QM 222 Class 13 Section D 1 Omitted
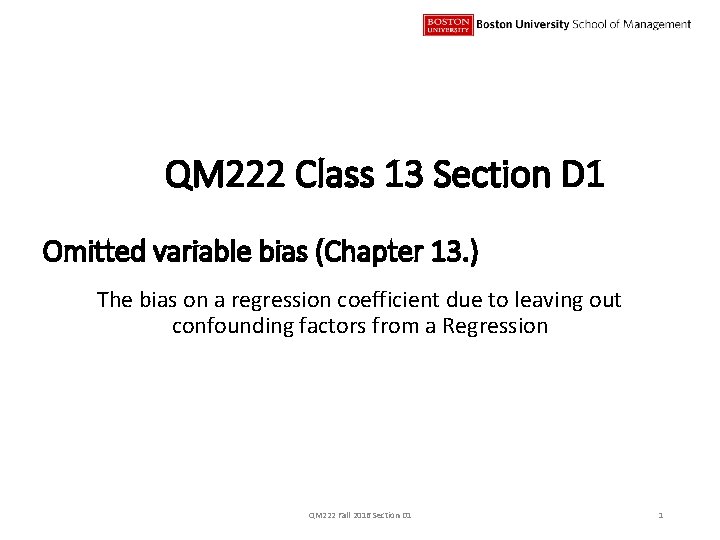
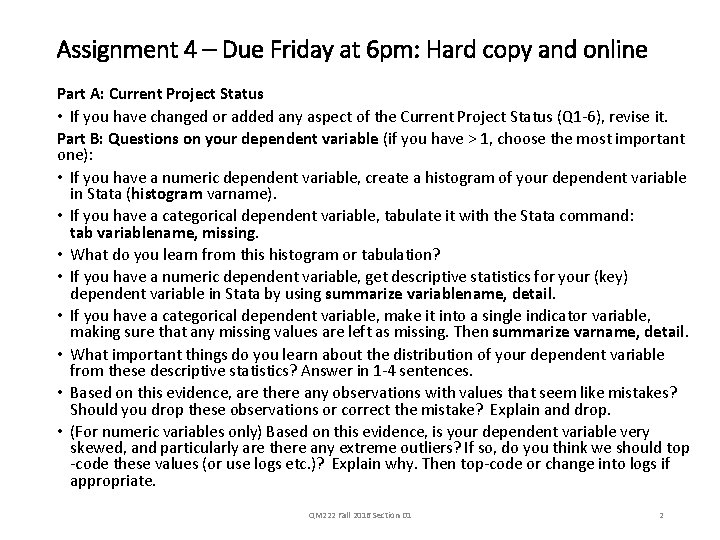
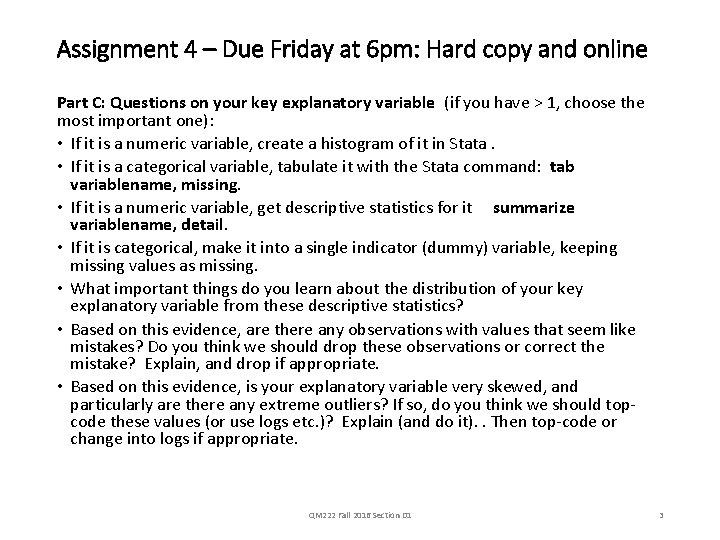
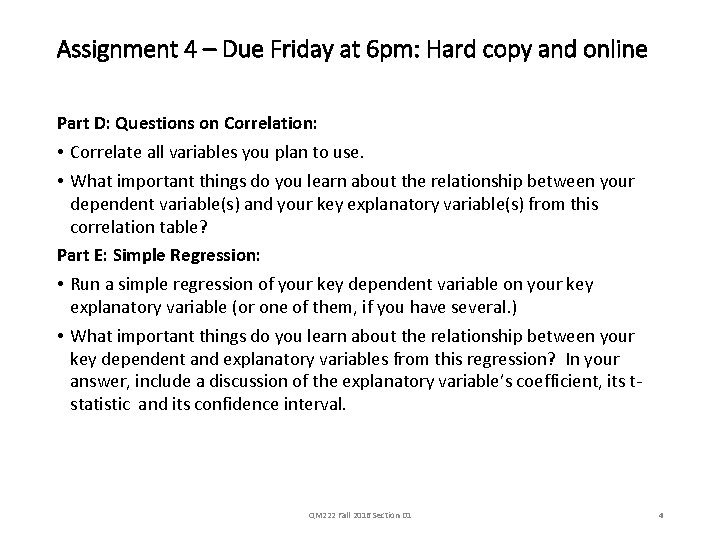
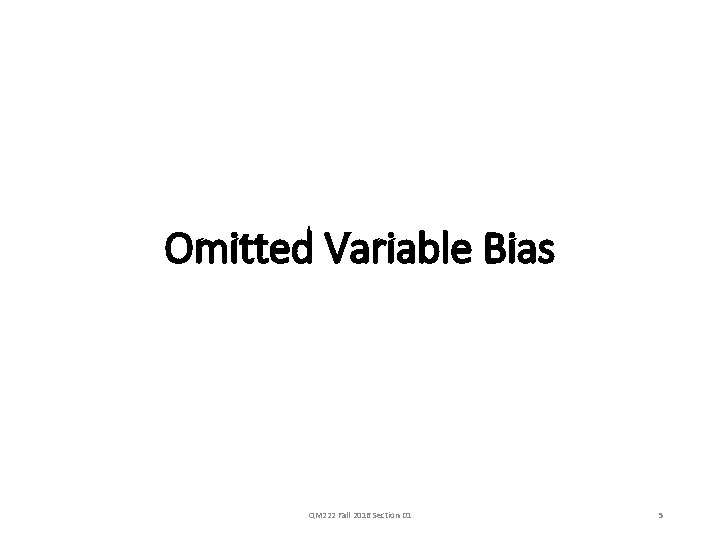
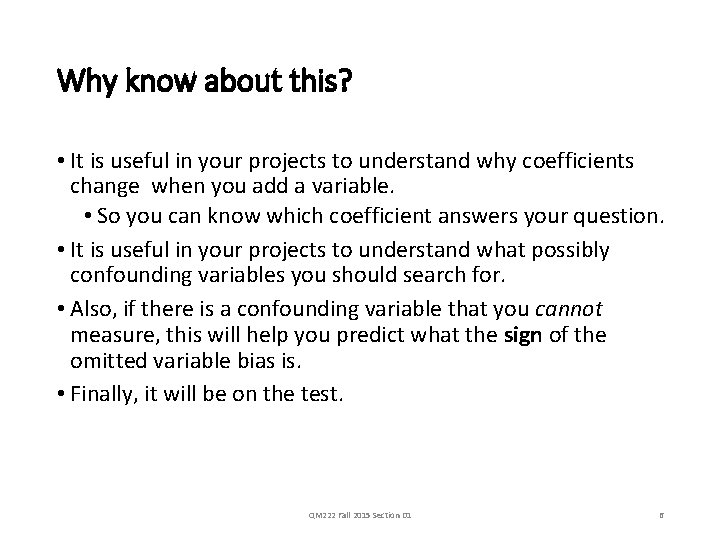
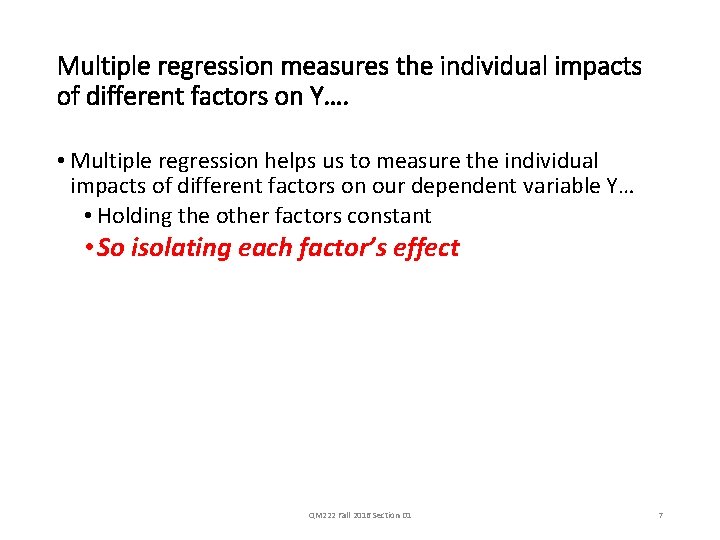
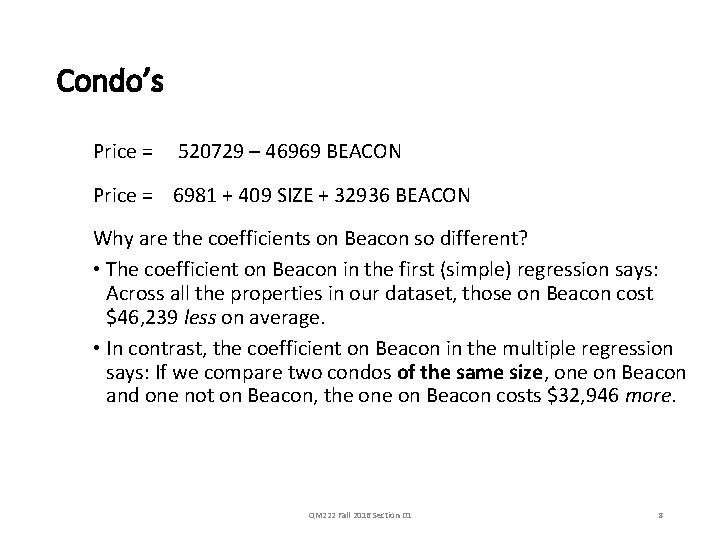
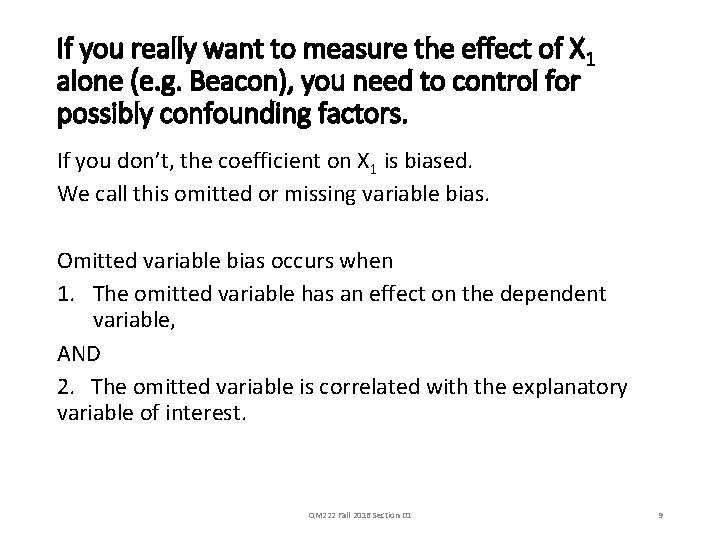
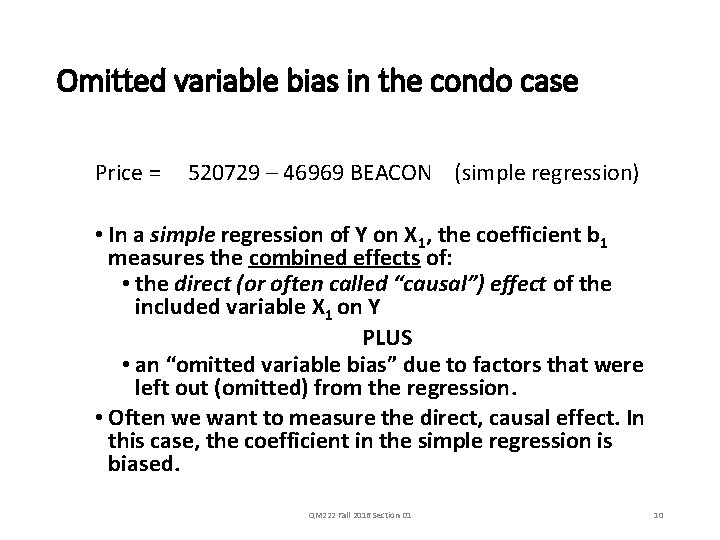
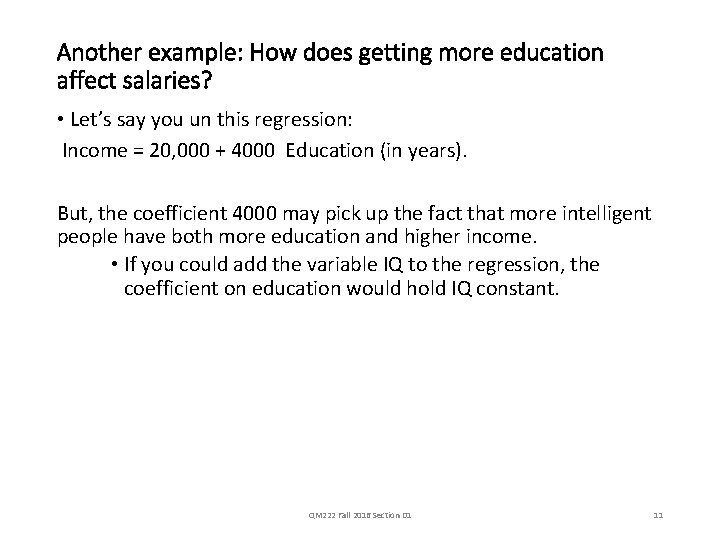
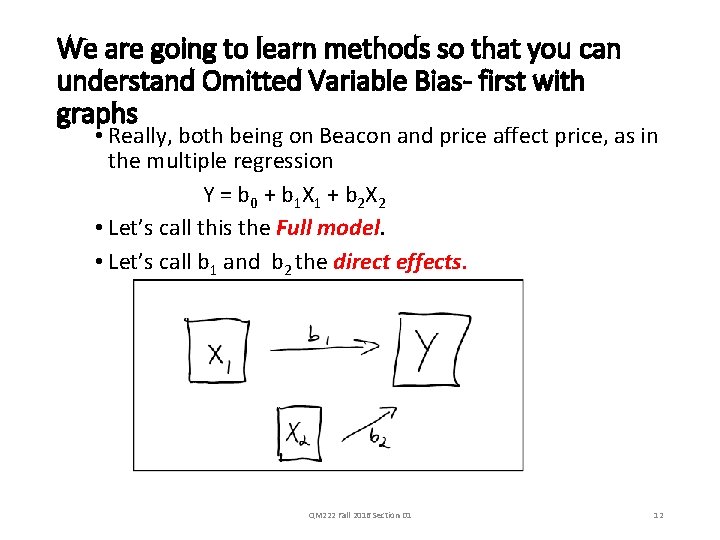
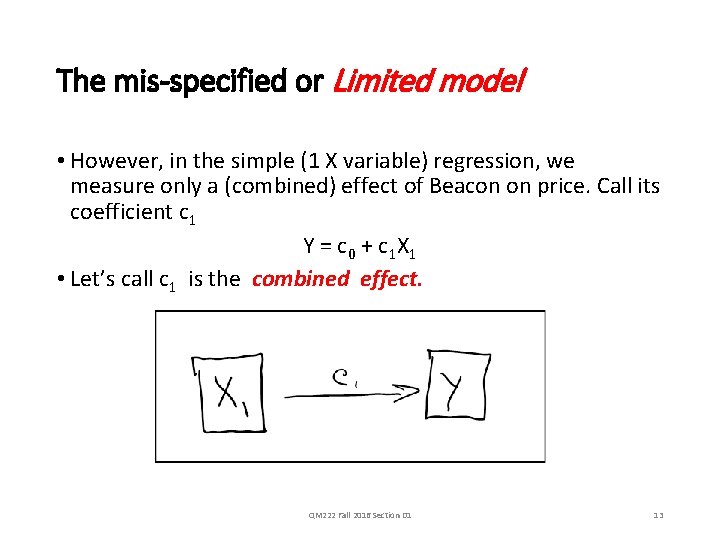
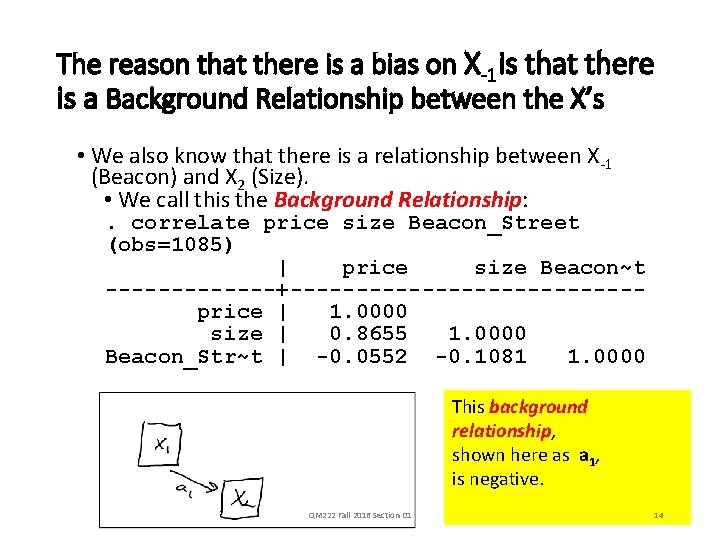
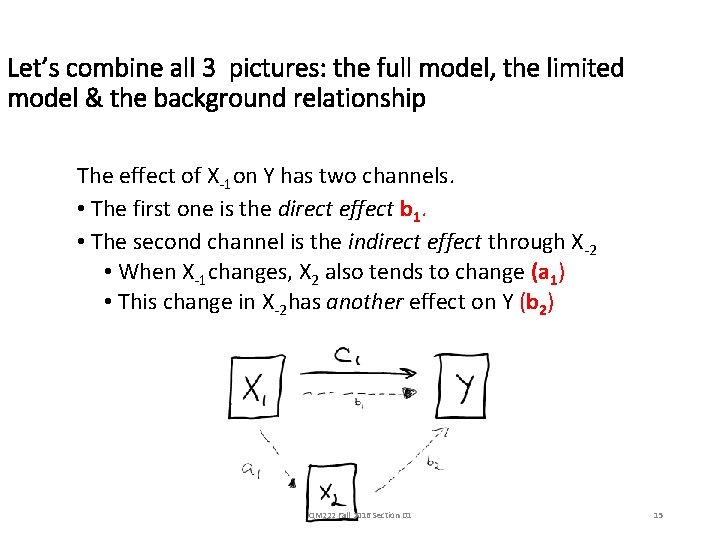
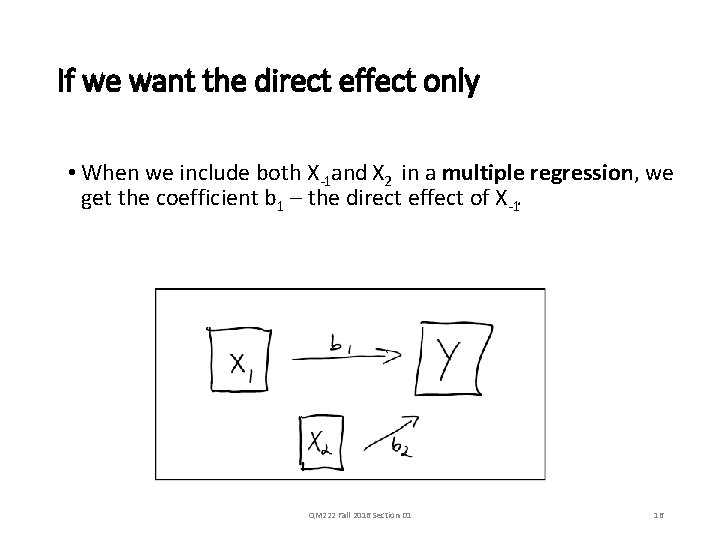
- Slides: 16
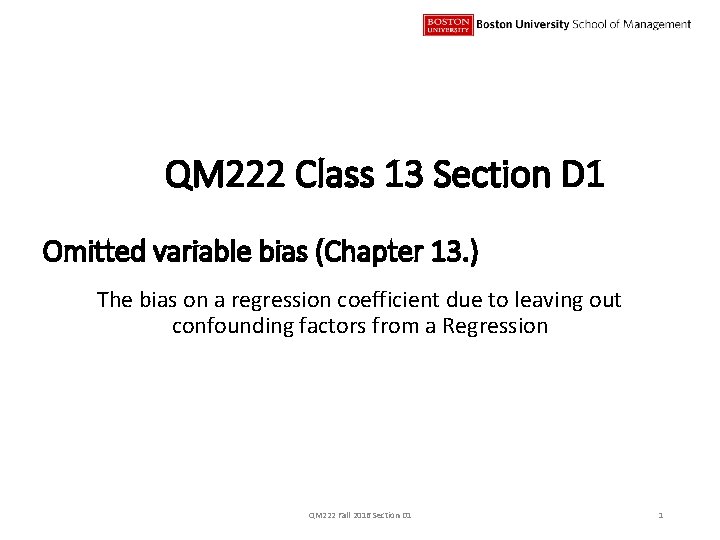
QM 222 Class 13 Section D 1 Omitted variable bias (Chapter 13. ) The bias on a regression coefficient due to leaving out confounding factors from a Regression QM 222 Fall 2016 Section D 1 1
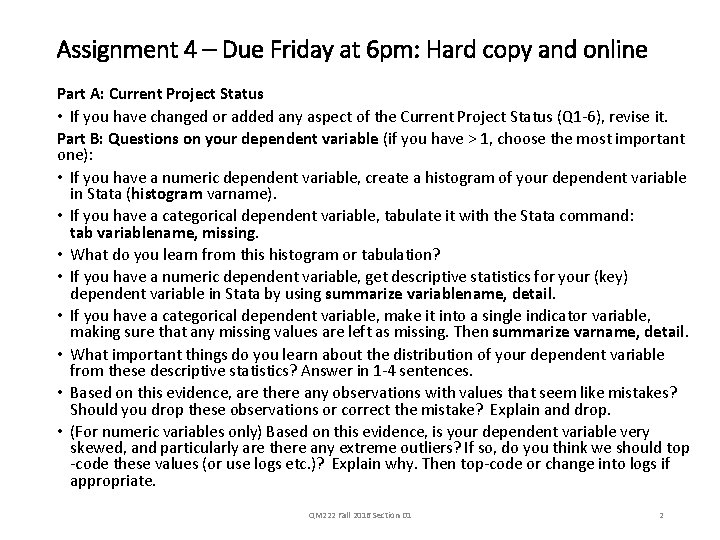
Assignment 4 – Due Friday at 6 pm: Hard copy and online Part A: Current Project Status • If you have changed or added any aspect of the Current Project Status (Q 1 6), revise it. Part B: Questions on your dependent variable (if you