Inferring airsea fluxes through dynamically consistent ocean state
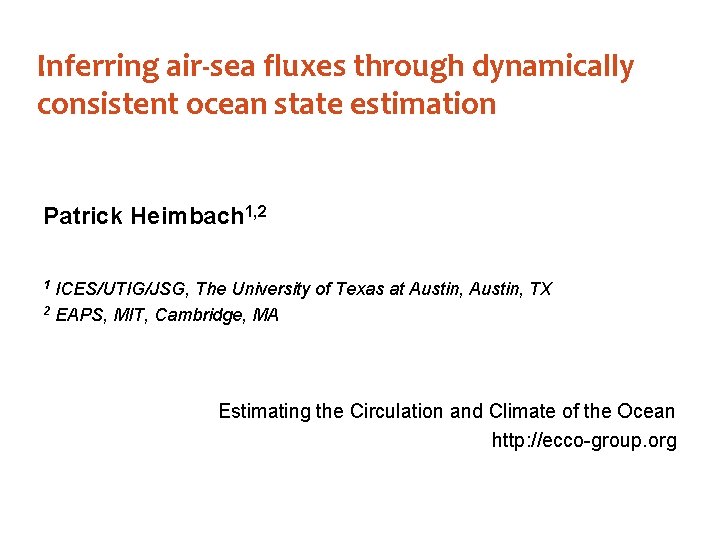
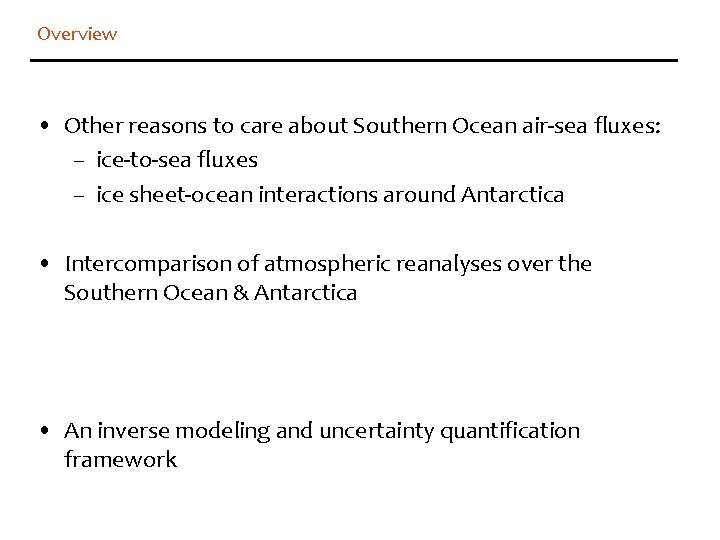
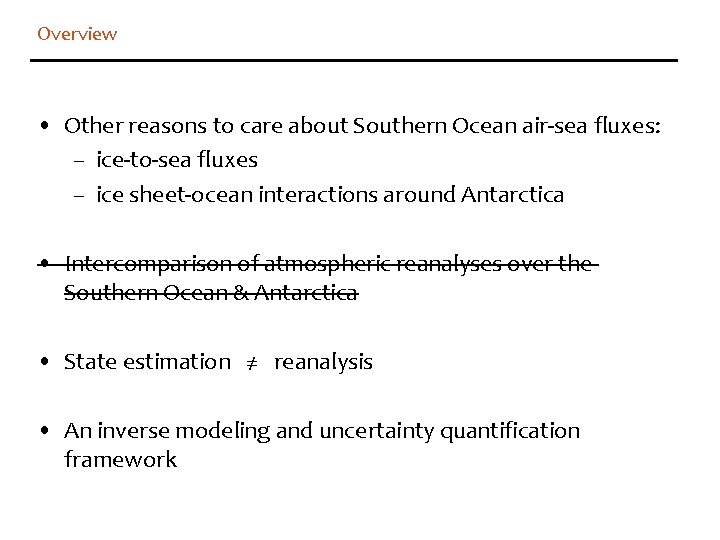
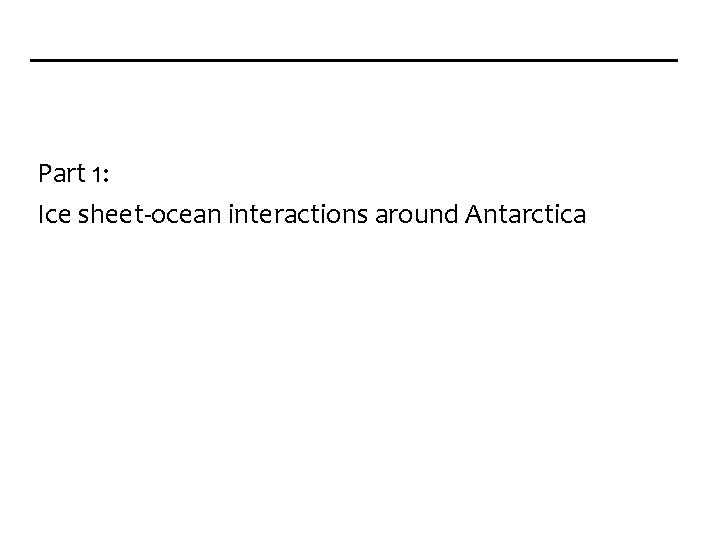
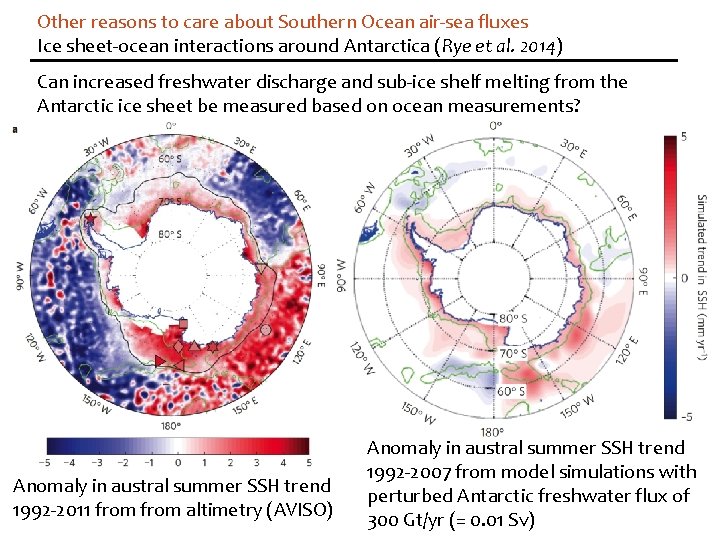
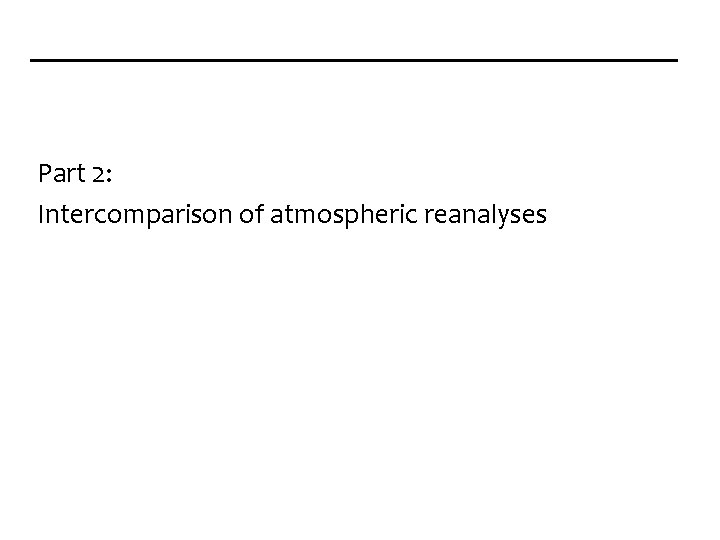
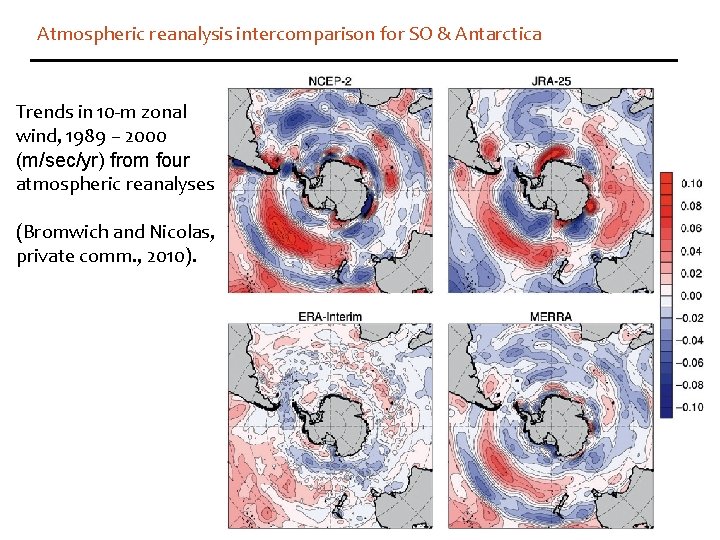
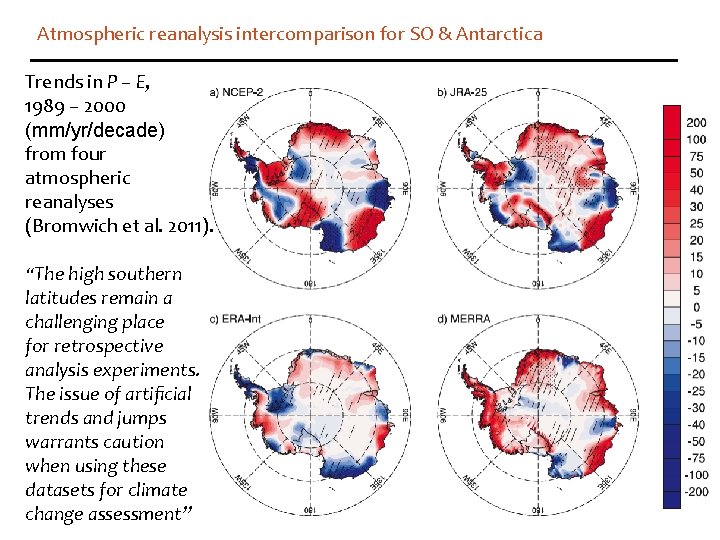
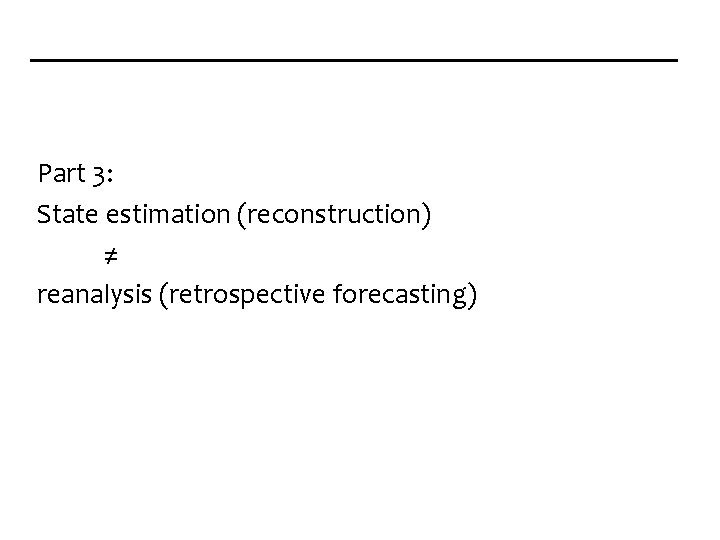
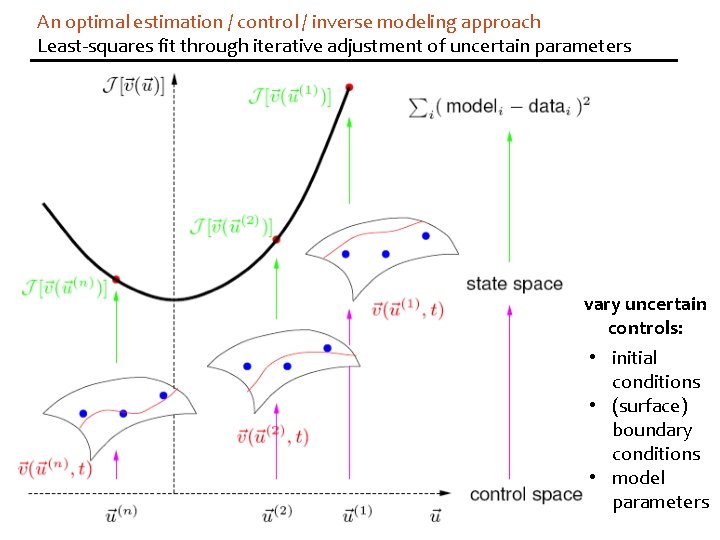
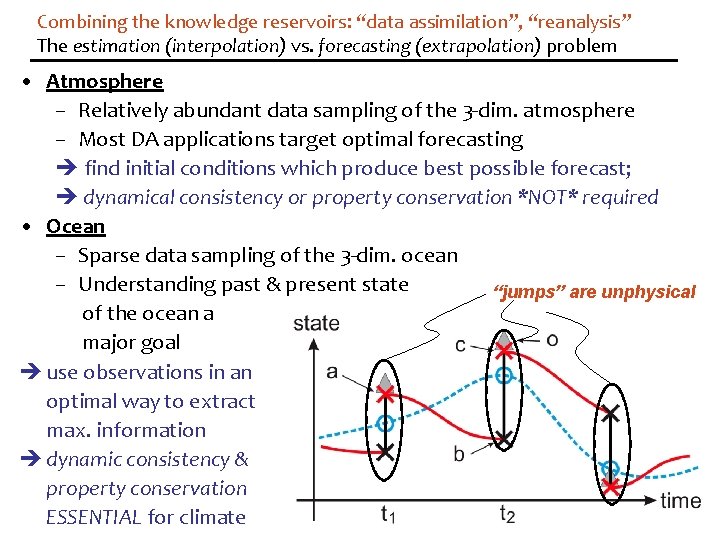
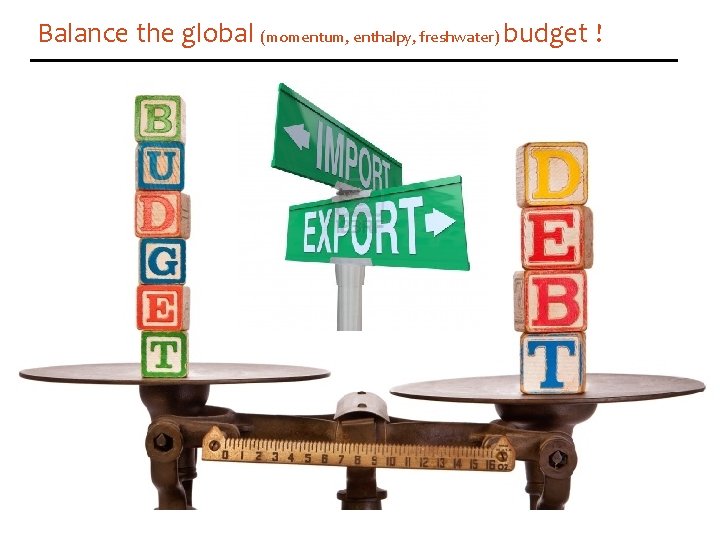
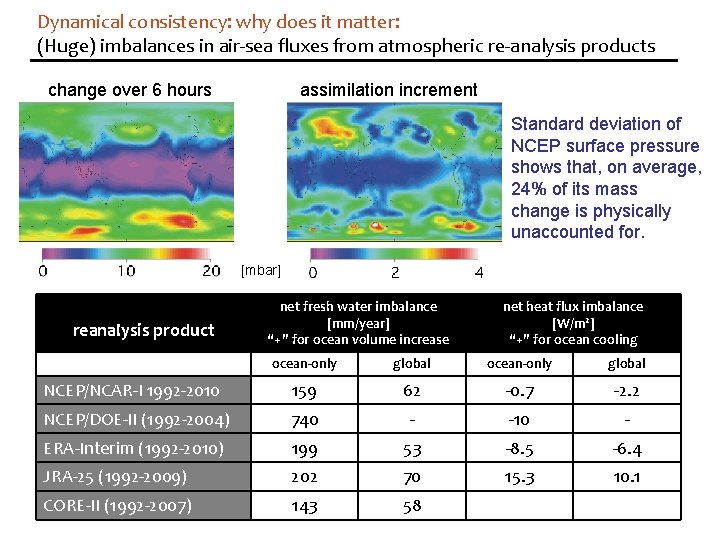
