HIA and MultiAgent Models New paradigm of heterogeneous
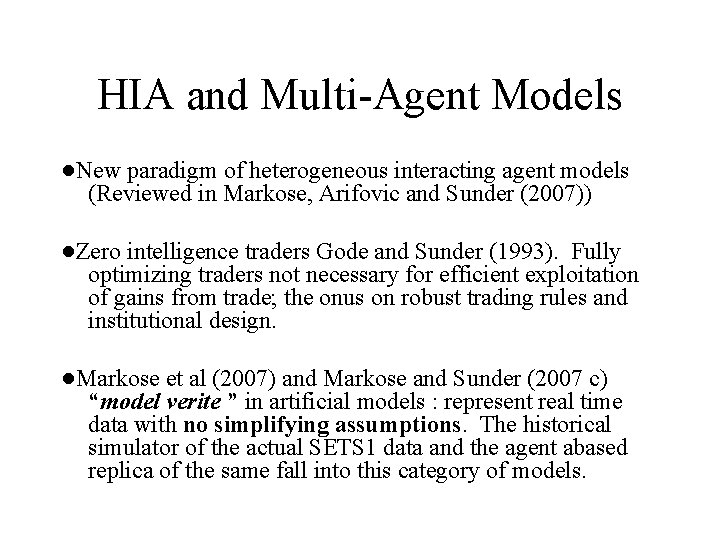
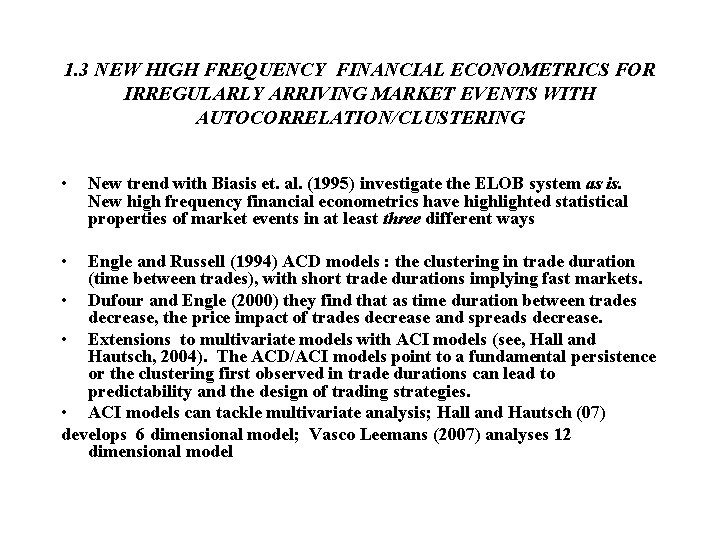
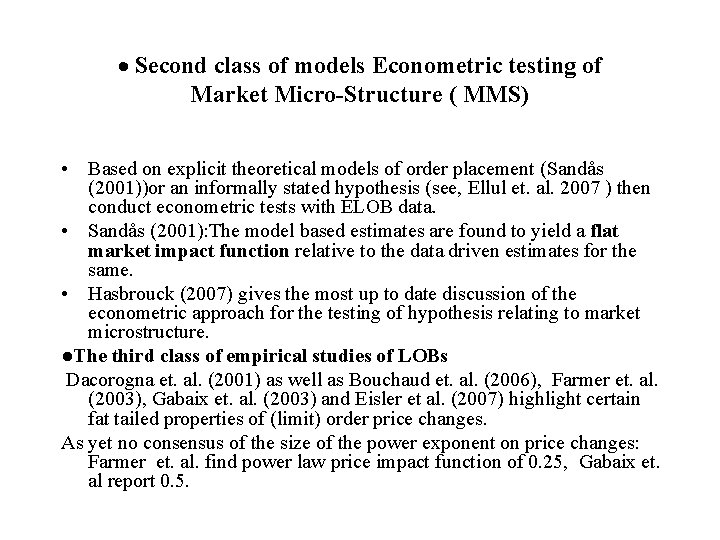
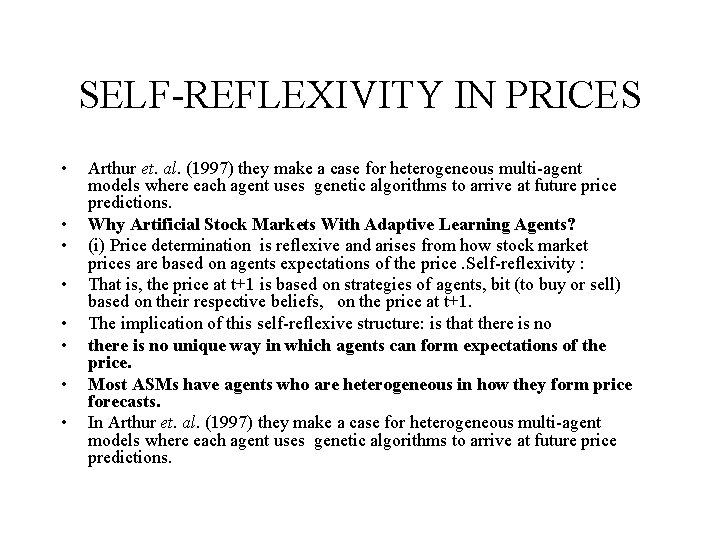
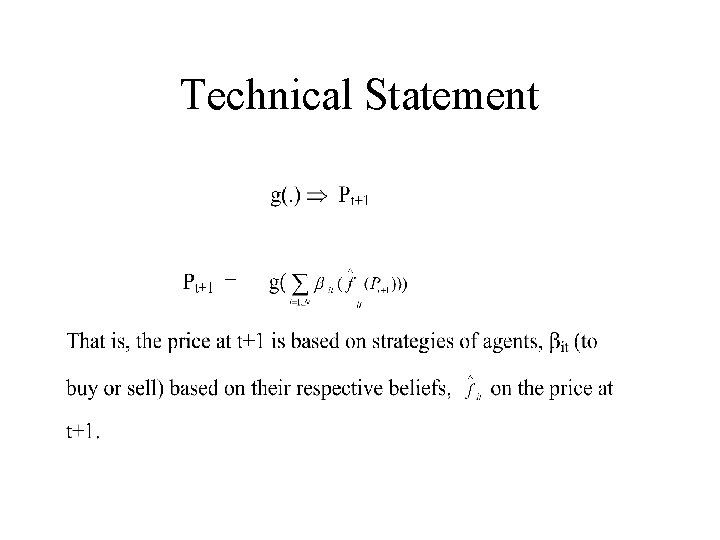
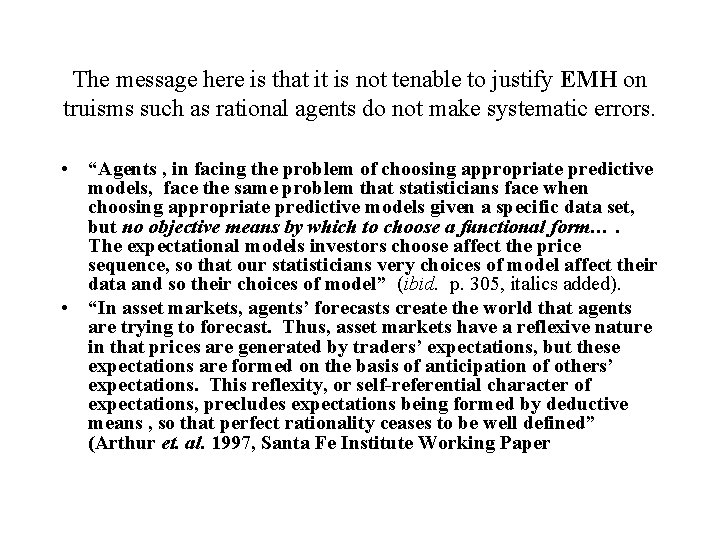