have > 1, choose the most important one): • If you have a numeric dependent variable, create a histogram of your dependent variable in Stata (histogram varname). • If you have a categorical dependent variable, tabulate it with the Stata command: tab variablename, missing. • What do you learn from this histogram or tabulation? • If you have a numeric dependent variable, get descriptive statistics for your (key) dependent variable in Stata by using summarize variablename, detail. • If you have a categorical dependent variable, make it into a single indicator variable, making sure that any missing values are left as missing. Then summarize varname, detail. • What important things do you learn about the distribution of your dependent variable from these descriptive statistics? Answer in 1 4 sentences. • Based on this evidence, are there any observations with values that seem like mistakes? Should you drop these observations or correct the mistake? Explain and drop. • (For numeric variables only) Based on this evidence, is your dependent variable very skewed, and particularly are there any extreme outliers? If so, do you think we should top code these values (or use logs etc. )? Explain why. Then top code or change into logs if appropriate. QM 222 Fall 2016 Section D 1 2
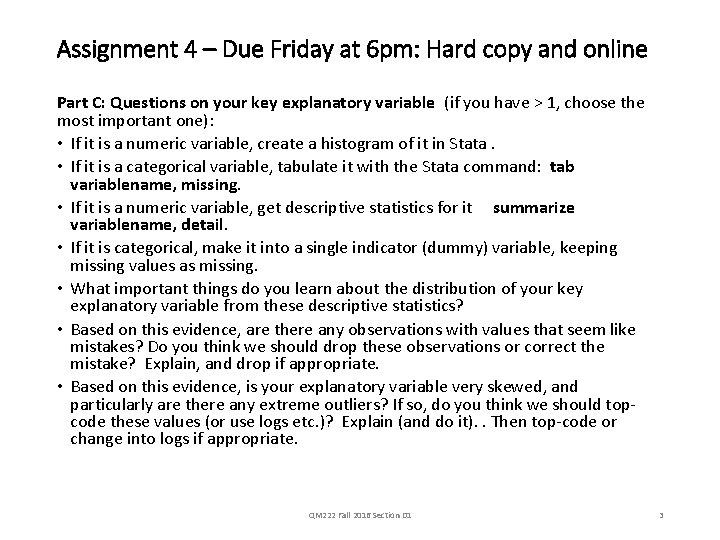
Assignment 4 – Due Friday at 6 pm: Hard copy and online Part C: Questions on your key explanatory variable (if you have > 1, choose the most important one): • If it is a numeric variable, create a histogram of it in Stata. • If it is a categorical variable, tabulate it with the Stata command: tab variablename, missing. • If it is a numeric variable, get descriptive statistics for it summarize variablename, detail. • If it is categorical, make it into a single indicator (dummy) variable, keeping missing values as missing. • What important things do you learn about the distribution of your key explanatory variable from these descriptive statistics? • Based on this evidence, are there any observations with values that seem like mistakes? Do you think we should drop these observations or correct the mistake? Explain, and drop if appropriate. • Based on this evidence, is your explanatory variable very skewed, and particularly are there any extreme outliers? If so, do you think we should top code these values (or use logs etc. )? Explain (and do it). . Then top code or change into logs if appropriate. QM 222 Fall 2016 Section D 1 3
