HighThroughput Machine Learning from EHR Data David Page
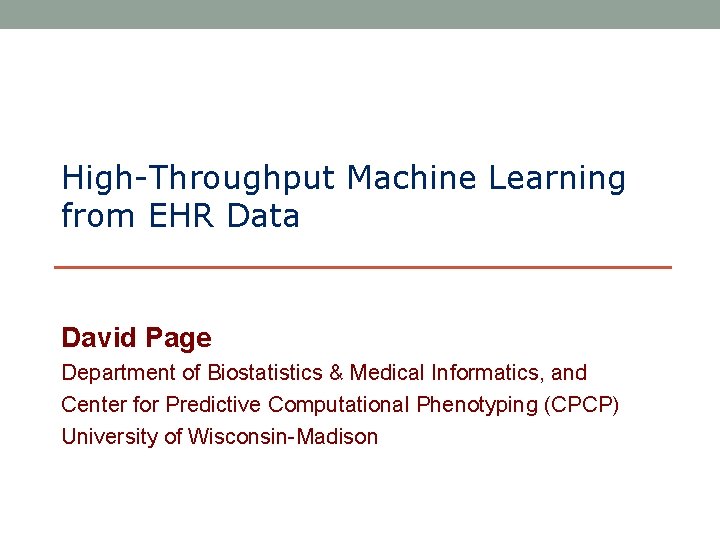
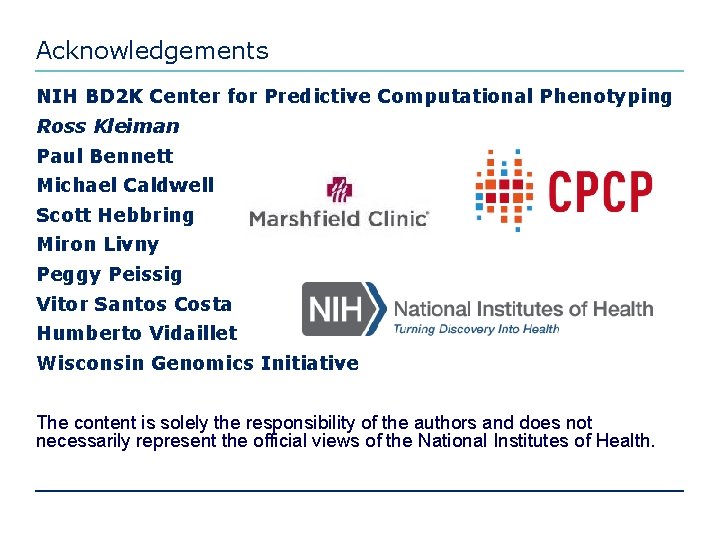
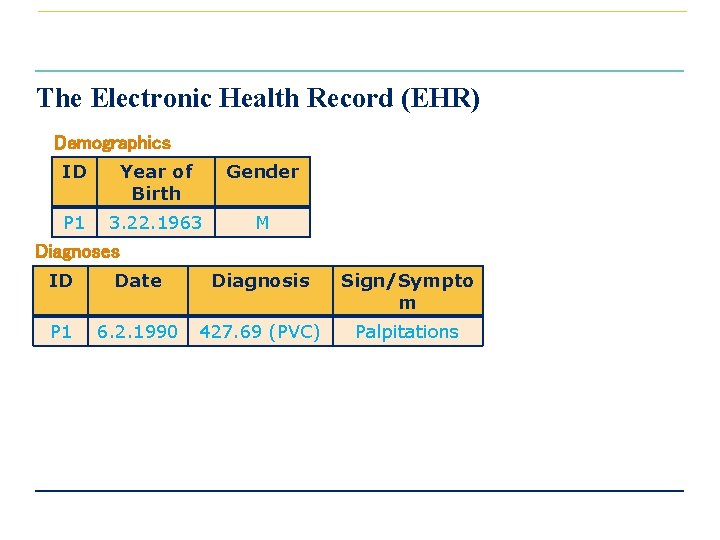
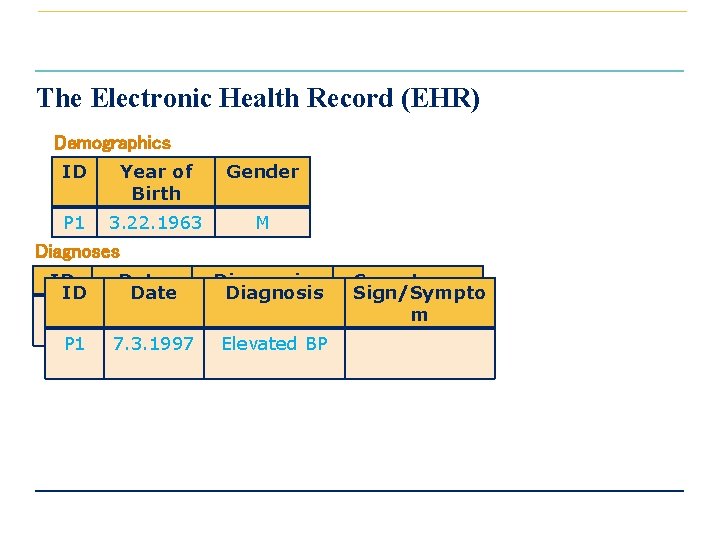
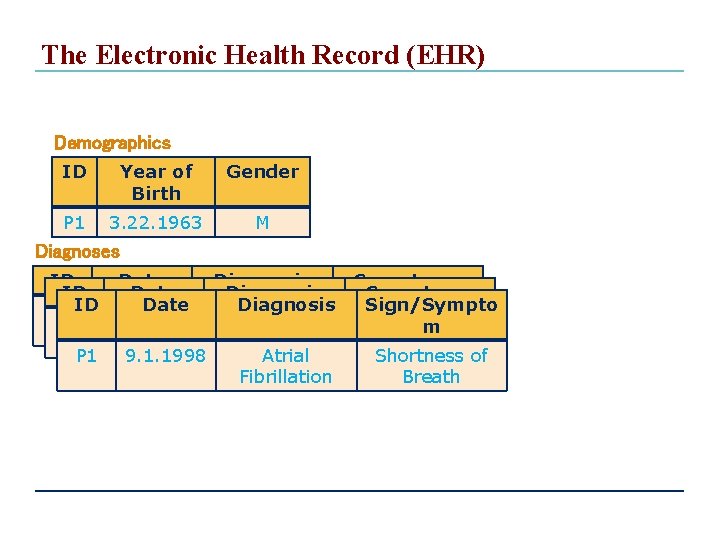
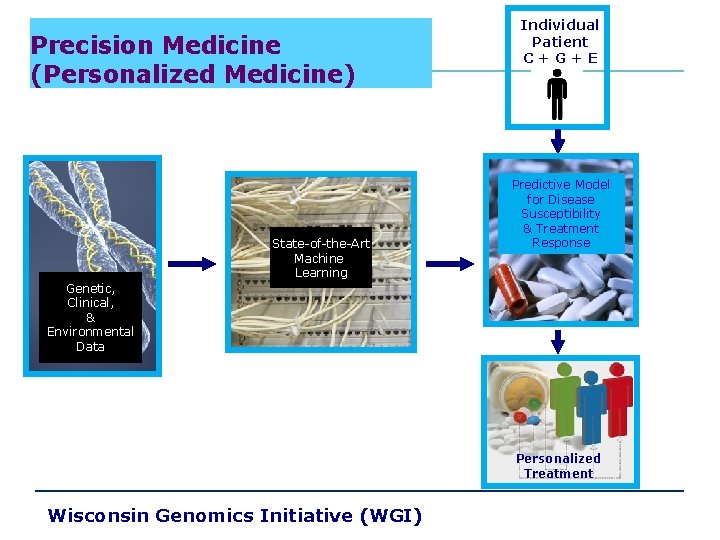
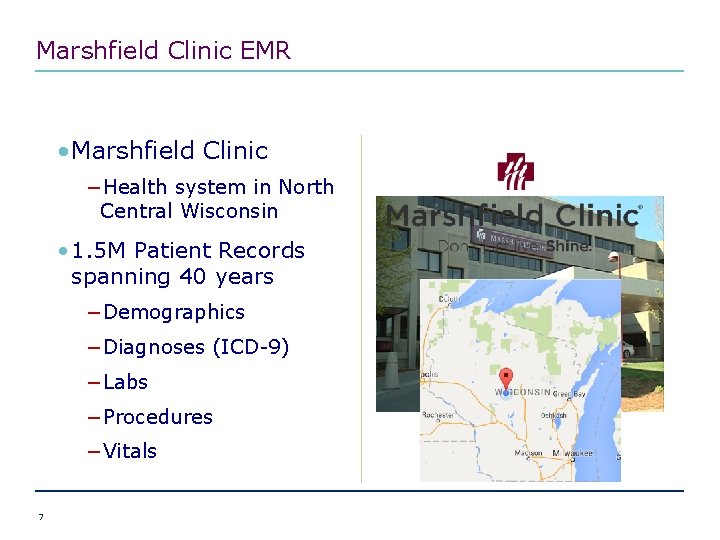
