Chapter 15 part C Multicollinearity V E Multicollinearity
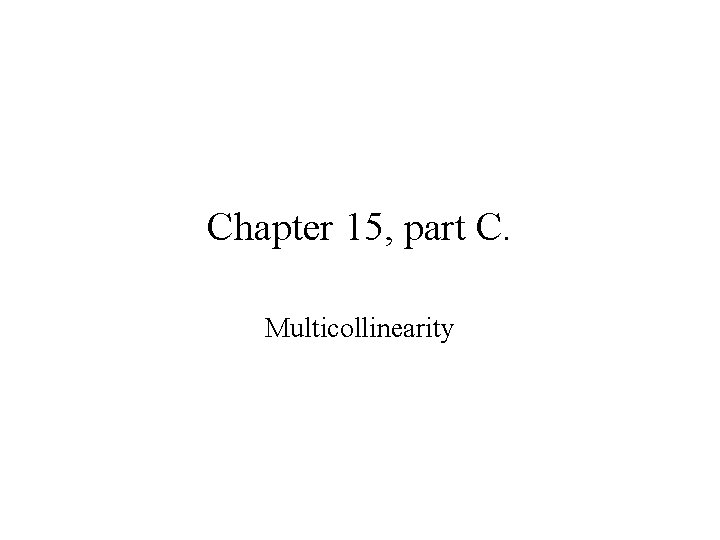
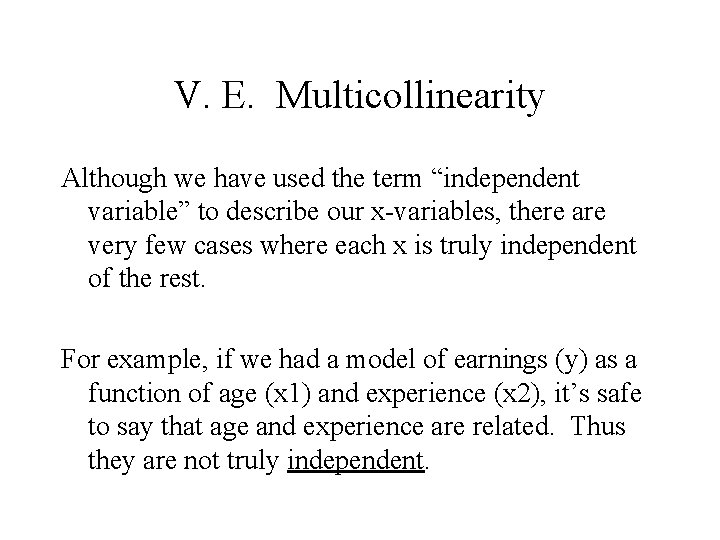
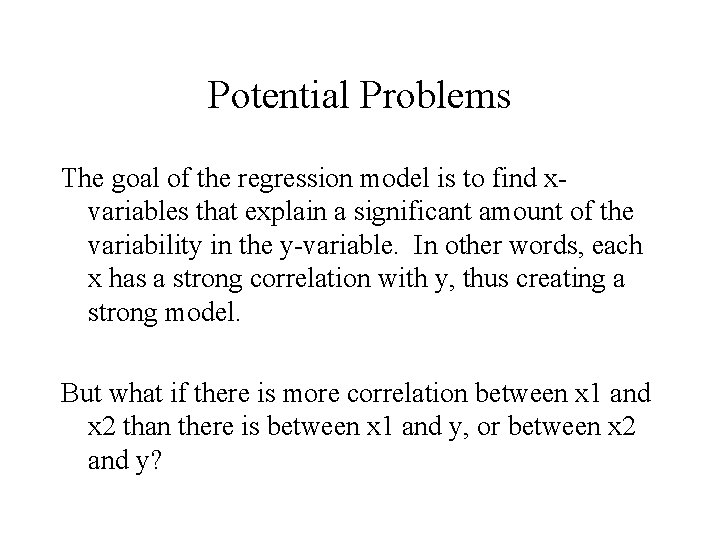
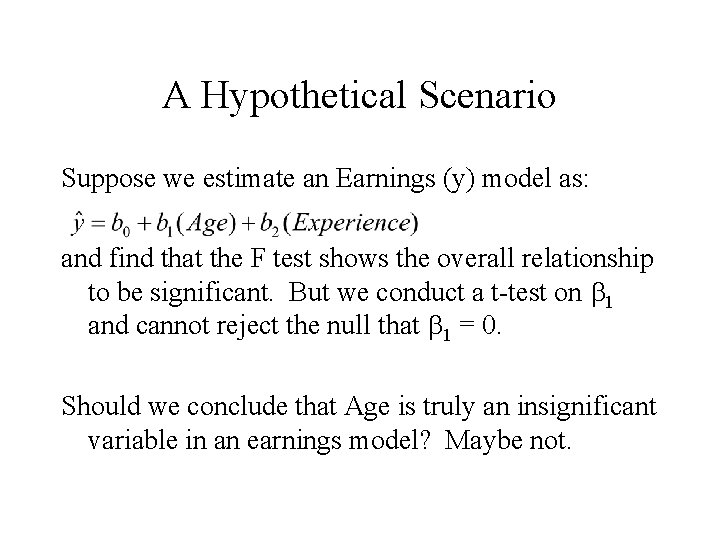