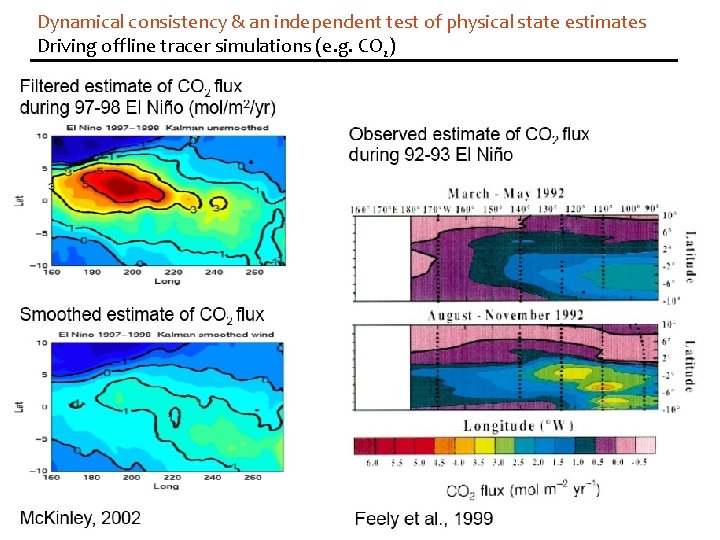
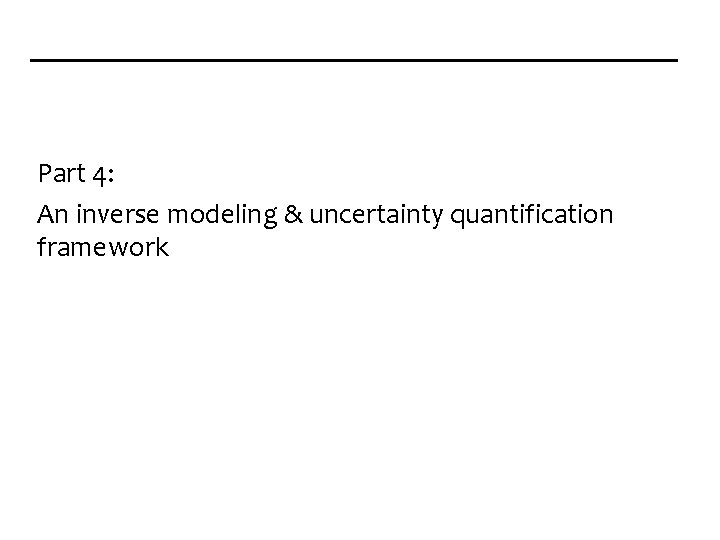
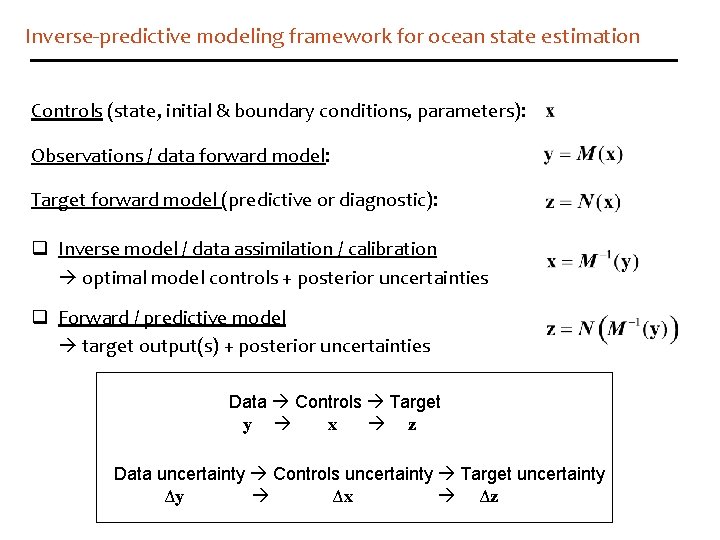
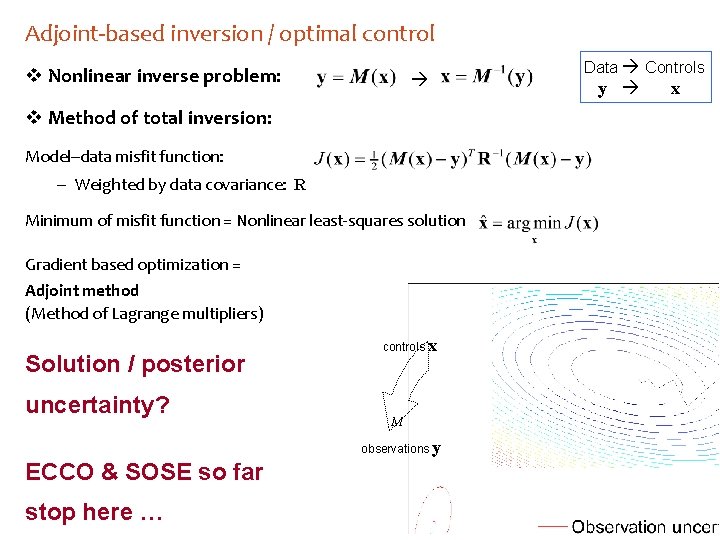
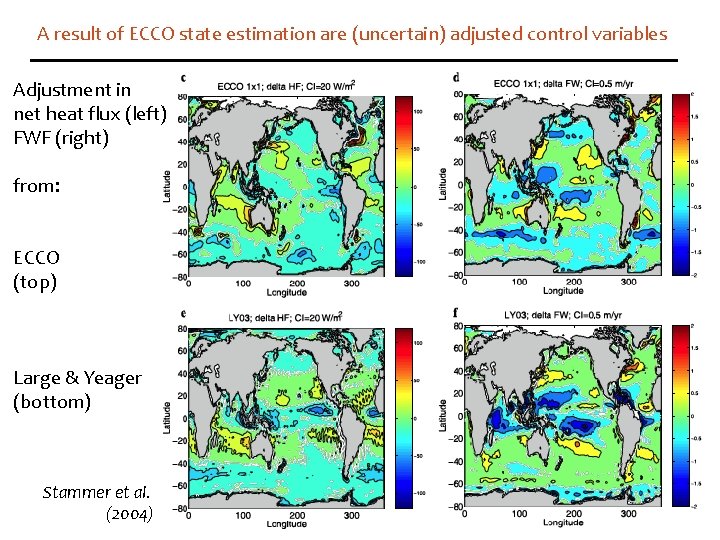
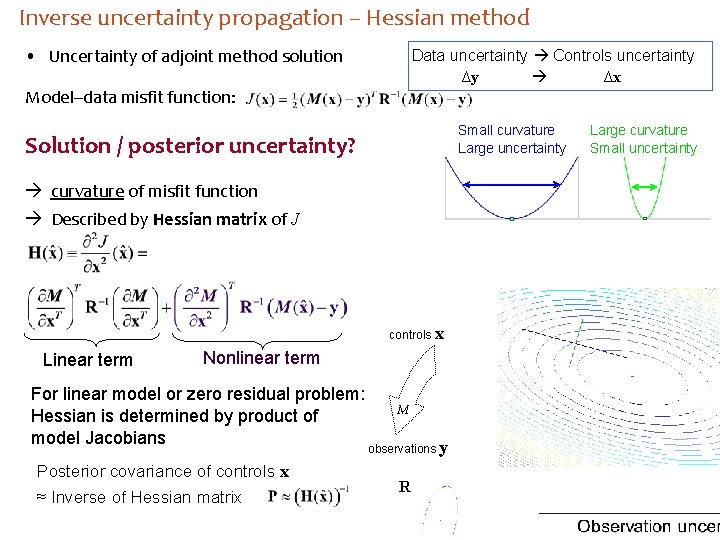
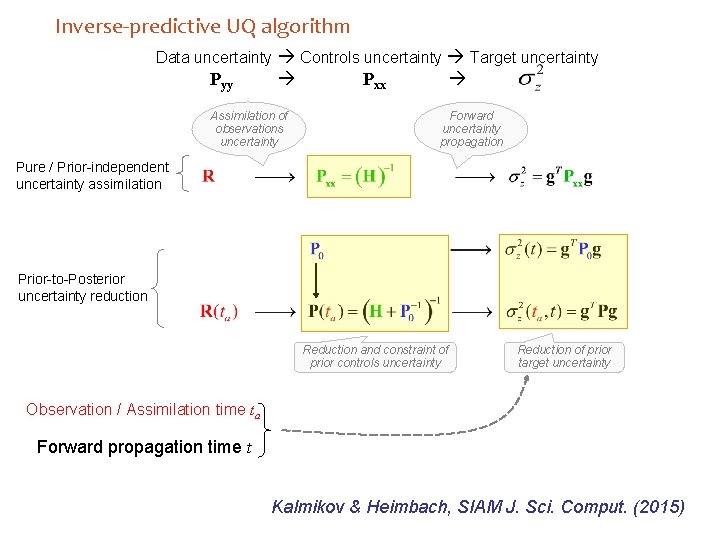
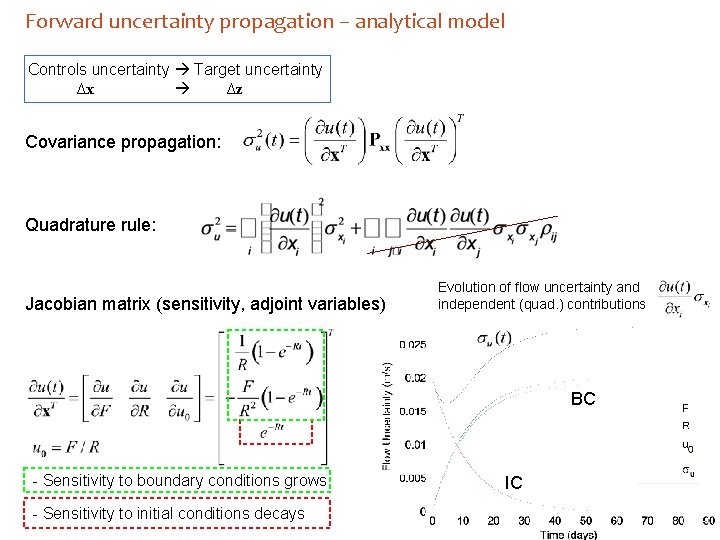
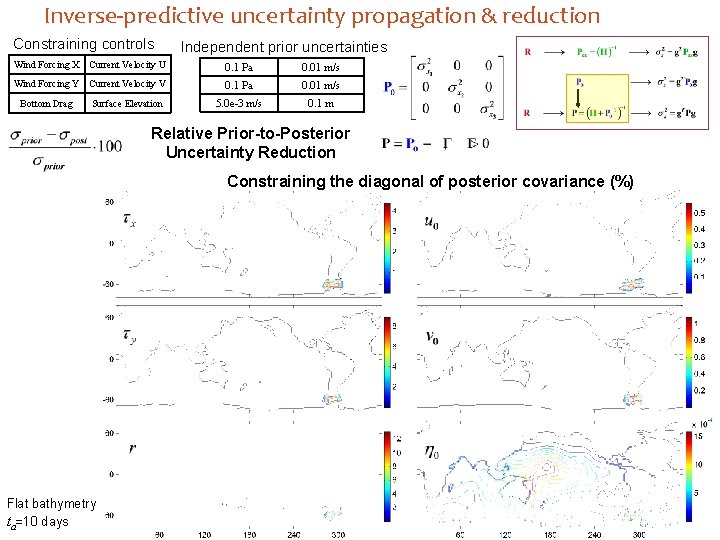
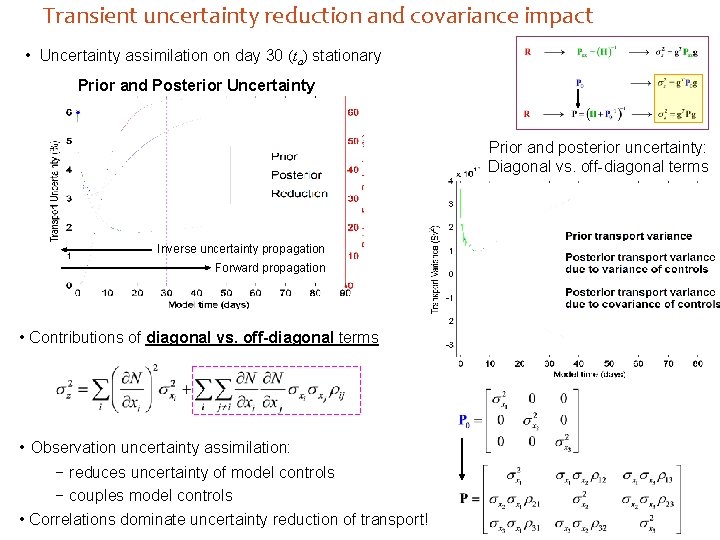
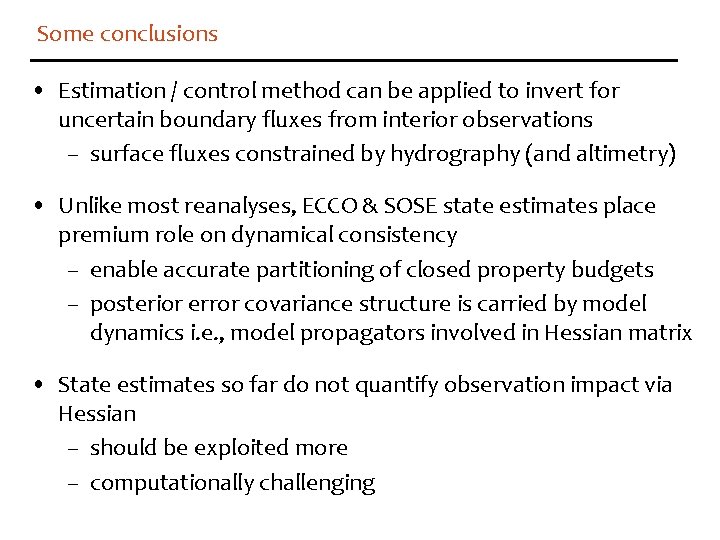
- Slides: 24
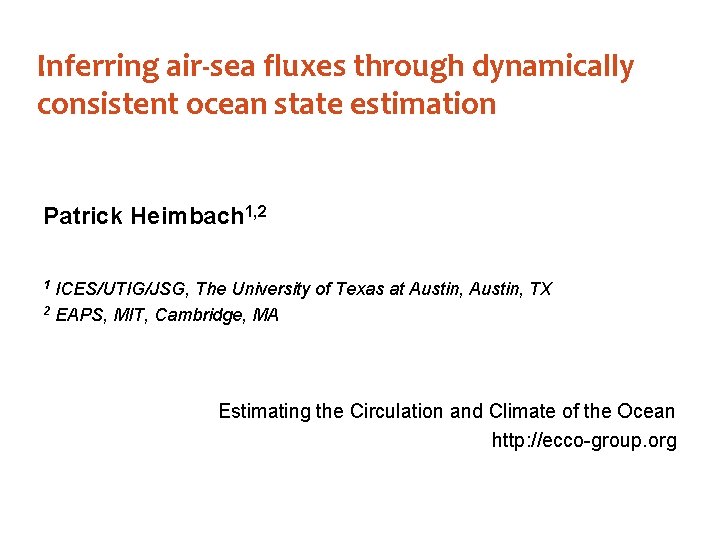