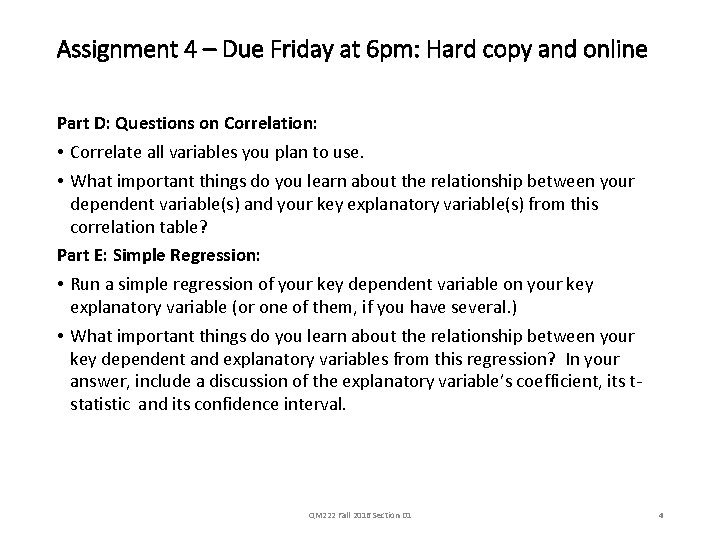
Assignment 4 – Due Friday at 6 pm: Hard copy and online Part D: Questions on Correlation: • Correlate all variables you plan to use. • What important things do you learn about the relationship between your dependent variable(s) and your key explanatory variable(s) from this correlation table? Part E: Simple Regression: • Run a simple regression of your key dependent variable on your key explanatory variable (or one of them, if you have several. ) • What important things do you learn about the relationship between your key dependent and explanatory variables from this regression? In your answer, include a discussion of the explanatory variable’s coefficient, its t statistic and its confidence interval. QM 222 Fall 2016 Section D 1 4
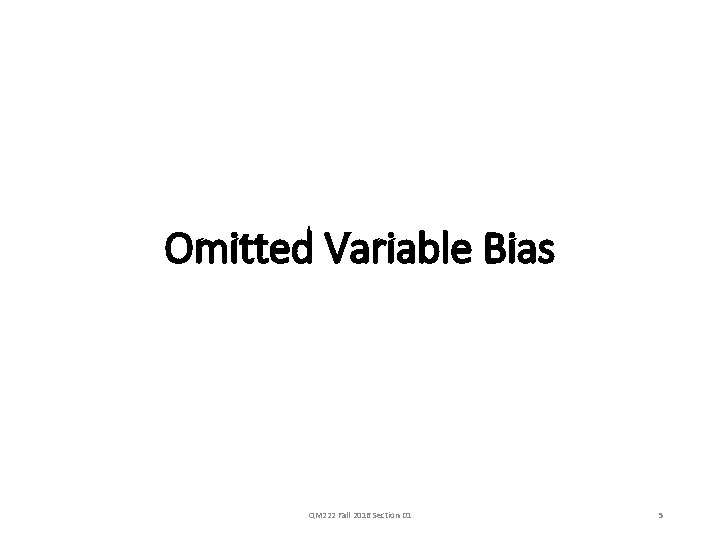
Omitted Variable Bias QM 222 Fall 2016 Section D 1 5
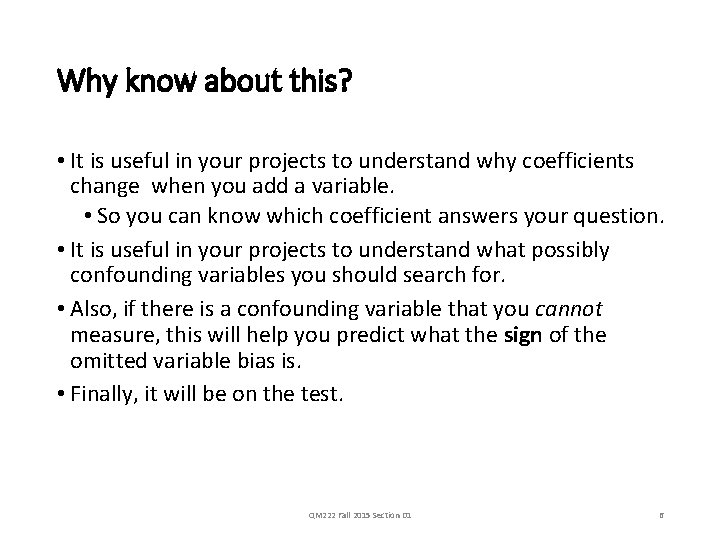
Why know about this? • It is useful in your projects to understand why coefficients change when you add a variable. • So you can know which coefficient answers your question. • It is useful in your projects to understand what possibly