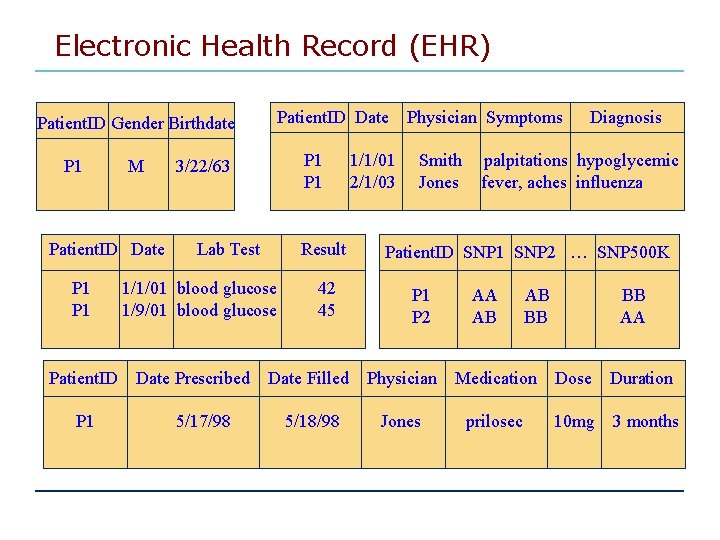
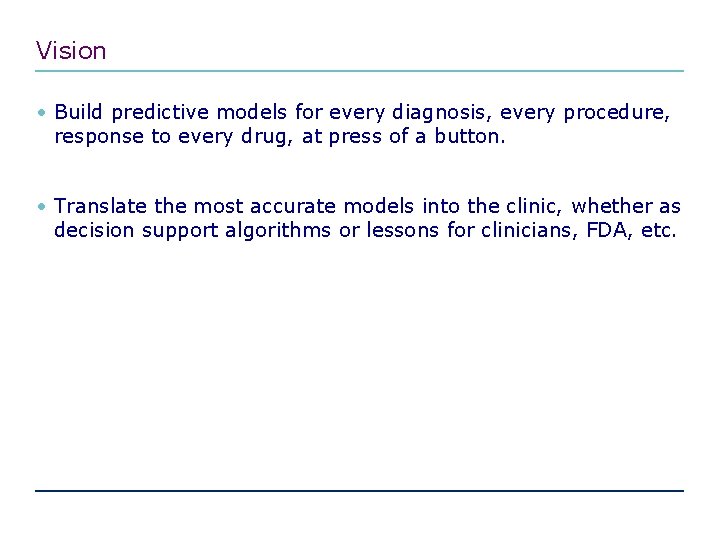
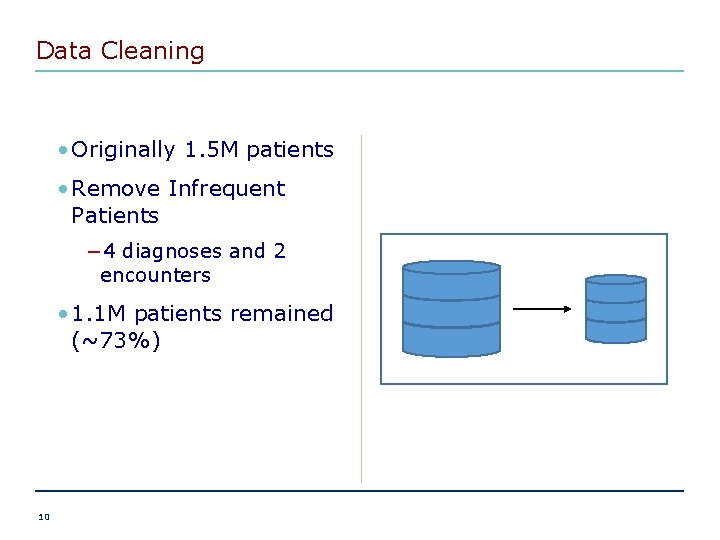
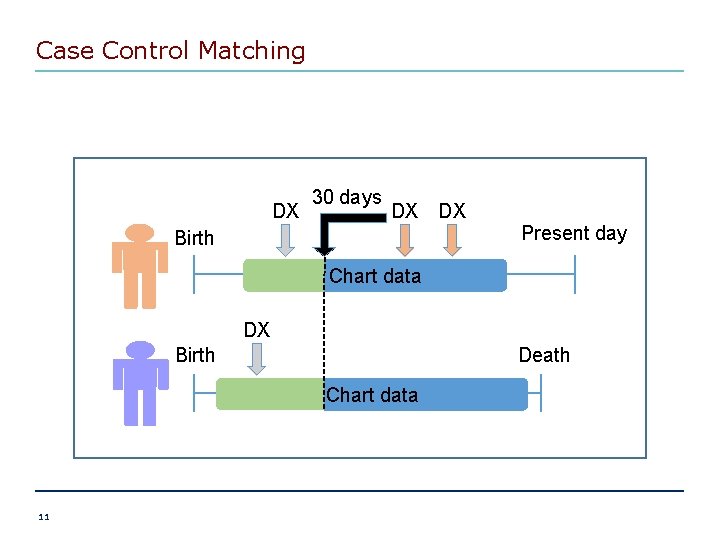
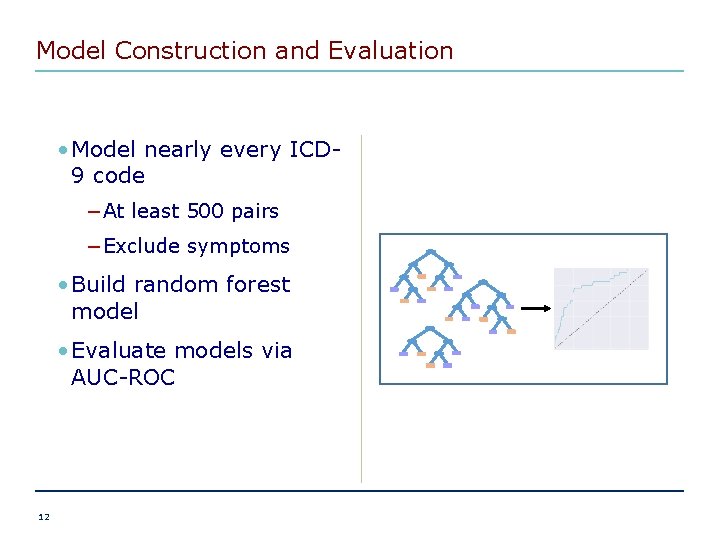
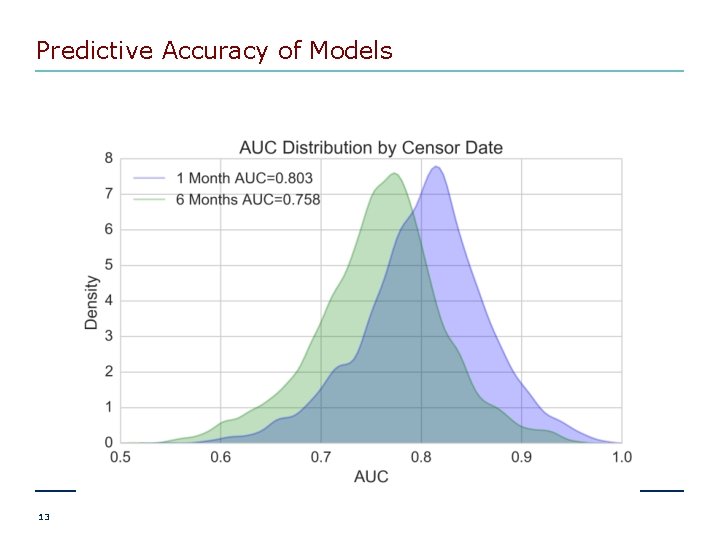
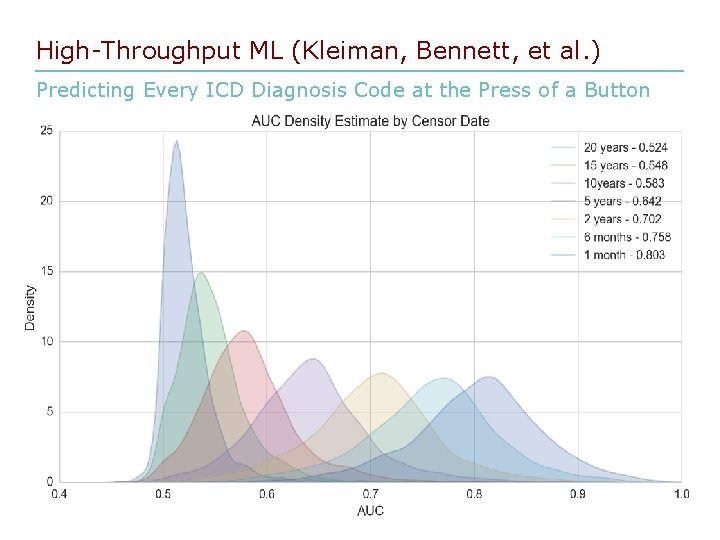
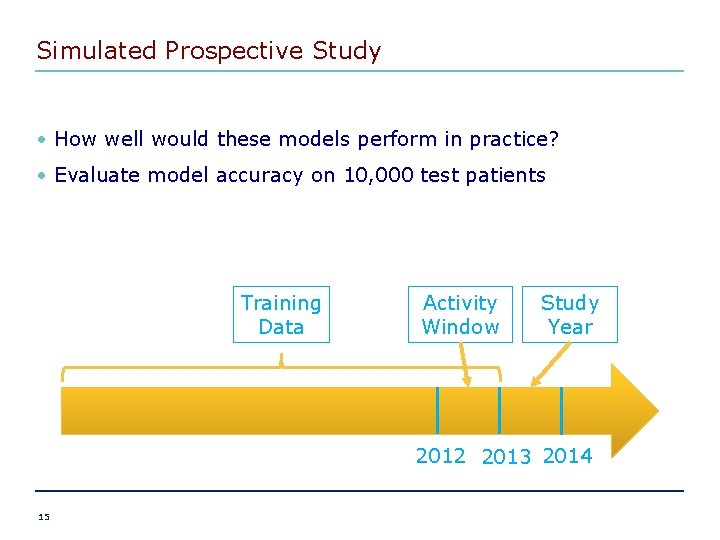
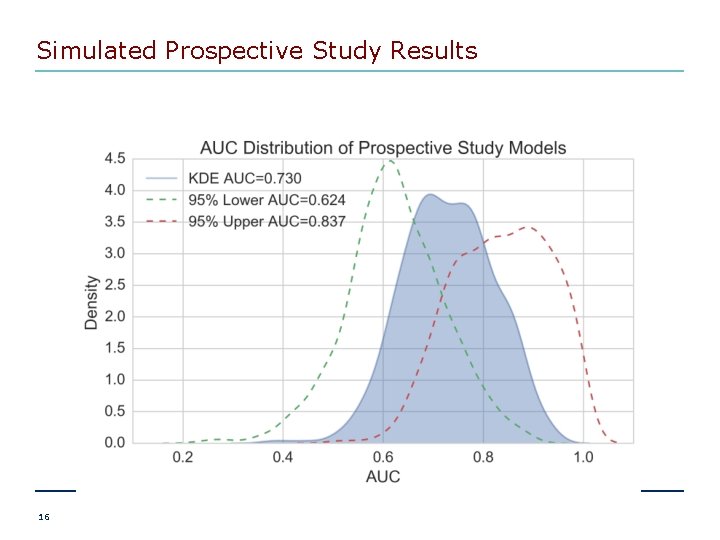
