Research Methods in IO Psychology Research is formalized
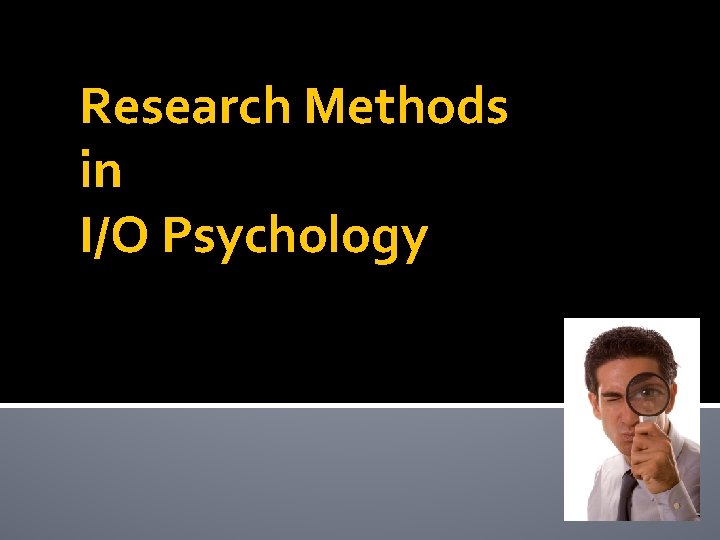
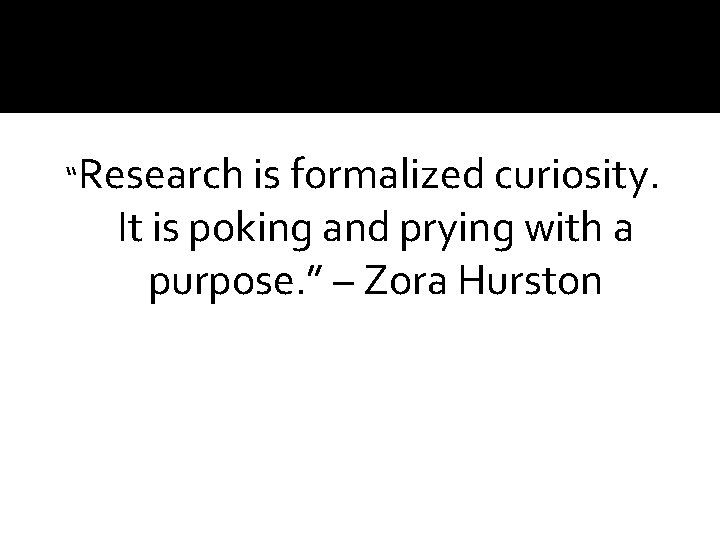
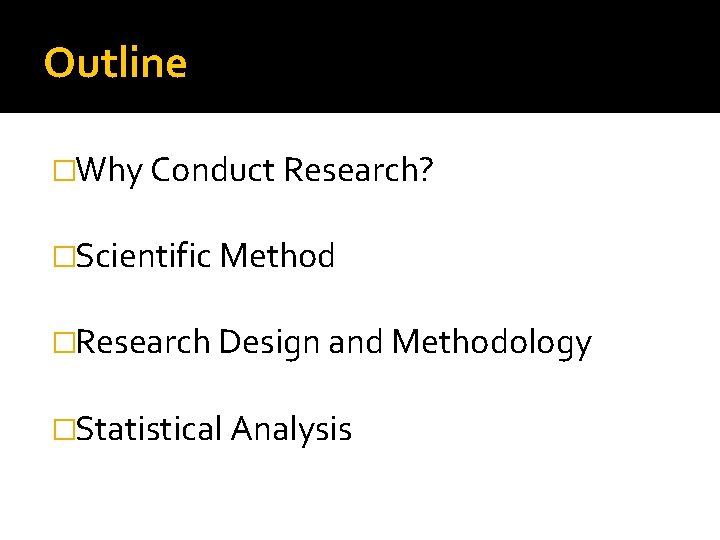
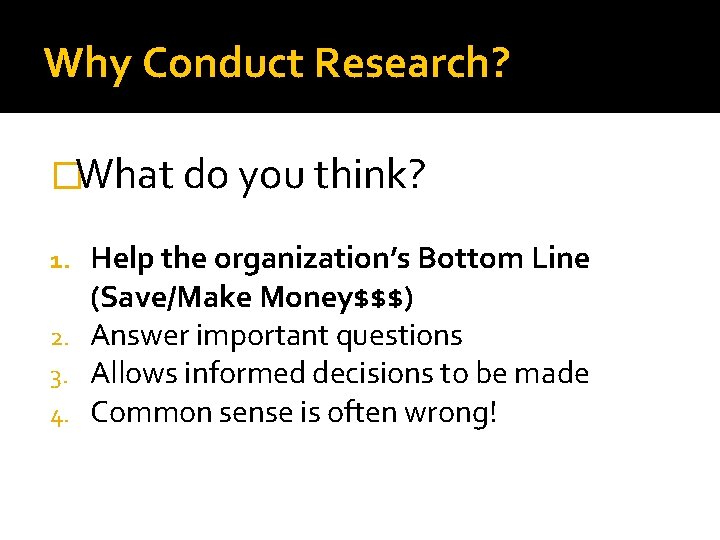
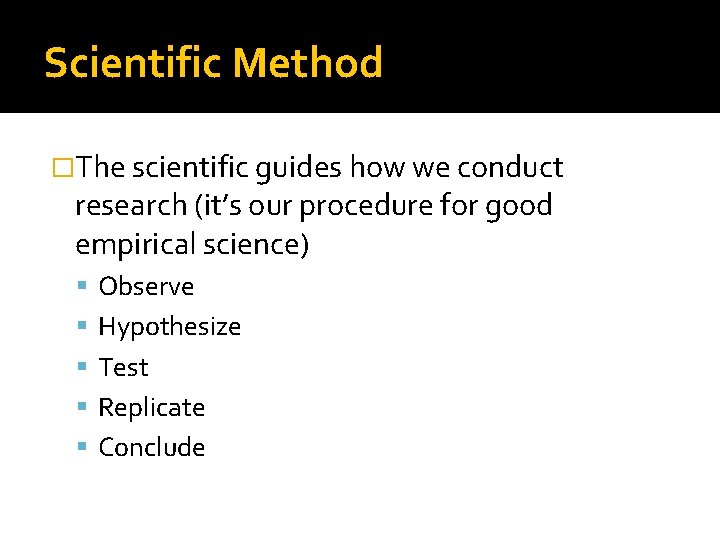
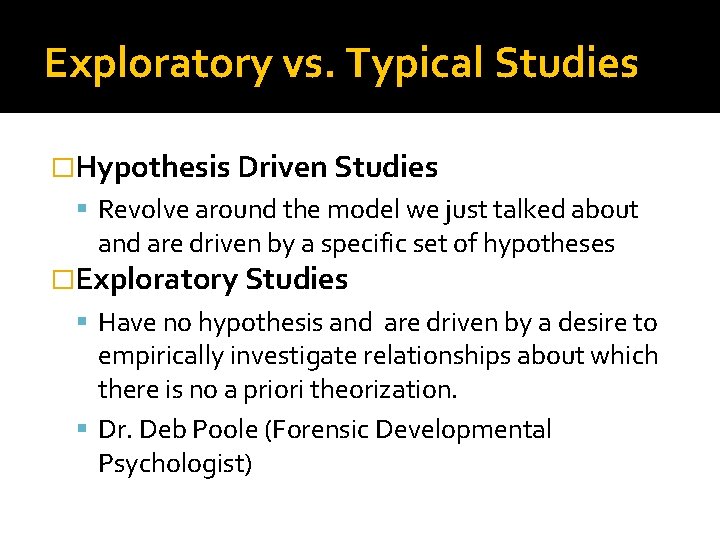
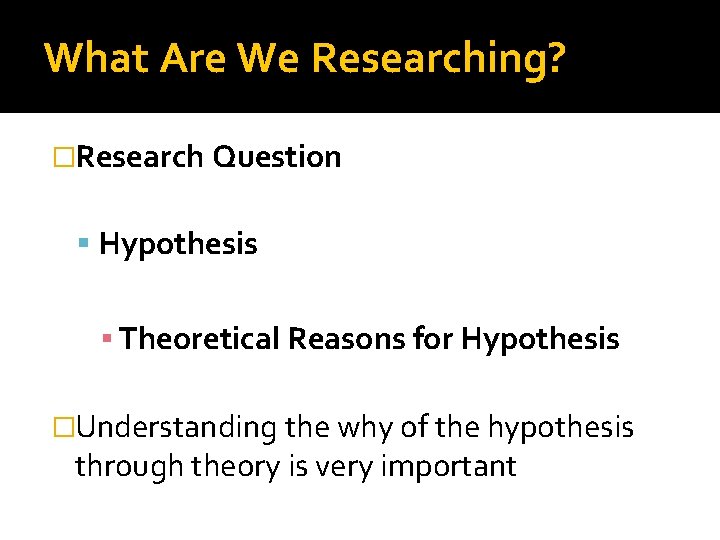
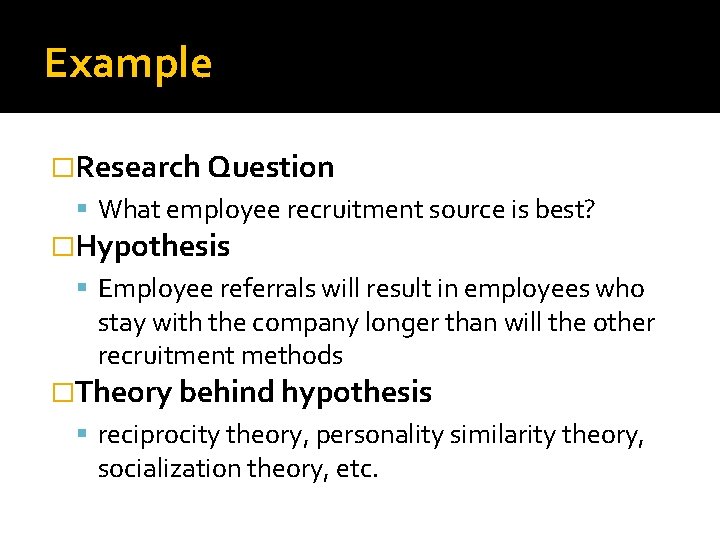
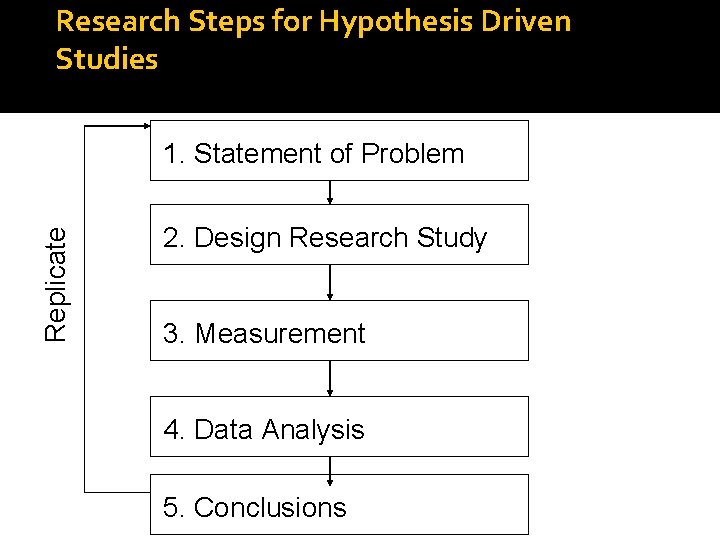
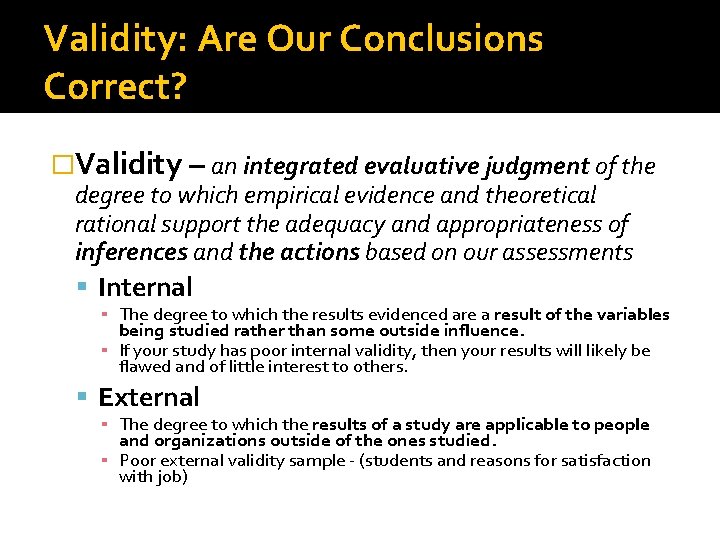
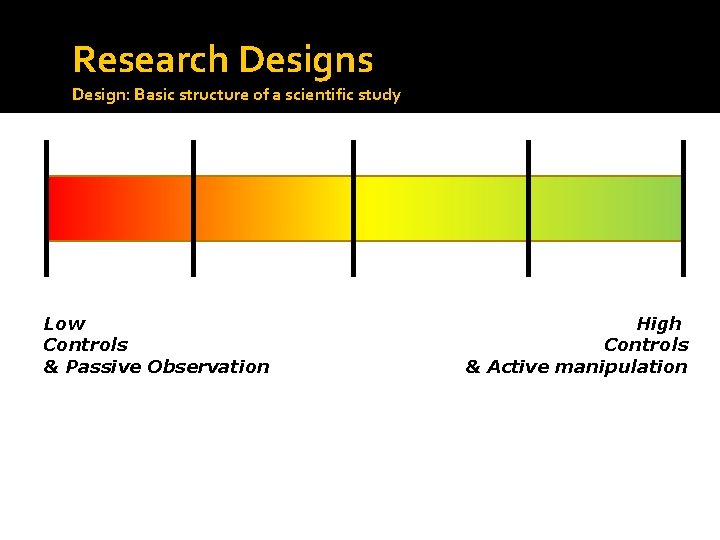
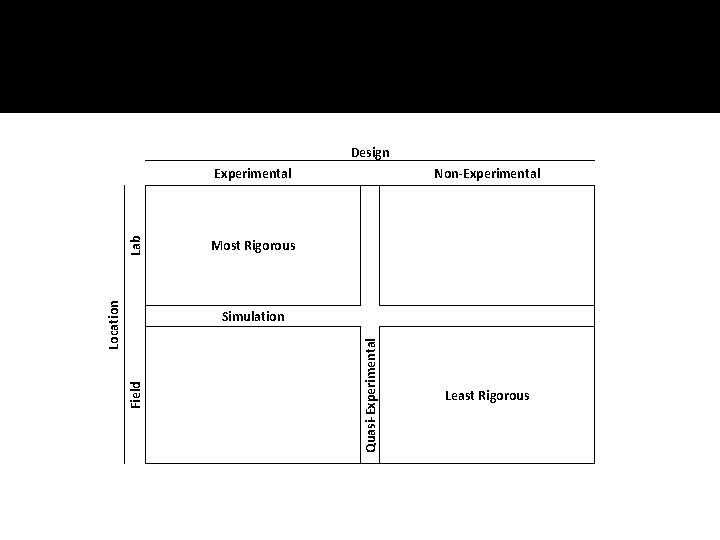
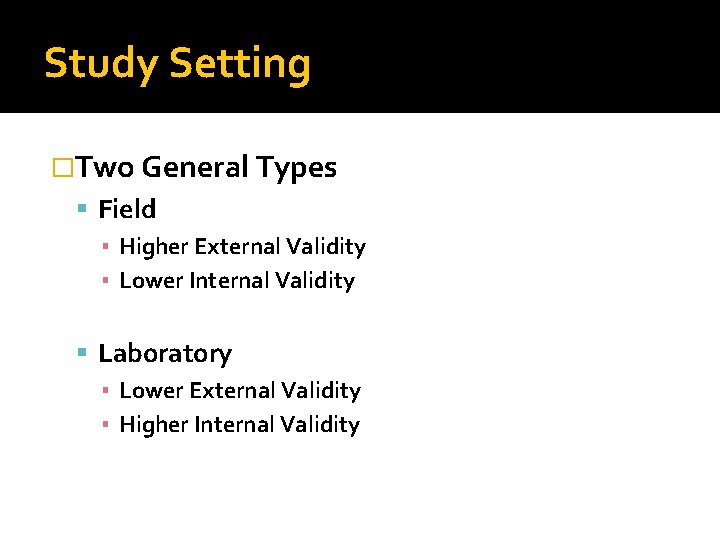
