Computational Characterization of DrugInduced Adverse Events with A
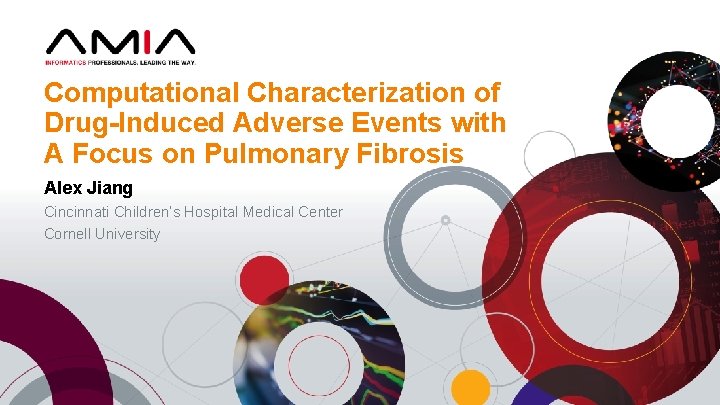
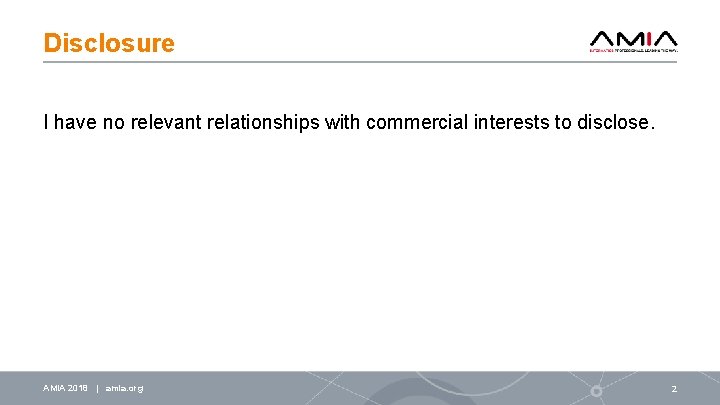
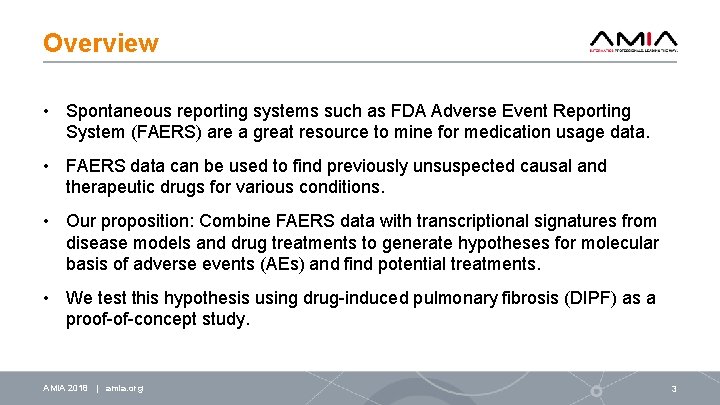
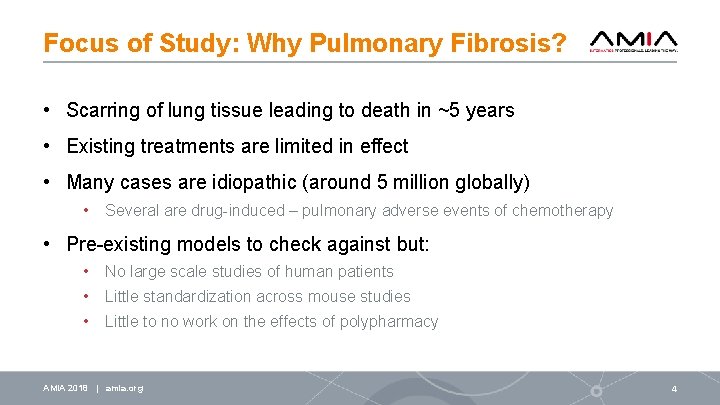
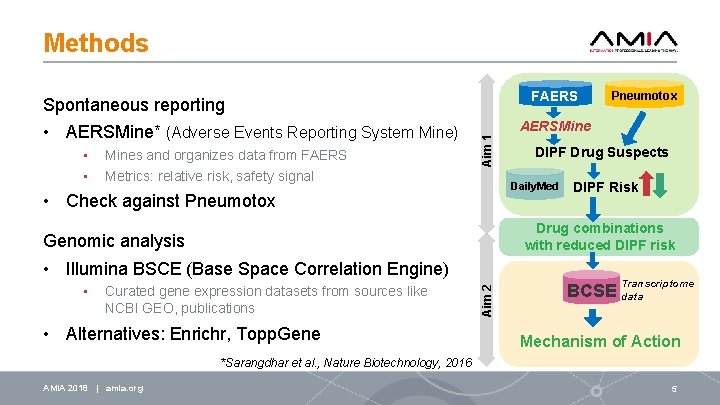
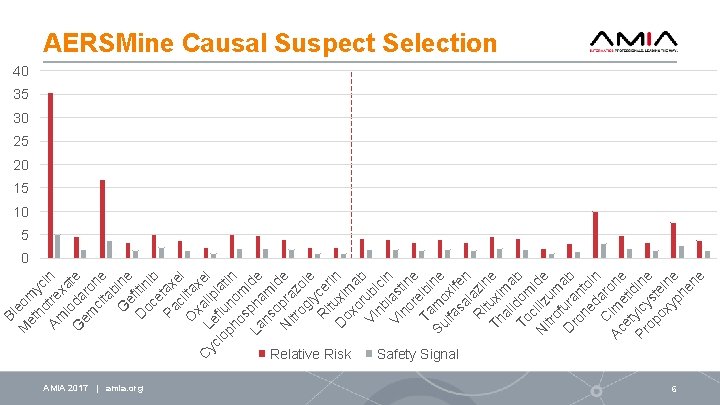
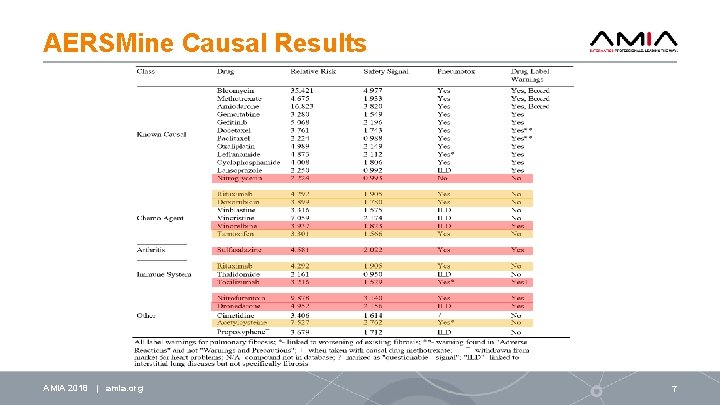
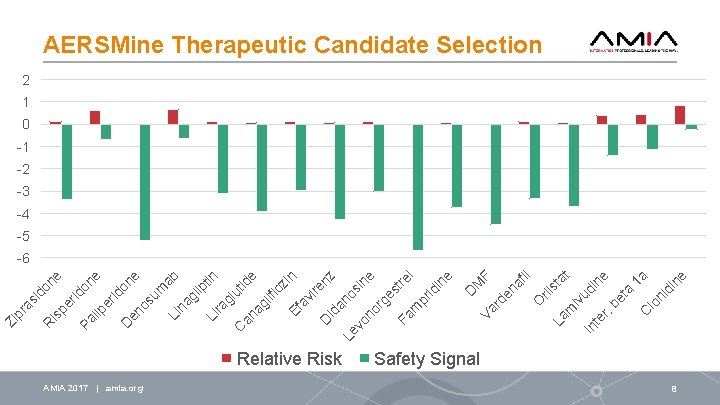
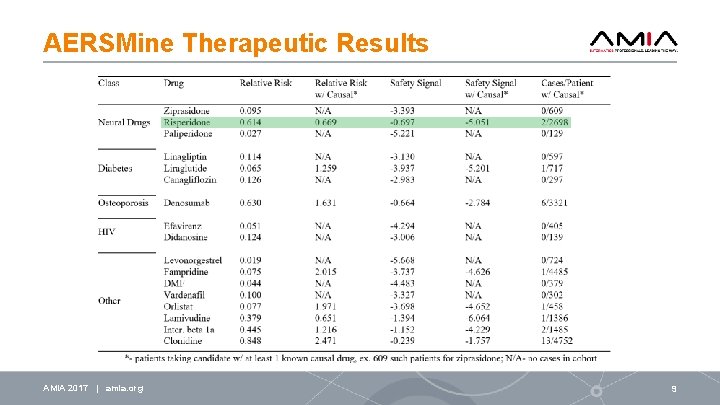
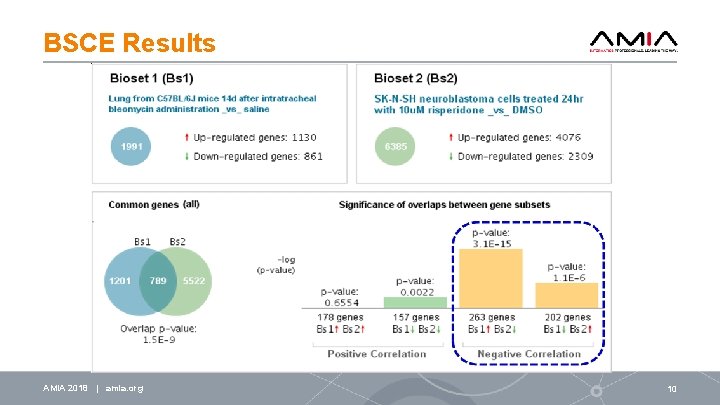
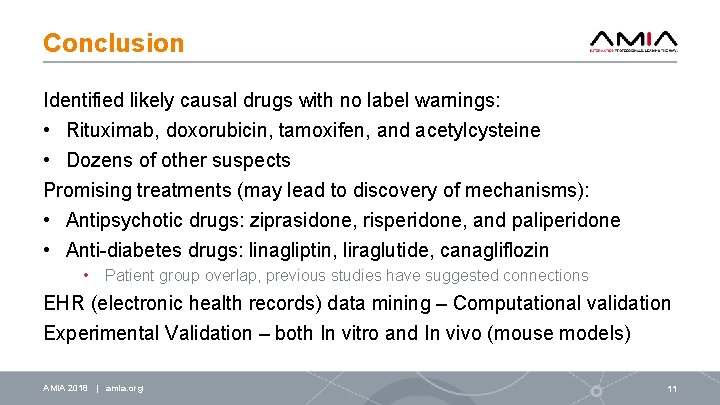
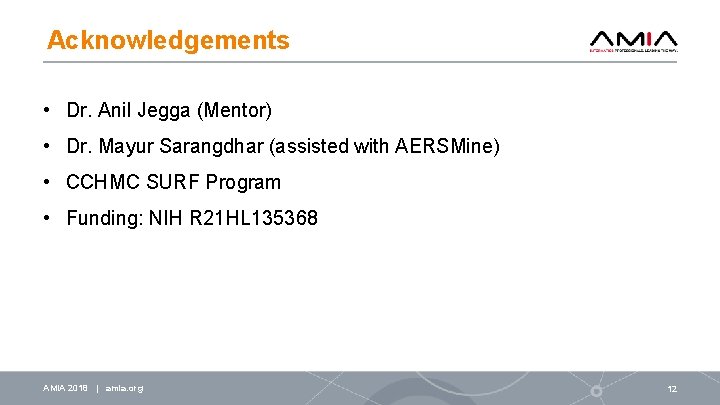
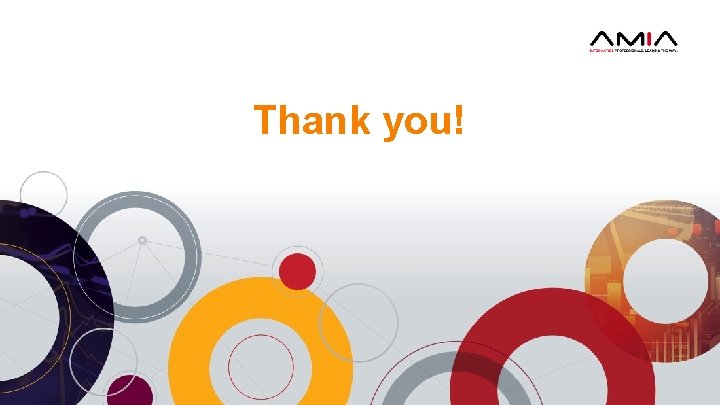
- Slides: 13
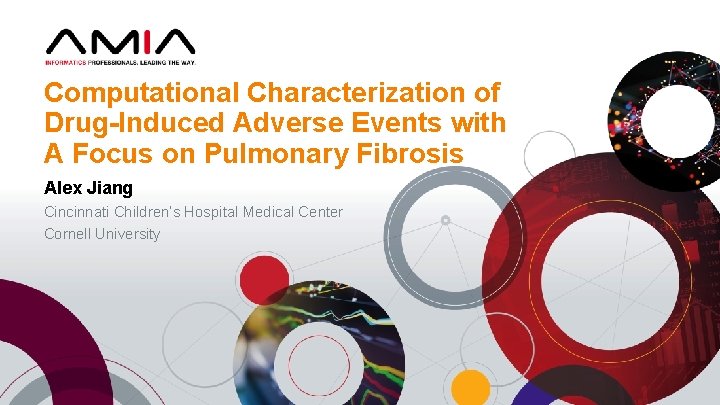
Computational Characterization of Drug-Induced Adverse Events with A Focus on Pulmonary Fibrosis Alex Jiang Cincinnati Children’s Hospital Medical Center Cornell University
