Potential Unintended Consequences of AI NAM Digital Learning
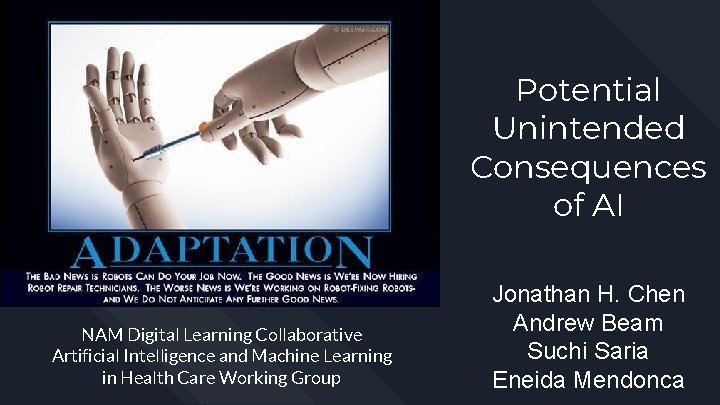
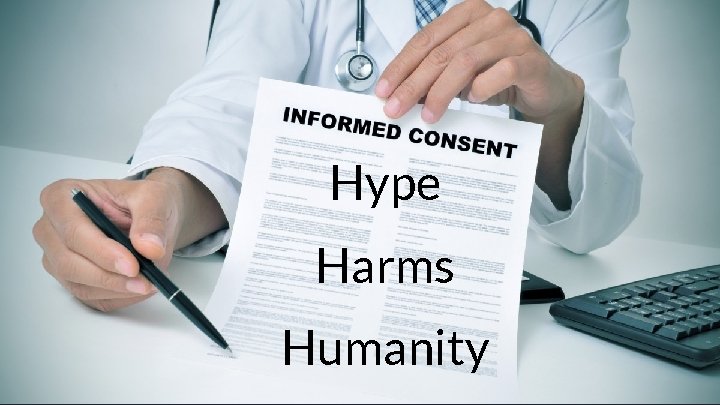
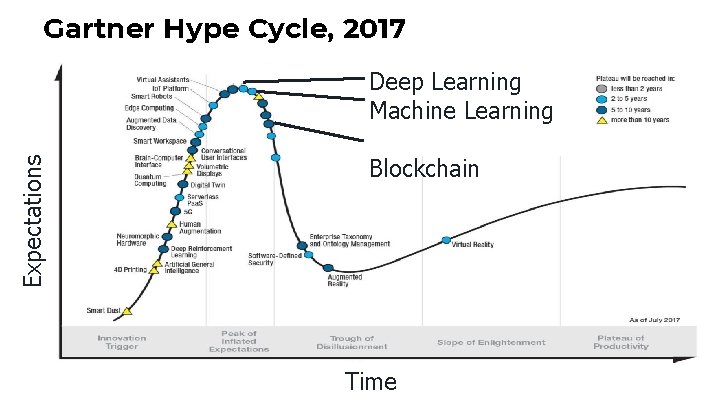
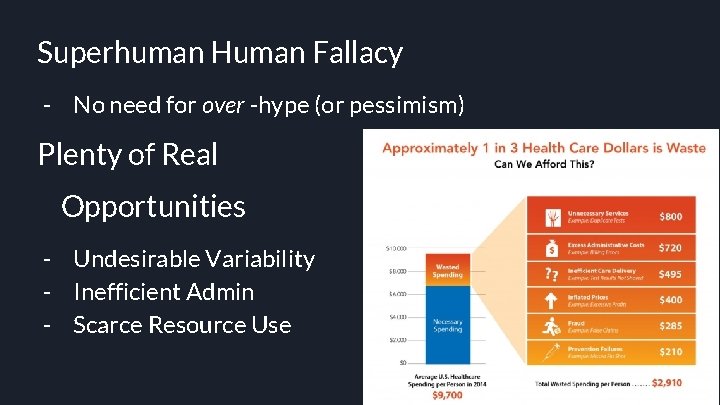
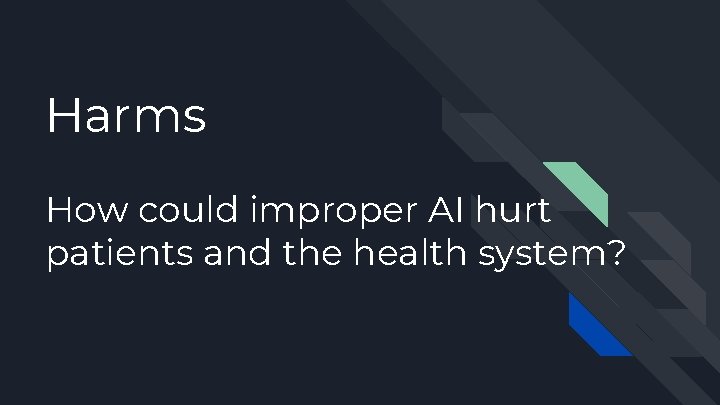
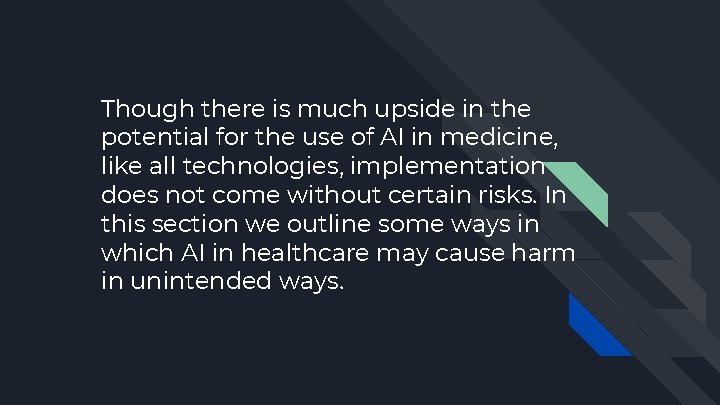
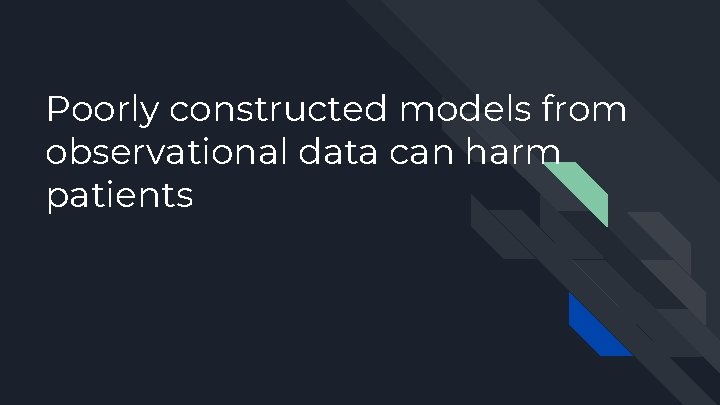
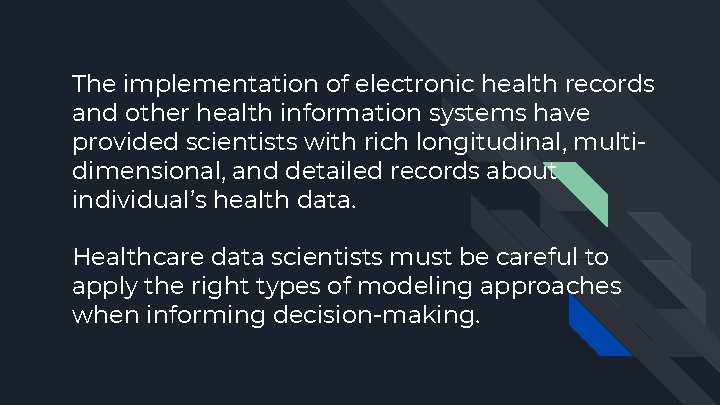
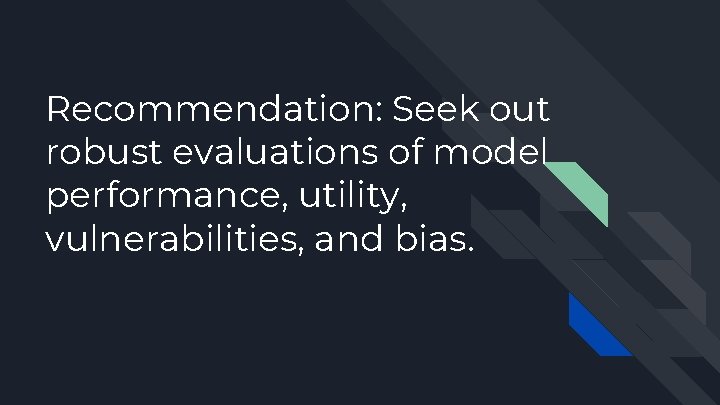
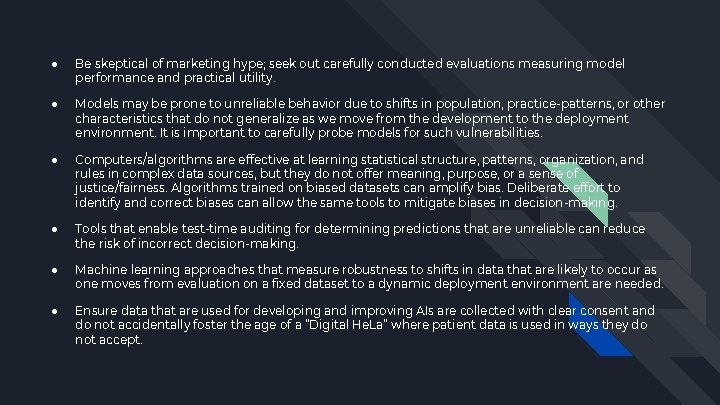