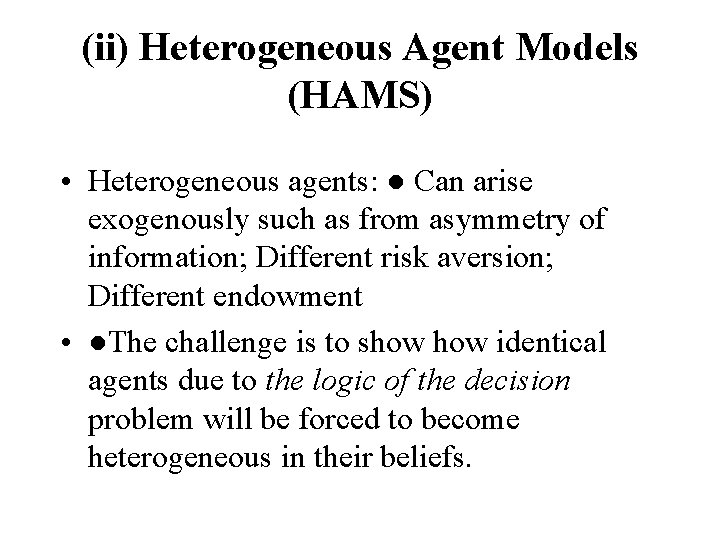
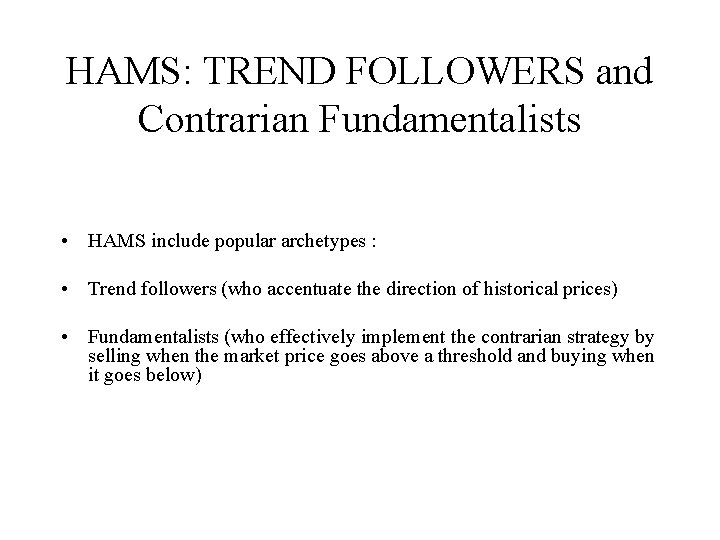
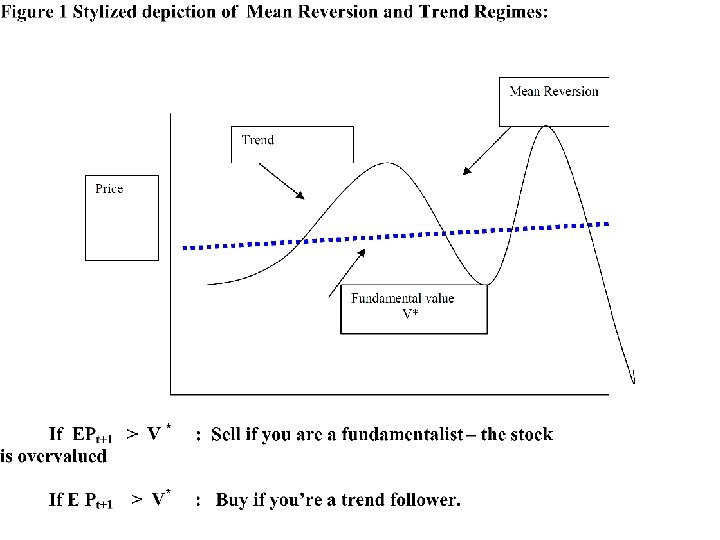
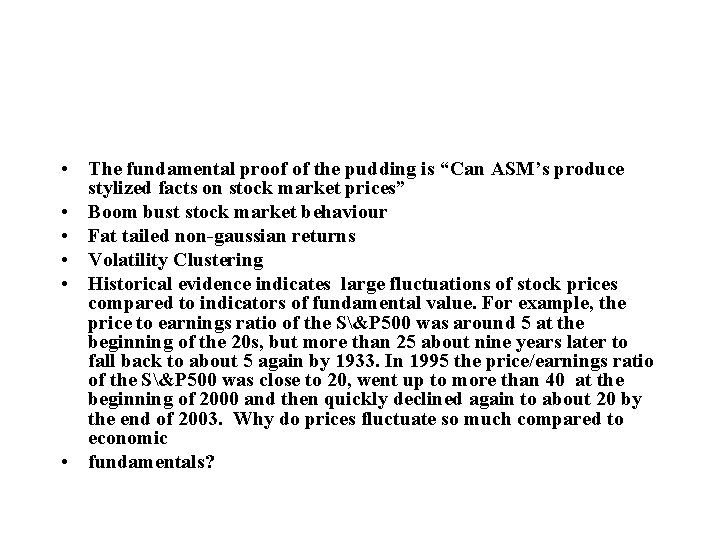
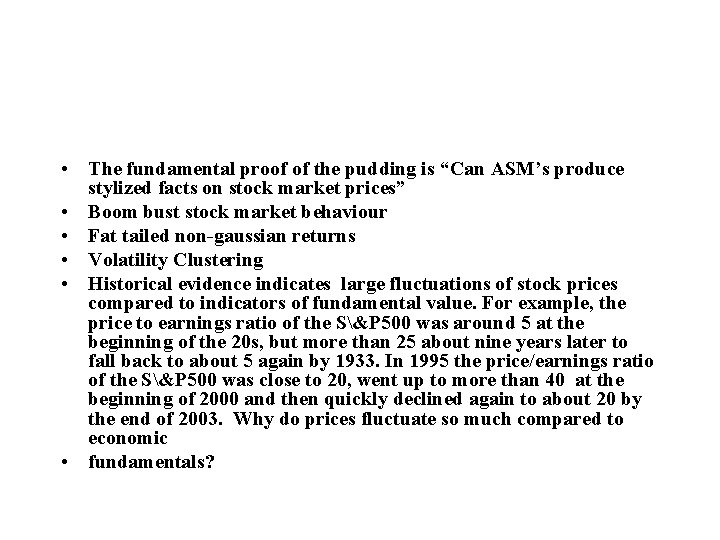
- Slides: 11
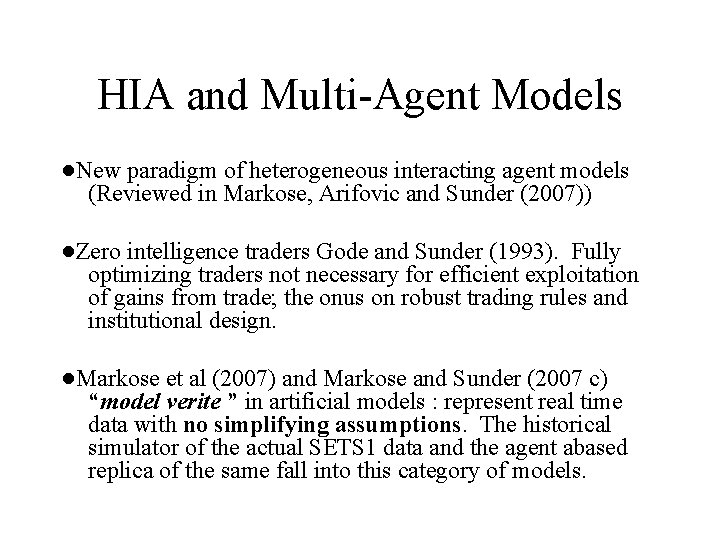
HIA and Multi-Agent Models ●New paradigm of heterogeneous interacting agent models (Reviewed in Markose, Arifovic and Sunder (2007)) ●Zero intelligence traders Gode and Sunder (1993). Fully optimizing traders not necessary for efficient exploitation of gains from trade; the onus on robust trading rules and institutional design. ●Markose et al (2007) and Markose and Sunder (2007 c) “model verite ” in artificial models : represent real time data with no simplifying assumptions. The historical simulator of the actual SETS 1 data and the agent abased replica of the same fall into this category of models.
