Introduction to Logistic Regression Coronary Heart Disease CHD
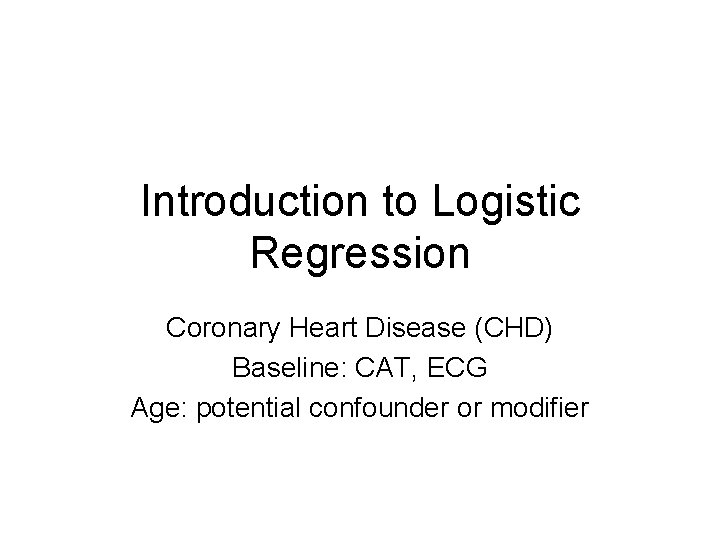
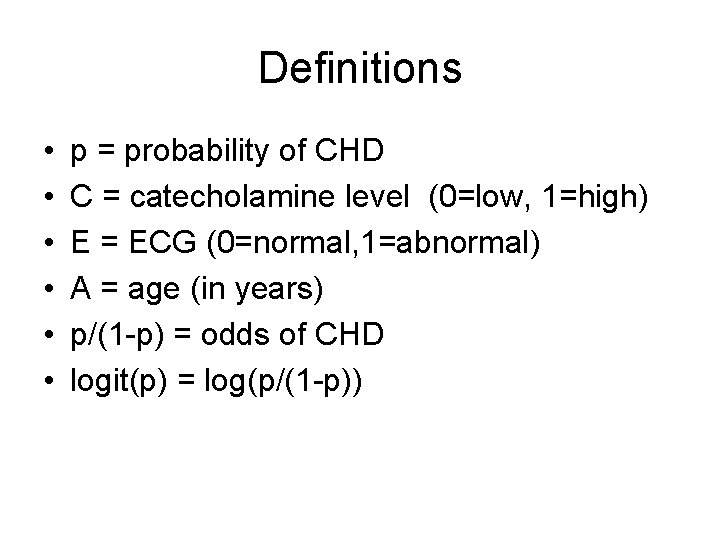
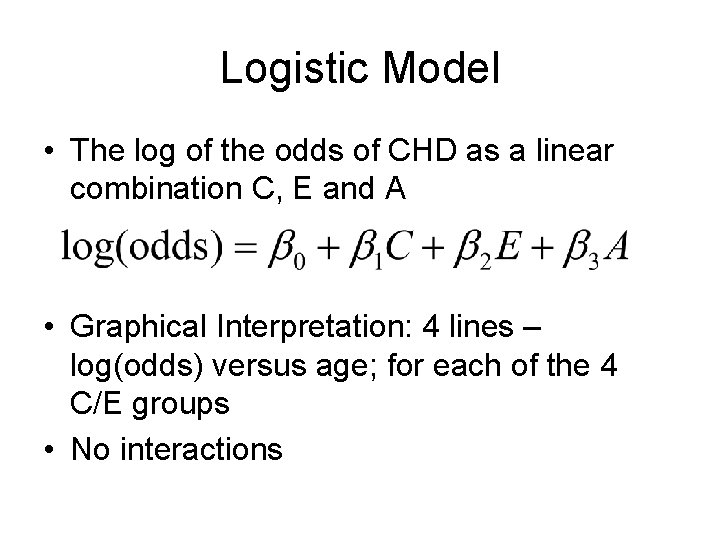
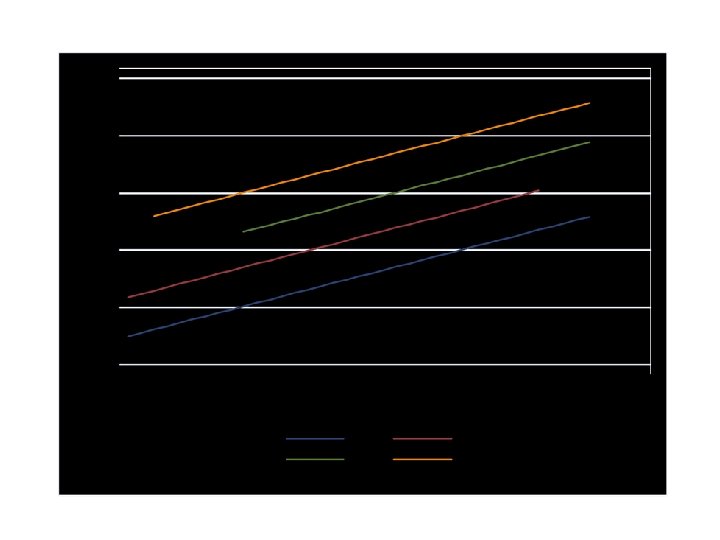
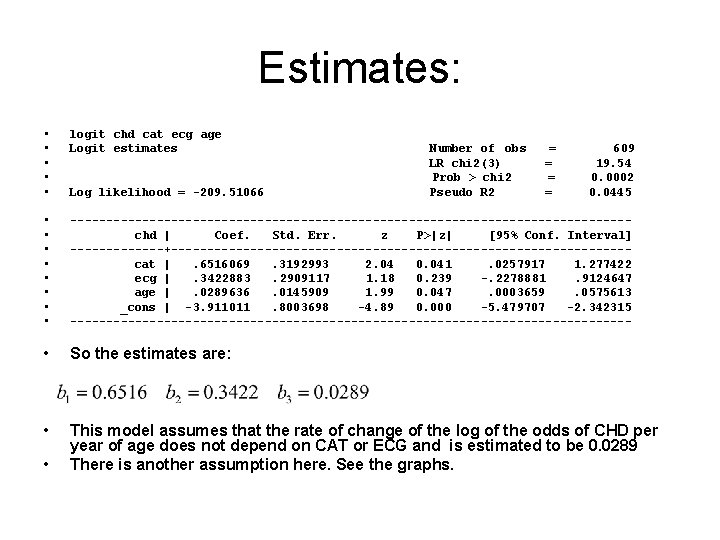
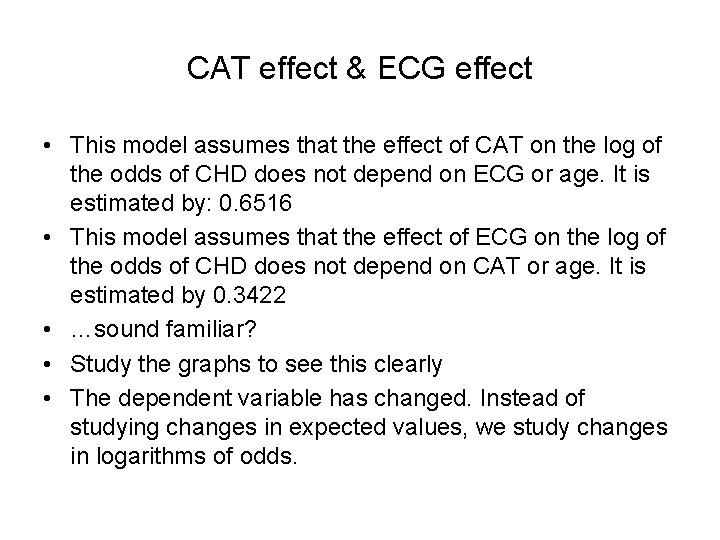
- Slides: 6
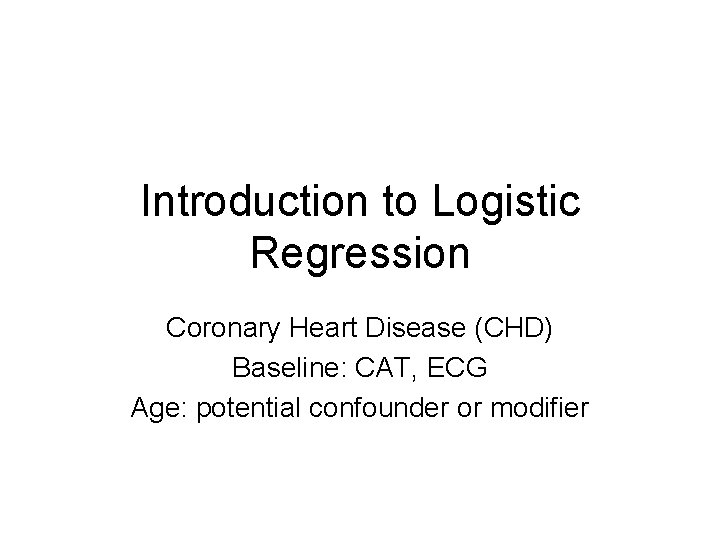
Introduction to Logistic Regression Coronary Heart Disease (CHD) Baseline: CAT, ECG Age: potential confounder or modifier
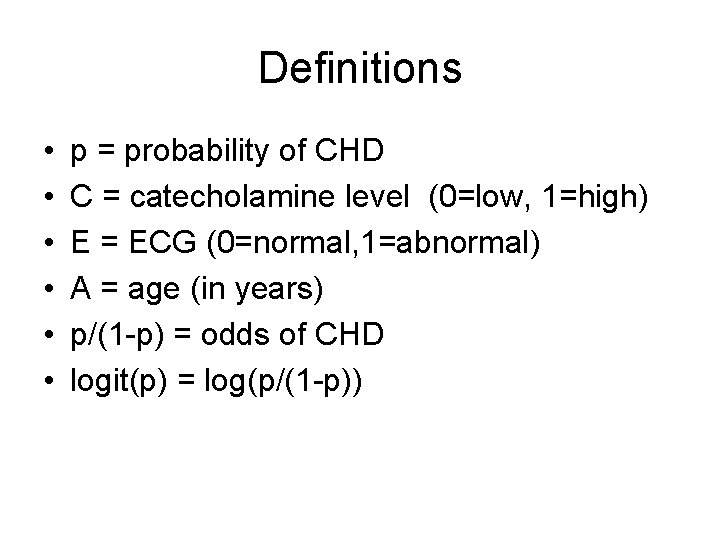