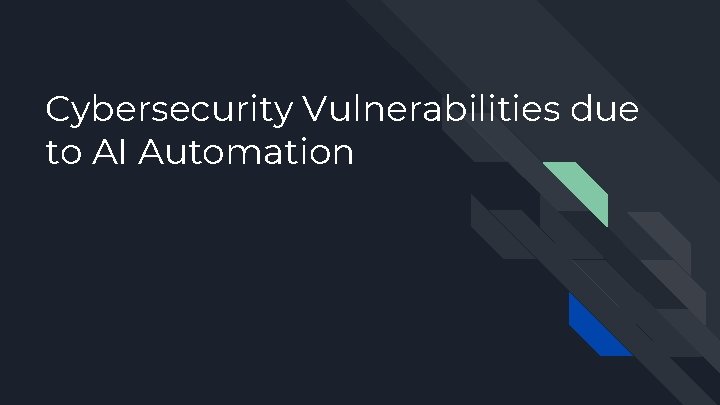
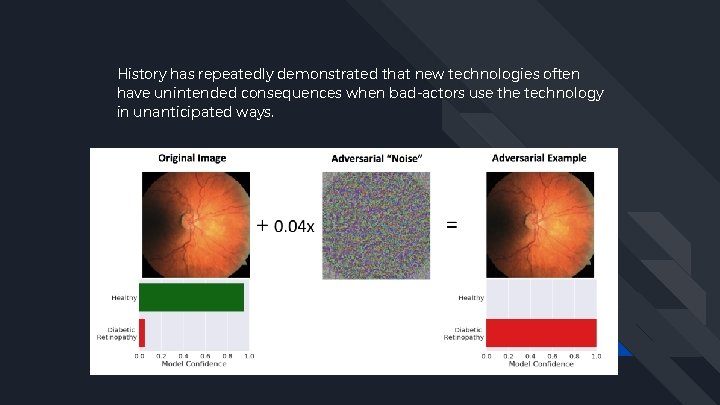
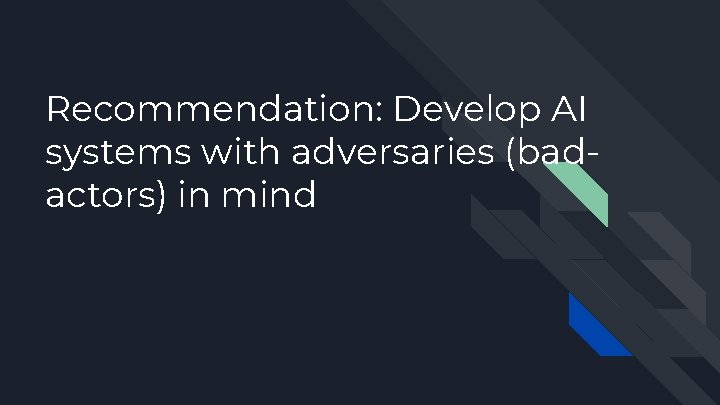
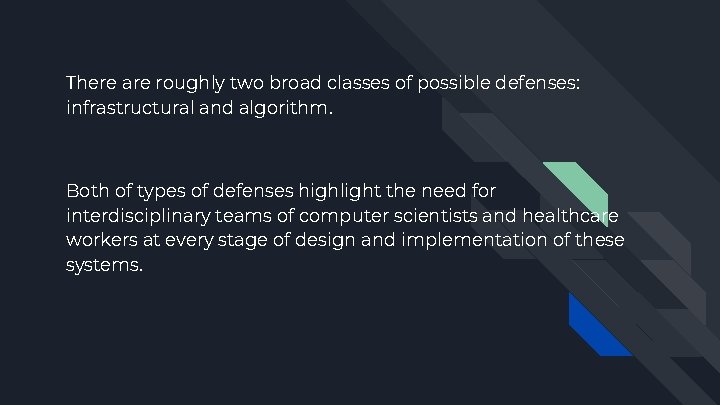
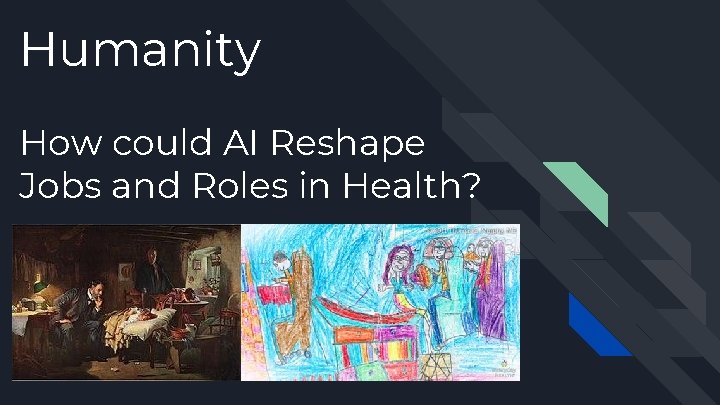
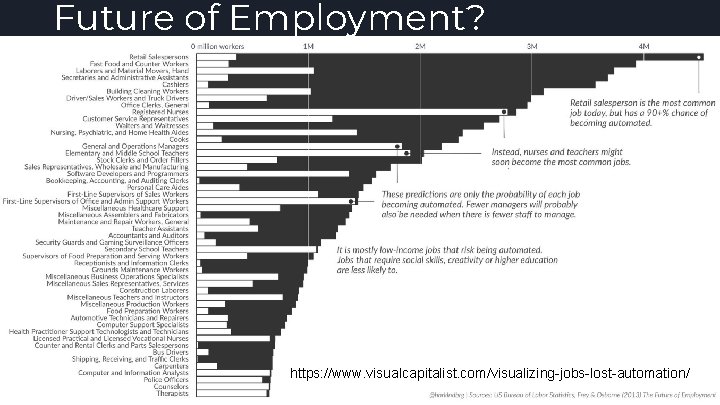
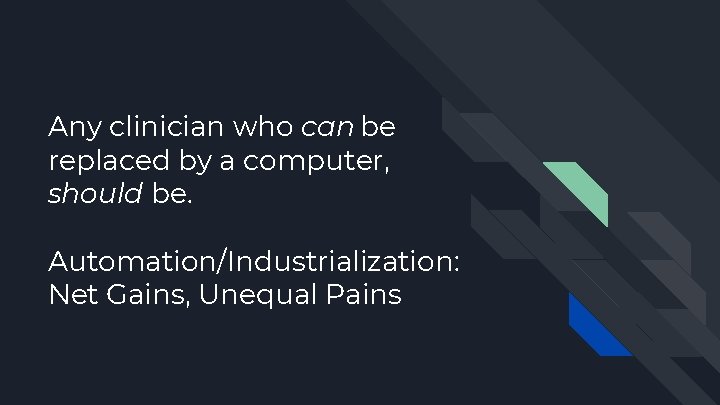
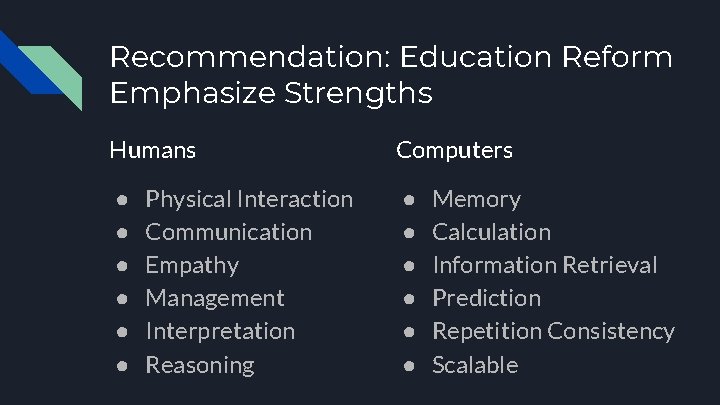
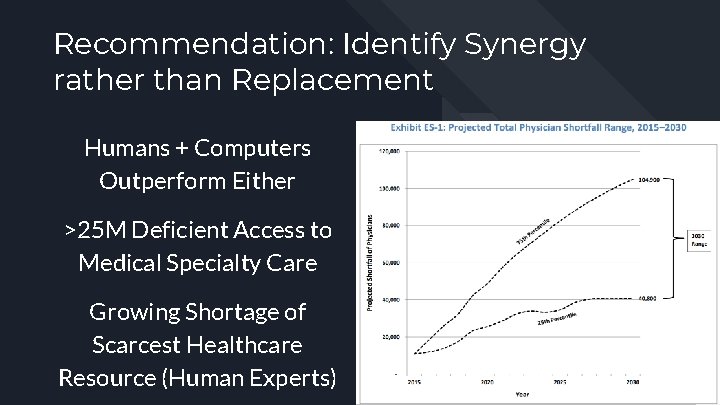
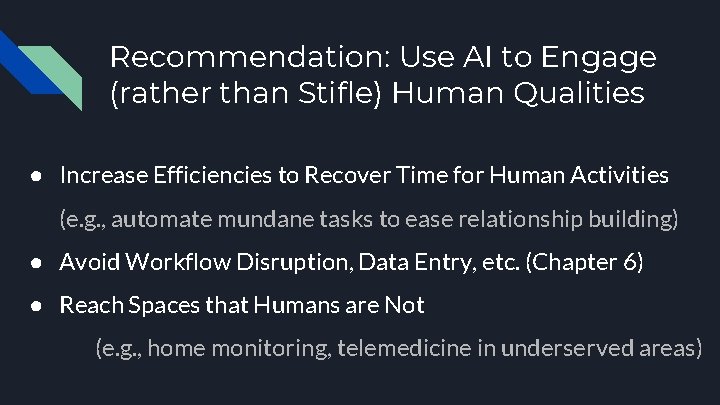
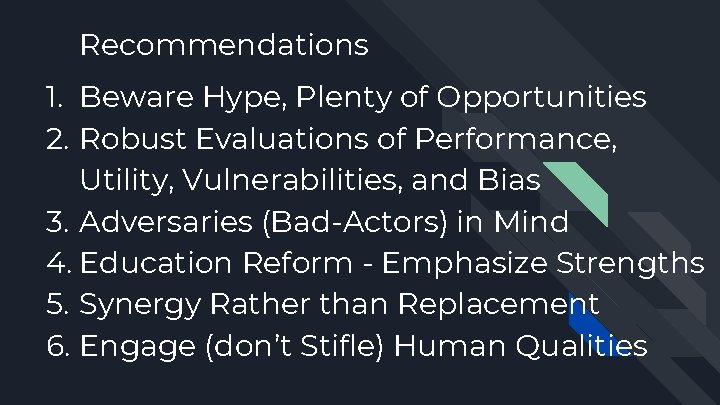
- Slides: 21
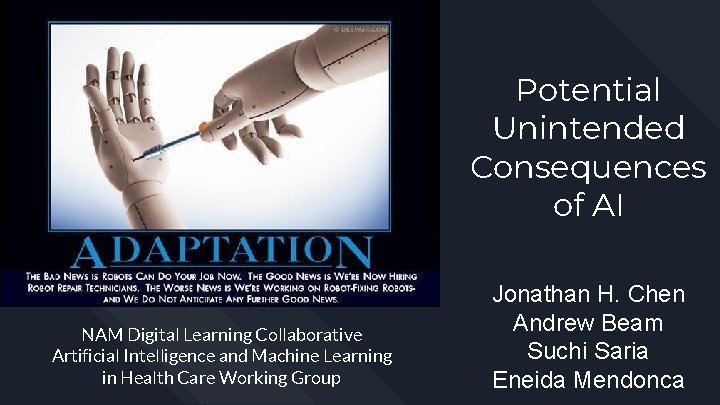