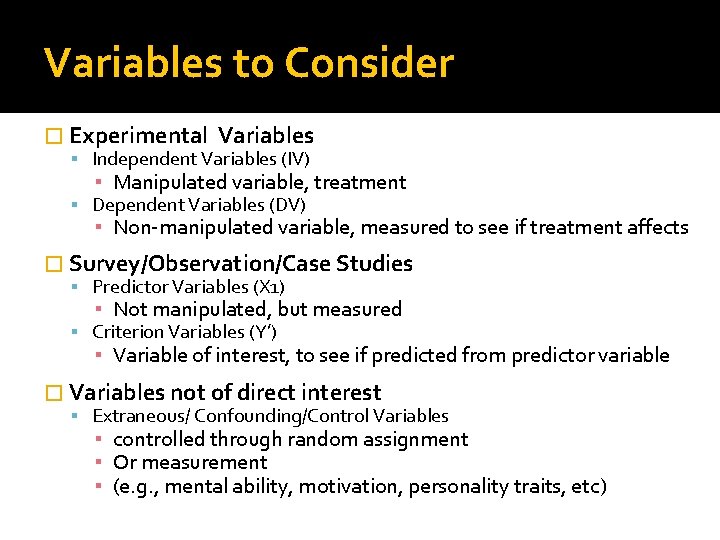
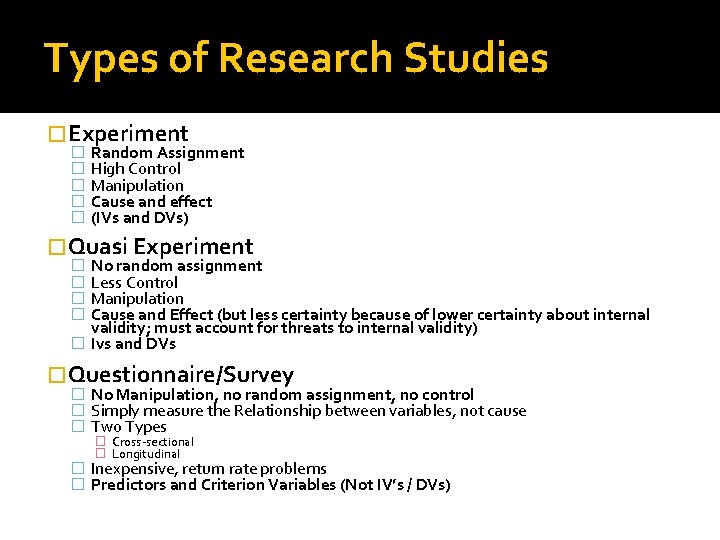
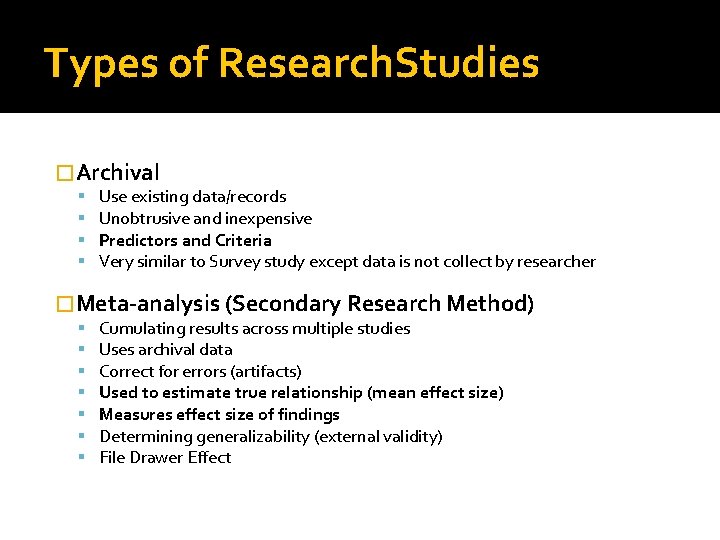
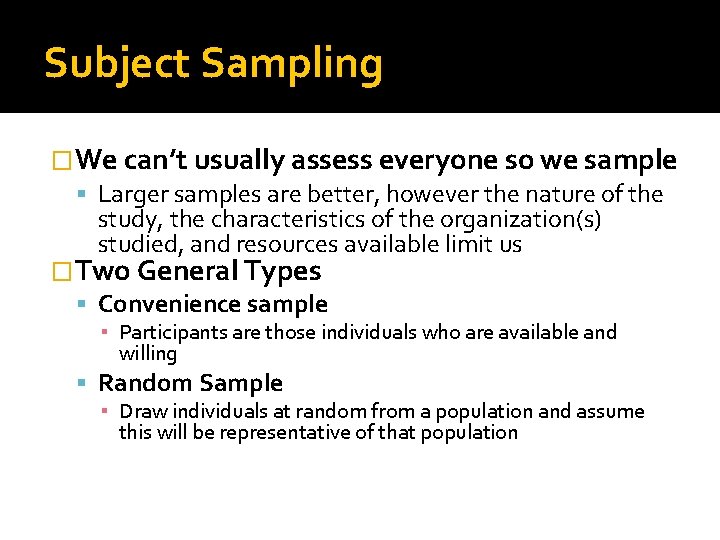
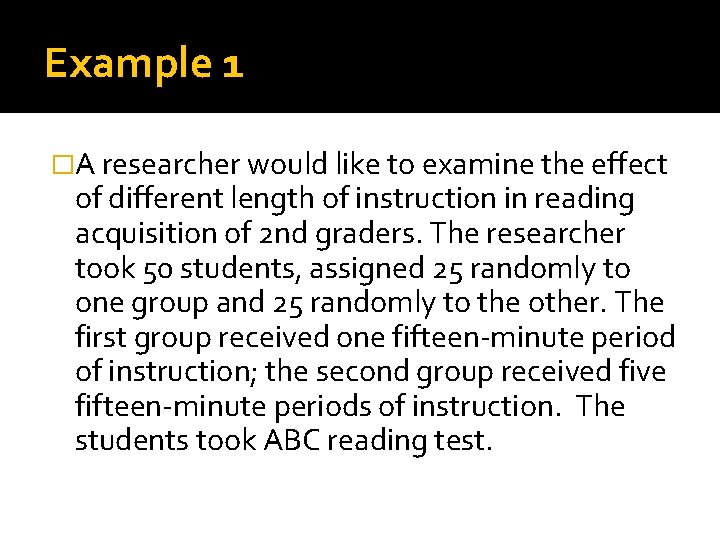
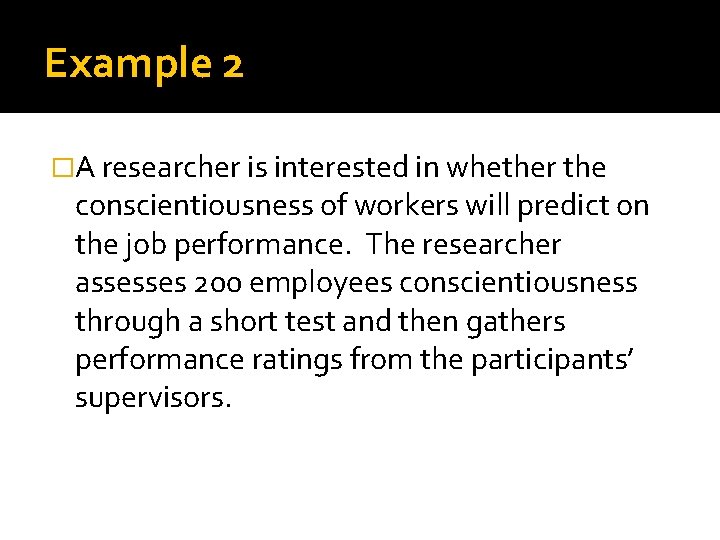
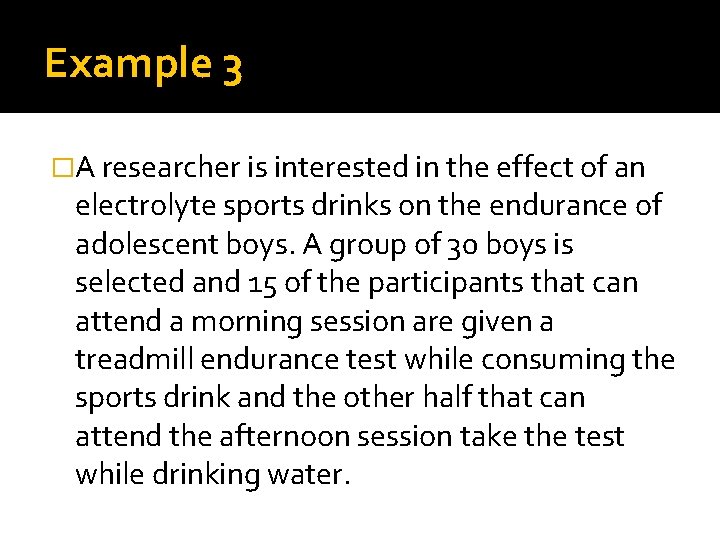
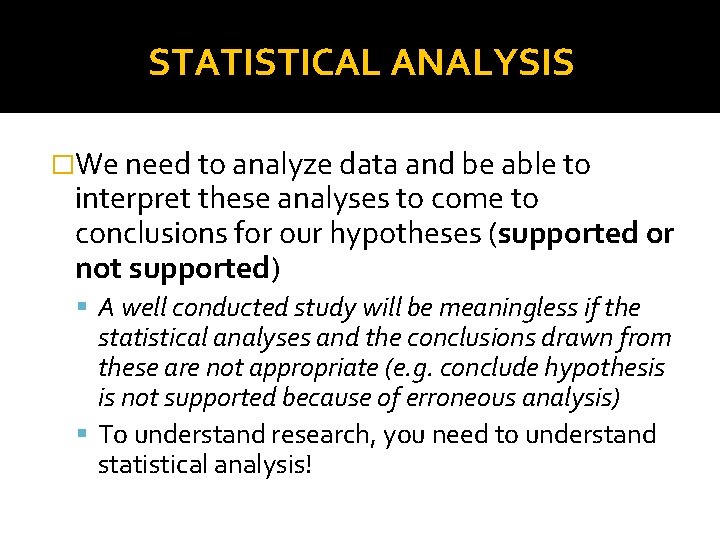
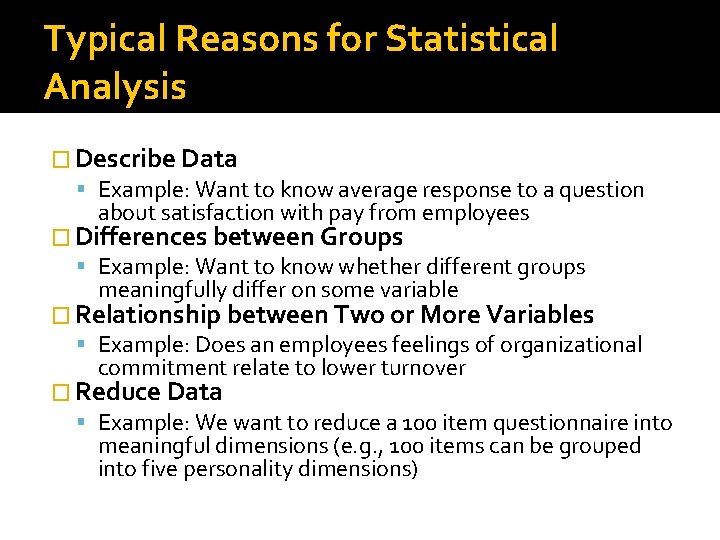
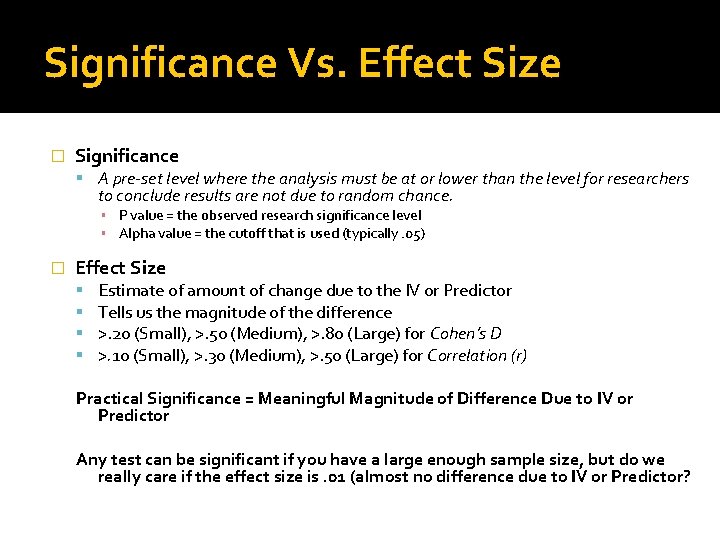
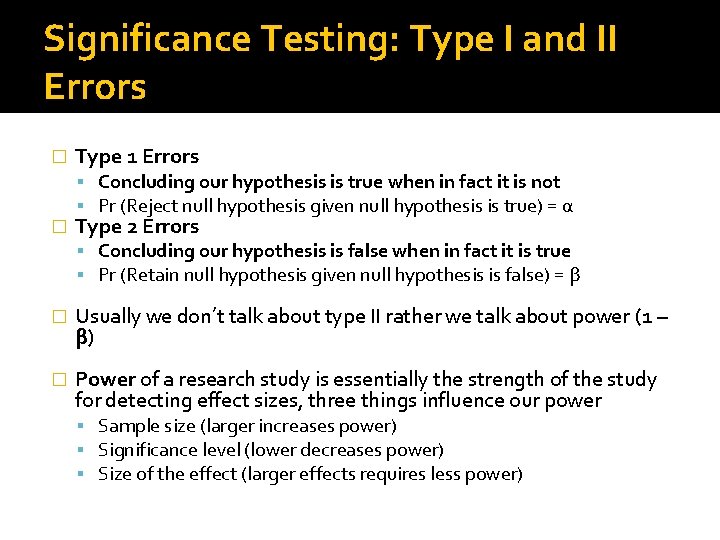
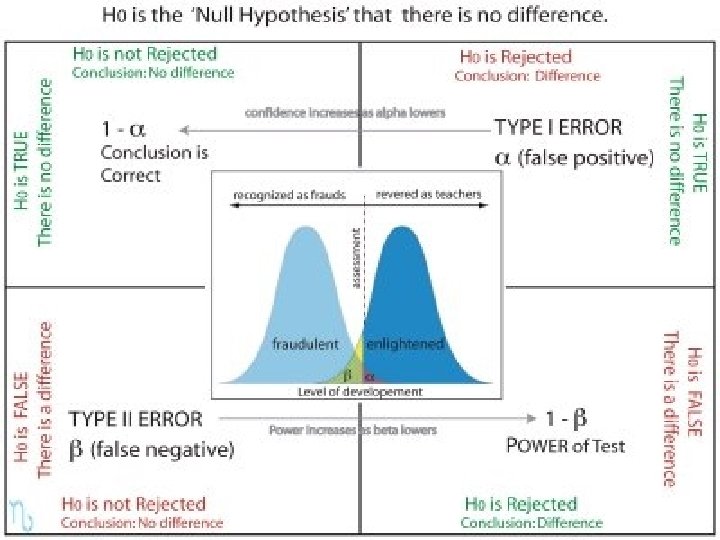
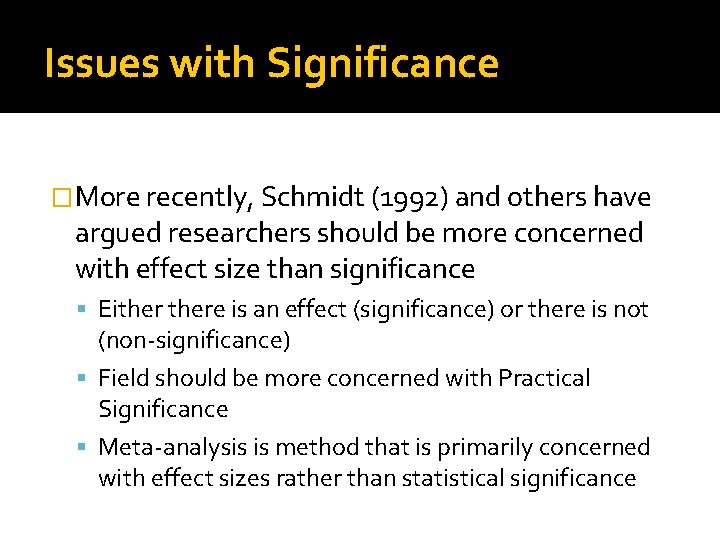
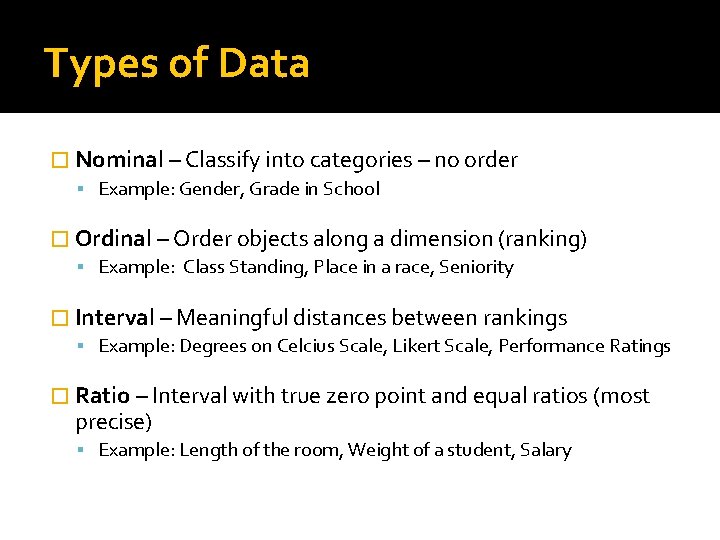
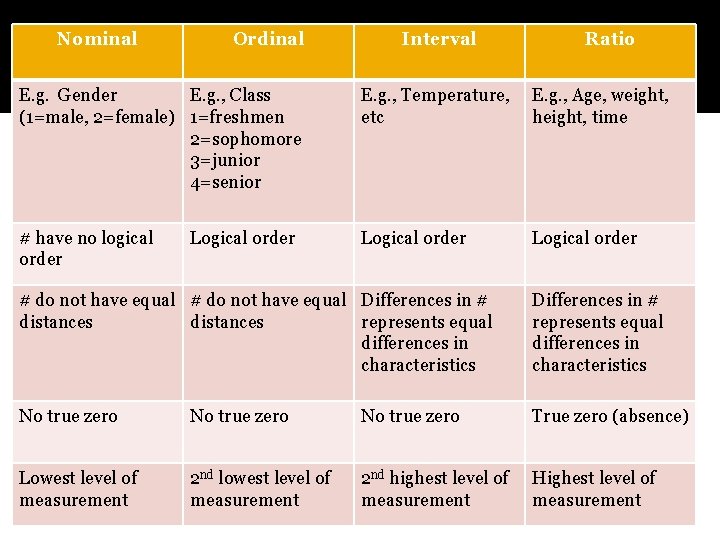