Definitions • • • p = probability of CHD C = catecholamine level (0=low, 1=high) E = ECG (0=normal, 1=abnormal) A = age (in years) p/(1 -p) = odds of CHD logit(p) = log(p/(1 -p))
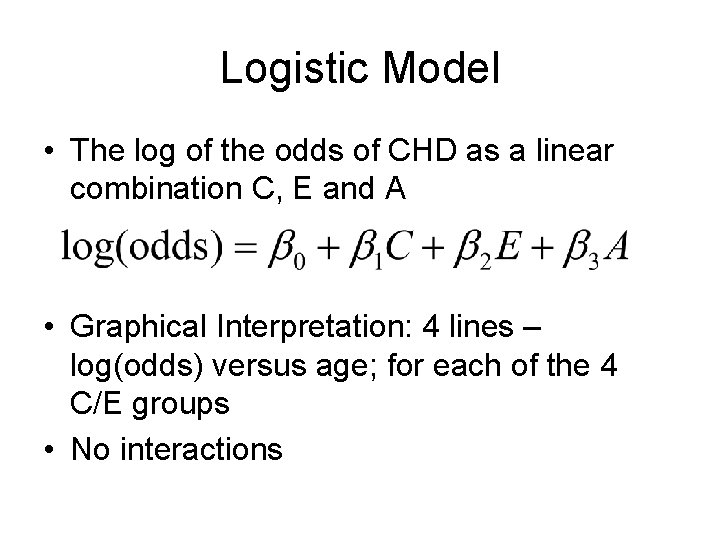
Logistic Model • The log of the odds of CHD as a linear combination C, E and A • Graphical Interpretation: 4 lines – log(odds) versus age; for each of the 4 C/E groups • No interactions
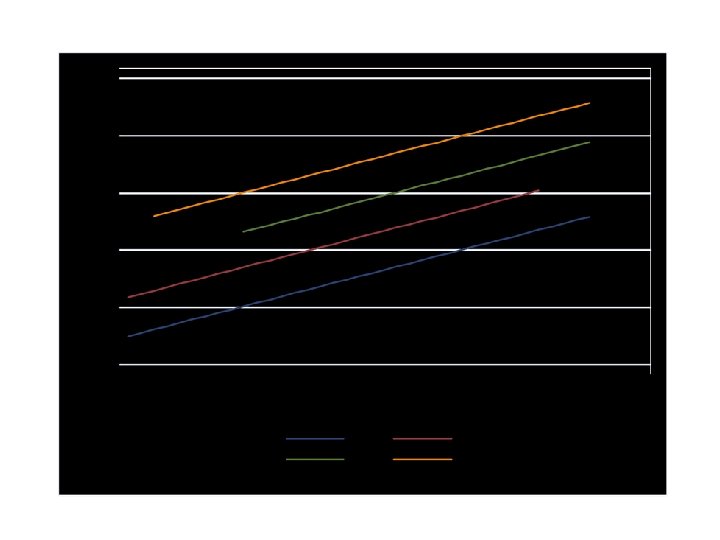
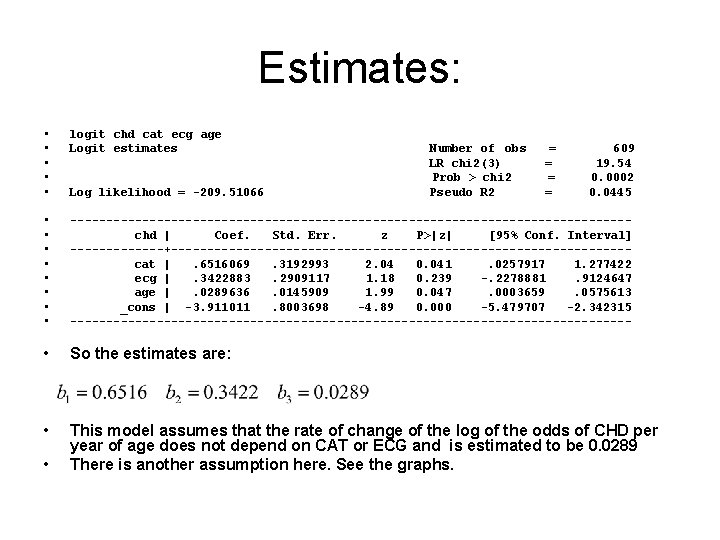
Estimates: • • • logit chd cat ecg age Logit estimates • • ---------------------------------------chd | Coef. Std. Err. z P>|z| [95% Conf. Interval] -------+--------------------------------cat |. 