Potential Unintended Consequences of AI NAM Digital Learning Collaborative Artificial Intelligence and Machine Learning in Health Care Working Group Jonathan H. Chen Andrew Beam Suchi Saria Eneida Mendonca
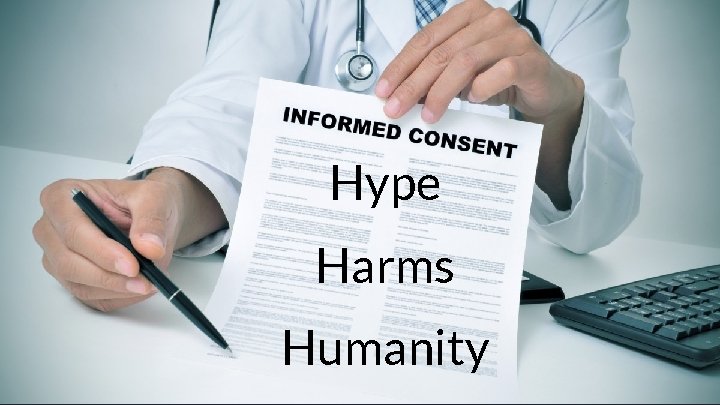
Hype Harms Humanity
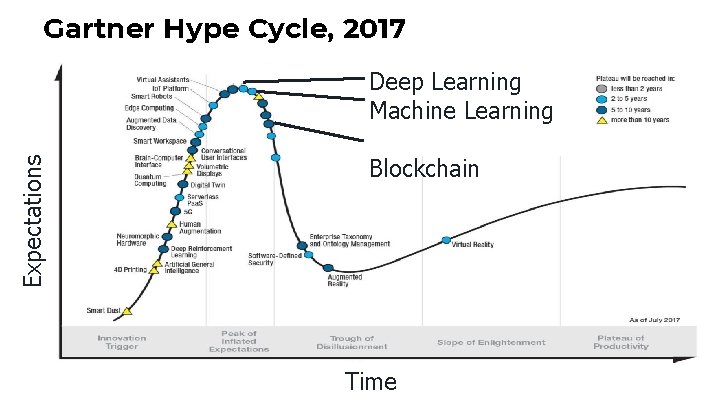
Gartner Hype Cycle, 2017 Expectations Deep Learning Machine Learning Blockchain Time
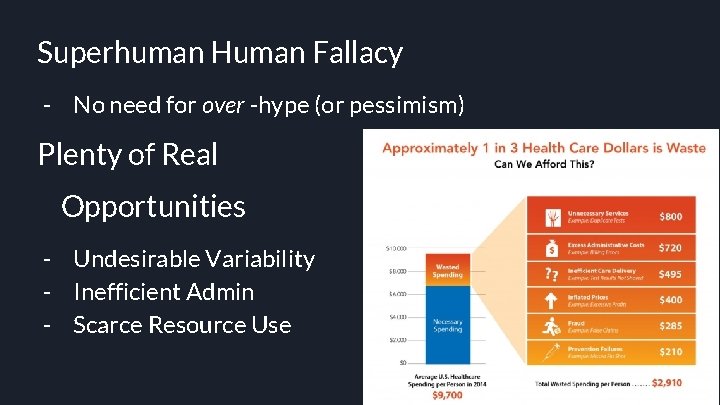