confounding variables you should search for. • Also, if there is a confounding variable that you cannot measure, this will help you predict what the sign of the omitted variable bias is. • Finally, it will be on the test. QM 222 Fall 2015 Section D 1 6
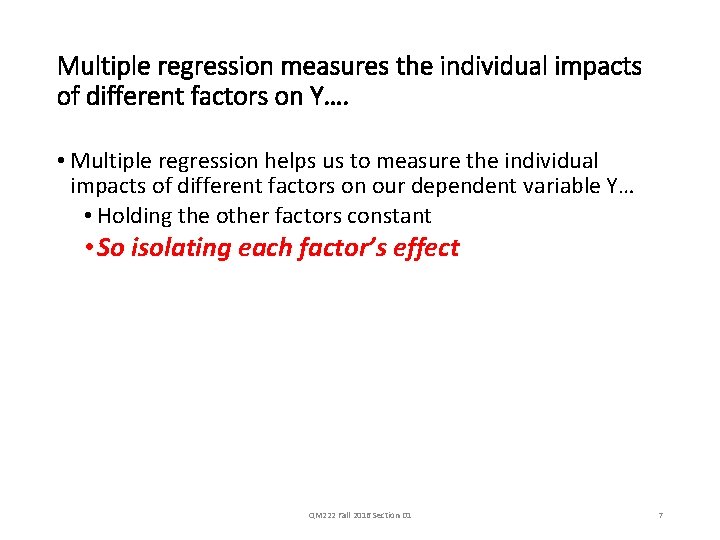
Multiple regression measures the individual impacts of different factors on Y…. • Multiple regression helps us to measure the individual impacts of different factors on our dependent variable Y… • Holding the other factors constant • So isolating each factor’s effect QM 222 Fall 2016 Section D 1 7
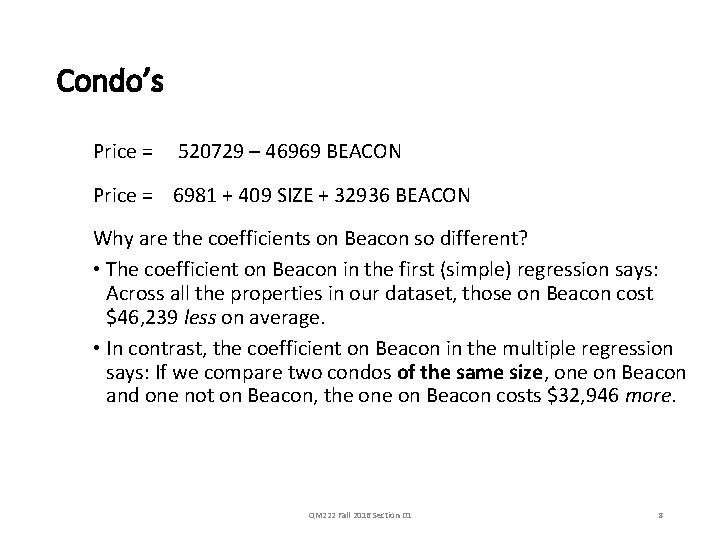
Condo’s Price = 520729 – 46969 BEACON Price = 6981 + 409 SIZE + 32936 BEACON Why are the coefficients on Beacon so different? • The coefficient on Beacon in the first (simple) regression says: Across all the properties in our dataset, those on Beacon cost $46, 239 less on average. • In contrast, the coefficient on Beacon in the multiple regression says: If we compare two condos of the same size, one on Beacon and one not on Beacon, the on Beacon costs $32, 946 more. QM 222 Fall 2016 Section D 1 8
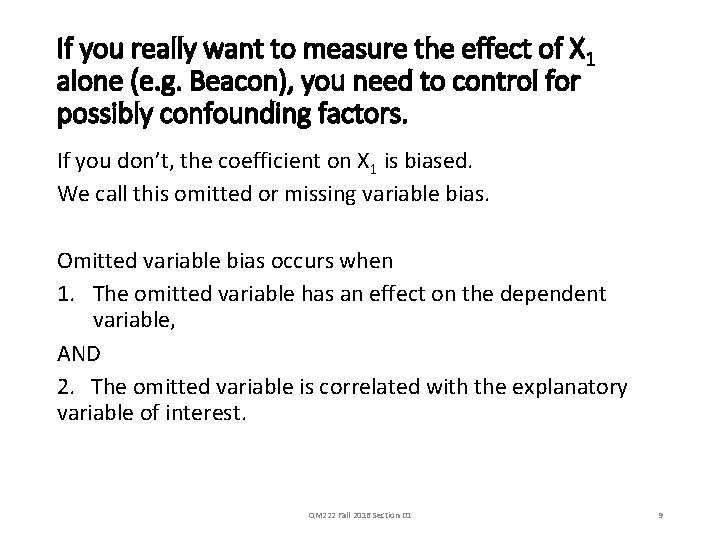