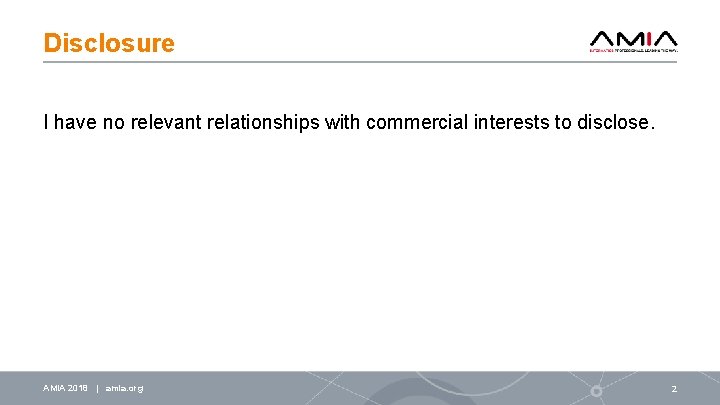
Disclosure I have no relevant relationships with commercial interests to disclose. AMIA 2018 | amia. org 2
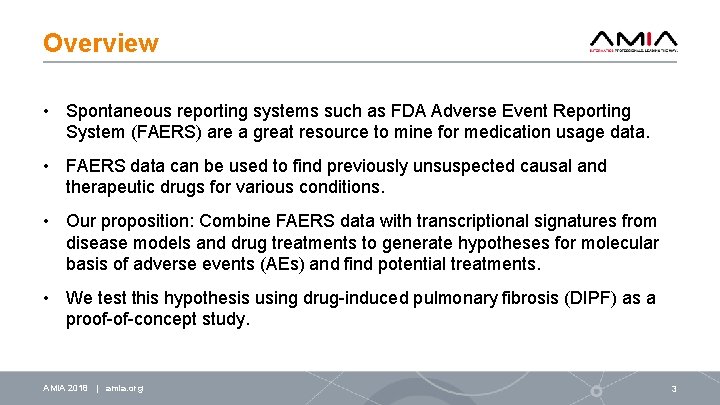
Overview • Spontaneous reporting systems such as FDA Adverse Event Reporting System (FAERS) are a great resource to mine for medication usage data. • FAERS data can be used to find previously unsuspected causal and therapeutic drugs for various conditions. • Our proposition: Combine FAERS data with transcriptional signatures from disease models and drug treatments to generate hypotheses for molecular basis of adverse events (AEs) and find potential treatments. • We test this hypothesis using drug-induced pulmonary fibrosis (DIPF) as a proof-of-concept study. AMIA 2018 | amia. org 3