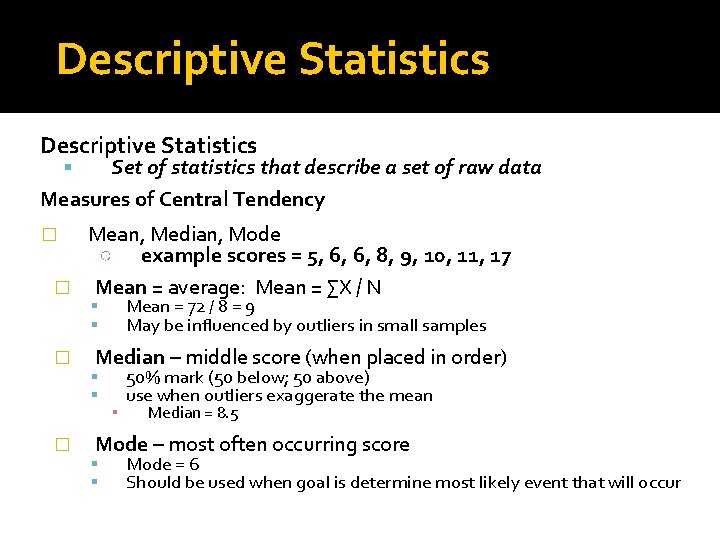
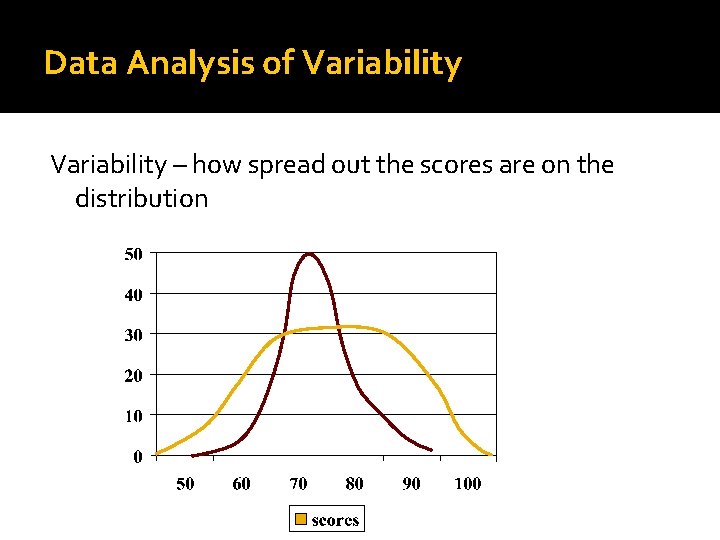
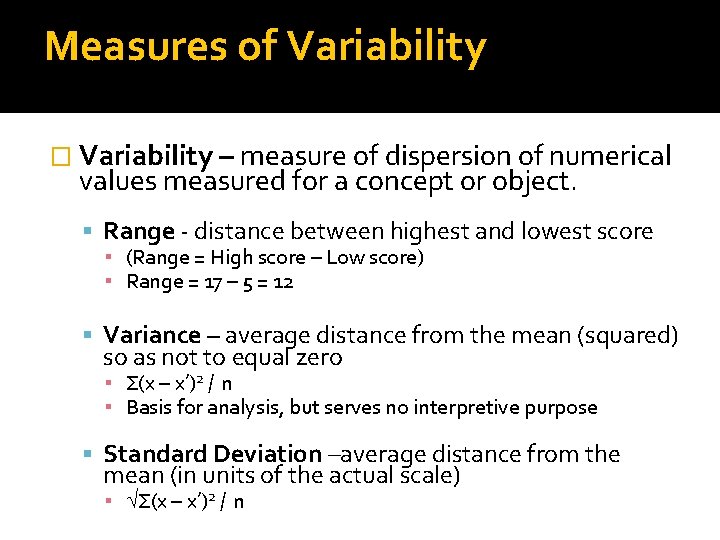
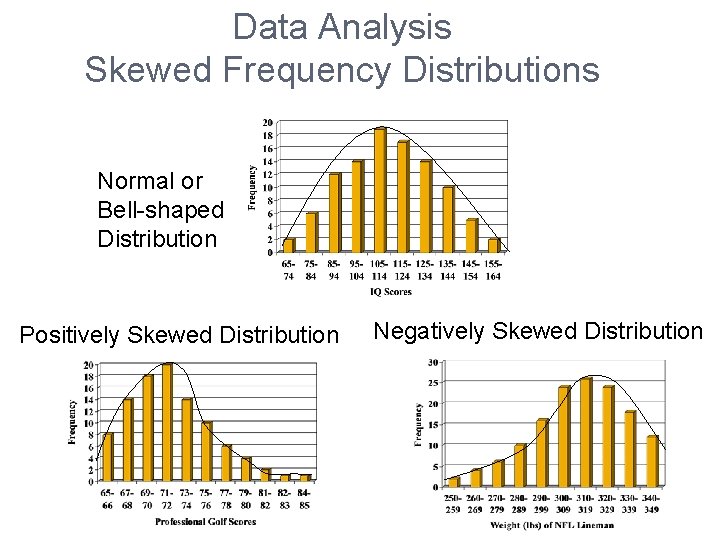
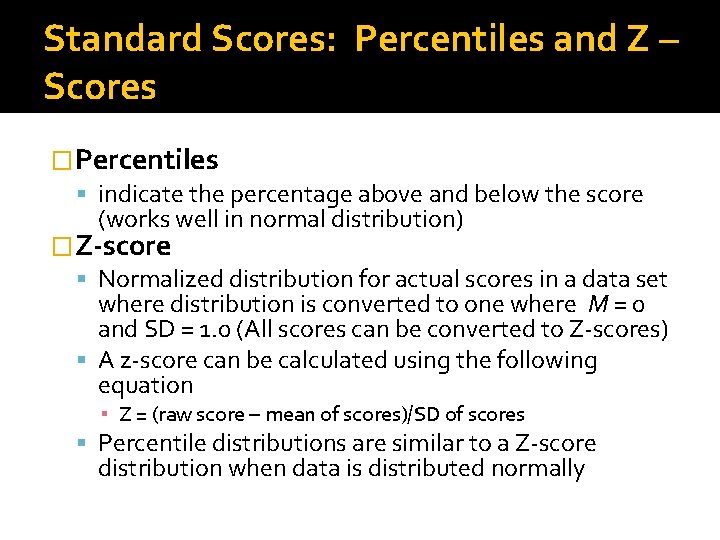
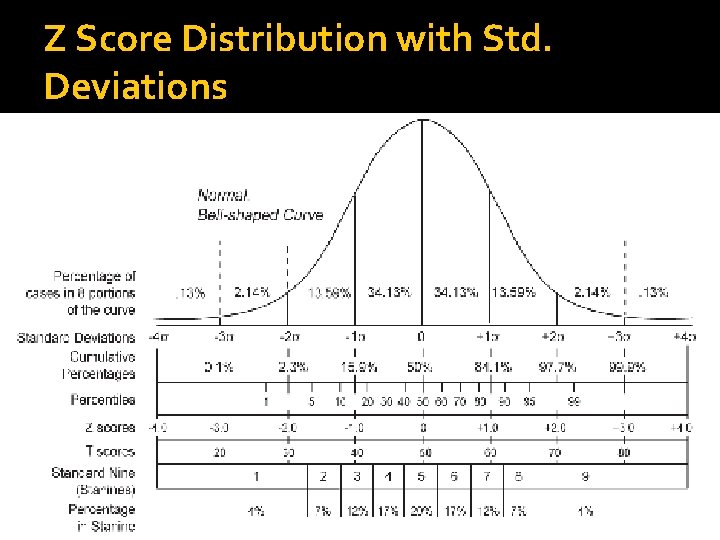
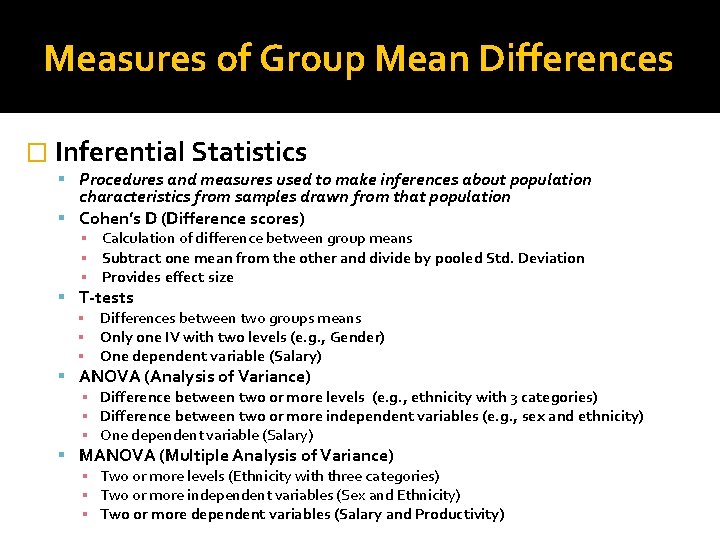
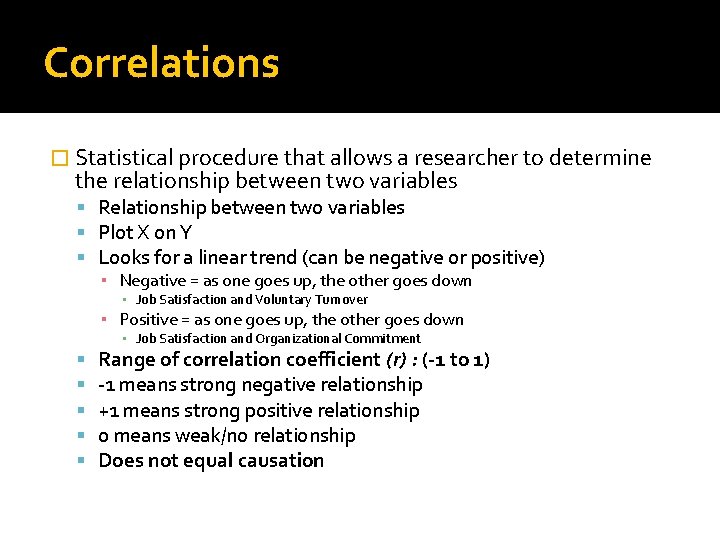
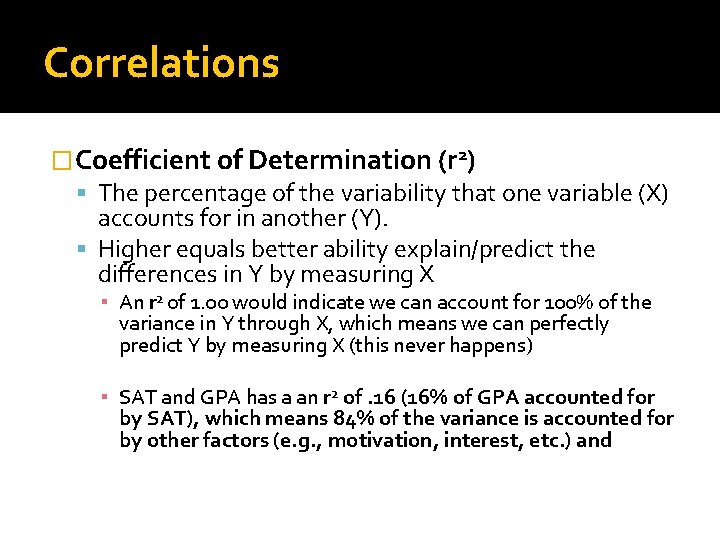
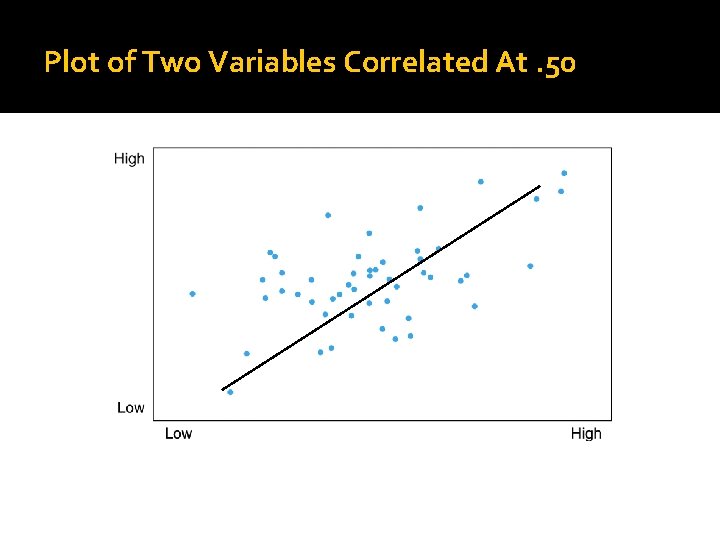
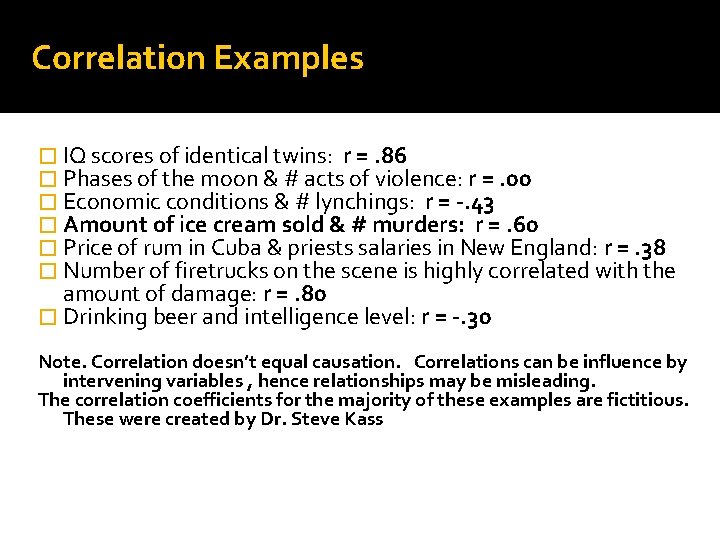
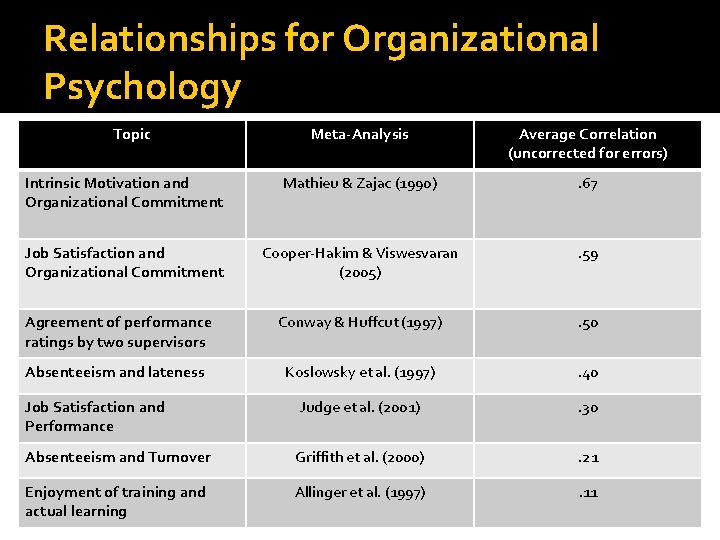
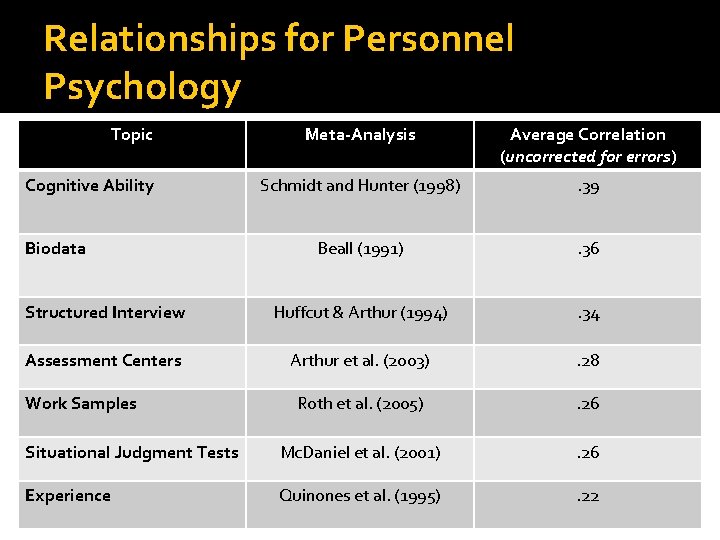
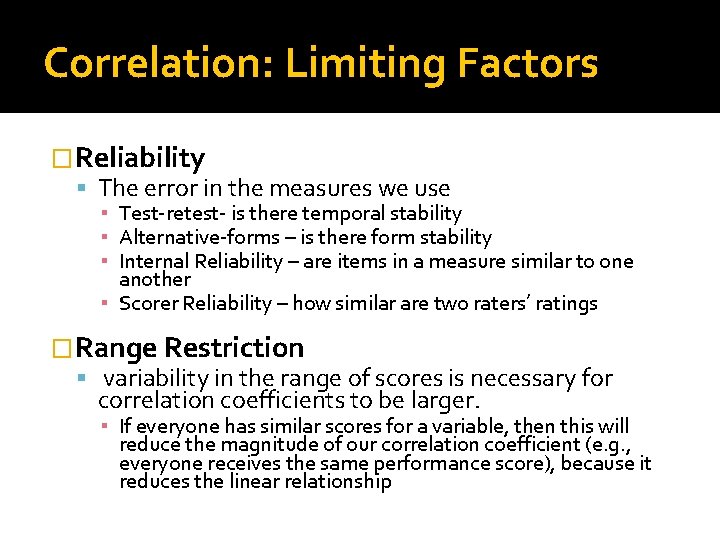
- Slides: 42