Superhuman Human Fallacy - No need for over -hype (or pessimism) Plenty of Real Opportunities - Undesirable Variability - Inefficient Admin - Scarce Resource Use
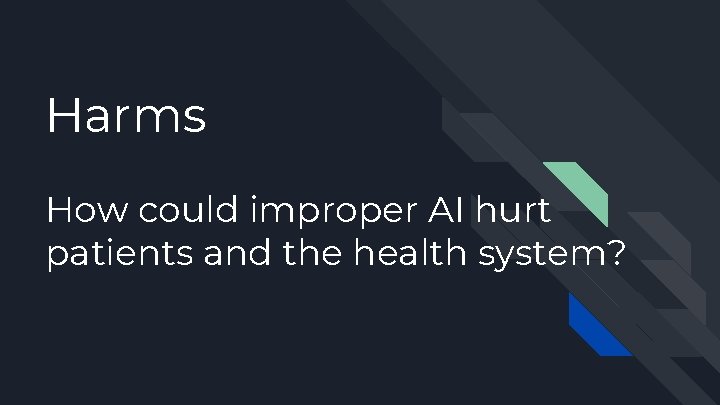
Harms How could improper AI hurt patients and the health system?
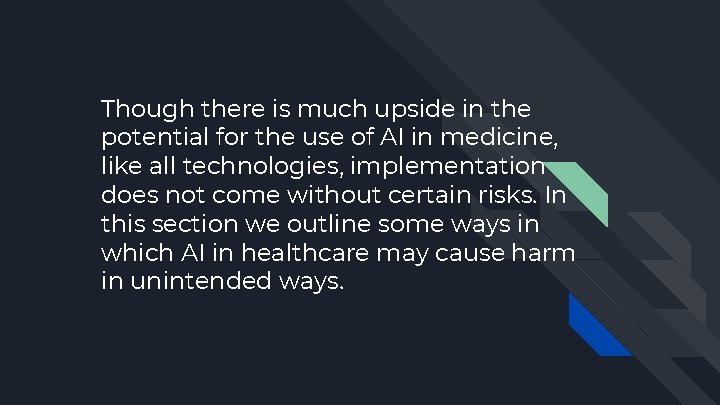
Though there is much upside in the potential for the use of AI in medicine, like all technologies, implementation does not come without certain risks. In this section we outline some ways in which AI in healthcare may cause harm in unintended ways.