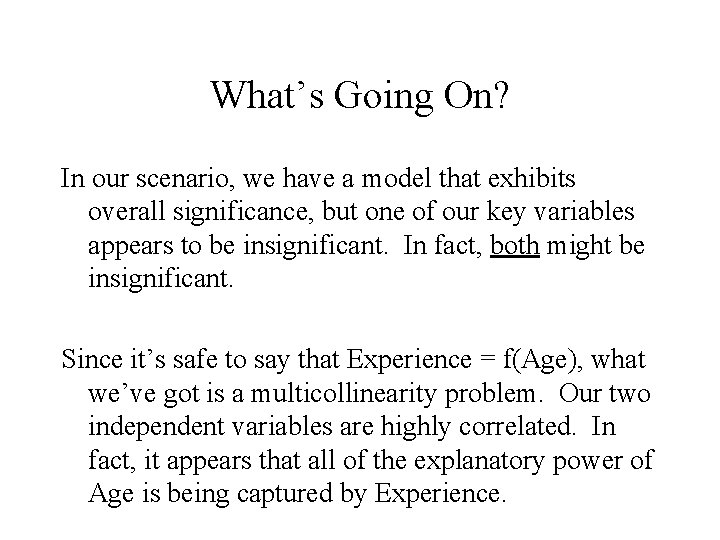
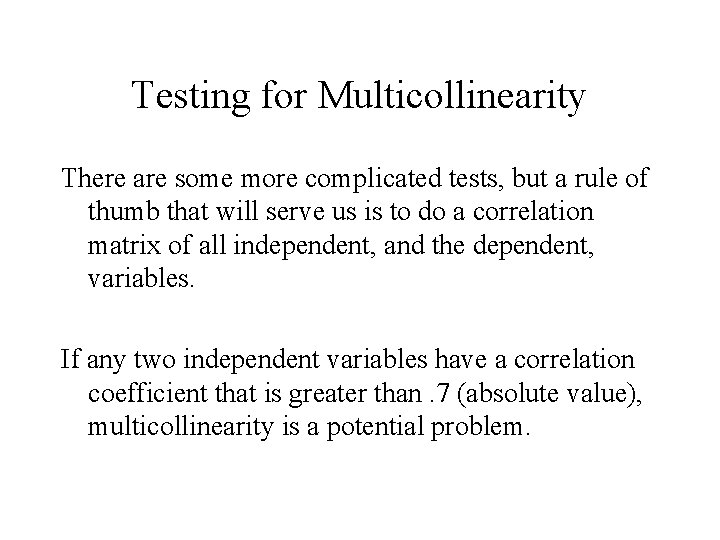
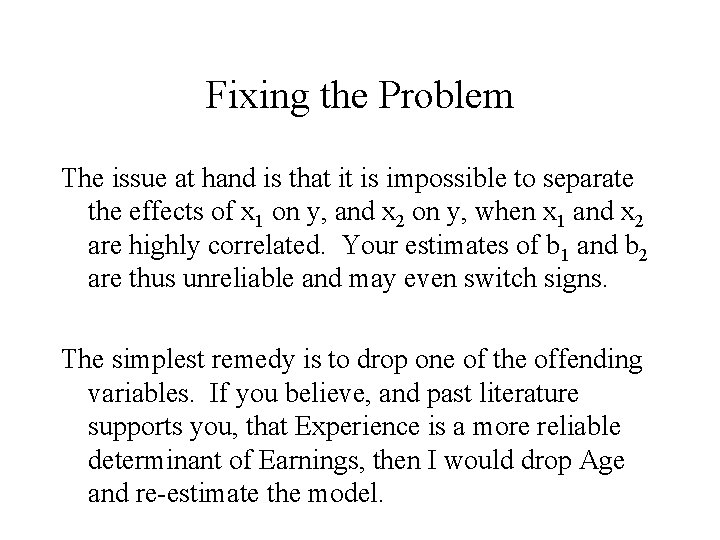
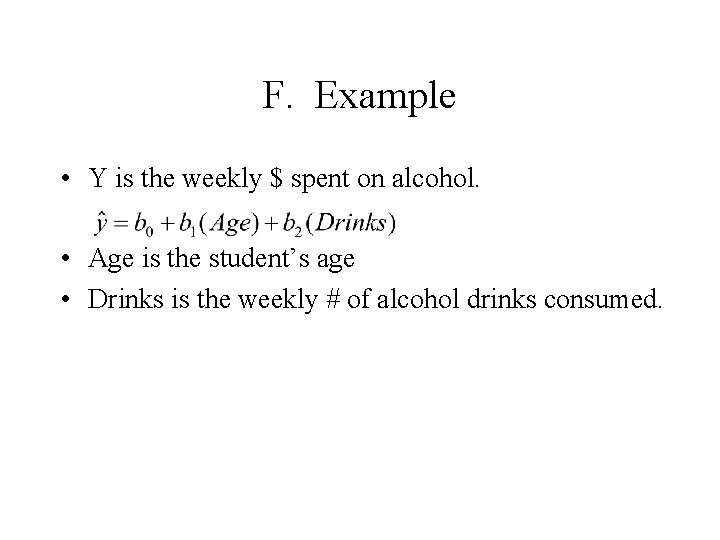
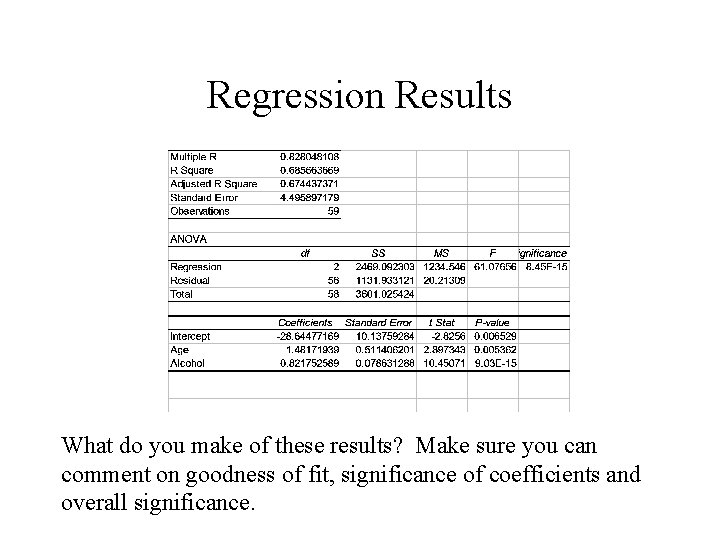
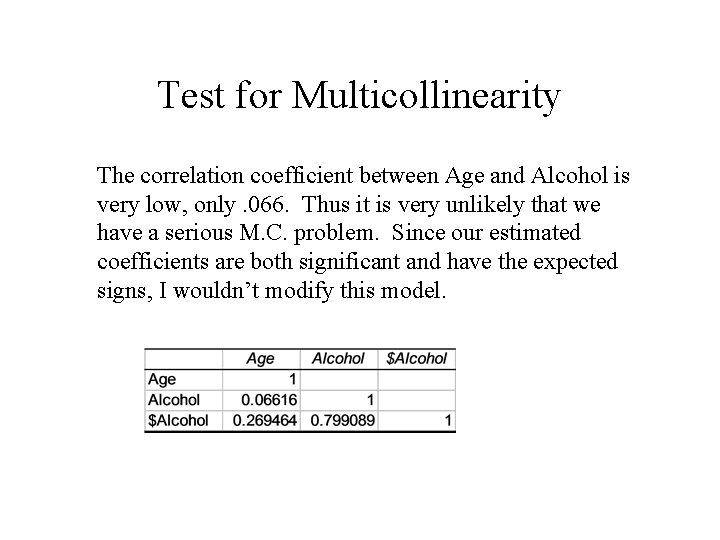
- Slides: 10