Inferring air-sea fluxes through dynamically consistent ocean state estimation Patrick Heimbach 1, 2 ICES/UTIG/JSG, The University of Texas at Austin, TX 2 EAPS, MIT, Cambridge, MA 1 Estimating the Circulation and Climate of the Ocean http: //ecco-group. org
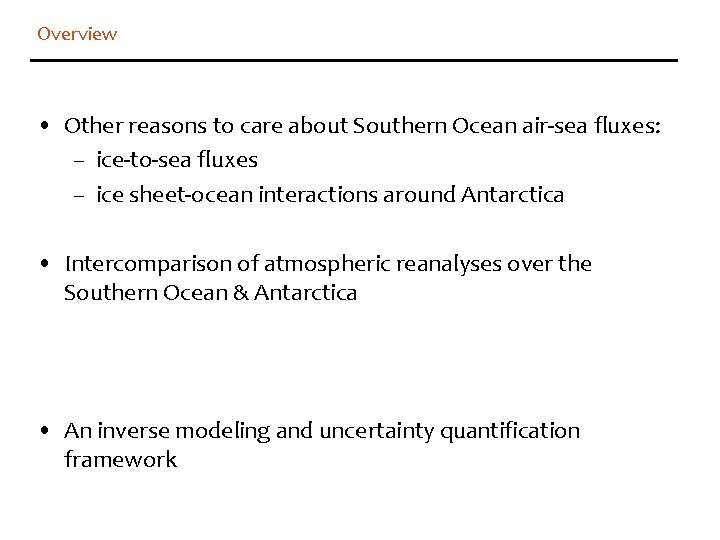
Overview • Other reasons to care about Southern Ocean air-sea fluxes: – ice-to-sea fluxes – ice sheet-ocean interactions around Antarctica • Intercomparison of atmospheric reanalyses over the Southern Ocean & Antarctica • An inverse modeling and uncertainty quantification framework
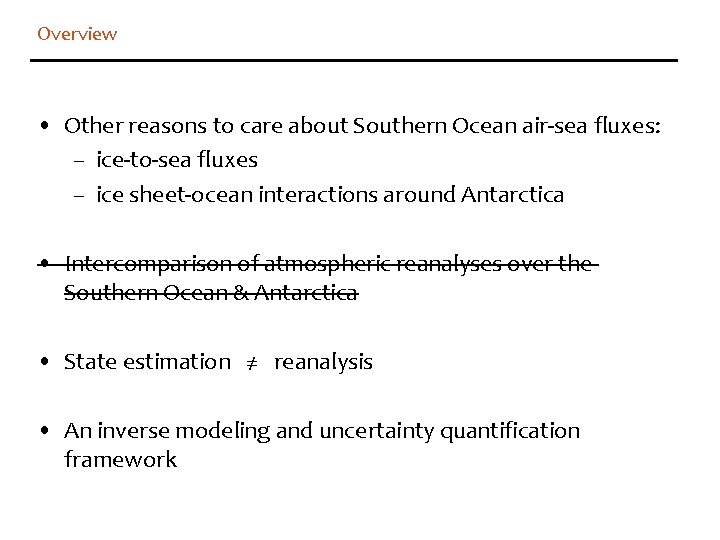