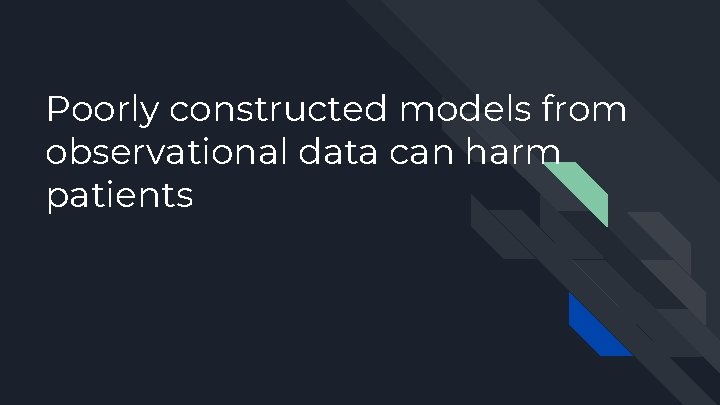
Poorly constructed models from observational data can harm patients
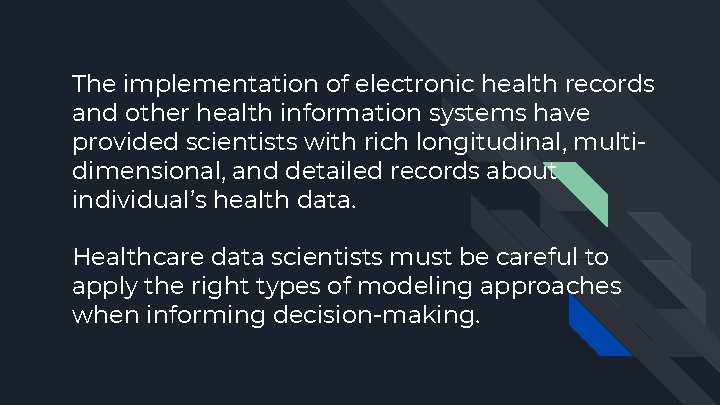
The implementation of electronic health records and other health information systems have provided scientists with rich longitudinal, multidimensional, and detailed records about individual’s health data. Healthcare data scientists must be careful to apply the right types of modeling approaches when informing decision-making.