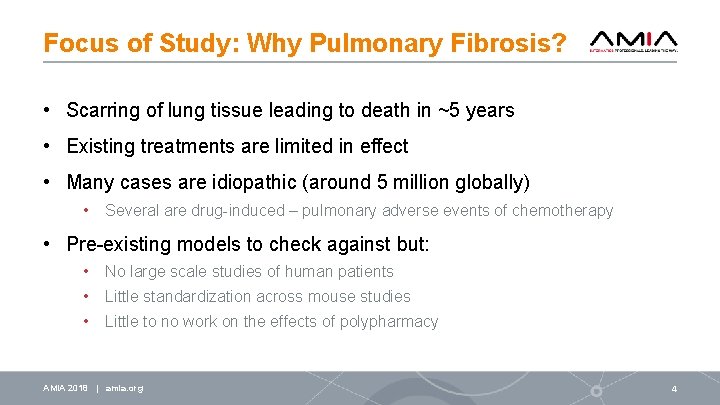
Focus of Study: Why Pulmonary Fibrosis? • Scarring of lung tissue leading to death in ~5 years • Existing treatments are limited in effect • Many cases are idiopathic (around 5 million globally) • Several are drug-induced – pulmonary adverse events of chemotherapy • Pre-existing models to check against but: • No large scale studies of human patients • Little standardization across mouse studies • Little to no work on the effects of polypharmacy AMIA 2018 | amia. org 4
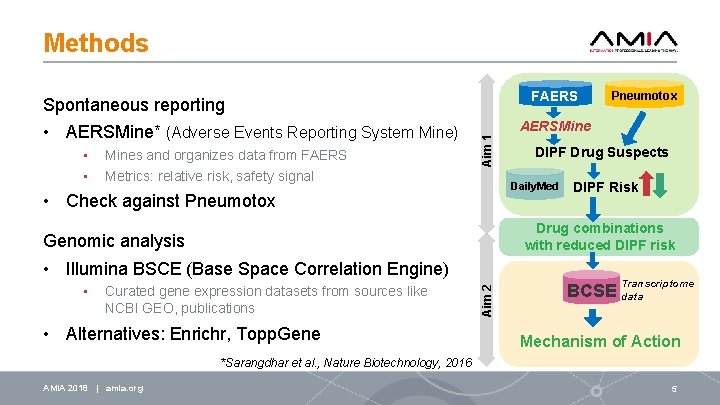
