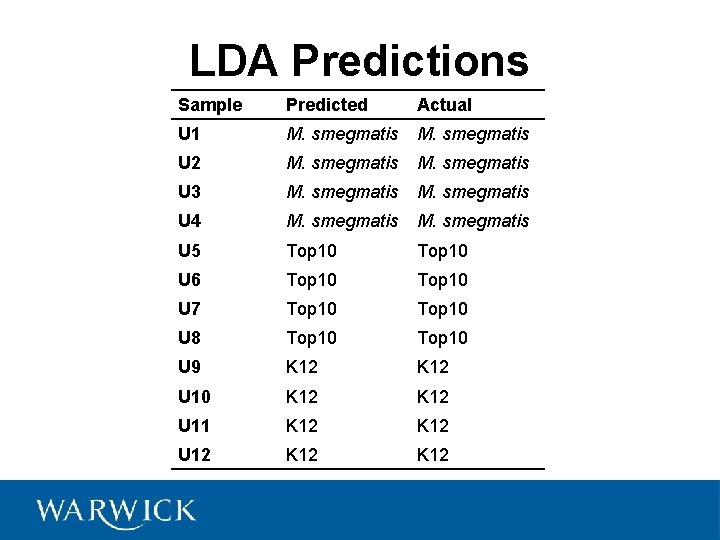
LDA Predictions Sample Predicted Actual U 1 M. smegmatis U 2 M. smegmatis U 3 M. smegmatis U 4 M. smegmatis U 5 Top 10 U 6 Top 10 U 7 Top 10 U 8 Top 10 U 9 K 12 U 10 K 12 U 11 K 12 U 12 K 12
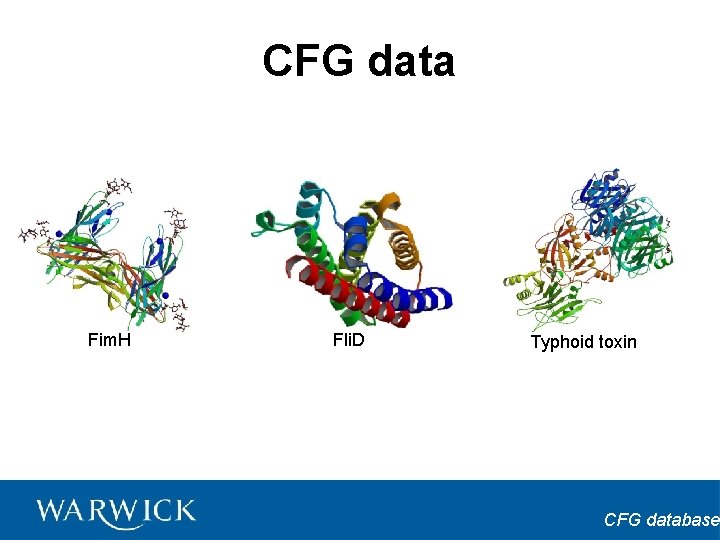
CFG data Fim. H Fli. D Typhoid toxin CFG database
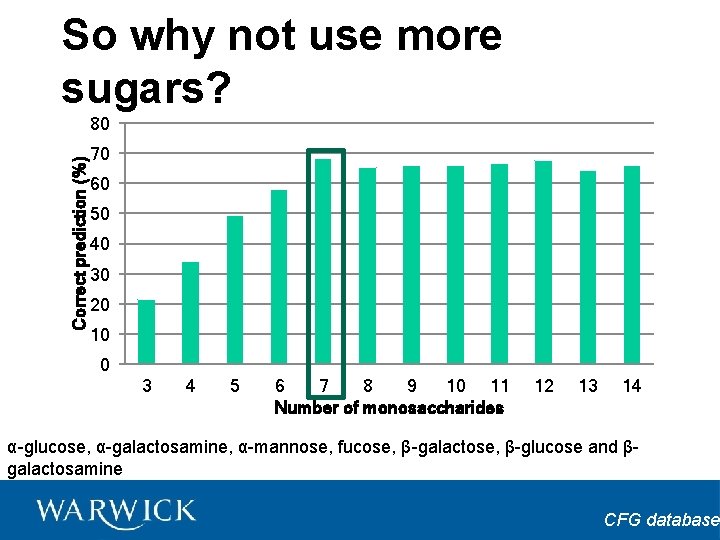
So why not use more sugars? Correct prediction (%) 80 70 60 50 40 30 20 10 0 3 4 5 6 7 8 9 10 11 Number of monosaccharides 12 13 14 α-glucose, α-galactosamine, α-mannose, fucose, β-galactose, β-glucose and βgalactosamine CFG database
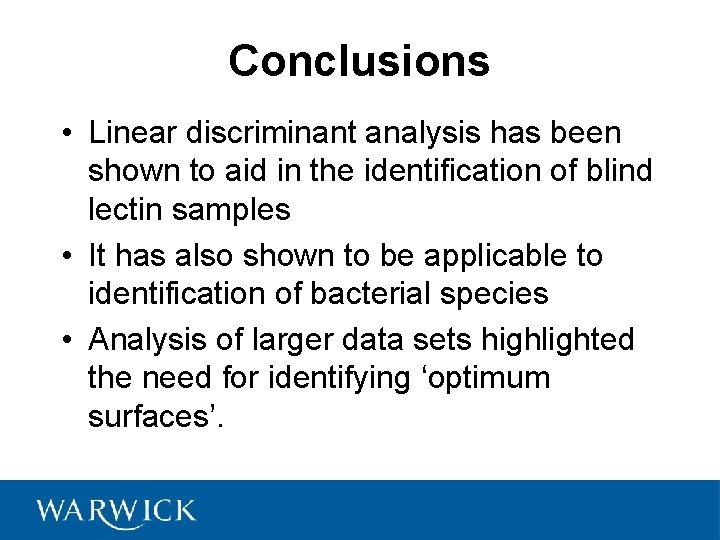
Conclusions • Linear discriminant analysis has been shown to aid in the identification of blind lectin samples • It has also shown to be applicable to identification of bacterial species • Analysis of larger data sets highlighted the need for identifying ‘optimum surfaces’.