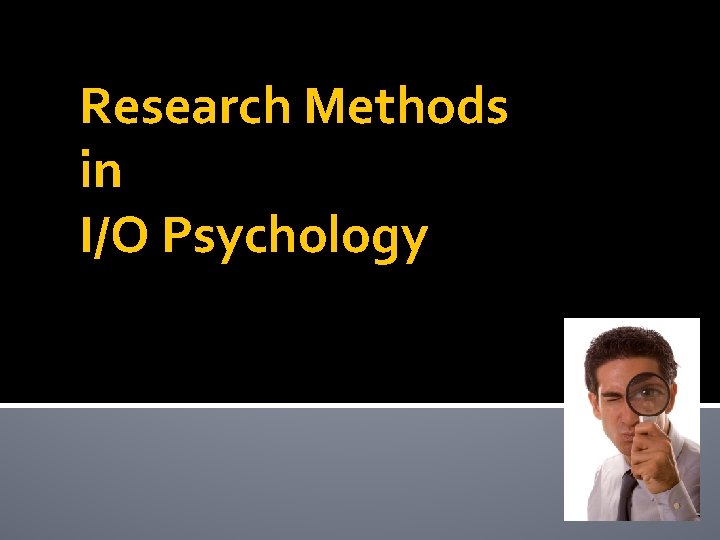
Research Methods in I/O Psychology
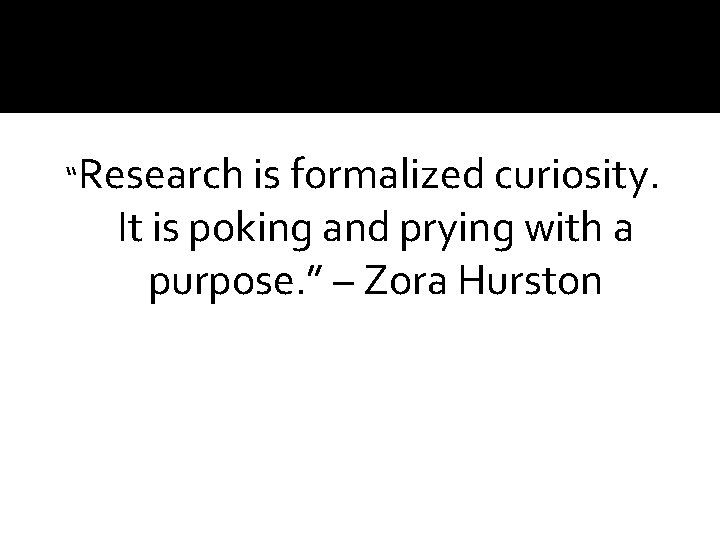
“Research is formalized curiosity. It is poking and prying with a purpose. ” – Zora Hurston
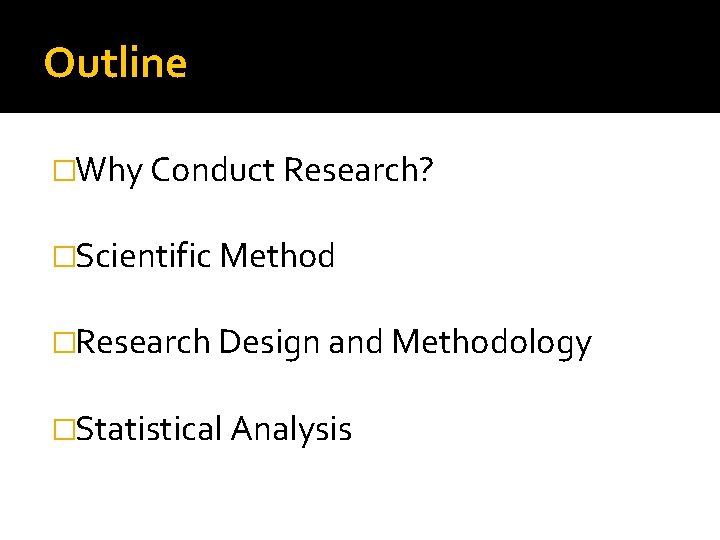
Outline �Why Conduct Research? �Scientific Method �Research Design and Methodology �Statistical Analysis
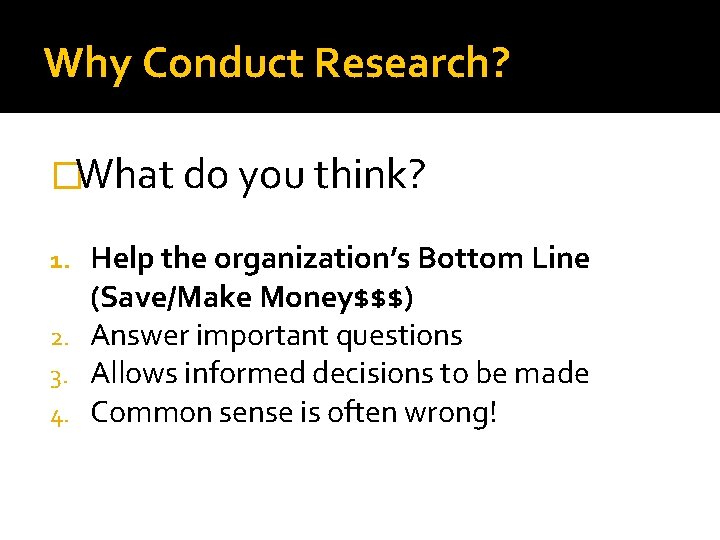
Why Conduct Research? �What do you think? Help the organization’s Bottom Line (Save/Make Money$$$) 2. Answer important questions 3. Allows informed decisions to be made 4. Common sense is often wrong! 1.