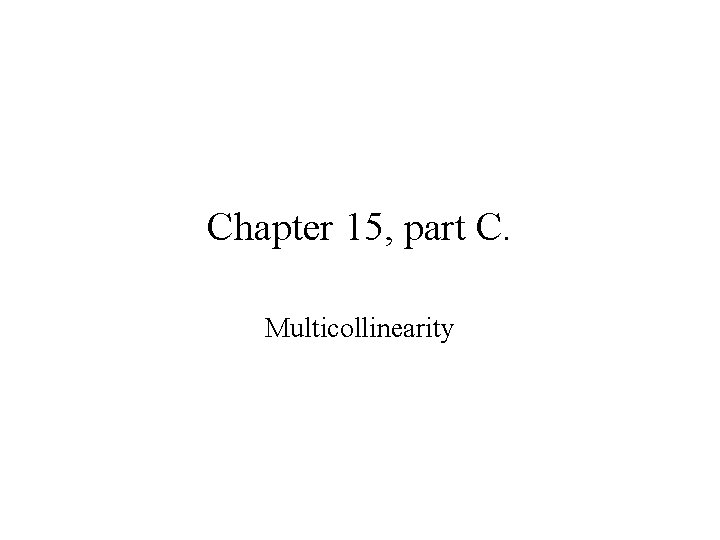
Chapter 15, part C. Multicollinearity
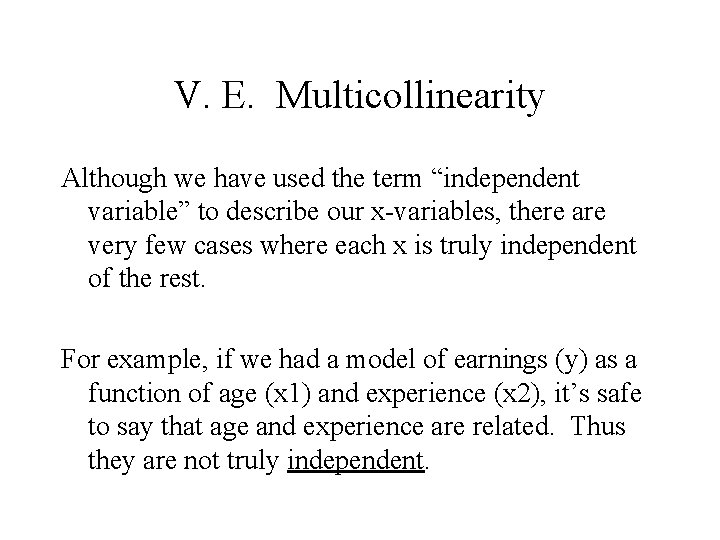
V. E. Multicollinearity Although we have used the term “independent variable” to describe our x-variables, there are very few cases where each x is truly independent of the rest. For example, if we had a model of earnings (y) as a function of age (x 1) and experience (x 2), it’s safe to say that age and experience are related. Thus they are not truly independent.