Overview • Other reasons to care about Southern Ocean air-sea fluxes: – ice-to-sea fluxes – ice sheet-ocean interactions around Antarctica • Intercomparison of atmospheric reanalyses over the Southern Ocean & Antarctica • State estimation ≠ reanalysis • An inverse modeling and uncertainty quantification framework
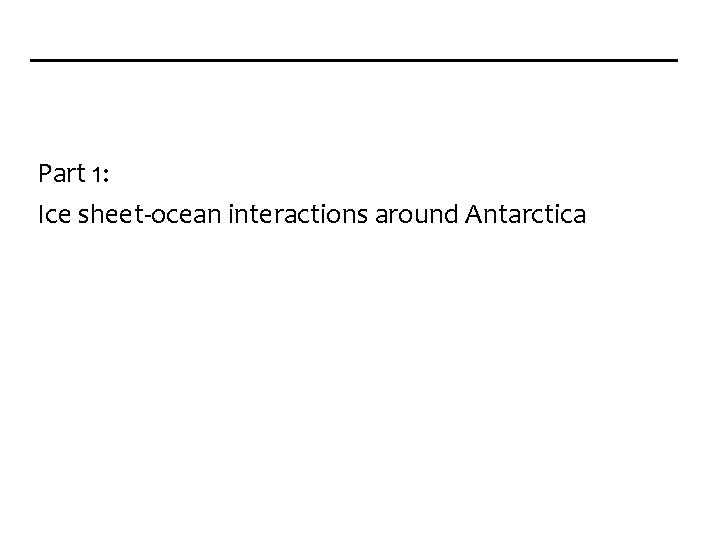
Part 1: Ice sheet-ocean interactions around Antarctica
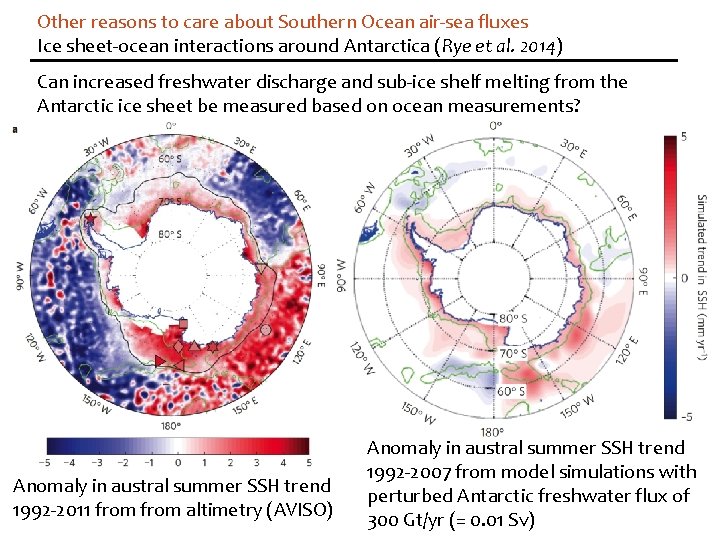
Other reasons to care about Southern Ocean air-sea fluxes Ice sheet-ocean interactions around Antarctica (Rye et al. 2014) Can increased freshwater discharge and sub-ice shelf melting from