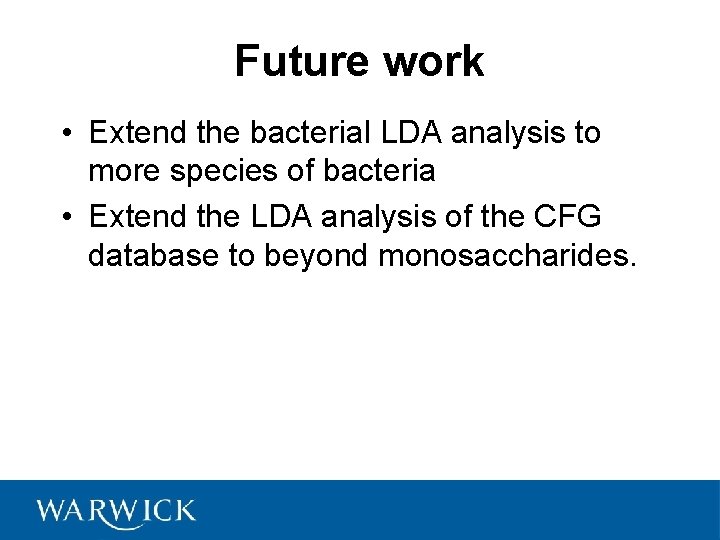
Future work • Extend the bacterial LDA analysis to more species of bacteria • Extend the LDA analysis of the CFG database to beyond monosaccharides.
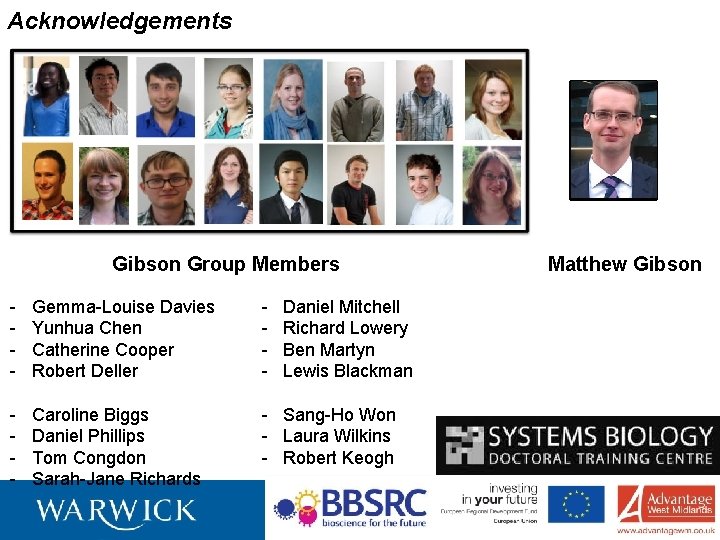
Acknowledgements Gibson Group Members - Gemma-Louise Davies Yunhua Chen Catherine Cooper Robert Deller - - Caroline Biggs Daniel Phillips Tom Congdon Sarah-Jane Richards - Sang-Ho Won - Laura Wilkins - Robert Keogh Daniel Mitchell Richard Lowery Ben Martyn Lewis Blackman Matthew Gibson
Vector labs lectin
Lectin pathway
Tongue twisters powerpoint
Presumptive identification vs positive identification
Section 2 reinforcement classifying chemical reactions
Chapter 7 chemical formulas and chemical compounds test
Chemical reactions section 2 classifying chemical reactions
Chemical reactions section 1 chemical changes
Modern chemistry chapter 7
Chapter 18 chemical reactions balancing chemical equations
Present simple past simple future simple exercises
Simple past simple present simple future
Present simple present continuous past simple future simple
Present simple present continuous past simple future simple
Simple present simple past simple future
Present simple past simple present continuous exercises
Walk simple past tense
Frases afirmativas simple present
Future simple present simple
Barium nitrate formula by criss cross method
Using system.collections.generic
Accumulator ac
Chapter 19 chemical reactions simple word equations
Simple sugar chemical bonds