If you really want to measure the effect of X 1 alone (e. g. Beacon), you need to control for possibly confounding factors. If you don’t, the coefficient on X 1 is biased. We call this omitted or missing variable bias. Omitted variable bias occurs when 1. The omitted variable has an effect on the dependent variable, AND 2. The omitted variable is correlated with the explanatory variable of interest. QM 222 Fall 2016 Section D 1 9
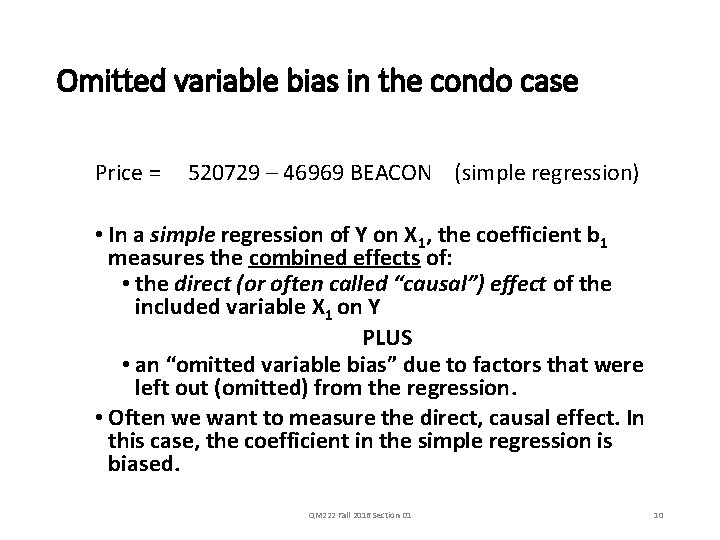
Omitted variable bias in the condo case Price = 520729 – 46969 BEACON (simple regression) • In a simple regression of Y on X 1, the coefficient b 1 measures the combined effects of: • the direct (or often called “causal”) effect of the included variable X 1 on Y PLUS • an “omitted variable bias” due to factors that were left out (omitted) from the regression. • Often we want to measure the direct, causal effect. In this case, the coefficient in the simple regression is biased. QM 222 Fall 2016 Section D 1 10
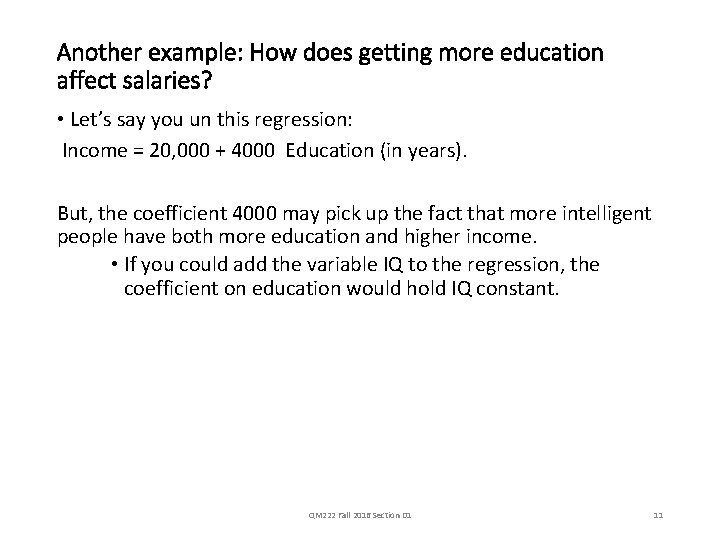
Another example: How does getting more education affect salaries? • Let’s say you un this regression: Income = 20, 000 + 4000 Education (in years). But, the coefficient 4000 may pick up the fact that more intelligent