the Antarctic ice sheet be measured based on ocean measurements? Anomaly in austral summer SSH trend 1992 -2011 from altimetry (AVISO) Anomaly in austral summer SSH trend 1992 -2007 from model simulations with perturbed Antarctic freshwater flux of 300 Gt/yr (= 0. 01 Sv)
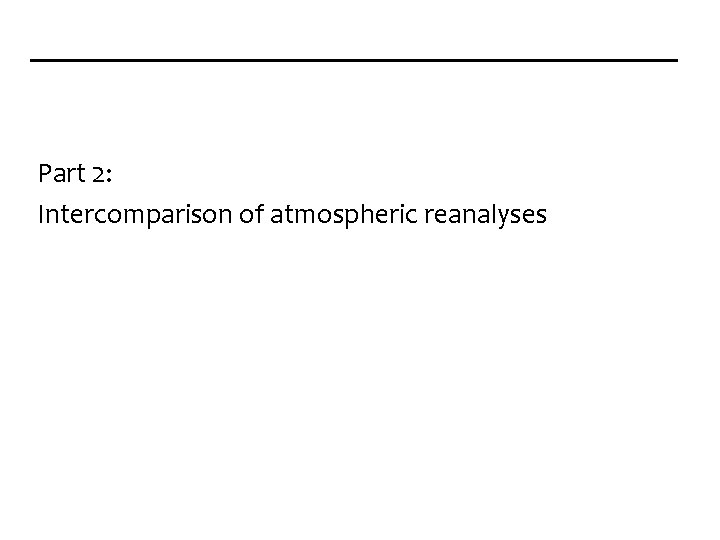
Part 2: Intercomparison of atmospheric reanalyses
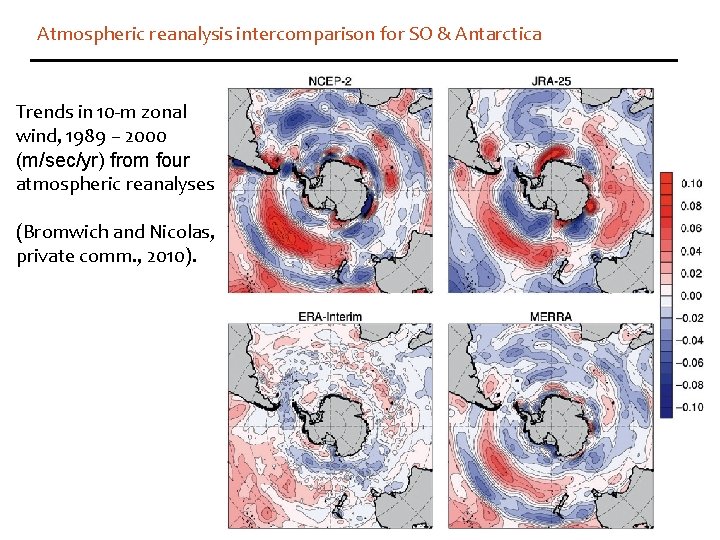
Atmospheric reanalysis intercomparison for SO & Antarctica Trends in 10 -m zonal wind, 1989 – 2000 (m/sec/yr) from four atmospheric reanalyses (Bromwich and Nicolas, private comm. , 2010).