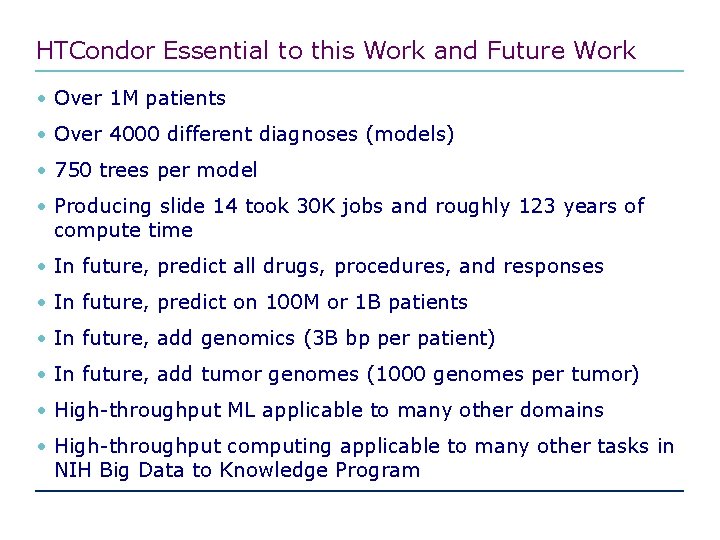
- Slides: 17
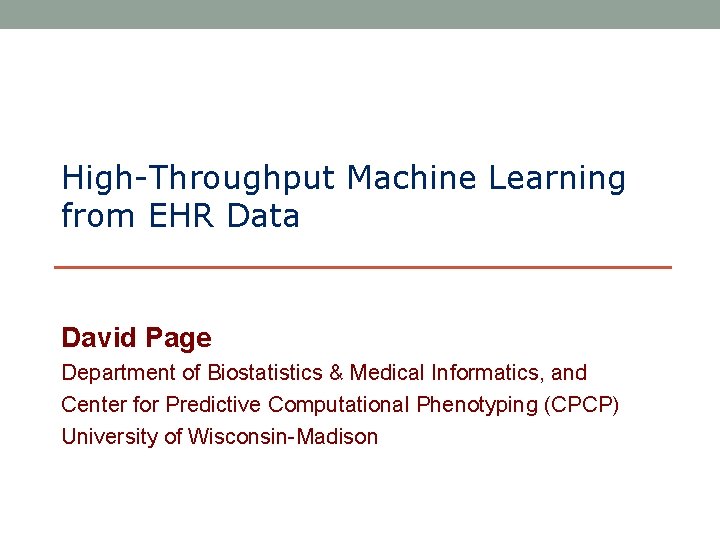
High-Throughput Machine Learning from EHR Data David Page Department of Biostatistics & Medical Informatics, and Center for Predictive Computational Phenotyping (CPCP) University of Wisconsin-Madison
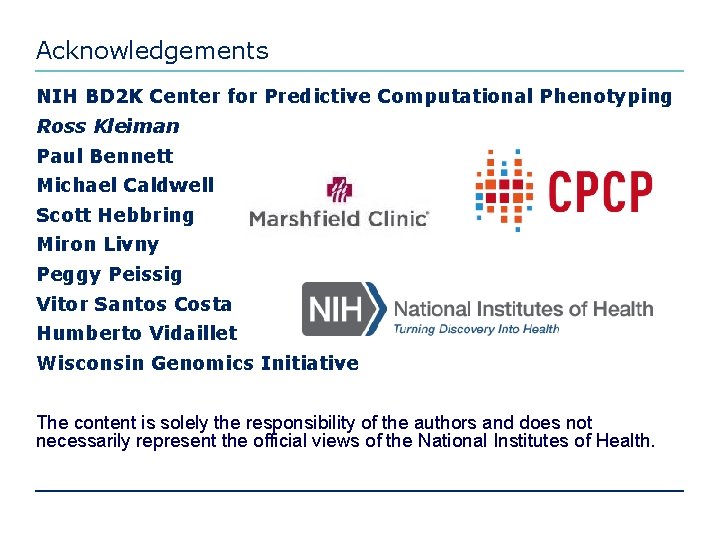
Acknowledgements NIH BD 2 K Center for Predictive Computational Phenotyping Ross Kleiman Paul Bennett Michael Caldwell Scott Hebbring Miron Livny Peggy Peissig Vitor Santos Costa Humberto Vidaillet Wisconsin Genomics Initiative The content is solely the responsibility of the authors and does not necessarily represent the official views of the National Institutes of Health.