Methods FAERS Spontaneous reporting • • Mines and organizes data from FAERS Metrics: relative risk, safety signal AERSMine Aim 1 • AERSMine* (Adverse Events Reporting System Mine) DIPF Drug Suspects Daily. Med • Check against Pneumotox DIPF Risk Drug combinations with reduced DIPF risk Genomic analysis Curated gene expression datasets from sources like NCBI GEO, publications • Alternatives: Enrichr, Topp. Gene Aim 2 • Illumina BSCE (Base Space Correlation Engine) • Pneumotox Transcriptome BCSE data Mechanism of Action *Sarangdhar et al. , Nature Biotechnology, 2016 AMIA 2018 | amia. org 5
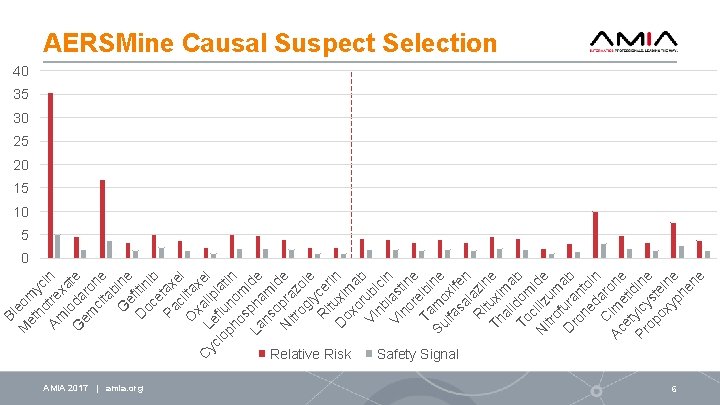
Bl M eom et ho yc Am tre in io xat G da e em ro ci ne ta b G ine e D fitin oc ib e Pa tax c el O lita xa xe C L yc e lipl l lo flu at ph no in os m La pha ide ns m o id N pra e itr og zol ly e R ce it ri D uxi