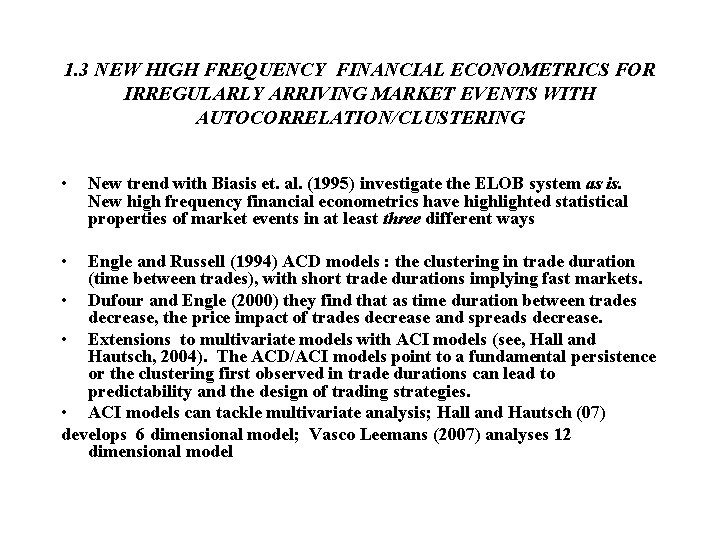
1. 3 NEW HIGH FREQUENCY FINANCIAL ECONOMETRICS FOR IRREGULARLY ARRIVING MARKET EVENTS WITH AUTOCORRELATION/CLUSTERING • • New trend with Biasis et. al. (1995) investigate the ELOB system as is. New high frequency financial econometrics have highlighted statistical properties of market events in at least three different ways Engle and Russell (1994) ACD models : the clustering in trade duration (time between trades), with short trade durations implying fast markets. • Dufour and Engle (2000) they find that as time duration between trades decrease, the price impact of trades decrease and spreads decrease. • Extensions to multivariate models with ACI models (see, Hall and Hautsch, 2004). The ACD/ACI models point to a fundamental persistence or the clustering first observed in trade durations can lead to predictability and the design of trading strategies. • ACI models can tackle multivariate analysis; Hall and Hautsch (07) develops 6 dimensional model; Vasco Leemans (2007) analyses 12 dimensional model
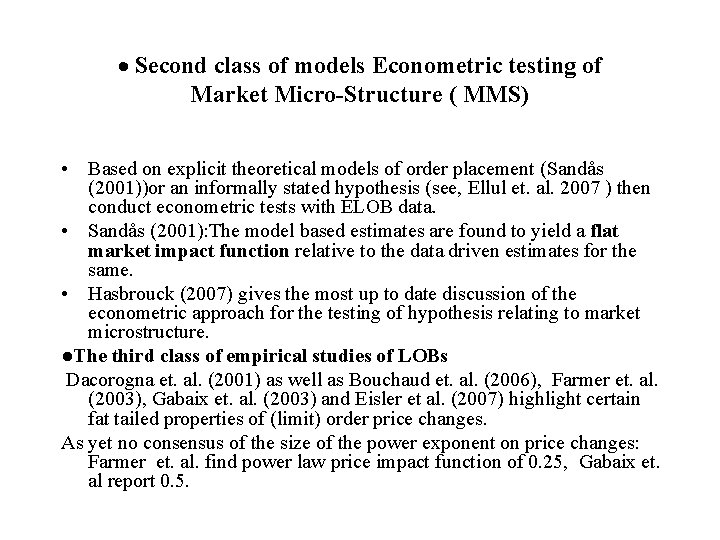
Second class of models Econometric testing of Market Micro-Structure ( MMS) • Based on explicit theoretical models of order placement (Sandås (2001))or an informally stated hypothesis (see, Ellul et. al. 2007 ) then conduct econometric tests with ELOB data. • Sandås (2001): The model based estimates are found to yield a flat market impact function relative to the data driven estimates for the same. • Hasbrouck (2007) gives the most up to date discussion of the econometric approach for the testing of hypothesis relating to market microstructure. ●The third class of empirical studies of LOBs Dacorogna et. al. (2001) as well as Bouchaud et. al. (2006), Farmer et. al. (2003), Gabaix et. al. (2003) and Eisler et al. (2007) highlight certain fat tailed properties of (limit) order price changes. As yet no consensus of the size of the power exponent on price changes: Farmer et. al. find power law price impact function of 0. 25, Gabaix et. al report 0. 5.
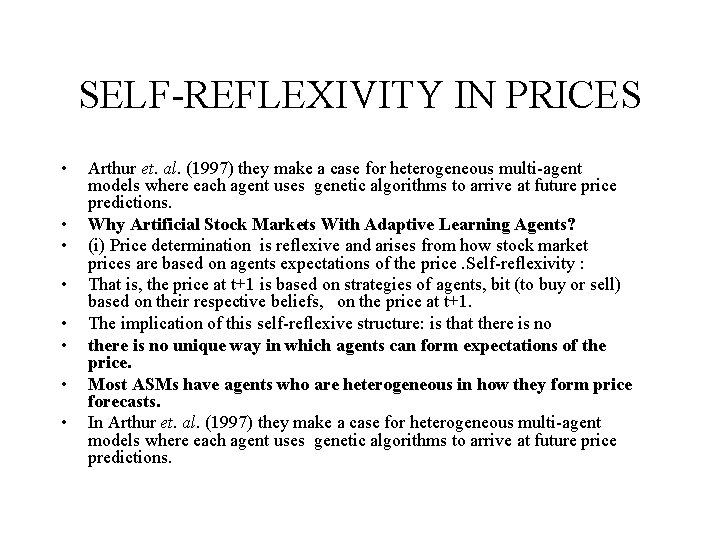
SELF-REFLEXIVITY IN PRICES • • Arthur et. al. (1997) they make a case for heterogeneous multi-agent models where each agent uses genetic algorithms to arrive at future price predictions. Why Artificial Stock Markets With Adaptive Learning Agents? (i) Price determination is reflexive and arises from how stock market prices are based on agents expectations of the price. Self-reflexivity : That is, the price at t+1 is based on strategies of agents, bit (to buy or sell) based on their respective beliefs, on the price at t+1. The implication of this self-reflexive structure: is that there is no unique way in which agents can form expectations of the price. Most ASMs have agents who are heterogeneous in how they form price forecasts. In Arthur et. al. (1997) they make a case for heterogeneous multi-agent models where each agent uses genetic algorithms to arrive at future price predictions.