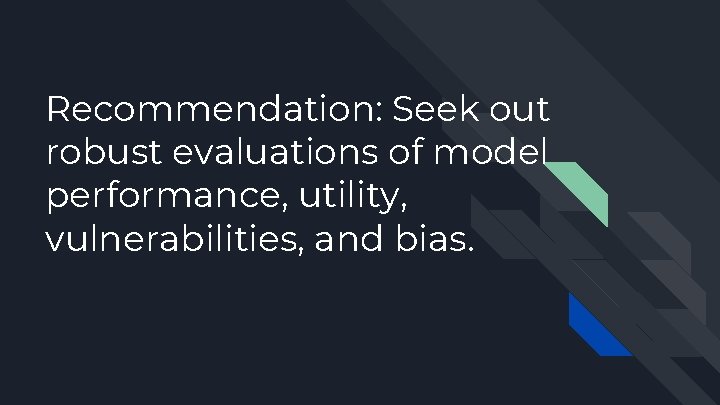
Recommendation: Seek out robust evaluations of model performance, utility, vulnerabilities, and bias.
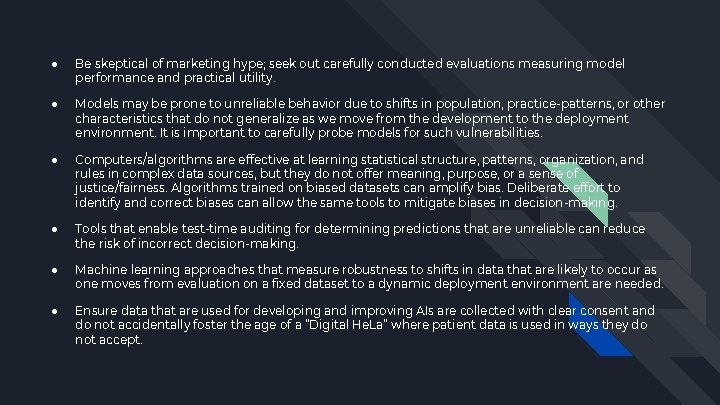
● Be skeptical of marketing hype; seek out carefully conducted evaluations measuring model performance and practical utility. ● Models may be prone to unreliable behavior due to shifts in population, practice-patterns, or other characteristics that do not generalize as we move from the development to the deployment environment. It is important to carefully probe models for such vulnerabilities. ● Computers/algorithms are effective at learning statistical structure, patterns, organization, and rules in complex data sources, but they do not offer meaning, purpose, or a sense of justice/fairness. Algorithms trained on biased datasets can amplify bias. Deliberate effort to identify and correct biases can allow the same tools to mitigate biases in decision-making. ● Tools that enable test-time auditing for determining predictions that are unreliable can reduce the risk of incorrect decision-making. ● Machine learning approaches that measure robustness to shifts in data that are likely to occur as one moves from evaluation on a fixed dataset to a dynamic deployment environment are needed. ● Ensure data that are used for developing and improving AIs are collected with clear consent and do not accidentally foster the age of a “Digital He. La” where patient data is used in ways they do not accept.
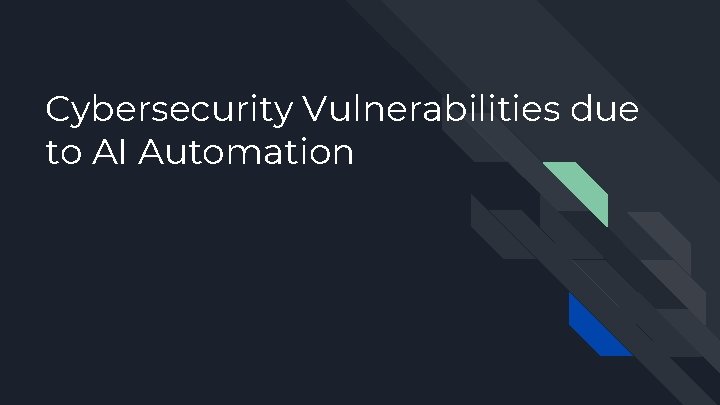
Cybersecurity Vulnerabilities due to AI Automation
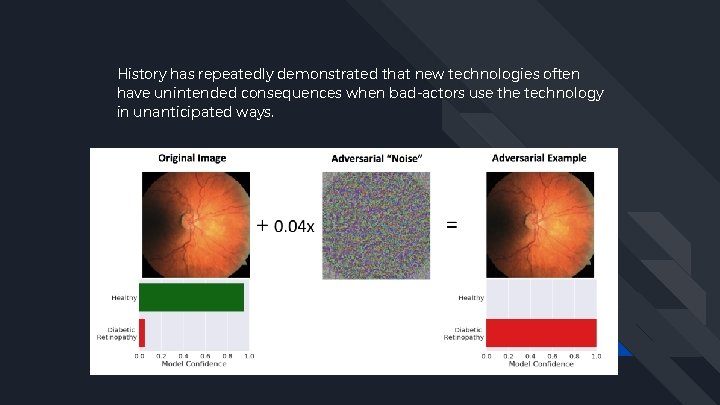
History has repeatedly demonstrated that new technologies often have unintended consequences when bad-actors use the technology in unanticipated ways.