n ox m o ab Vi rub nb ici n Vi las no tin r e Ta elbi Su mo ne lfa xi sa fen l R azin i Th tuxi e al ma i To dom b c N iliz ide itr u of m D ura ab ro n ne to d in C aro Ac im ne et eti Pr ylcy dine op st ox ein yp e he ne AERSMine Causal Suspect Selection 40 35 30 25 20 15 10 5 0 Relative Risk AMIA 2017 | amia. org Safety Signal 6
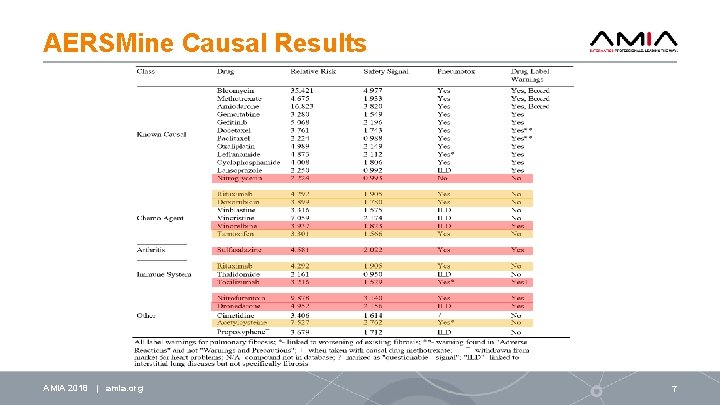
AERSMine Causal Results AMIA 2018 | amia. org 7
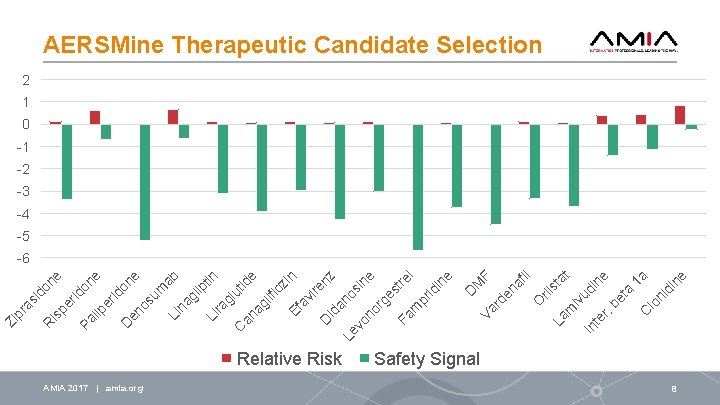
er id is p ne Relative Risk AMIA 2017 | amia. org C te r. In ne ne 1 a id i lo n ta be fil st at rli iv ud i m La O F M en a Va rd D on Pa e lip er id on D en e os um ab Li na gl ip tin Li ra gl ut C id an e ag lif lo zi n Ef av ire D nz id an Le os vo in e no rg es tre Fa l m pr id in e R as id o Zi pr AERSMine Therapeutic Candidate Selection 2 1 0 -1 -2 -3 -4 -5 -6 Safety Signal 8