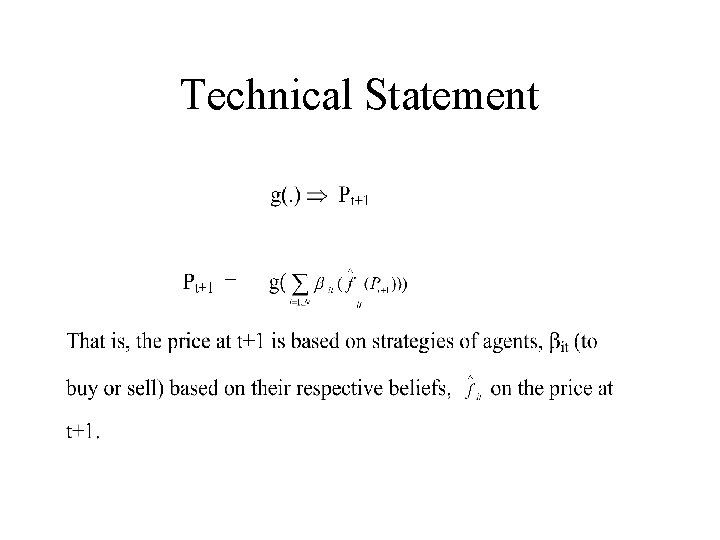
Technical Statement
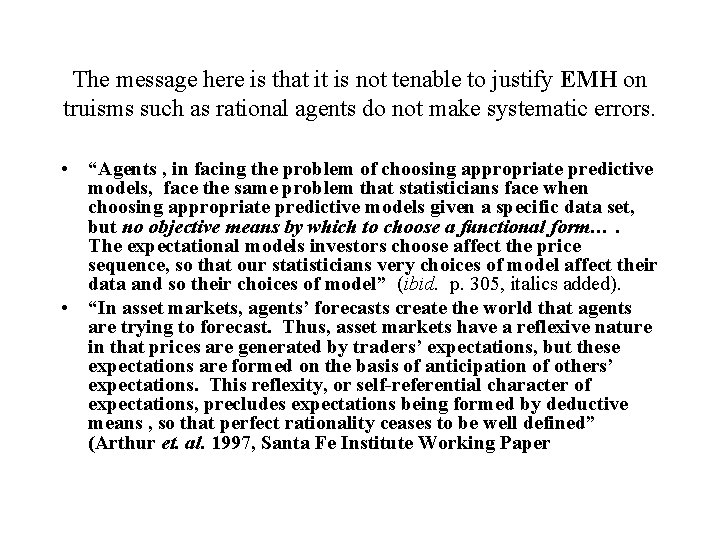
The message here is that it is not tenable to justify EMH on truisms such as rational agents do not make systematic errors. • “Agents , in facing the problem of choosing appropriate predictive models, face the same problem that statisticians face when choosing appropriate predictive models given a specific data set, but no objective means by which to choose a functional form…. The expectational models investors choose affect the price sequence, so that our statisticians very choices of model affect their data and so their choices of model” (ibid. p. 305, italics added). • “In asset markets, agents’ forecasts create the world that agents are trying to forecast. Thus, asset markets have a reflexive nature in that prices are generated by traders’ expectations, but these expectations are formed on the basis of anticipation of others’ expectations. This reflexity, or self-referential character of expectations, precludes expectations being formed by deductive means , so that perfect rationality ceases to be well defined” (Arthur et. al. 1997, Santa Fe Institute Working Paper
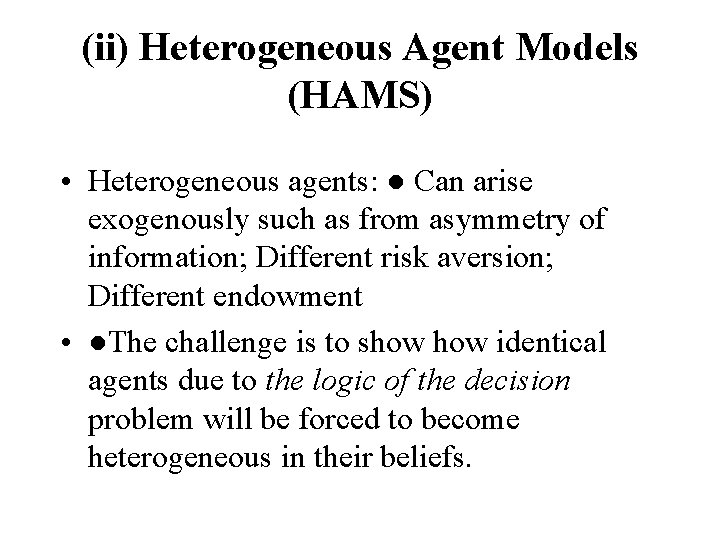