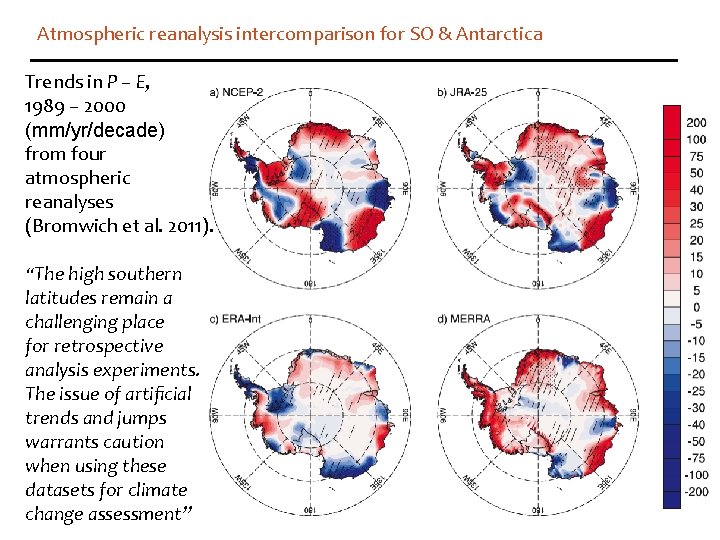
Atmospheric reanalysis intercomparison for SO & Antarctica Trends in P – E, 1989 – 2000 (mm/yr/decade) from four atmospheric reanalyses (Bromwich et al. 2011). “The high southern latitudes remain a challenging place for retrospective analysis experiments. The issue of artificial trends and jumps warrants caution when using these datasets for climate change assessment”
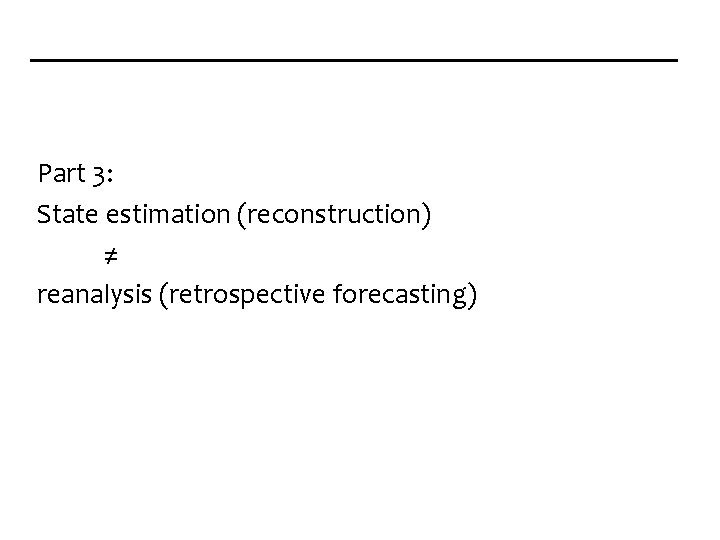
Part 3: State estimation (reconstruction) ≠ reanalysis (retrospective forecasting)
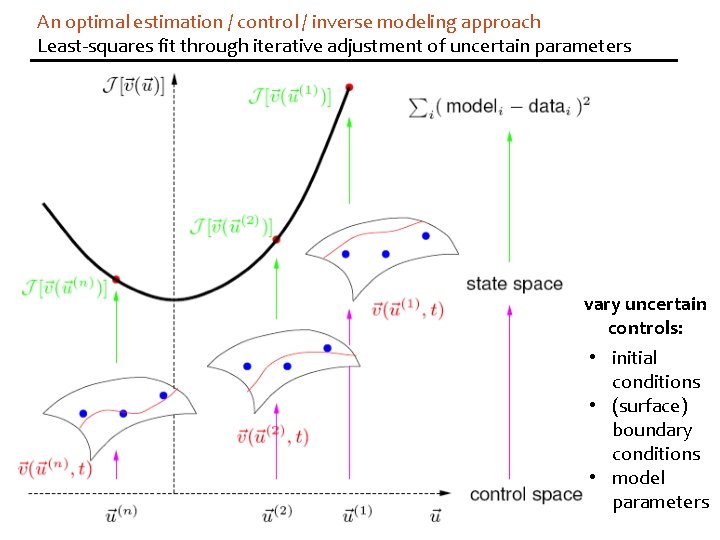
An optimal estimation / control / inverse modeling approach Least-squares fit through iterative adjustment of uncertain parameters vary uncertain controls: • initial conditions • (surface) boundary conditions • model parameters
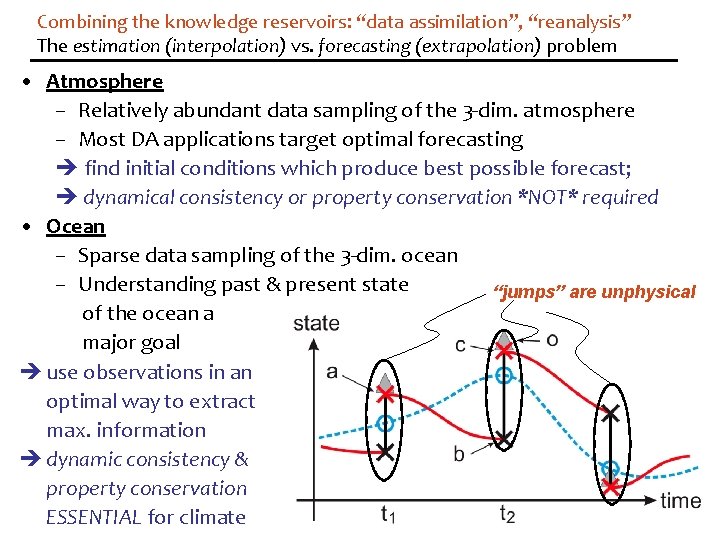
Combining the knowledge reservoirs: “data assimilation”, “reanalysis” The estimation (interpolation) vs. forecasting (extrapolation) problem • Atmosphere – Relatively abundant data sampling of the 3 -dim. atmosphere – Most DA applications target optimal forecasting find initial conditions which produce best possible forecast; dynamical consistency or property conservation *NOT* required • Ocean – Sparse data sampling of the 3 -dim. ocean – Understanding past & present state “jumps” are unphysical of the ocean a major goal use observations in an optimal way to extract max. information dynamic consistency & property conservation ESSENTIAL for climate
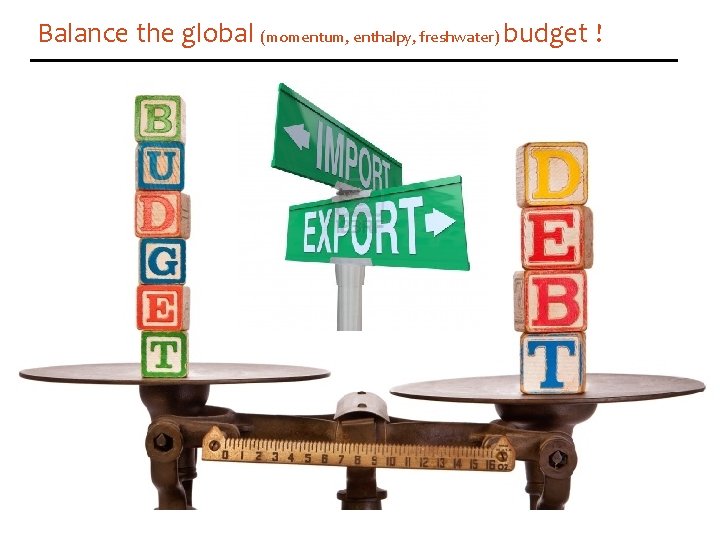
Balance the global (momentum, enthalpy, freshwater) budget !
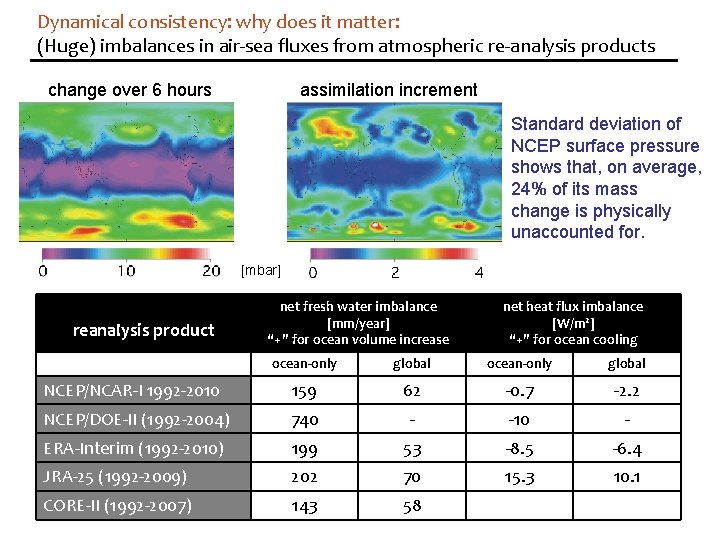
Dynamical consistency: why does it matter: (Huge) imbalances in air-sea fluxes from atmospheric re-analysis products change over 6 hours assimilation increment Standard deviation of NCEP surface pressure shows that, on average, 24% of its mass change is physically unaccounted for. [mbar] reanalysis product net fresh water imbalance [mm/year] “+” for ocean volume increase net heat flux imbalance [W/m 2] “+” for ocean cooling ocean-only global NCEP/NCAR-I 1992 -2010 159 62 -0. 7 -2. 2 NCEP/DOE-II (1992 -2004) 740 - -10 - ERA-Interim (1992 -2010) 199 53 -8. 5 -6. 4 JRA-25 (1992 -2009) 202 70 15. 3 10. 1 CORE-II (1992 -2007) 143 58
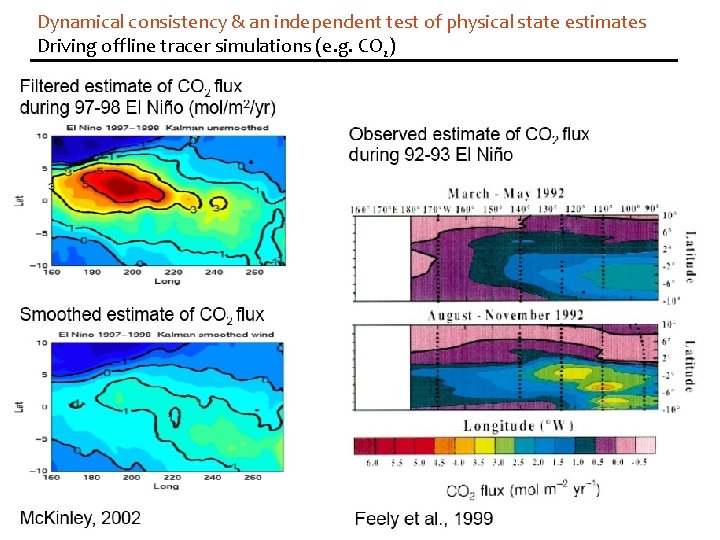
Dynamical consistency & an independent test of physical state estimates Driving offline tracer simulations (e. g. CO 2)
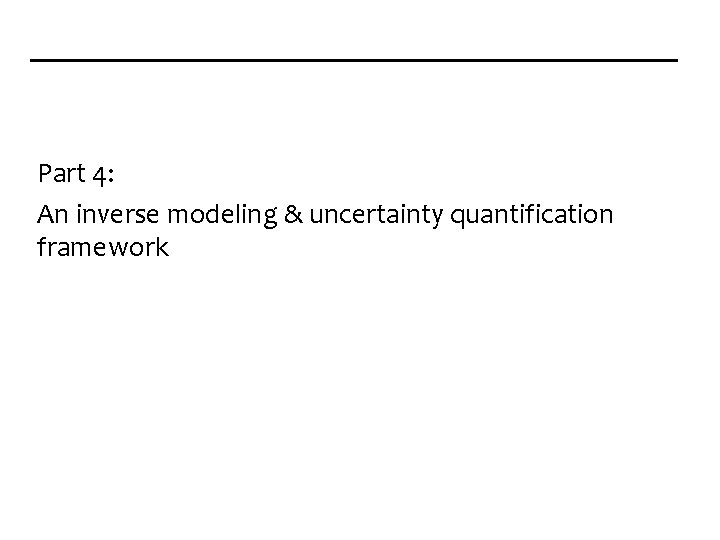
Part 4: An inverse modeling & uncertainty quantification framework