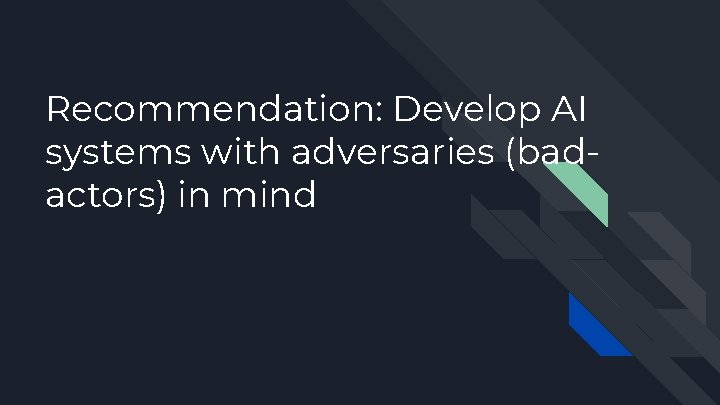
Recommendation: Develop AI systems with adversaries (badactors) in mind
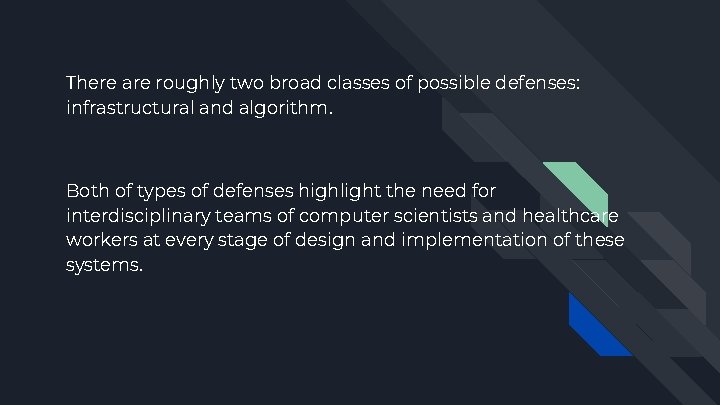
There are roughly two broad classes of possible defenses: infrastructural and algorithm. Both of types of defenses highlight the need for interdisciplinary teams of computer scientists and healthcare workers at every stage of design and implementation of these systems.
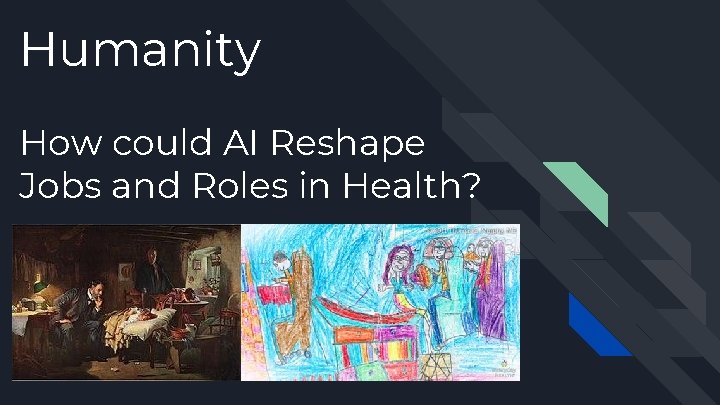
Humanity How could AI Reshape Jobs and Roles in Health?
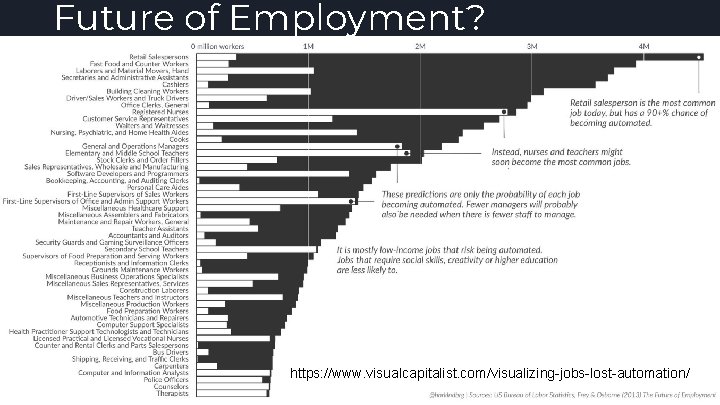
Future of Employment? https: //www. visualcapitalist. com/visualizing-jobs-lost-automation/
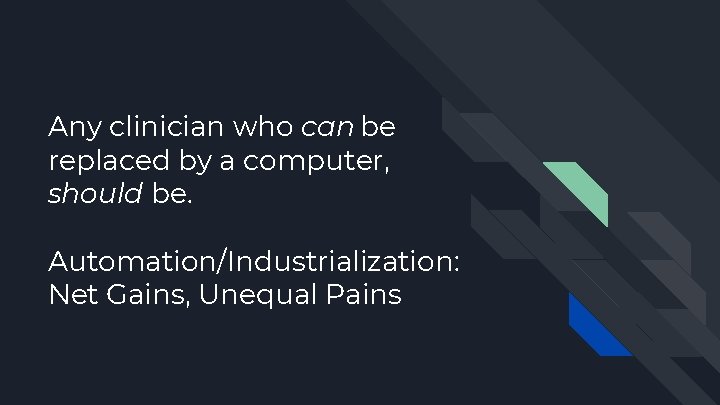
Any clinician who can be replaced by a computer, should be. Automation/Industrialization: Net Gains, Unequal Pains
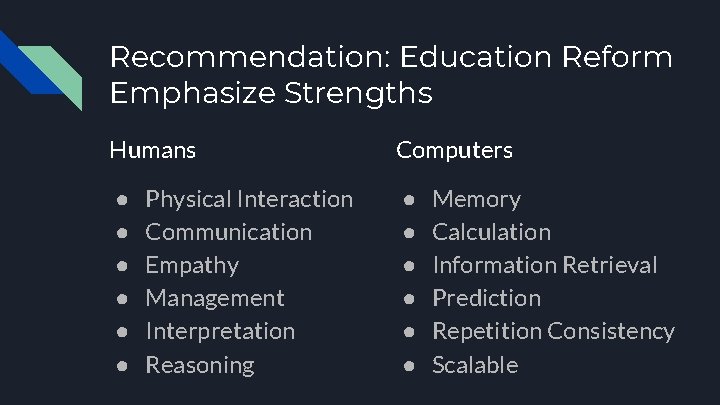
Recommendation: Education Reform Emphasize Strengths Humans ● ● ● Physical Interaction Communication Empathy Management Interpretation Reasoning Computers ● ● ● Memory Calculation Information Retrieval Prediction Repetition Consistency Scalable
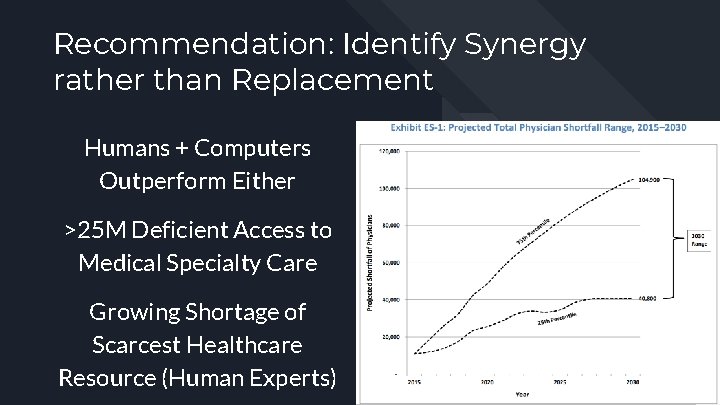
Recommendation: Identify Synergy rather than Replacement Humans + Computers Outperform Either >25 M Deficient Access to Medical Specialty Care Growing Shortage of Scarcest Healthcare Resource (Human Experts)