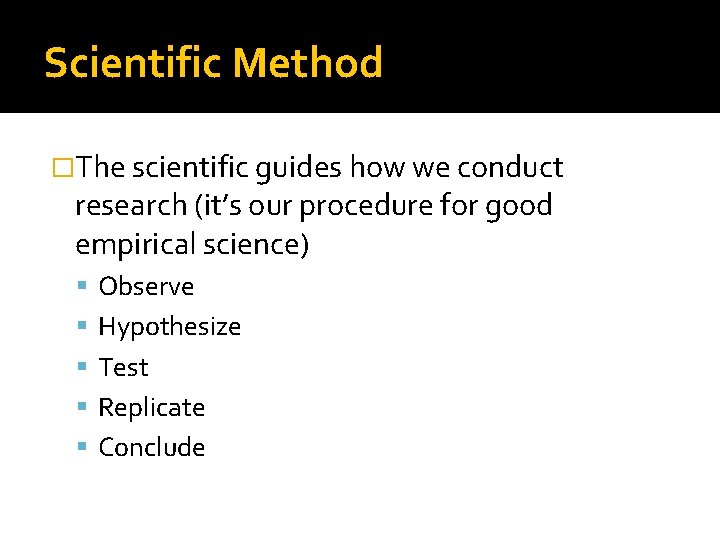
Scientific Method �The scientific guides how we conduct research (it’s our procedure for good empirical science) Observe Hypothesize Test Replicate Conclude
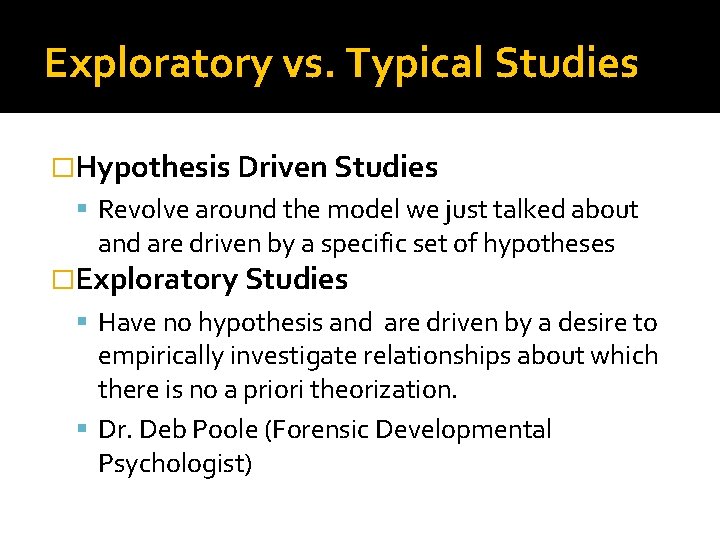
Exploratory vs. Typical Studies �Hypothesis Driven Studies Revolve around the model we just talked about and are driven by a specific set of hypotheses �Exploratory Studies Have no hypothesis and are driven by a desire to empirically investigate relationships about which there is no a priori theorization. Dr. Deb Poole (Forensic Developmental Psychologist)
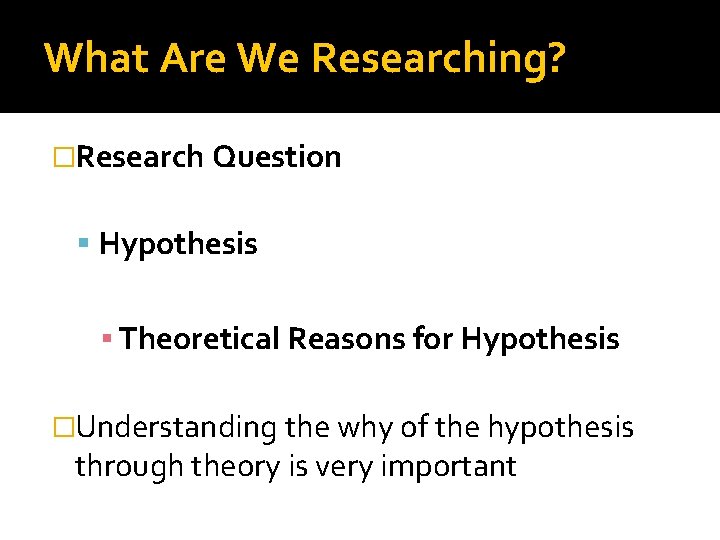
What Are We Researching? �Research Question Hypothesis ▪ Theoretical Reasons for Hypothesis �Understanding the why of the hypothesis through theory is very important
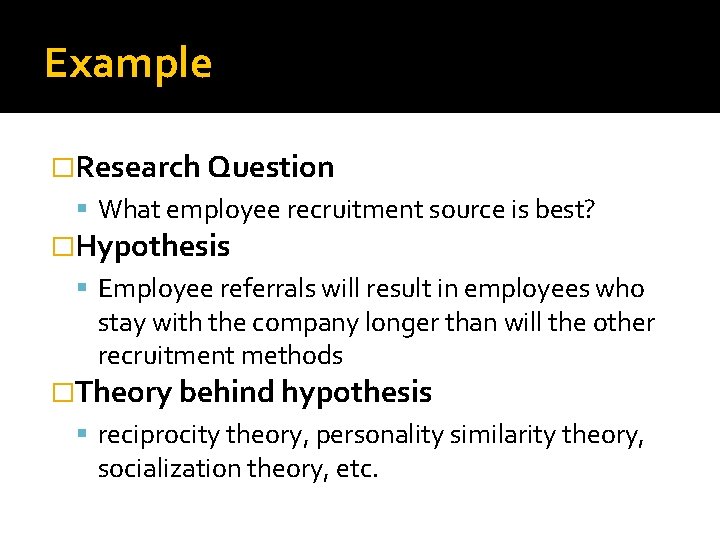
Example �Research Question What employee recruitment source is best? �Hypothesis Employee referrals will result in employees who stay with the company longer than will the other recruitment methods �Theory behind hypothesis reciprocity theory, personality similarity theory, socialization theory, etc.
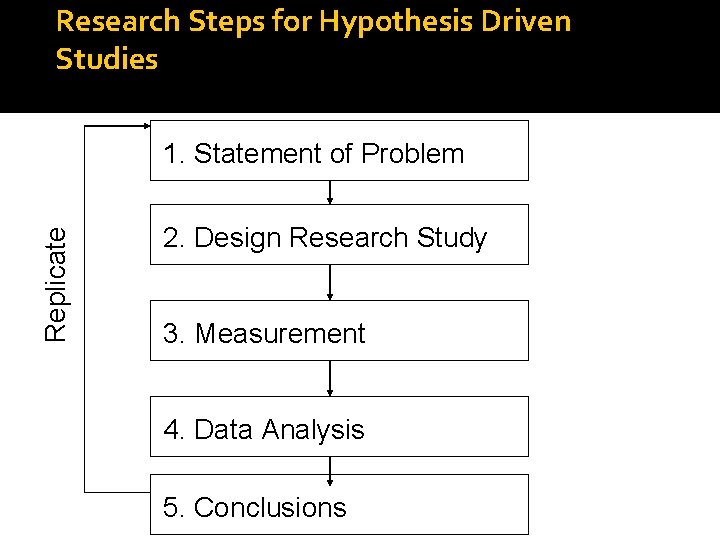
Research Steps for Hypothesis Driven Studies Replicate 1. Statement of Problem 2. Design Research Study 3. Measurement 4. Data Analysis 5. Conclusions
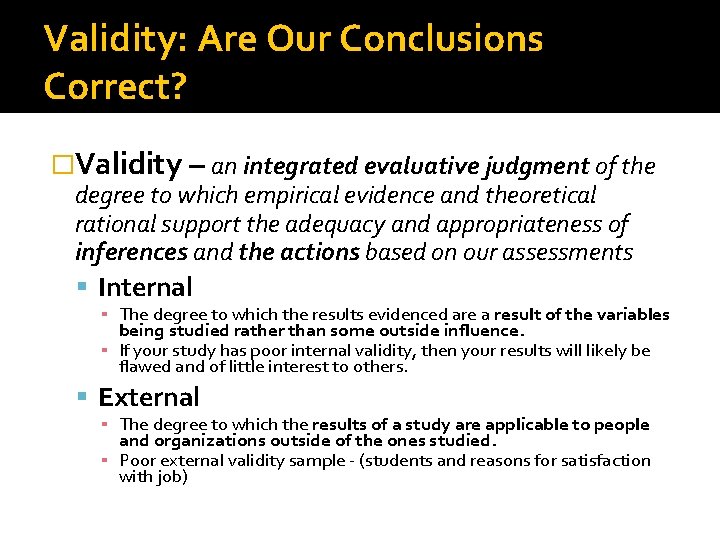
Validity: Are Our Conclusions Correct? �Validity – an integrated evaluative judgment of the degree to which empirical evidence and theoretical rational support the adequacy and appropriateness of inferences and the actions based on our assessments Internal ▪ The degree to which the results evidenced are a result of the variables being studied rather than some outside influence. ▪ If your study has poor internal validity, then your results will likely be flawed and of little interest to others. External ▪ The degree to which the results of a study are applicable to people and organizations outside of the ones studied. ▪ Poor external validity sample - (students and reasons for satisfaction with job)
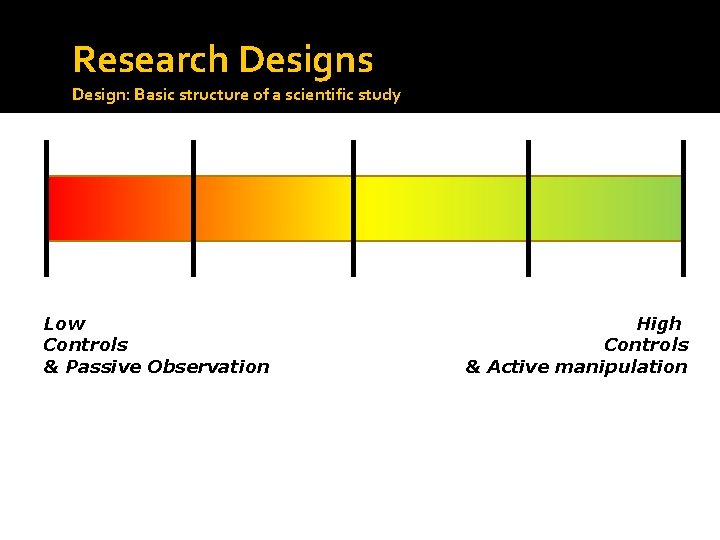
27 Research Designs Design: Basic structure of a scientific study Low Controls & Passive Observation High Controls & Active manipulation
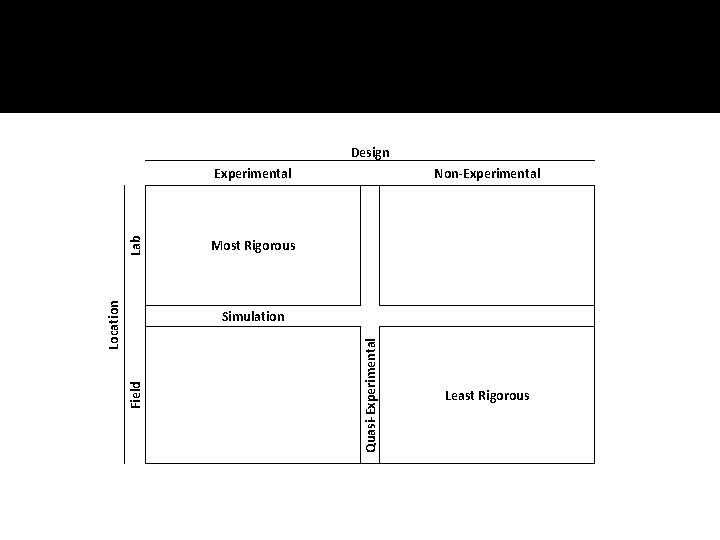
28 Design Lab Experimental Non-Experimental Most Rigorous Location Quasi-Experimental Field Simulation Least Rigorous
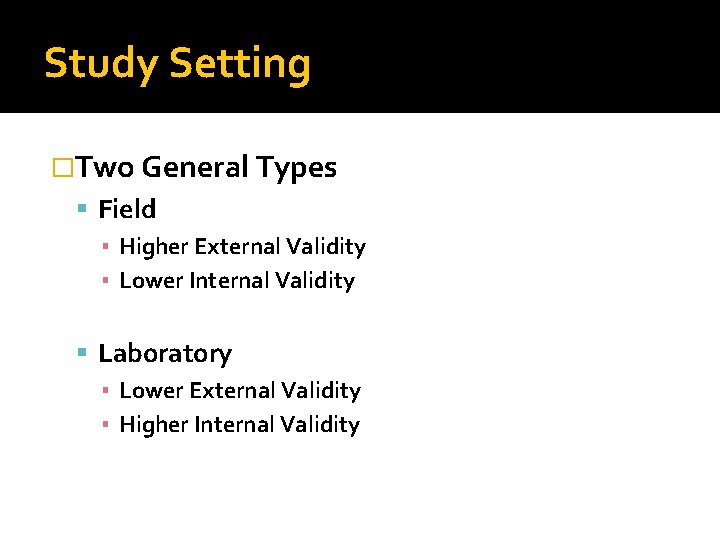
Study Setting �Two General Types Field ▪ Higher External Validity ▪ Lower Internal Validity Laboratory ▪ Lower External Validity ▪ Higher Internal Validity
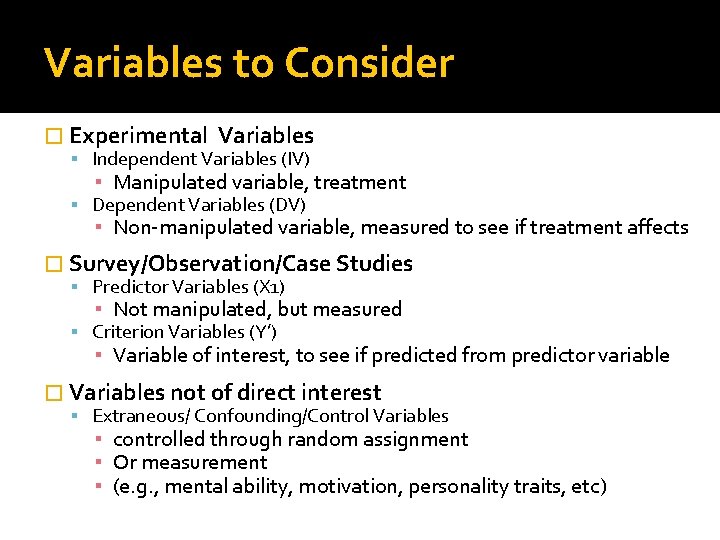
Variables to Consider � Experimental Variables Independent Variables (IV) ▪ Manipulated variable, treatment Dependent Variables (DV) ▪ Non-manipulated variable, measured to see if treatment affects � Survey/Observation/Case Studies Predictor Variables (X 1) ▪ Not manipulated, but measured Criterion Variables (Y’) ▪ Variable of interest, to see if predicted from predictor variable � Variables not of direct interest Extraneous/ Confounding/Control Variables ▪ controlled through random assignment ▪ Or measurement ▪ (e. g. , mental ability, motivation, personality traits, etc)
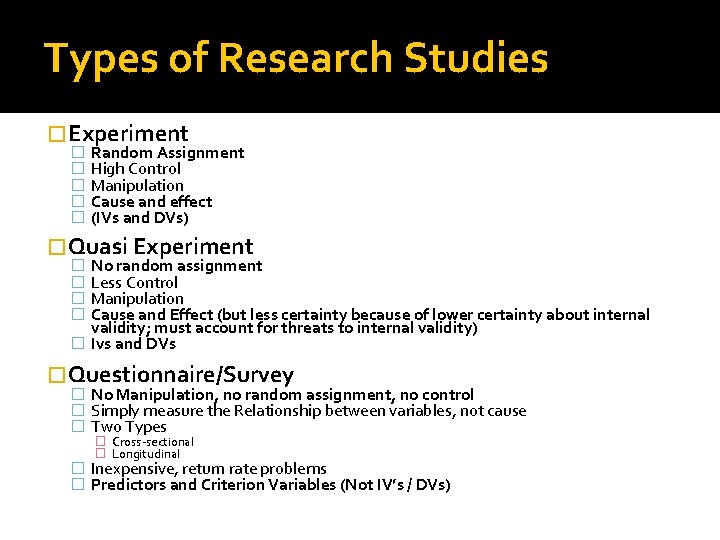
Types of Research Studies � Experiment � � � Random Assignment High Control Manipulation Cause and effect (IVs and DVs) � Quasi Experiment No random assignment Less Control Manipulation Cause and Effect (but less certainty because of lower certainty about internal validity; must account for threats to internal validity) � Ivs and DVs � � � Questionnaire/Survey � No Manipulation, no random assignment, no control � Simply measure the Relationship between variables, not cause � Two Types � Cross-sectional � Longitudinal � Inexpensive, return rate problems � Predictors and Criterion Variables (Not IV’s / DVs)
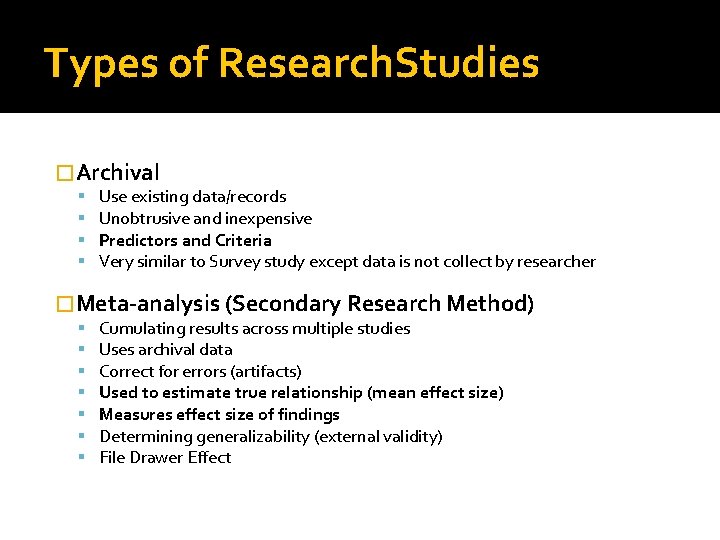