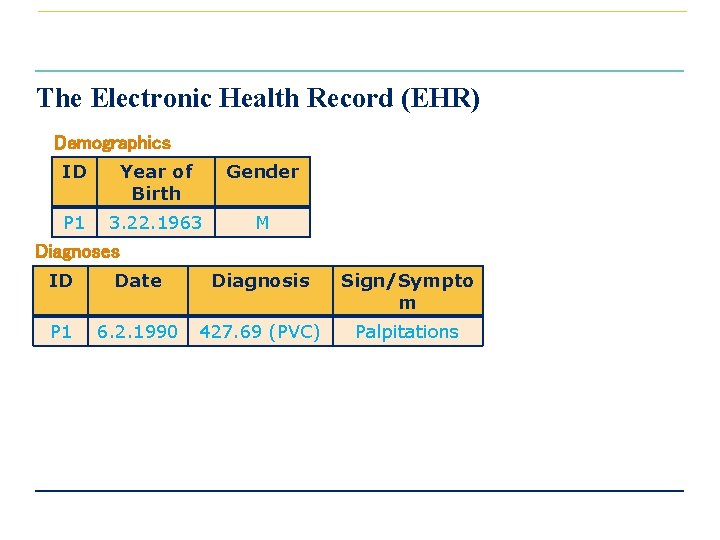
The Electronic Health Record (EHR) Demographics ID Year of Birth Gender P 1 3. 22. 1963 M Diagnoses ID Date Diagnosis Sign/Sympto m P 1 6. 2. 1990 427. 69 (PVC) Palpitations
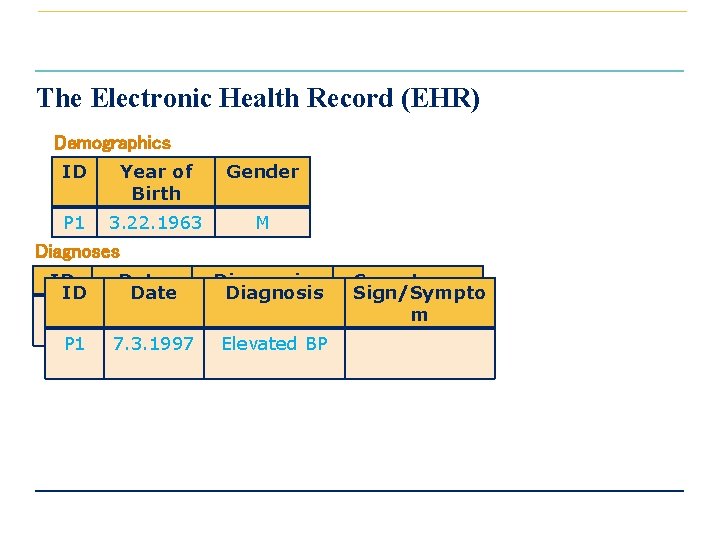
The Electronic Health Record (EHR) Demographics ID Year of Birth Gender P 1 3. 22. 1963 M Diagnoses ID Date P 1 2011. 06. 02 P 1 7. 3. 1997 Diagnosis Atrial fibrillation Elevated BP Symptoms Sign/Sympto Dizzy, m discomfort
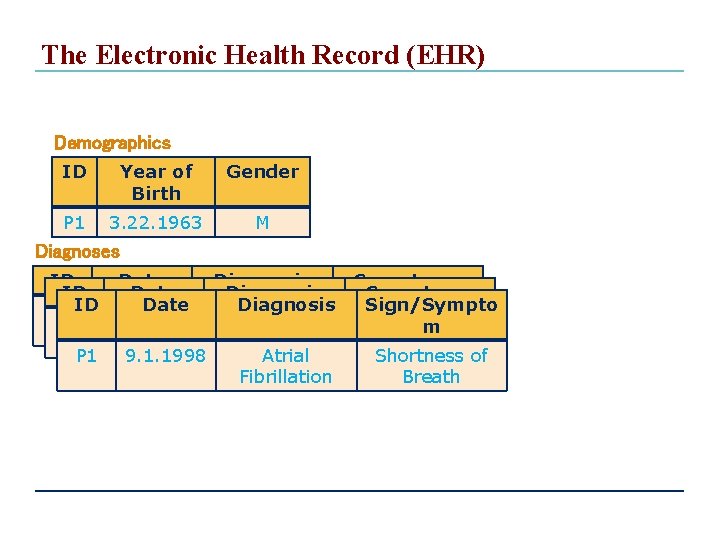
The Electronic Health Record (EHR) Demographics ID Year of Birth Gender P 1 3. 22. 1963 M Diagnoses ID Date Diagnosis P 1 2011. 06. Atrial 02 fibrillation P 1 9. 1. 1998 Atrial Fibrillation Symptoms Sign/Sympto Dizzy, m discomfort Shortness of Breath
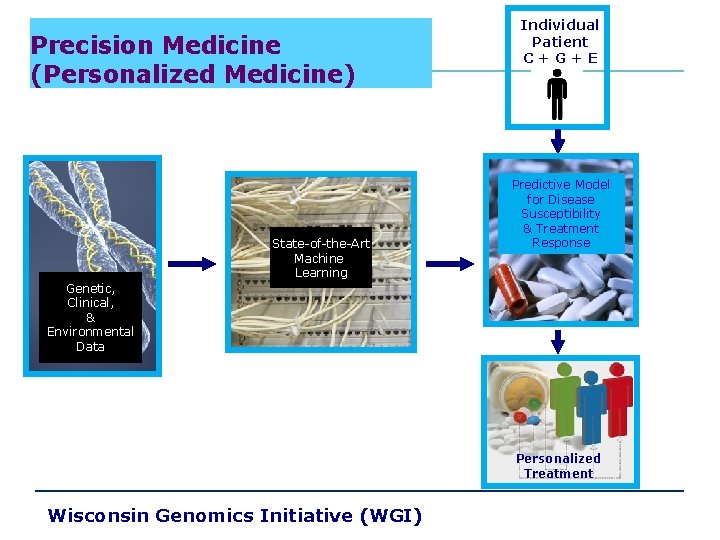
Precision Medicine (Personalized Medicine) State-of-the-Art Machine Learning Individual Patient C+G+E Predictive Model for Disease Susceptibility & Treatment Response Genetic, Clinical, & Environmental Data Personalized Treatment Wisconsin Genomics Initiative (WGI)