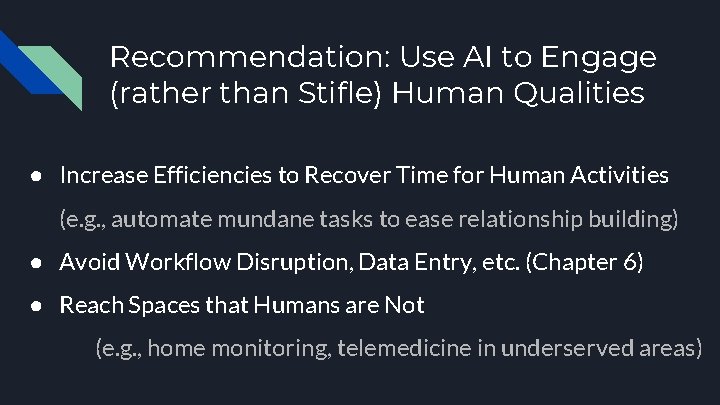
Recommendation: Use AI to Engage (rather than Stifle) Human Qualities ● Increase Efficiencies to Recover Time for Human Activities (e. g. , automate mundane tasks to ease relationship building) ● Avoid Workflow Disruption, Data Entry, etc. (Chapter 6) ● Reach Spaces that Humans are Not (e. g. , home monitoring, telemedicine in underserved areas)
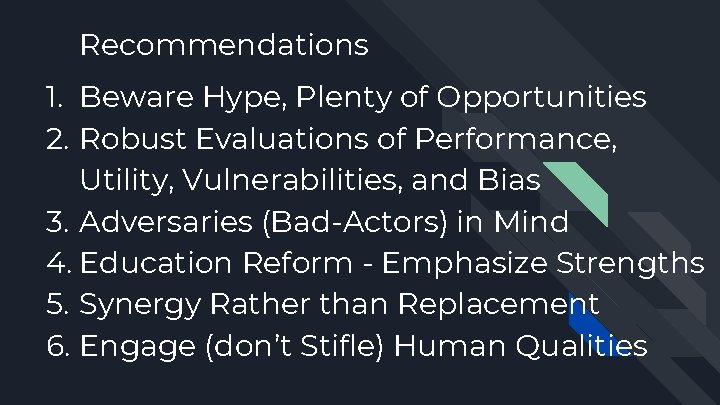
Recommendations 1. Beware Hype, Plenty of Opportunities 2. Robust Evaluations of Performance, Utility, Vulnerabilities, and Bias 3. Adversaries (Bad-Actors) in Mind 4. Education Reform - Emphasize Strengths 5. Synergy Rather than Replacement 6. Engage (don’t Stifle) Human Qualities
Unintended consequences
Unintended consequences
Daj nam svima duha svog tekst
Pertulangan daun jati
Bắc cực
Lịch sử hà nội từ năm 1802 đến năm 1918
Osmotic potential vs water potential
Water potential definition biology
Water potential equation
Graded potential vs action potential
Graded potentials
Graded potential definition
Graded potential vs action potential
Absolute refractory period
Osmotic potential vs water potential
Source of bioelectric potential
End-plate potential vs action potential
Suggmadex
Action potential resting potential
Action potential resting potential
Market potential and forecasting
Define electric potential and potential difference.