Types of Research. Studies �Archival Use existing data/records Unobtrusive and inexpensive Predictors and Criteria Very similar to Survey study except data is not collect by researcher �Meta-analysis (Secondary Research Method) Cumulating results across multiple studies Uses archival data Correct for errors (artifacts) Used to estimate true relationship (mean effect size) Measures effect size of findings Determining generalizability (external validity) File Drawer Effect
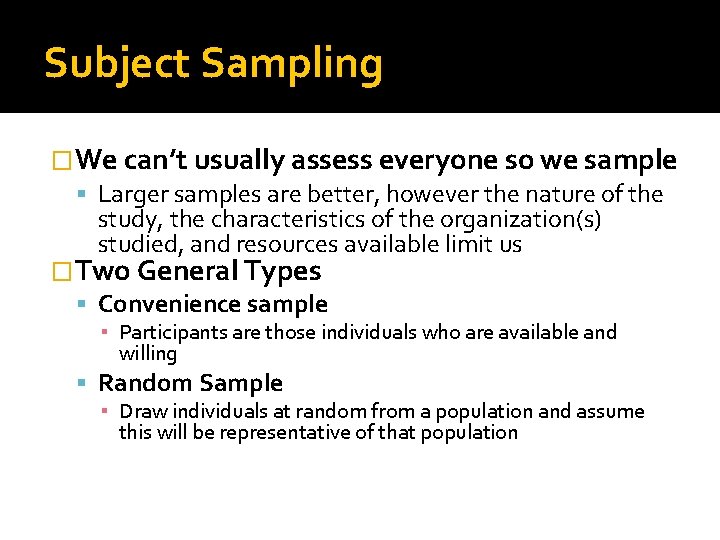
Subject Sampling �We can’t usually assess everyone so we sample Larger samples are better, however the nature of the study, the characteristics of the organization(s) studied, and resources available limit us �Two General Types Convenience sample ▪ Participants are those individuals who are available and willing Random Sample ▪ Draw individuals at random from a population and assume this will be representative of that population
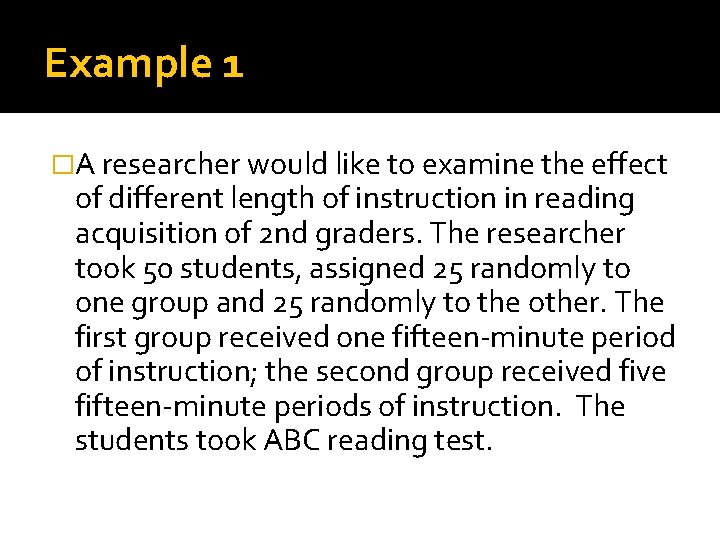
Example 1 �A researcher would like to examine the effect of different length of instruction in reading acquisition of 2 nd graders. The researcher took 50 students, assigned 25 randomly to one group and 25 randomly to the other. The first group received one fifteen-minute period of instruction; the second group received five fifteen-minute periods of instruction. The students took ABC reading test.
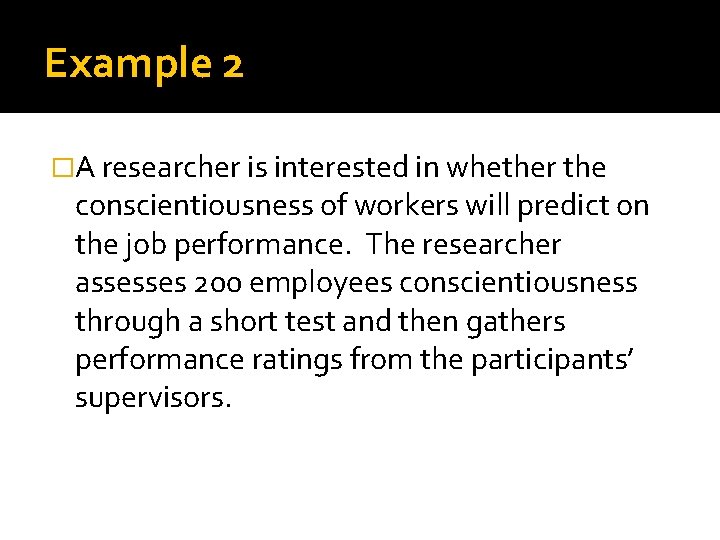
Example 2 �A researcher is interested in whether the conscientiousness of workers will predict on the job performance. The researcher assesses 200 employees conscientiousness through a short test and then gathers performance ratings from the participants’ supervisors.
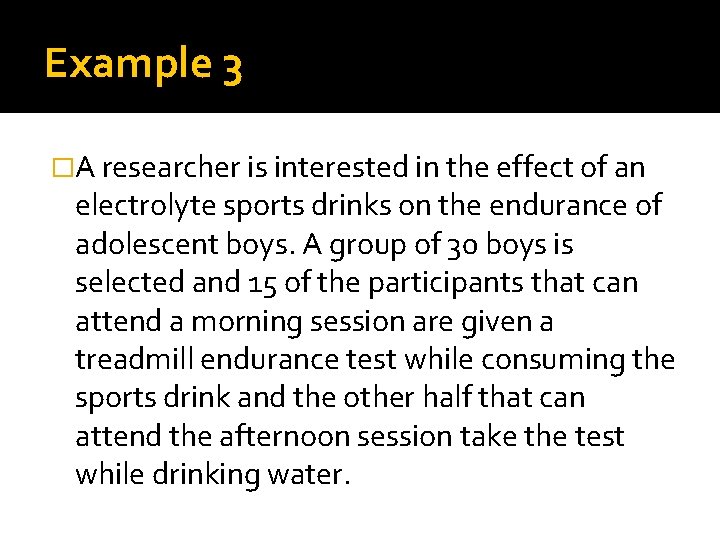
Example 3 �A researcher is interested in the effect of an electrolyte sports drinks on the endurance of adolescent boys. A group of 30 boys is selected and 15 of the participants that can attend a morning session are given a treadmill endurance test while consuming the sports drink and the other half that can attend the afternoon session take the test while drinking water.
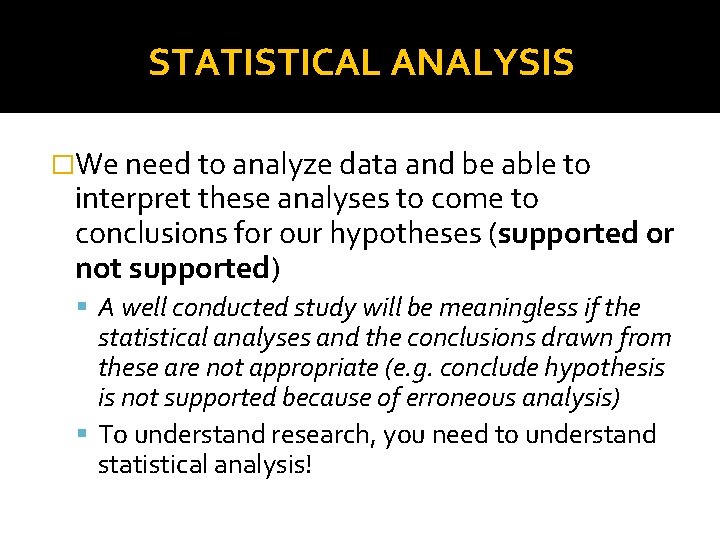
STATISTICAL ANALYSIS �We need to analyze data and be able to interpret these analyses to come to conclusions for our hypotheses (supported or not supported) A well conducted study will be meaningless if the statistical analyses and the conclusions drawn from these are not appropriate (e. g. conclude hypothesis is not supported because of erroneous analysis) To understand research, you need to understand statistical analysis!
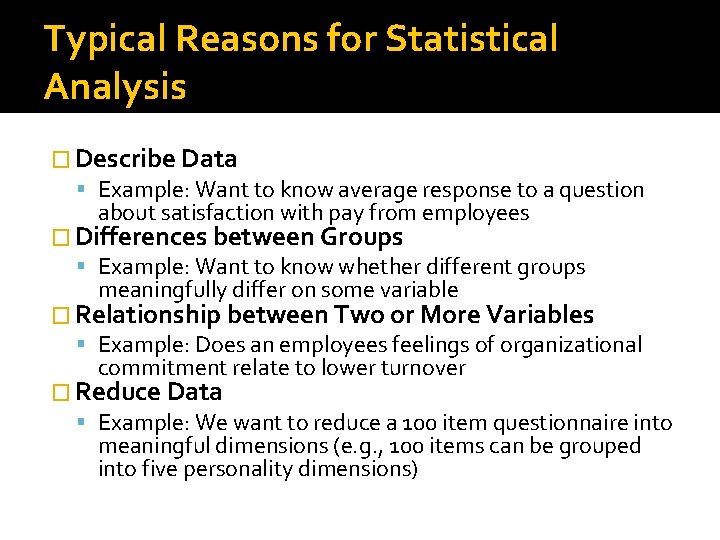
Typical Reasons for Statistical Analysis � Describe Data Example: Want to know average response to a question about satisfaction with pay from employees � Differences between Groups Example: Want to know whether different groups meaningfully differ on some variable � Relationship between Two or More Variables Example: Does an employees feelings of organizational commitment relate to lower turnover � Reduce Data Example: We want to reduce a 100 item questionnaire into meaningful dimensions (e. g. , 100 items can be grouped into five personality dimensions)
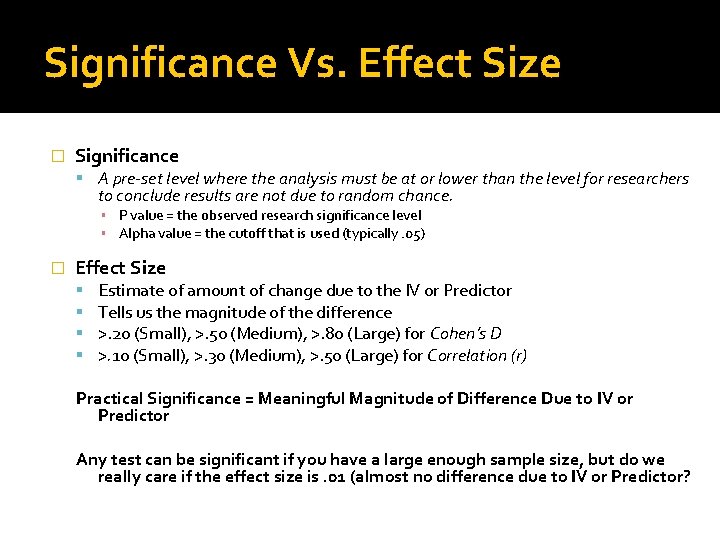
Significance Vs. Effect Size � Significance A pre-set level where the analysis must be at or lower than the level for researchers to conclude results are not due to random chance. ▪ P value = the observed research significance level ▪ Alpha value = the cutoff that is used (typically. 05) � Effect Size Estimate of amount of change due to the IV or Predictor Tells us the magnitude of the difference >. 20 (Small), >. 50 (Medium), >. 80 (Large) for Cohen’s D >. 10 (Small), >. 30 (Medium), >. 50 (Large) for Correlation (r) Practical Significance = Meaningful Magnitude of Difference Due to IV or Predictor Any test can be significant if you have a large enough sample size, but do we really care if the effect size is. 01 (almost no difference due to IV or Predictor?