people have both more education and higher income. • If you could add the variable IQ to the regression, the coefficient on education would hold IQ constant. QM 222 Fall 2016 Section D 1 11
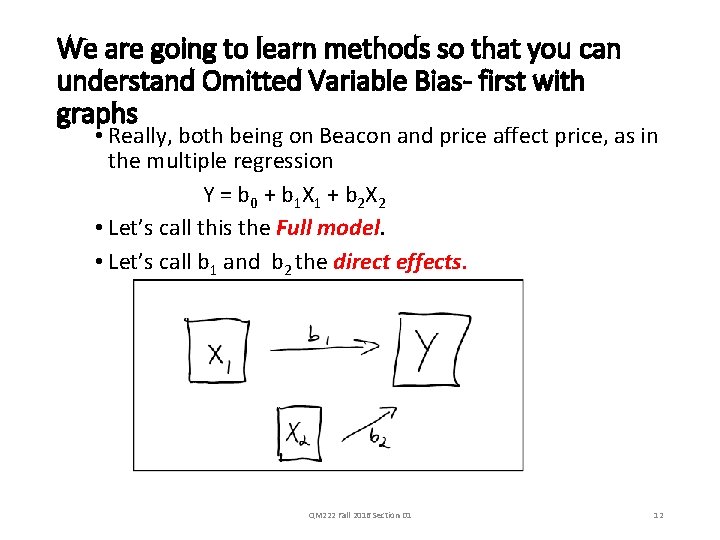
We are going to learn methods so that you can understand Omitted Variable Bias first with graphs • Really, both being on Beacon and price affect price, as in the multiple regression Y = b 0 + b 1 X 1 + b 2 X 2 • Let’s call this the Full model. • Let’s call b 1 and b 2 the direct effects. QM 222 Fall 2016 Section D 1 12
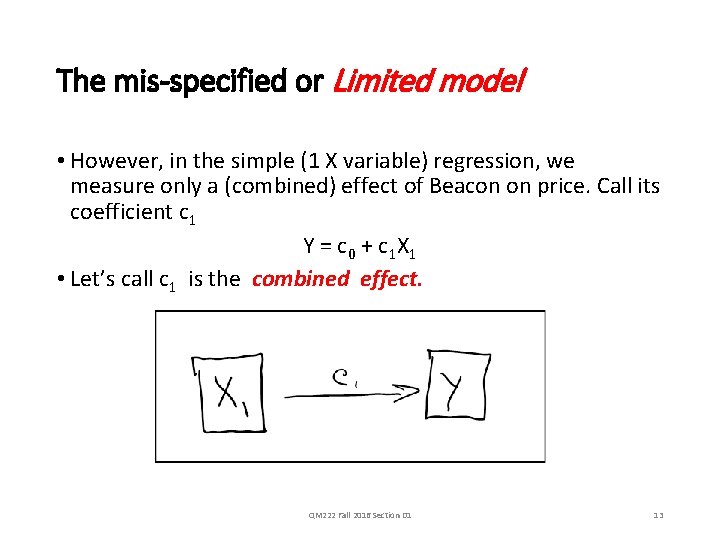
The mis specified or Limited model • However, in the simple (1 X variable) regression, we measure only a (combined) effect of Beacon on price. Call its coefficient c 1 Y = c 0 + c 1 X 1 • Let’s call c 1 is the combined effect. QM 222 Fall 2016 Section D 1 13
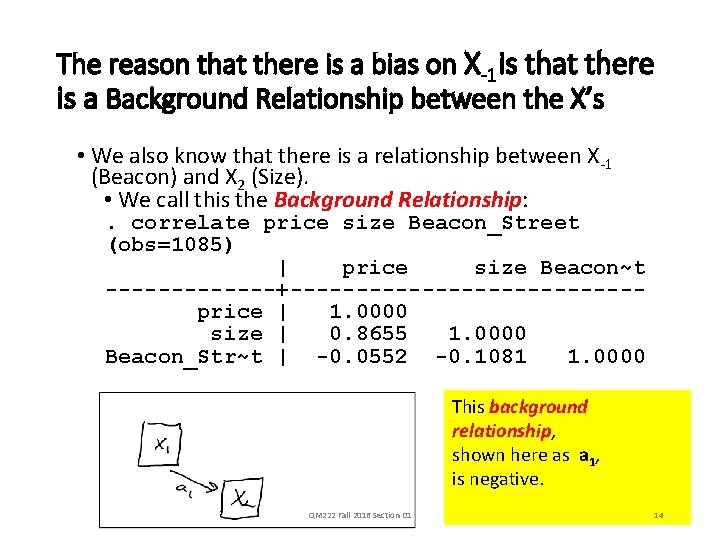
The reason that there is a bias on X 1 is that there is a Background Relationship between the X’s • We also know that there is a relationship between X 1 (Beacon) and X 2 (Size). • We call this the Background Relationship: . correlate price size Beacon_Street (obs=1085) | price size Beacon~t -------+-------------price | 1. 0000 size | 0. 8655 1. 0000 Beacon_Str~t | -0. 0552 -0. 1081 1. 0000 This background relationship, shown here as a 1, is negative. QM 222 Fall 2016 Section D 1 14