6516069. 3192993 2. 04 0. 041. 0257917 1. 277422 ecg |. 3422883. 2909117 1. 18 0. 239 -. 2278881. 9124647 age |. 0289636. 0145909 1. 99 0. 047. 0003659. 0575613 _cons | -3. 911011. 8003698 -4. 89 0. 000 -5. 479707 -2. 342315 --------------------------------------- • So the estimates are: • This model assumes that the rate of change of the log of the odds of CHD per year of age does not depend on CAT or ECG and is estimated to be 0. 0289 There is another assumption here. See the graphs. • Log likelihood = -209. 51066 Number of obs LR chi 2(3) Prob > chi 2 Pseudo R 2 = = 609 19. 54 0. 0002 0. 0445
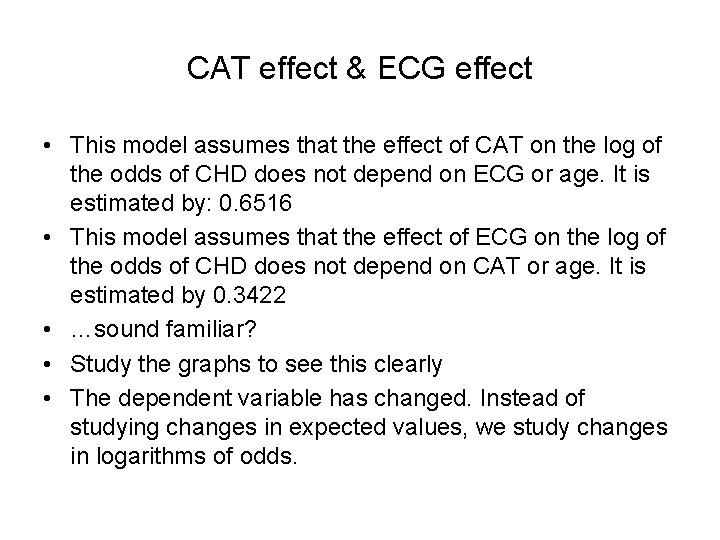
CAT effect & ECG effect • This model assumes that the effect of CAT on the log of the odds of CHD does not depend on ECG or age. It is estimated by: 0. 6516 • This model assumes that the effect of ECG on the log of the odds of CHD does not depend on CAT or age. It is estimated by 0. 3422 • …sound familiar? • Study the graphs to see this clearly • The dependent variable has changed. Instead of studying changes in expected values, we study changes in logarithms of odds.