(ii) Heterogeneous Agent Models (HAMS) • Heterogeneous agents: ● Can arise exogenously such as from asymmetry of information; Different risk aversion; Different endowment • ●The challenge is to show identical agents due to the logic of the decision problem will be forced to become heterogeneous in their beliefs.
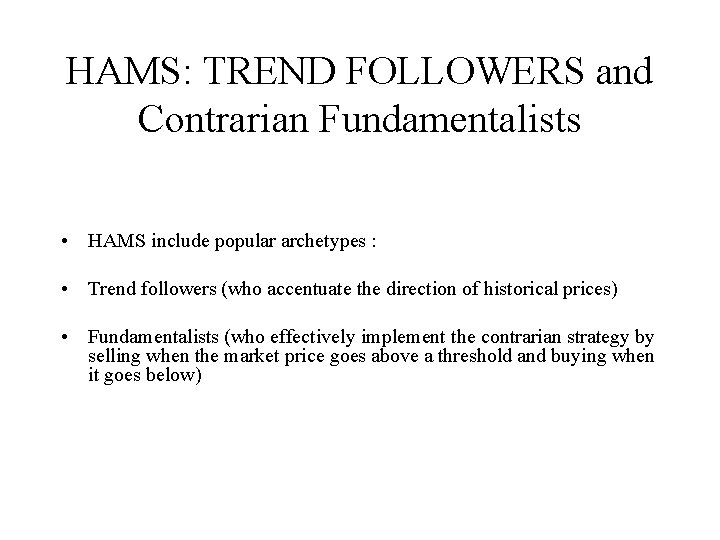
HAMS: TREND FOLLOWERS and Contrarian Fundamentalists • HAMS include popular archetypes : • Trend followers (who accentuate the direction of historical prices) • Fundamentalists (who effectively implement the contrarian strategy by selling when the market price goes above a threshold and buying when it goes below)
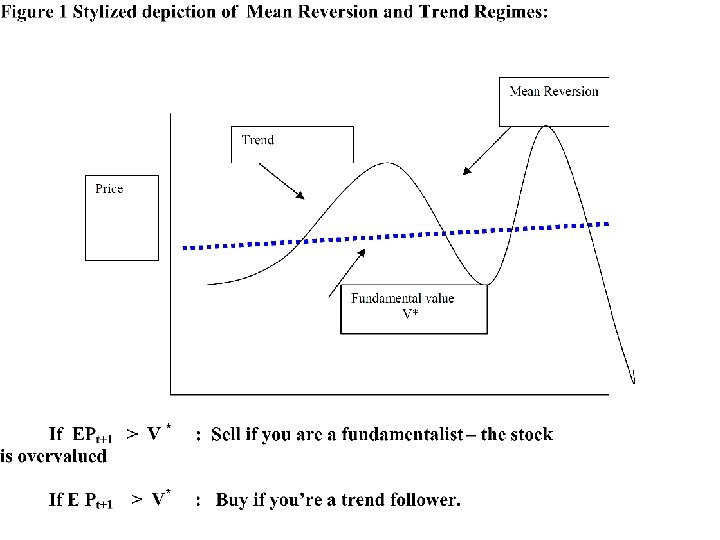
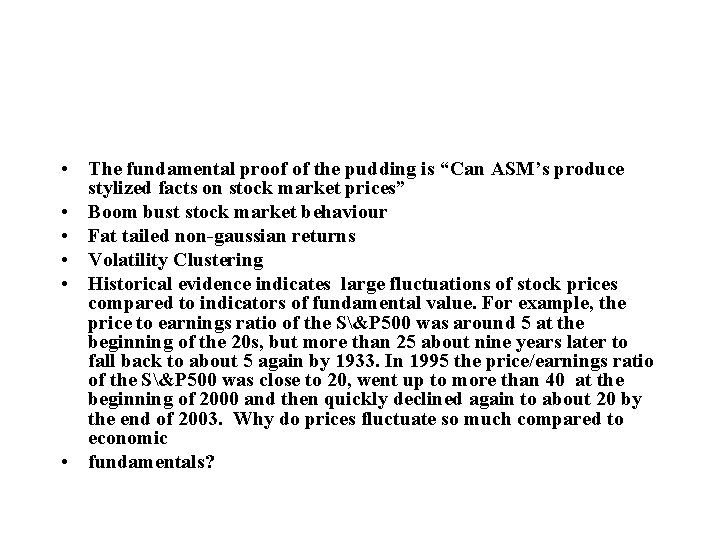
• The fundamental proof of the pudding is “Can ASM’s produce stylized facts on stock market prices” • Boom bust stock market behaviour • Fat tailed non-gaussian returns • Volatility Clustering • Historical evidence indicates large fluctuations of stock prices compared to indicators of fundamental value. For example, the price to earnings ratio of the S&P 500 was around 5 at the beginning of the 20 s, but more than 25 about nine years later to fall back to about 5 again by 1933. In 1995 the price/earnings ratio of the S&P 500 was close to 20, went up to more than 40 at the beginning of 2000 and then quickly declined again to about 20 by the end of 2003. Why do prices fluctuate so much compared to economic • fundamentals?