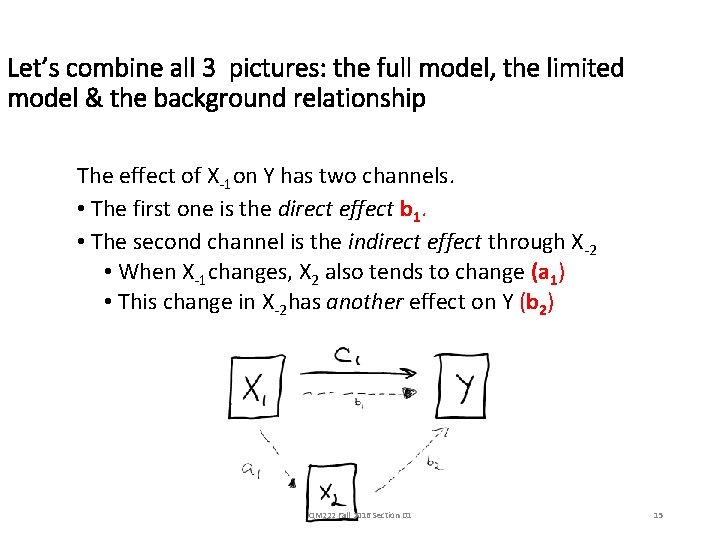
Let’s combine all 3 pictures: the full model, the limited model & the background relationship The effect of X 1 on Y has two channels. • The first one is the direct effect b 1. • The second channel is the indirect effect through X 2. • When X 1 changes, X 2 also tends to change (a 1) • This change in X 2 has another effect on Y (b 2) QM 222 Fall 2016 Section D 1 15
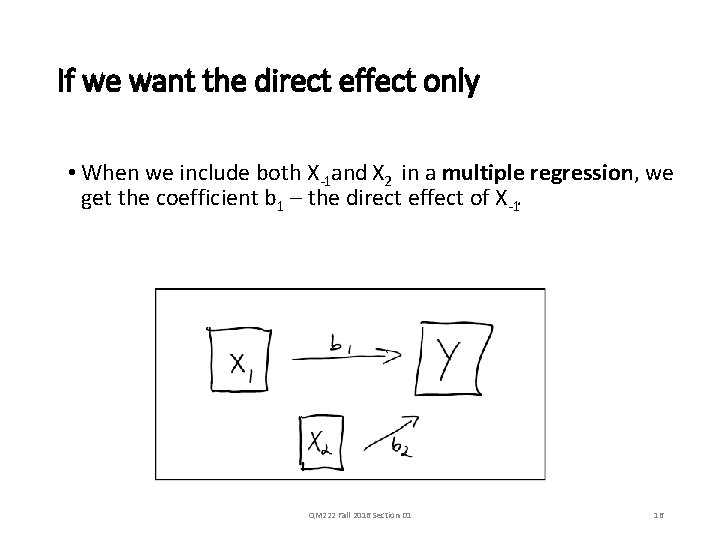
If we want the direct effect only • When we include both X 1 and X 2 in a multiple regression, we get the coefficient b 1 – the direct effect of X 1. QM 222 Fall 2016 Section D 1 16
Opendns family shield
Class is omitted
Texas transportation code 521.025
Revolved sections
What symbol is commonly used for half-section view?
How to detect omitted variable bias
Multiple regression analysis estimation
Omitted variable bias
Omitted variable bias
With the omitted results
Significant omitted information
Xxaxis matlab 222
Pineer species
Império do jogo do bicho
Log222
Funk radius
Mism-222