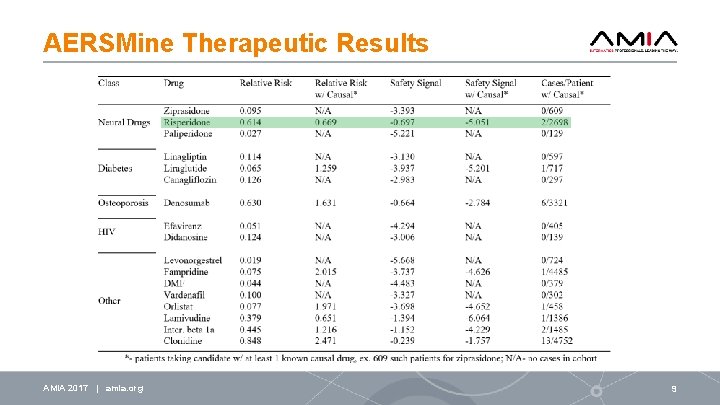
AERSMine Therapeutic Results AMIA 2017 | amia. org 9
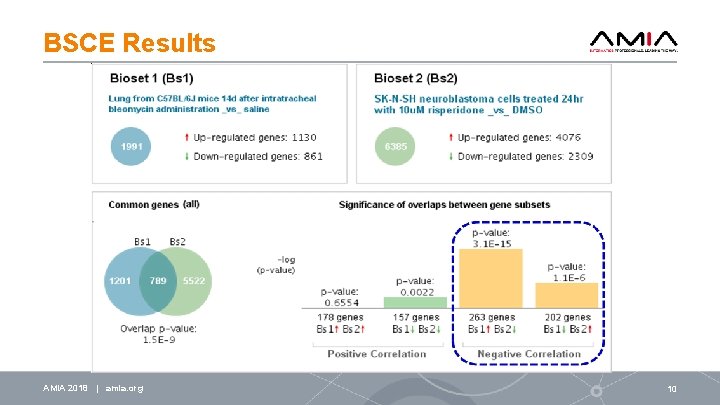
BSCE Results AMIA 2018 | amia. org 10
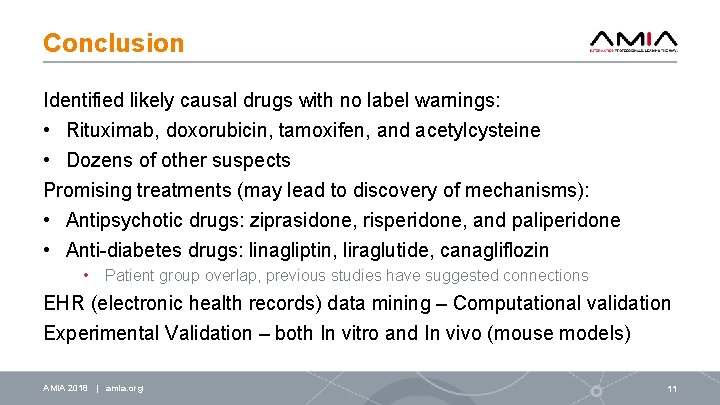
Conclusion Identified likely causal drugs with no label warnings: • Rituximab, doxorubicin, tamoxifen, and acetylcysteine • Dozens of other suspects Promising treatments (may lead to discovery of mechanisms): • Antipsychotic drugs: ziprasidone, risperidone, and paliperidone • Anti-diabetes drugs: linagliptin, liraglutide, canagliflozin • Patient group overlap, previous studies have suggested connections EHR (electronic health records) data