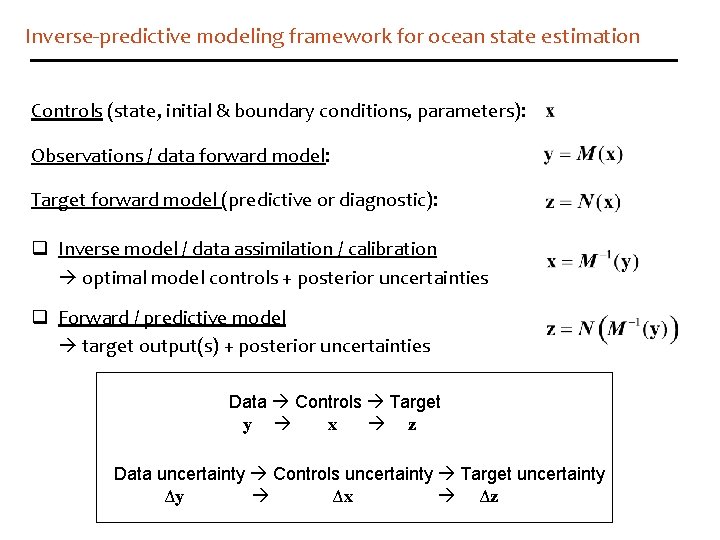
Inverse-predictive modeling framework for ocean state estimation Controls (state, initial & boundary conditions, parameters): Observations / data forward model: Target forward model (predictive or diagnostic): q Inverse model / data assimilation / calibration optimal model controls + posterior uncertainties q Forward / predictive model target output(s) + posterior uncertainties Data Controls Target y x z Data uncertainty Controls uncertainty Target uncertainty Δy Δx Δz
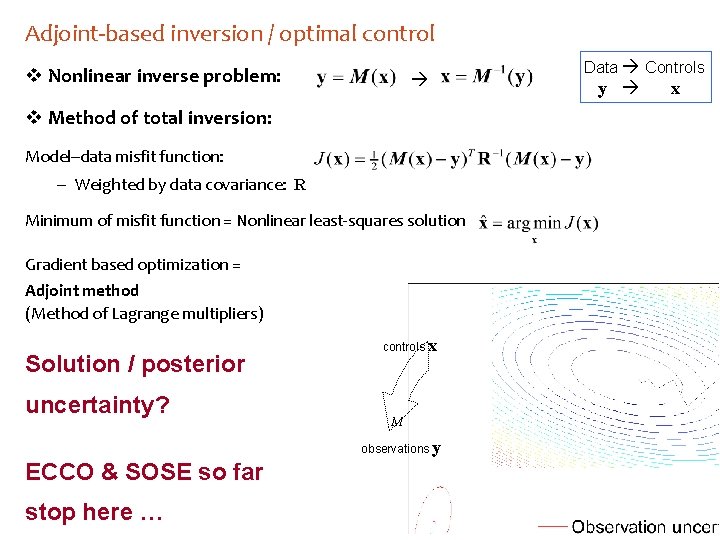
Adjoint-based inversion / optimal control v Nonlinear inverse problem: v Method of total inversion: Model–data misfit function: – Weighted by data covariance: R Minimum of misfit function = Nonlinear least-squares solution Gradient based optimization = Adjoint method (Method of Lagrange multipliers) Solution / posterior uncertainty? controls x M observations y ECCO & SOSE so far stop here … Data Controls y x
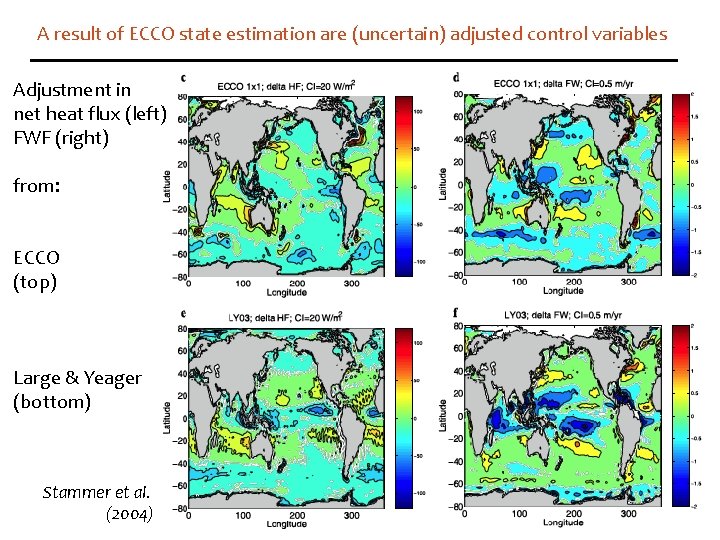
A result of ECCO state estimation are (uncertain) adjusted control variables Adjustment in net heat flux (left) FWF (right) from: ECCO (top) Large & Yeager (bottom) Stammer et al. (2004)
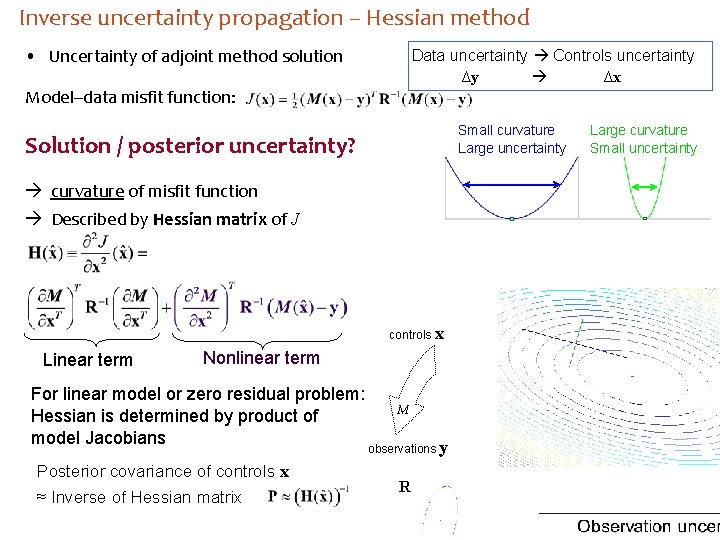
Inverse uncertainty propagation – Hessian method • Uncertainty of adjoint method solution Data uncertainty Controls uncertainty Δy Δx Model–data misfit function: Small curvature Large uncertainty Solution / posterior uncertainty? curvature of misfit function Described by Hessian matrix of J controls x Linear term Nonlinear term For linear model or zero residual problem: Hessian is determined by product of model Jacobians Posterior covariance of controls x ≈ Inverse of Hessian matrix M observations y R Large curvature Small uncertainty
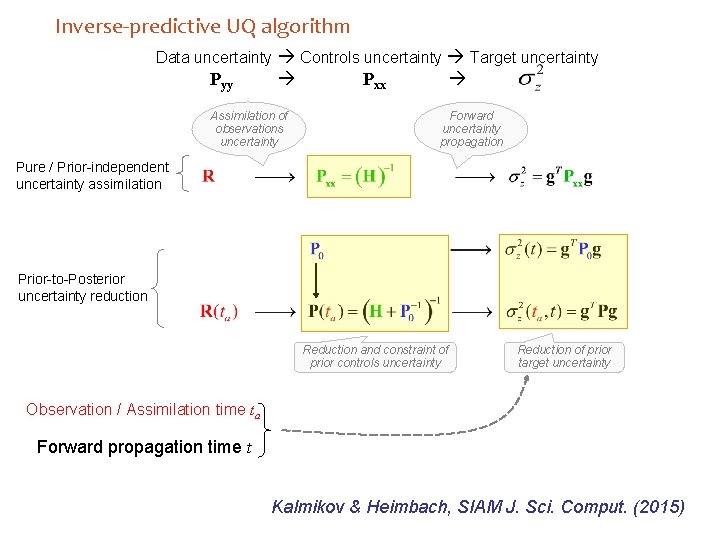
Inverse-predictive UQ algorithm Data uncertainty Controls uncertainty Target uncertainty Pyy Assimilation of observations uncertainty Pxx Forward uncertainty propagation Pure / Prior-independent uncertainty assimilation Prior-to-Posterior uncertainty reduction Reduction and constraint of prior controls uncertainty Reduction of prior target uncertainty Observation / Assimilation time ta Forward propagation time t Kalmikov & Heimbach, SIAM J. Sci. Comput. (2015)
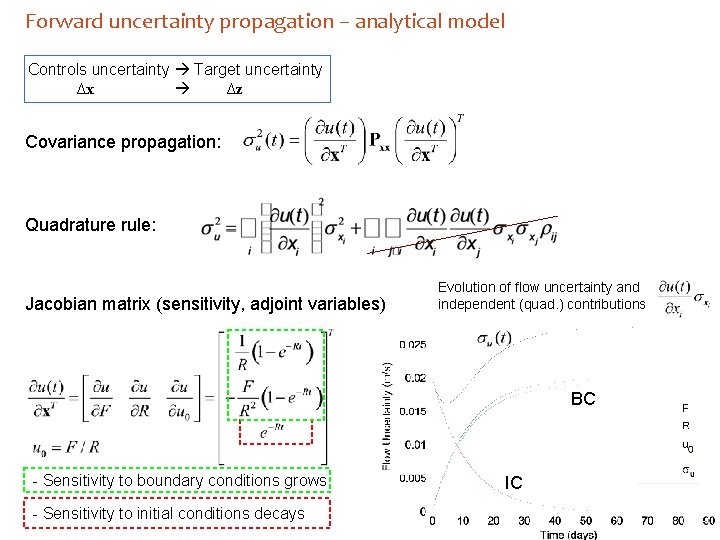
Forward uncertainty propagation – analytical model Controls uncertainty Target uncertainty Δx Δz Covariance propagation: Quadrature rule: Jacobian matrix (sensitivity, adjoint variables) Evolution of flow uncertainty and independent (quad. ) contributions BC - Sensitivity to boundary conditions grows - Sensitivity to initial conditions decays IC