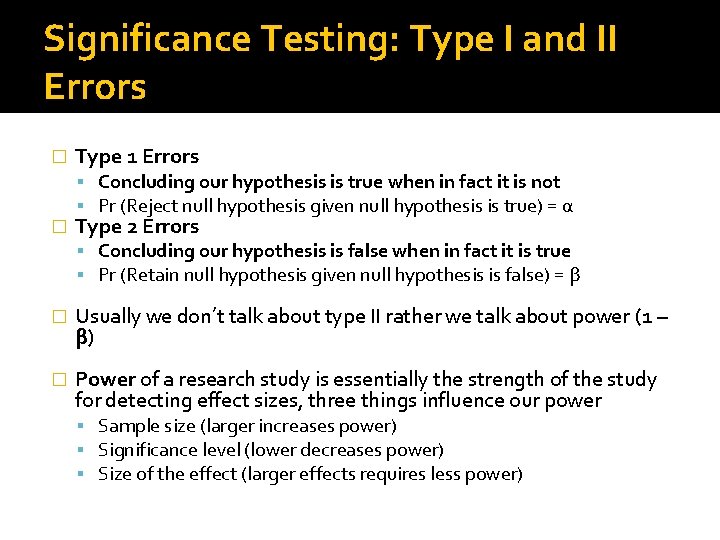
Significance Testing: Type I and II Errors � � Type 1 Errors Concluding our hypothesis is true when in fact it is not Pr (Reject null hypothesis given null hypothesis is true) = α Type 2 Errors Concluding our hypothesis is false when in fact it is true Pr (Retain null hypothesis given null hypothesis is false) = β � Usually we don’t talk about type II rather we talk about power (1 – β) � Power of a research study is essentially the strength of the study for detecting effect sizes, three things influence our power Sample size (larger increases power) Significance level (lower decreases power) Size of the effect (larger effects requires less power)
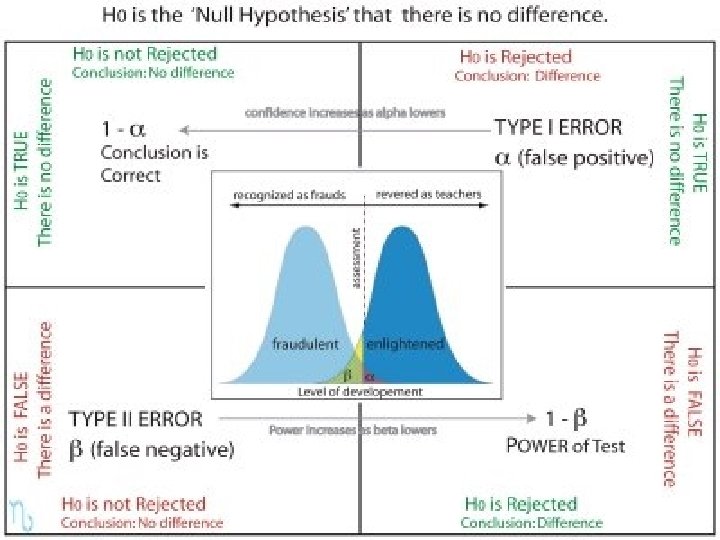
We assume type 1 error if not in this small area of the distribution
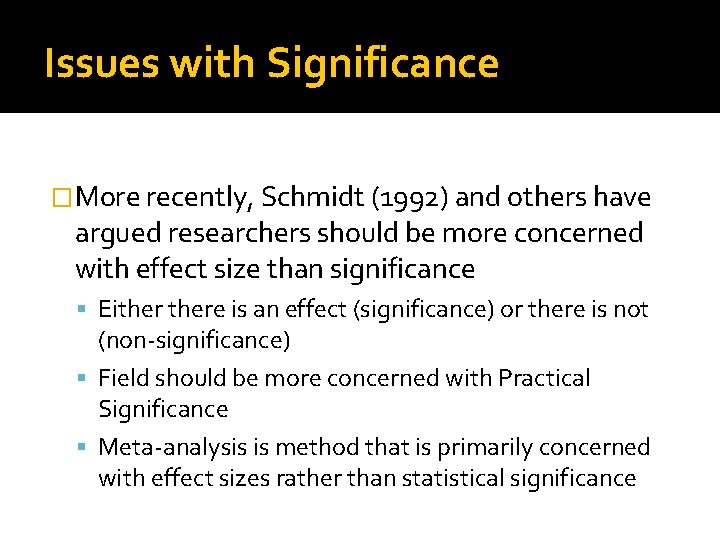
Issues with Significance �More recently, Schmidt (1992) and others have argued researchers should be more concerned with effect size than significance Eithere is an effect (significance) or there is not (non-significance) Field should be more concerned with Practical Significance Meta-analysis is method that is primarily concerned with effect sizes rather than statistical significance
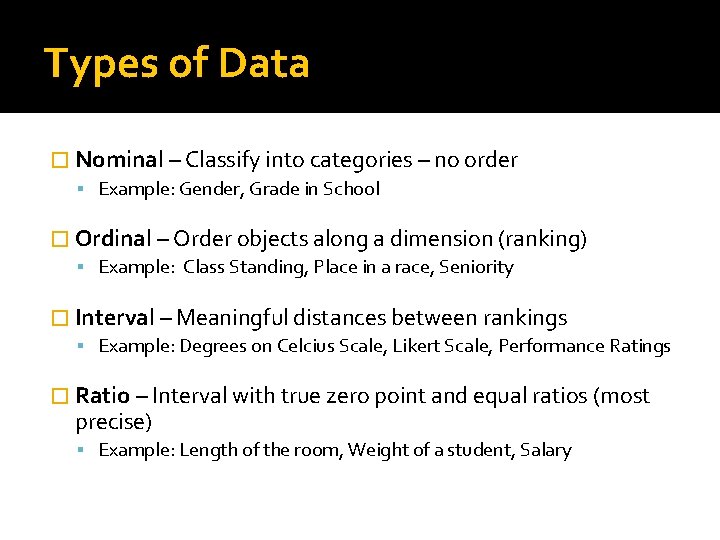
Types of Data � Nominal – Classify into categories – no order Example: Gender, Grade in School � Ordinal – Order objects along a dimension (ranking) Example: Class Standing, Place in a race, Seniority � Interval – Meaningful distances between rankings Example: Degrees on Celcius Scale, Likert Scale, Performance Ratings � Ratio – Interval with true zero point and equal ratios (most precise) Example: Length of the room, Weight of a student, Salary
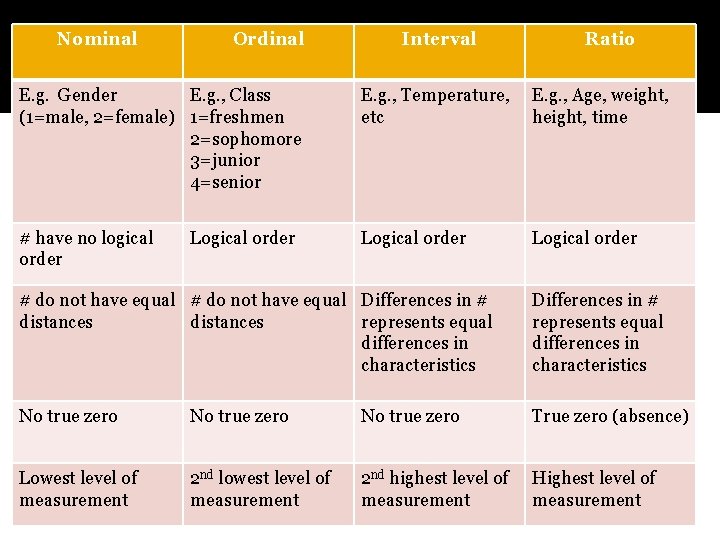
Nominal Ordinal Interval Ratio E. g. Gender E. g. , Class (1=male, 2=female) 1=freshmen 2=sophomore 3=junior 4=senior E. g. , Temperature, etc E. g. , Age, weight, height, time # have no logical order Logical order # do not have equal Differences in # distances represents equal differences in characteristics Differences in # represents equal differences in characteristics No true zero True zero (absence) Lowest level of measurement 2 nd lowest level of measurement 2 nd highest level of measurement Highest level of measurement
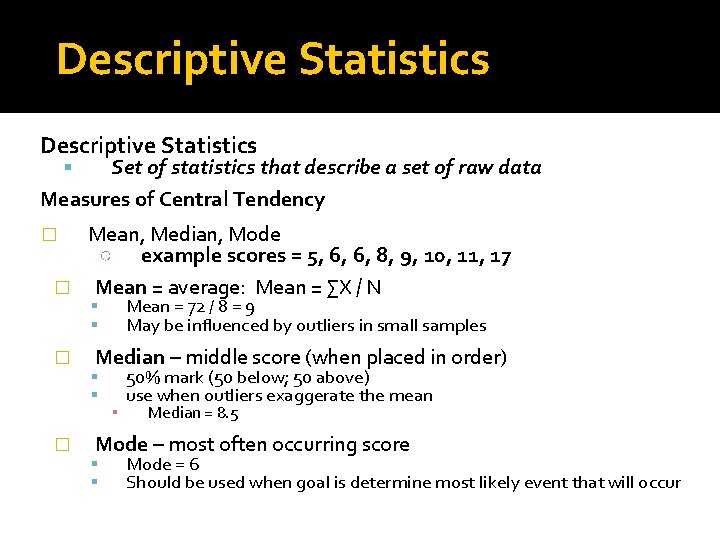
Descriptive Statistics Set of statistics that describe a set of raw data Measures of Central Tendency Mean, Median, Mode ○ example scores = 5, 6, 6, 8, 9, 10, 11, 17 � Mean = average: Mean = ∑X / N � Mean = 72 / 8 = 9 May be influenced by outliers in small samples � Median – middle score (when placed in order) � ▪ 50% mark (50 below; 50 above) use when outliers exaggerate the mean Median = 8. 5 Mode – most often occurring score Mode = 6 Should be used when goal is determine most likely event that will occur
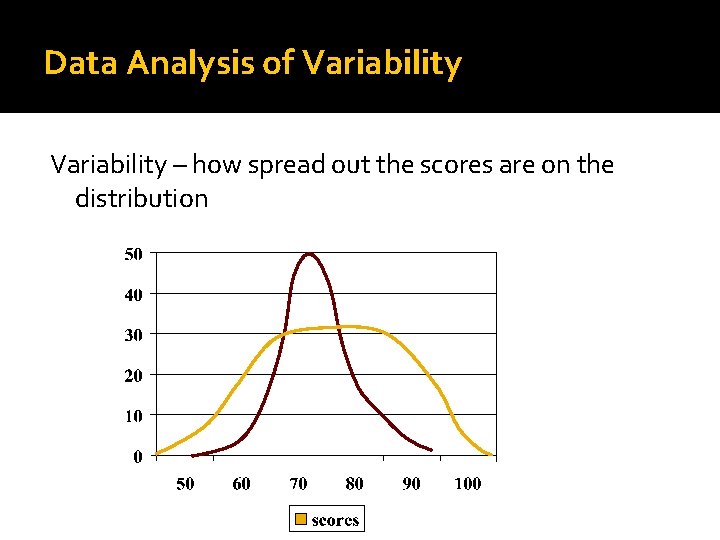
Data Analysis of Variability – how spread out the scores are on the distribution
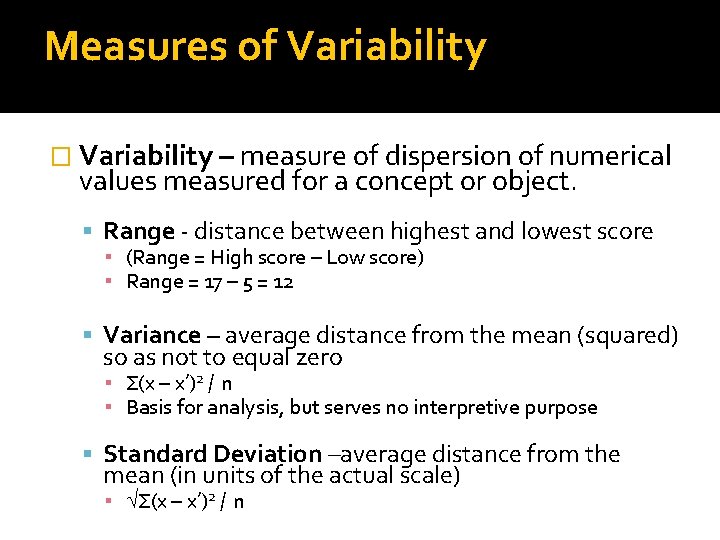
Measures of Variability � Variability – measure of dispersion of numerical values measured for a concept or object. Range - distance between highest and lowest score ▪ (Range = High score – Low score) ▪ Range = 17 – 5 = 12 Variance – average distance from the mean (squared) so as not to equal zero ▪ Σ(x – x’)2 / n ▪ Basis for analysis, but serves no interpretive purpose Standard Deviation –average distance from the mean (in units of the actual scale) ▪ Σ(x – x’)2 / n
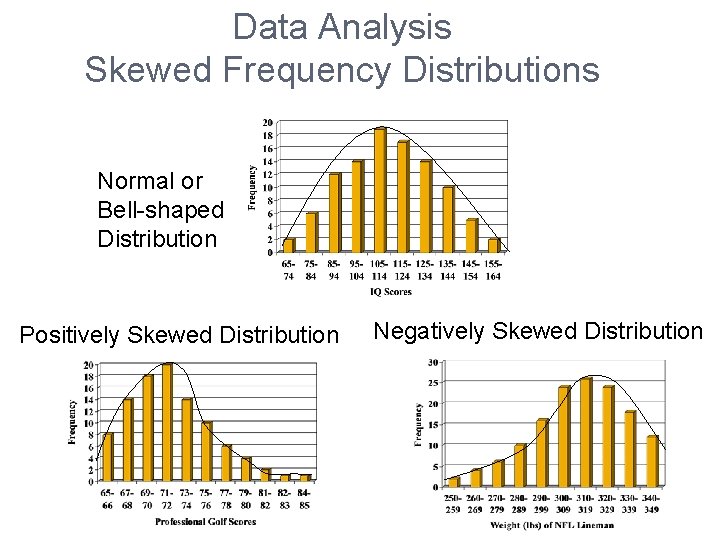
Data Analysis Skewed Frequency Distributions Normal or Bell-shaped Distribution Positively Skewed Distribution Negatively Skewed Distribution
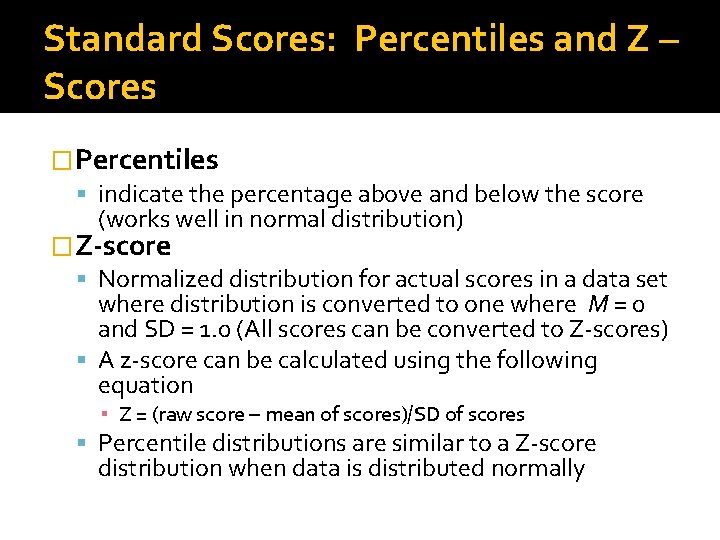
Standard Scores: Percentiles and Z – Scores �Percentiles indicate the percentage above and below the score (works well in normal distribution) �Z-score Normalized distribution for actual scores in a data set where distribution is converted to one where M = 0 and SD = 1. 0 (All scores can be converted to Z-scores) A z-score can be calculated using the following equation ▪ Z = (raw score – mean of scores)/SD of scores Percentile distributions are similar to a Z-score distribution when data is distributed normally
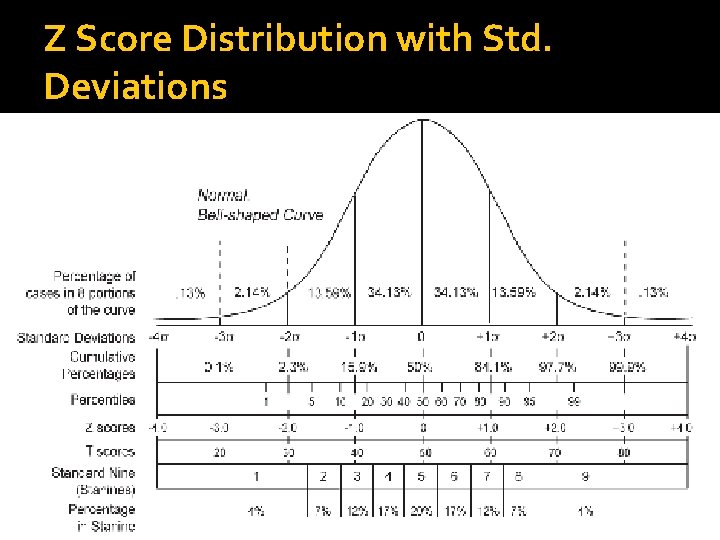
Z Score Distribution with Std. Deviations
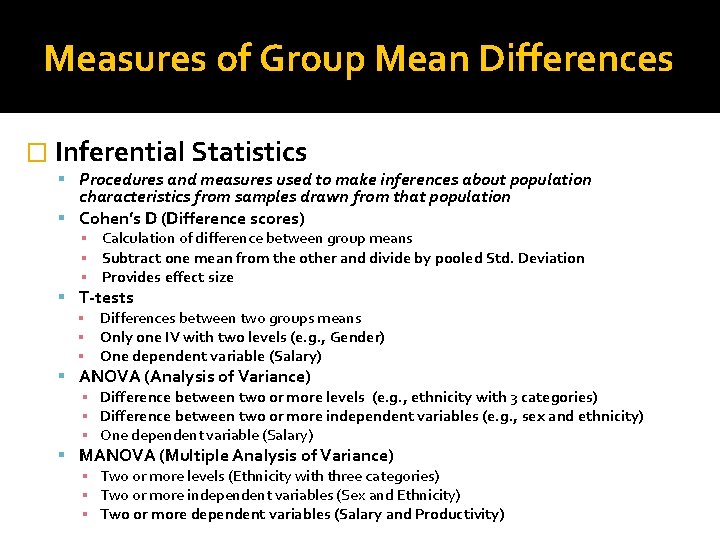
Measures of Group Mean Differences � Inferential Statistics Procedures and measures used to make inferences about population characteristics from samples drawn from that population Cohen’s D (Difference scores) ▪ Calculation of difference between group means ▪ Subtract one mean from the other and divide by pooled Std. Deviation ▪ Provides effect size T-tests ▪ Differences between two groups means ▪ Only one IV with two levels (e. g. , Gender) ▪ One dependent variable (Salary) ANOVA (Analysis of Variance) ▪ Difference between two or more levels (e. g. , ethnicity with 3 categories) ▪ Difference between two or more independent variables (e. g. , sex and ethnicity) ▪ One dependent variable (Salary) MANOVA (Multiple Analysis of Variance) ▪ Two or more levels (Ethnicity with three categories) ▪ Two or more independent variables (Sex and Ethnicity) ▪ Two or more dependent variables (Salary and Productivity)