mining – Computational validation Experimental Validation – both In vitro and In vivo (mouse models) AMIA 2018 | amia. org 11
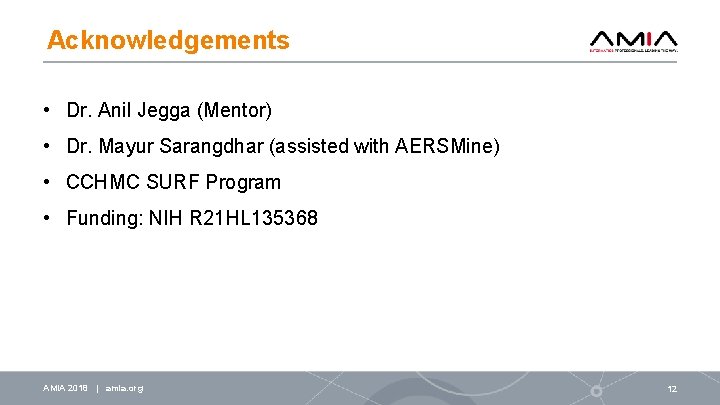
Acknowledgements • Dr. Anil Jegga (Mentor) • Dr. Mayur Sarangdhar (assisted with AERSMine) • CCHMC SURF Program • Funding: NIH R 21 HL 135368 AMIA 2018 | amia. org 12
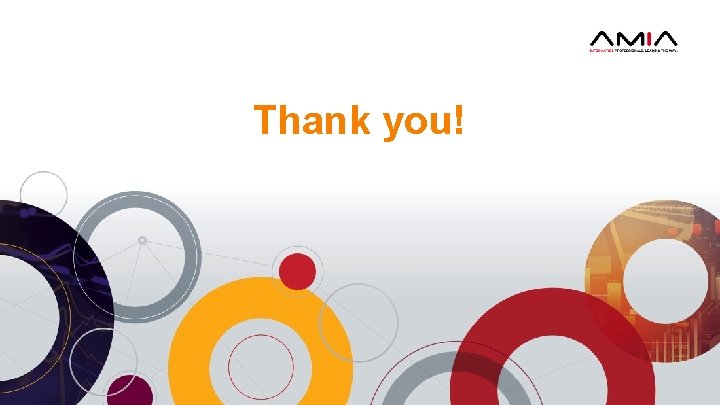
Thank you!
Adverse events in hospital
Adverse events in hospital
Aefi examples
Adverse events in hospital
Direct indirect characterization
What is direct and indirect characterization
Mutually exclusive vs non mutually exclusive
Adverse yaw
Adverse treatment
Analgesic mechanism
Ahca adverse incident reporting
Definition of adverse event
Adverse event adalah
Sentinal event