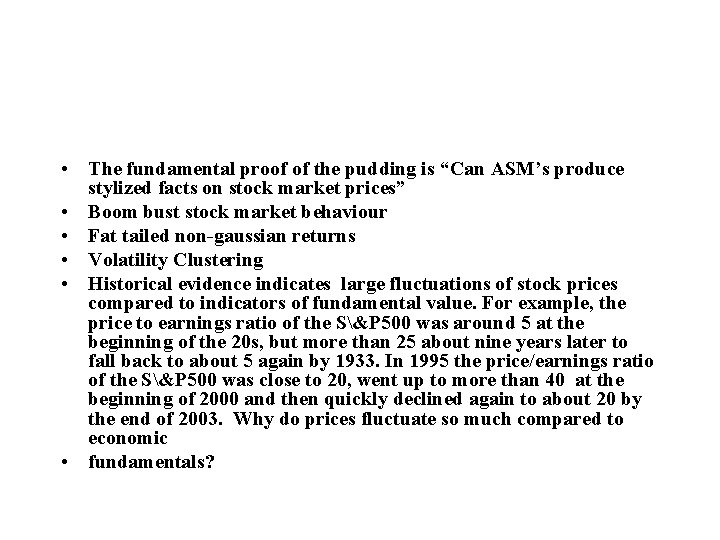
• The fundamental proof of the pudding is “Can ASM’s produce stylized facts on stock market prices” • Boom bust stock market behaviour • Fat tailed non-gaussian returns • Volatility Clustering • Historical evidence indicates large fluctuations of stock prices compared to indicators of fundamental value. For example, the price to earnings ratio of the S&P 500 was around 5 at the beginning of the 20 s, but more than 25 about nine years later to fall back to about 5 again by 1933. In 1995 the price/earnings ratio of the S&P 500 was close to 20, went up to more than 40 at the beginning of 2000 and then quickly declined again to about 20 by the end of 2003. Why do prices fluctuate so much compared to economic • fundamentals?
Old paradigm vs new paradigm examples
Gent urban dictionary
Multiagent learning using a variable learning rate
Poslopja na kmetiji
New paradigm leadership
New paradigm leadership
Diestel graph theory
What is the difference between modals and semi modals
Homogeneous mixture and heterogeneous mixture class 9
Homogeneous and heterogeneous distributed database
7 habits paradigms
Syntagmatic analysis