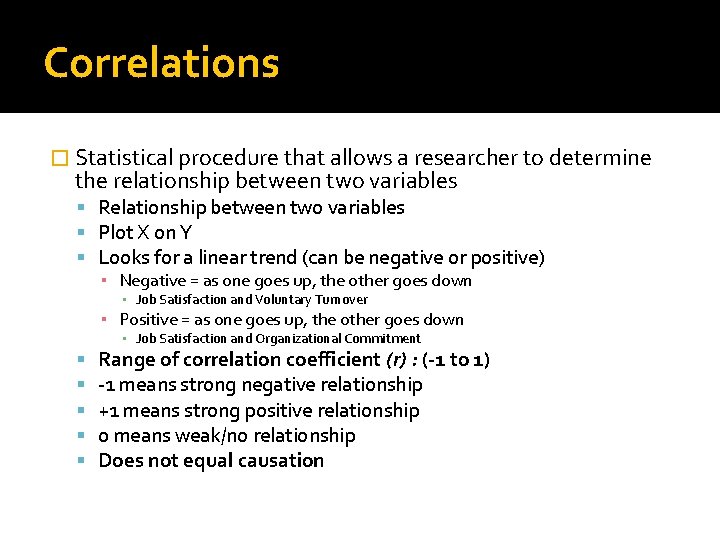
Correlations � Statistical procedure that allows a researcher to determine the relationship between two variables Relationship between two variables Plot X on Y Looks for a linear trend (can be negative or positive) ▪ Negative = as one goes up, the other goes down ▪ Job Satisfaction and Voluntary Turnover ▪ Positive = as one goes up, the other goes down ▪ Job Satisfaction and Organizational Commitment Range of correlation coefficient (r) : (-1 to 1) -1 means strong negative relationship +1 means strong positive relationship 0 means weak/no relationship Does not equal causation
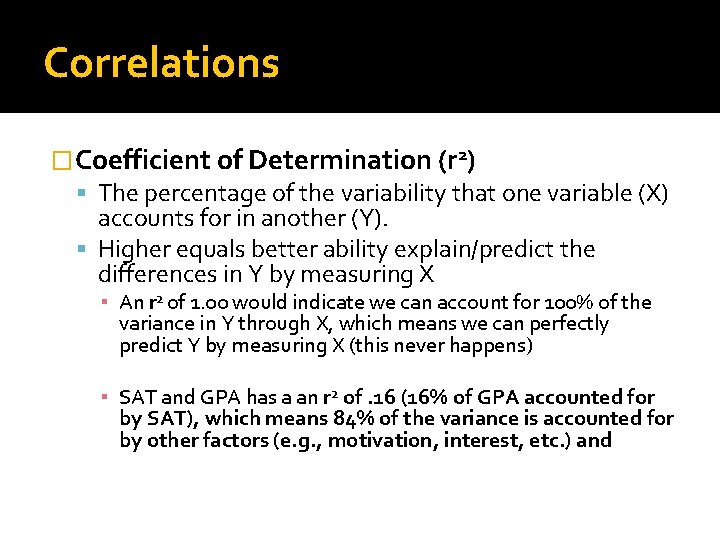
Correlations � Coefficient of Determination (r 2) The percentage of the variability that one variable (X) accounts for in another (Y). Higher equals better ability explain/predict the differences in Y by measuring X ▪ An r 2 of 1. 00 would indicate we can account for 100% of the variance in Y through X, which means we can perfectly predict Y by measuring X (this never happens) ▪ SAT and GPA has a an r 2 of. 16 (16% of GPA accounted for by SAT), which means 84% of the variance is accounted for by other factors (e. g. , motivation, interest, etc. ) and
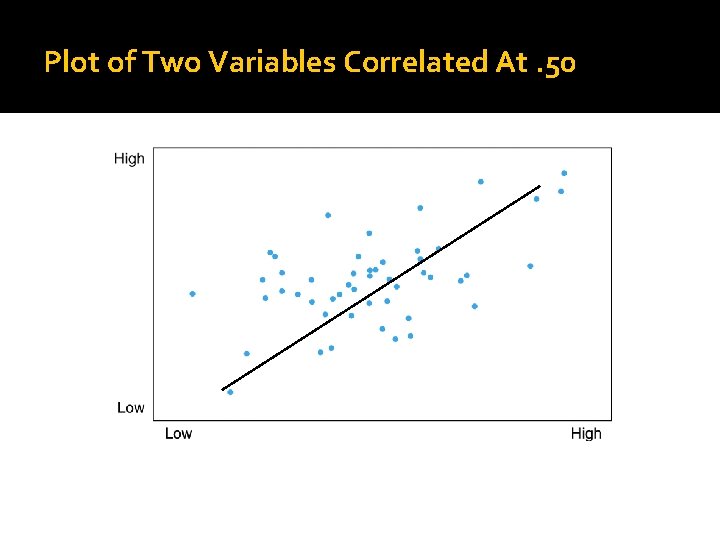
Plot of Two Variables Correlated At. 50
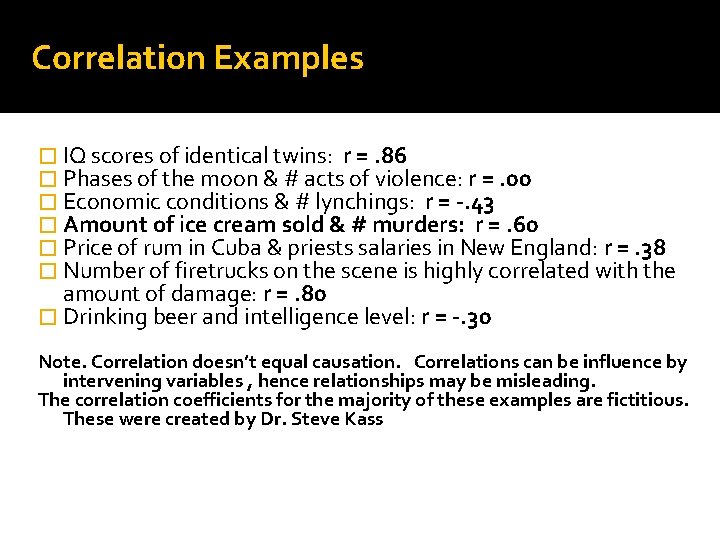
Correlation Examples � IQ scores of identical twins: r =. 86 � Phases of the moon & # acts of violence: r =. 00 � Economic conditions & # lynchings: r = -. 43 � Amount of ice cream sold & # murders: r =. 60 � Price of rum in Cuba & priests salaries in New England: r =. 38 � Number of firetrucks on the scene is highly correlated with the amount of damage: r =. 80 � Drinking beer and intelligence level: r = -. 30 Note. Correlation doesn’t equal causation. Correlations can be influence by intervening variables , hence relationships may be misleading. The correlation coefficients for the majority of these examples are fictitious. These were created by Dr. Steve Kass
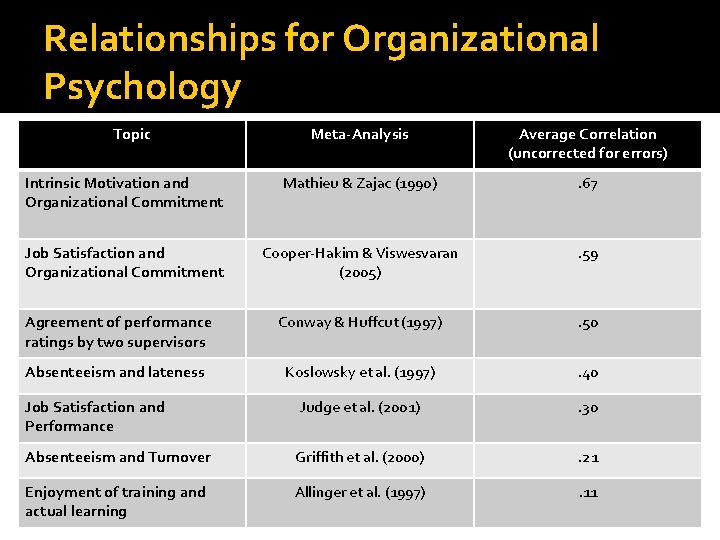
Relationships for Organizational Psychology Topic Meta-Analysis Average Correlation (uncorrected for errors) Intrinsic Motivation and Organizational Commitment Mathieu & Zajac (1990) . 67 Job Satisfaction and Organizational Commitment Cooper-Hakim & Viswesvaran (2005) . 59 Agreement of performance ratings by two supervisors Conway & Huffcut (1997) . 50 Absenteeism and lateness Koslowsky et al. (1997) . 40 Job Satisfaction and Performance Judge et al. (2001) . 30 Absenteeism and Turnover Griffith et al. (2000) . 21 Enjoyment of training and actual learning Allinger et al. (1997) . 11
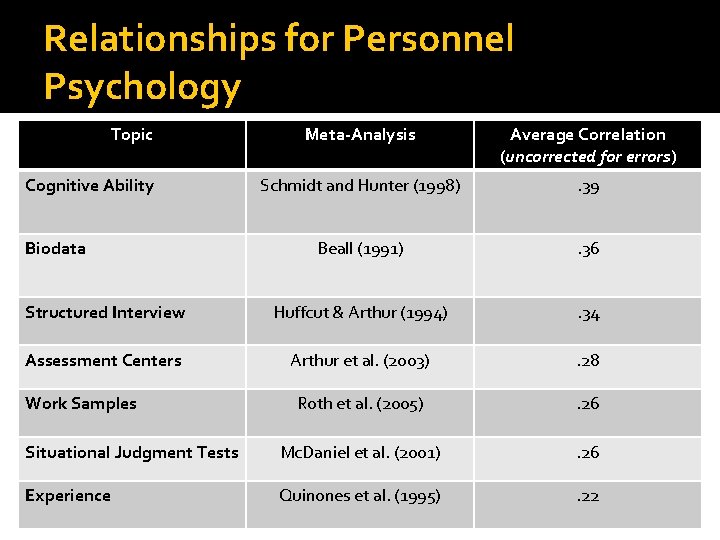
Relationships for Personnel Psychology Topic Meta-Analysis Average Correlation (uncorrected for errors) Schmidt and Hunter (1998) . 39 Beall (1991) . 36 Structured Interview Huffcut & Arthur (1994) . 34 Assessment Centers Arthur et al. (2003) . 28 Roth et al. (2005) . 26 Situational Judgment Tests Mc. Daniel et al. (2001) . 26 Experience Quinones et al. (1995) . 22 Cognitive Ability Biodata Work Samples
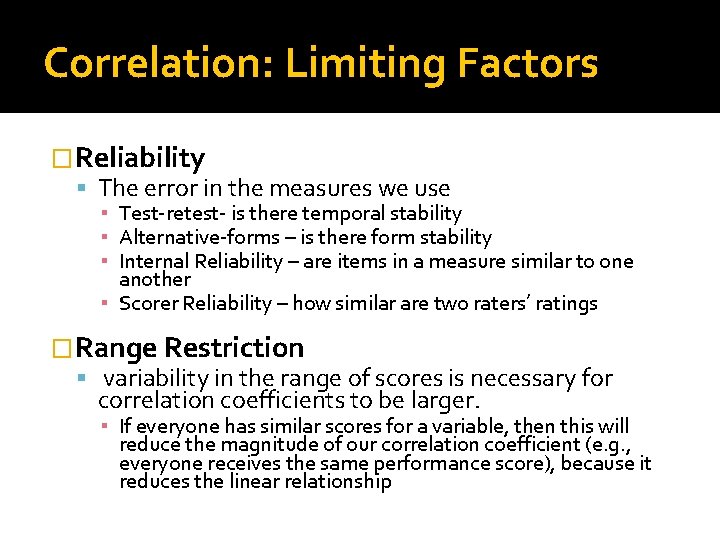
Correlation: Limiting Factors �Reliability The error in the measures we use ▪ Test-retest- is there temporal stability ▪ Alternative-forms – is there form stability ▪ Internal Reliability – are items in a measure similar to one another ▪ Scorer Reliability – how similar are two raters’ ratings �Range Restriction variability in the range of scores is necessary for correlation coefficients to be larger. ▪ If everyone has similar scores for a variable, then this will reduce the magnitude of our correlation coefficient (e. g. , everyone receives the same performance score), because it reduces the linear relationship
Poking and prying with a purpose meaning
Formalized research
Methods of social psychology
Research methods in developmental psychology
Research methods in abnormal psychology
Ocr psychology research methods
Critical thinking chapter 2 answers
Fabrication of wax pattern
What are the sampling methods in psychology
Field psychology definition
Job analysis in industrial psychology
Descriptive methods psychology
Introspection methods of psychology
Positive psychology ap psychology definition
Altruism definition psychology
Social psychology ap psychology
Social psychology is the scientific study of:
Health psychology definition ap psychology
Alan bryman social research methods
Notes on research methods
Elementary dimensions of research
Research design example
Public relations research methods
What are the advantages of primary research
Examples of quantitative research questions and hypotheses
Mixed methods research examples
Business research methods assignment
Descriptive method research
Business research methods mba
Types of consumer research methods
Factor analysis
Advanced business research methods
Midterm exam for applied research methods
Qualitative analysis political science
Slidetodoc.com
Importance of data in legal research
Ontology in qualitative research
Business research process
Zikmund business research methods
Technical writing thesis
Expected results research proposal example
Research methods in project management
Secondary data advantages