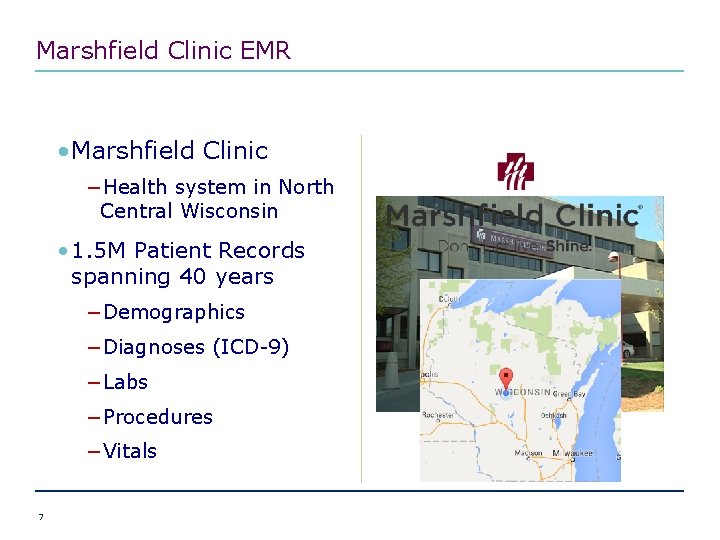
Marshfield Clinic EMR • Marshfield Clinic −Health system in North Central Wisconsin • 1. 5 M Patient Records spanning 40 years −Demographics −Diagnoses (ICD-9) −Labs −Procedures −Vitals 7
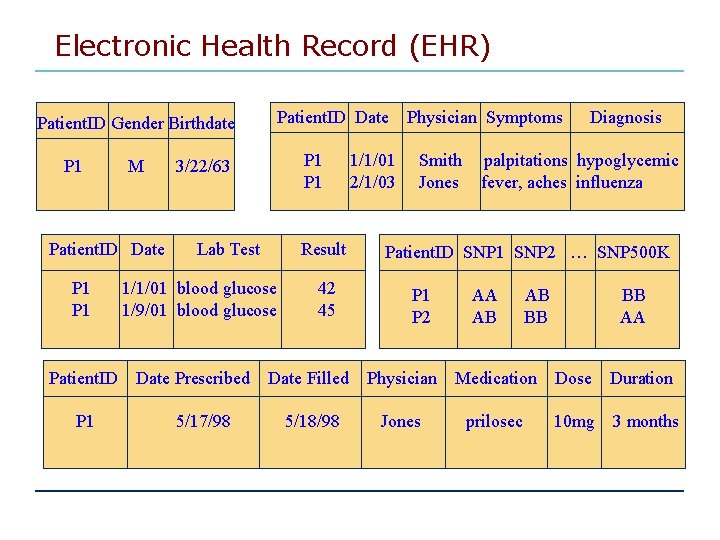
Electronic Health Record (EHR) Patient. ID Gender Birthdate P 1 M Patient. ID Date P 1 Patient. ID Date P 1 3/22/63 Lab Test Result 1/1/01 blood glucose 1/9/01 blood glucose Date Prescribed 5/17/98 42 45 1/1/01 2/1/03 Physician Symptoms Smith Jones Diagnosis palpitations hypoglycemic fever, aches influenza Patient. ID SNP 1 SNP 2 … SNP 500 K P 1 P 2 AA AB AB BB BB AA Date Filled Physician Medication Dose Duration 5/18/98 Jones prilosec 10 mg 3 months
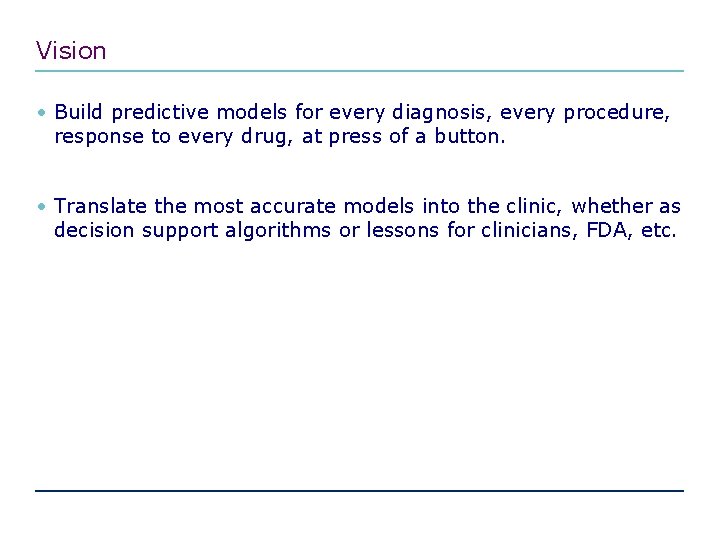
Vision • Build predictive models for every diagnosis, every procedure, response to every drug, at press of a button. • Translate the most accurate models into the clinic, whether as decision support algorithms or lessons for clinicians, FDA, etc.