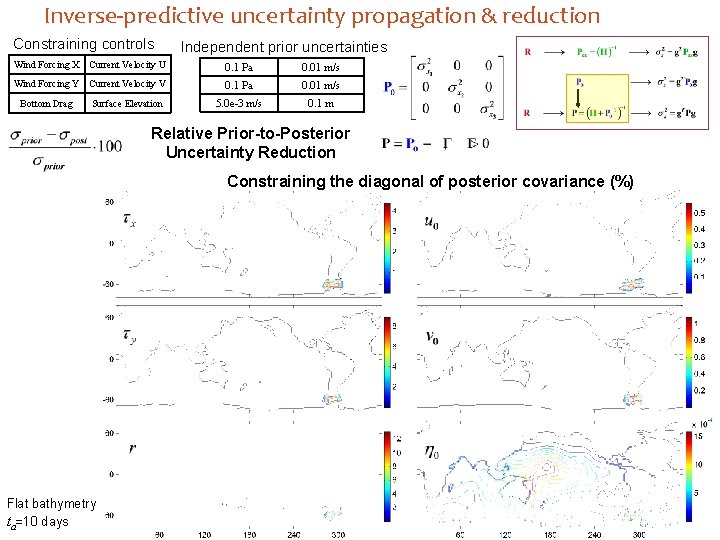
Inverse-predictive uncertainty propagation & reduction Constraining controls Independent prior uncertainties Wind Forcing X Current Velocity U 0. 1 Pa 0. 01 m/s Wind Forcing Y Current Velocity V 0. 1 Pa 0. 01 m/s 5. 0 e-3 m/s 0. 1 m Bottom Drag Surface Elevation Relative Prior-to-Posterior Uncertainty Reduction Constraining the diagonal of posterior covariance (%) Flat bathymetry ta=10 days
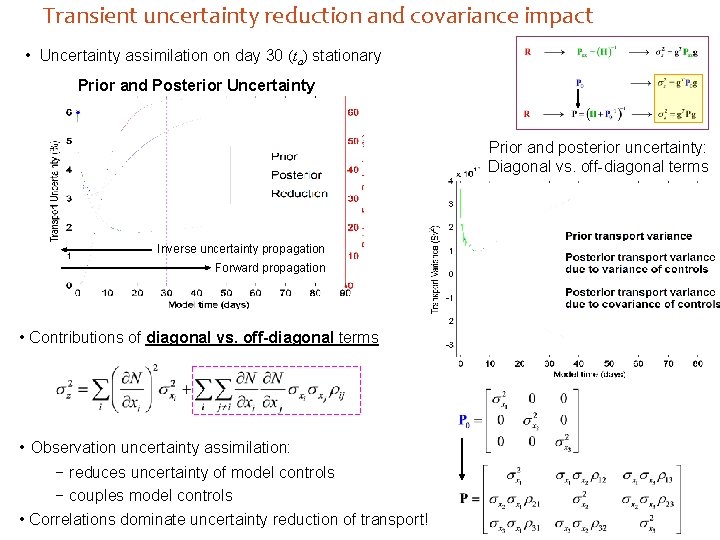
Transient uncertainty reduction and covariance impact • Uncertainty assimilation on day 30 (ta) stationary Prior and Posterior Uncertainty Prior and posterior uncertainty: Diagonal vs. off-diagonal terms Inverse uncertainty propagation Forward propagation • Contributions of diagonal vs. off-diagonal terms • Observation uncertainty assimilation: − reduces uncertainty of model controls − couples model controls • Correlations dominate uncertainty reduction of transport!
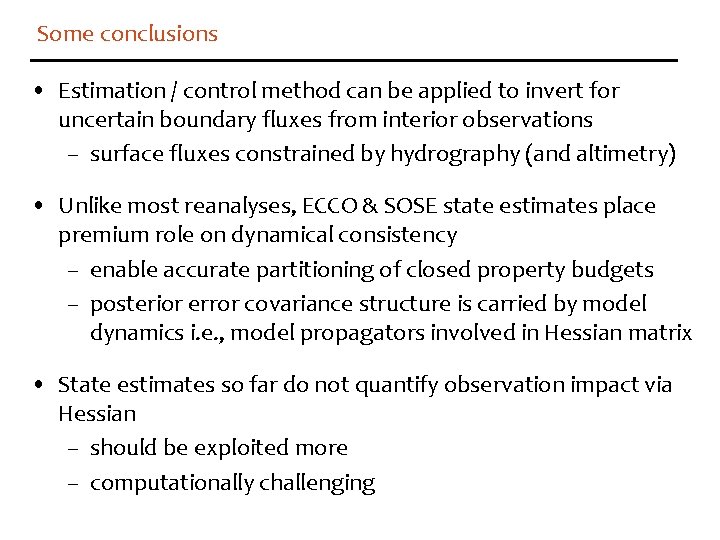
Some conclusions • Estimation / control method can be applied to invert for uncertain boundary fluxes from interior observations – surface fluxes constrained by hydrography (and altimetry) • Unlike most reanalyses, ECCO & SOSE state estimates place premium role on dynamical consistency – enable accurate partitioning of closed property budgets – posterior error covariance structure is carried by model dynamics i. e. , model propagators involved in Hessian matrix • State estimates so far do not quantify observation impact via Hessian – should be exploited more – computationally challenging
The giver character analysis
Attach additional responsibilities to an object dynamically
Dynamically continuous innovation examples
Dynamic code loading
Dynamically continuous innovation
Dynamically continuous innovation
What is global state in distributed system
Divergent plate boundary
Ocean ocean convergent boundary
Convergent boundary
Ocean ocean convergent boundary
Chapter 15 ocean water and ocean life wordwise answer key
Convergent continental boundary
Convergent oceanic oceanic plate boundary
Red ocean blue ocean strategi
Prediction reading strategy
Making inferences
Rudimentary rules
Making inferences
The grass is wet observation or inference
Inferring
Tone mood and purpose
Lancelet cladogram
Inferring networks of diffusion and influence
Through one man sin entered the world, and through one man