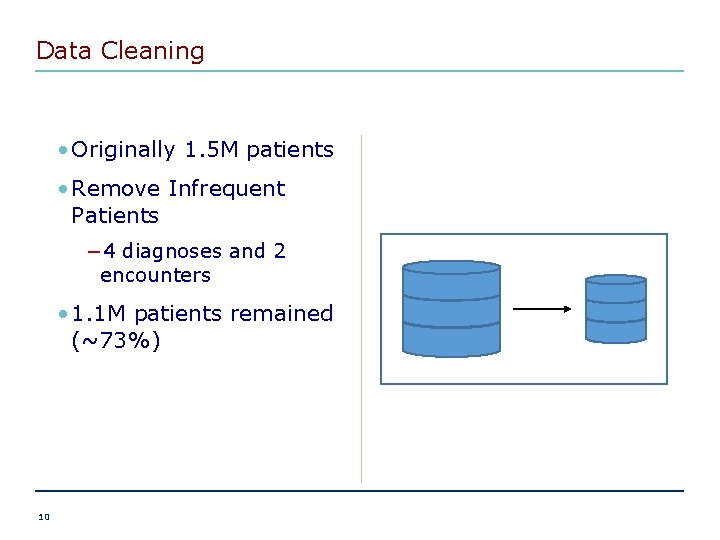
Data Cleaning • Originally 1. 5 M patients • Remove Infrequent Patients − 4 diagnoses and 2 encounters • 1. 1 M patients remained (~73%) 10
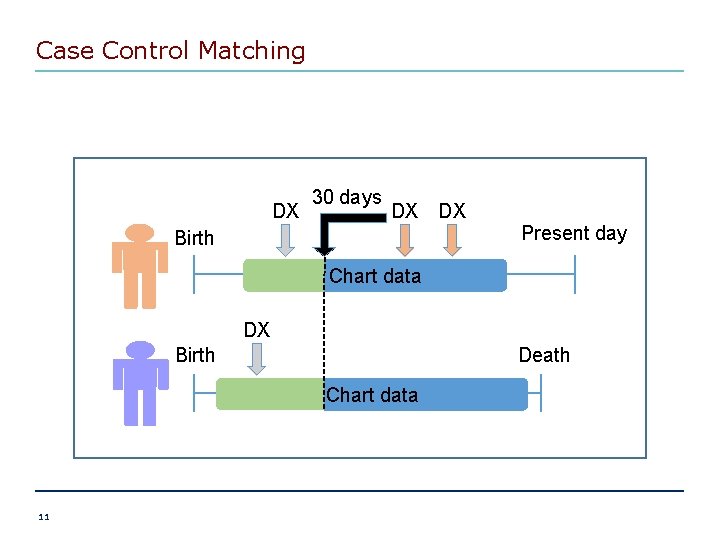
Case Control Matching DX 30 days DX DX Birth Present day Chart data Birth DX +++++ Death Chart data 11
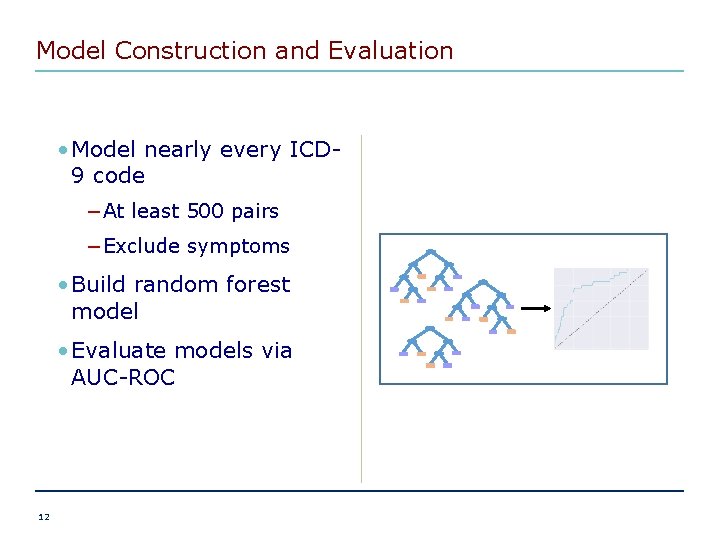
Model Construction and Evaluation • Model nearly every ICD 9 code −At least 500 pairs −Exclude symptoms • Build random forest model • Evaluate models via AUC-ROC 12
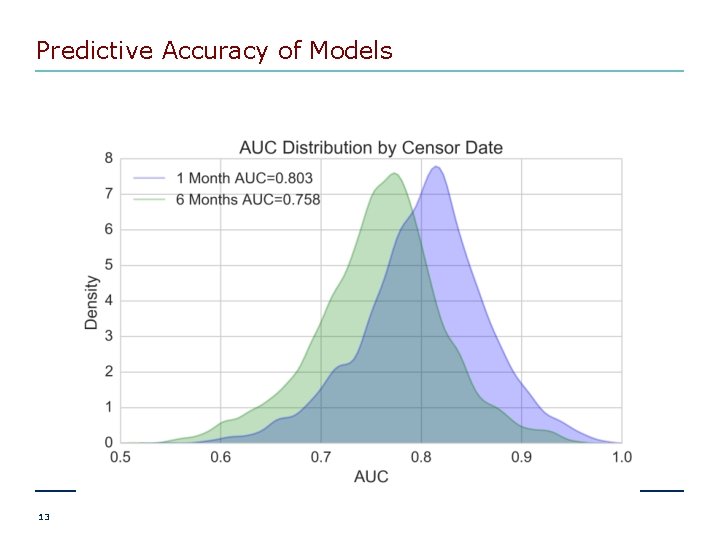
Predictive Accuracy of Models 13
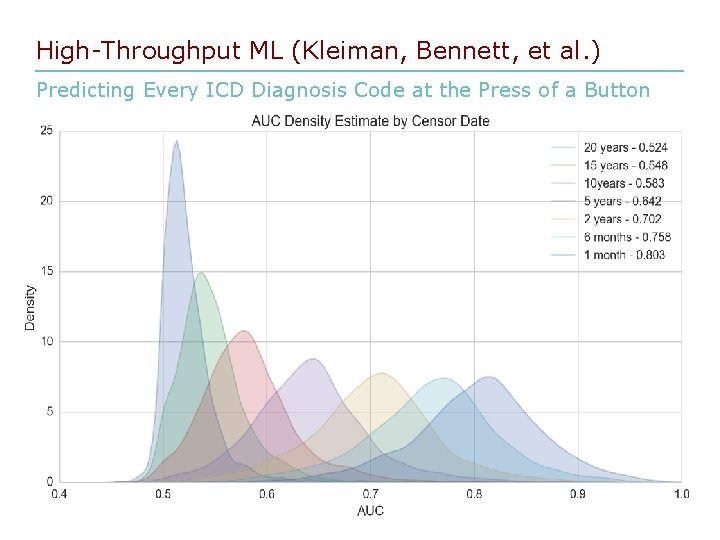
High-Throughput ML (Kleiman, Bennett, et al. ) Predicting Every ICD Diagnosis Code at the Press of a Button
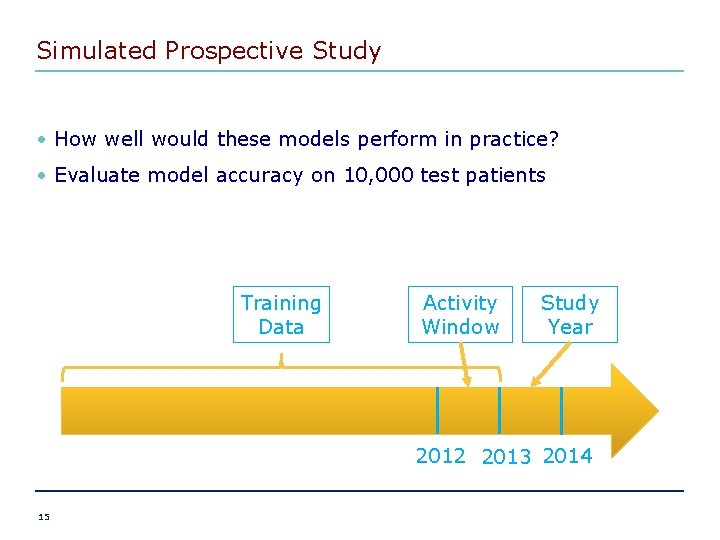
Simulated Prospective Study • How well would these models perform in practice? • Evaluate model accuracy on 10, 000 test patients Training Data Activity Window Study Year 2012 2013 2014 15
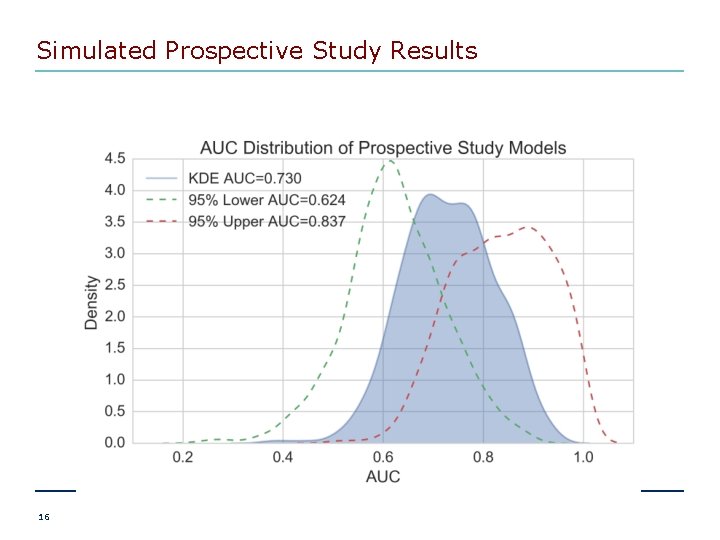
Simulated Prospective Study Results 16
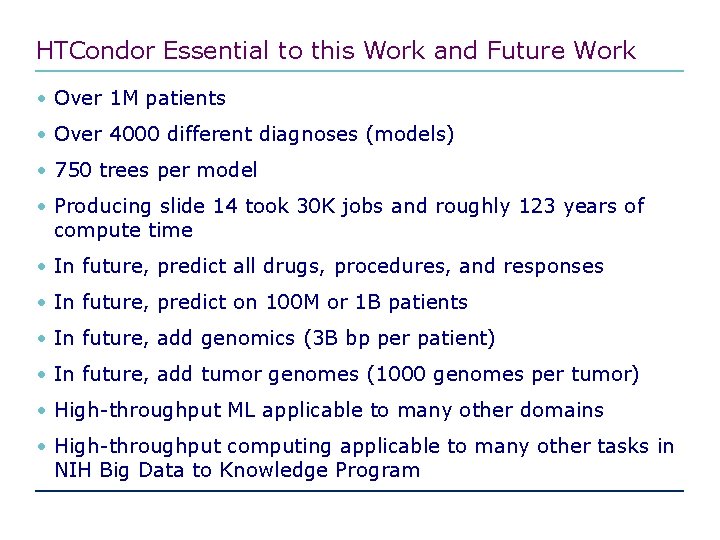
HTCondor Essential to this Work and Future Work • Over 1 M patients • Over 4000 different diagnoses (models) • 750 trees per model • Producing slide 14 took 30 K jobs and roughly 123 years of compute time • In future, predict all drugs, procedures, and responses • In future, predict on 100 M or 1 B patients • In future, add genomics (3 B bp per patient) • In future, add tumor genomes (1000 genomes per tumor) • High-throughput ML applicable to many other domains • High-throughput computing applicable to many other tasks in NIH Big Data to Knowledge Program
Concept learning task in machine learning
Analytical learning in machine learning
Pac learning model in machine learning
Pac learning model in machine learning
Inductive and analytical learning
Inductive and analytical learning
Instance based learning in machine learning
Inductive learning machine learning
First order rule learning in machine learning
Eager learner and lazy learner
Deep learning vs machine learning
Cover page in apa format
Cuadro comparativo e-learning m-learning b-learning
The non-iid data quagmire of decentralized machine learning
Machine learning and data mining
Data mining azure
Training data in machine learning with example
David rockefeller memoirs page 405