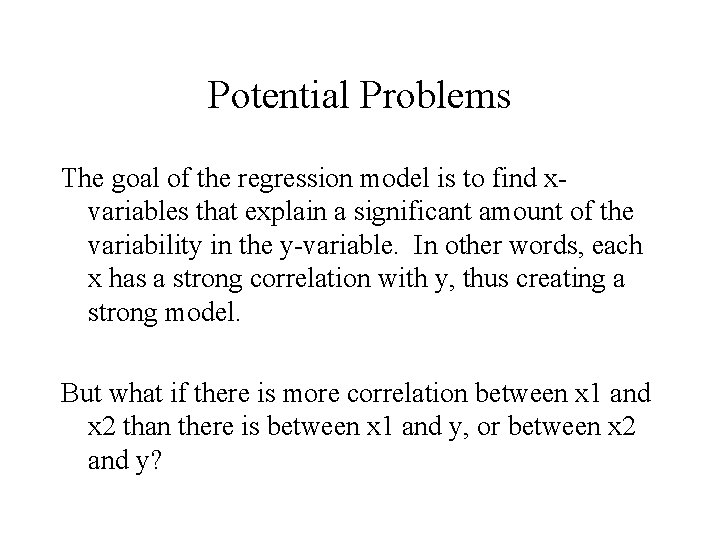
Potential Problems The goal of the regression model is to find xvariables that explain a significant amount of the variability in the y-variable. In other words, each x has a strong correlation with y, thus creating a strong model. But what if there is more correlation between x 1 and x 2 than there is between x 1 and y, or between x 2 and y?
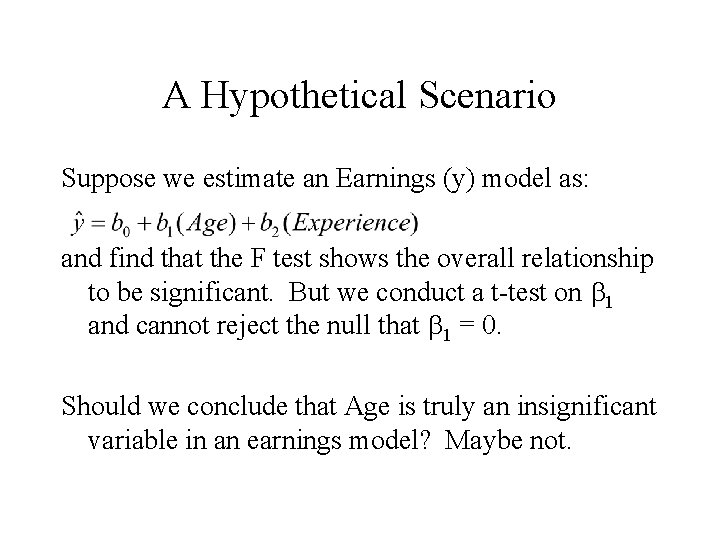
A Hypothetical Scenario Suppose we estimate an Earnings (y) model as: and find that the F test shows the overall relationship to be significant. But we conduct a t-test on 1 and cannot reject the null that 1 = 0. Should we conclude that Age is truly an insignificant variable in an earnings model? Maybe not.
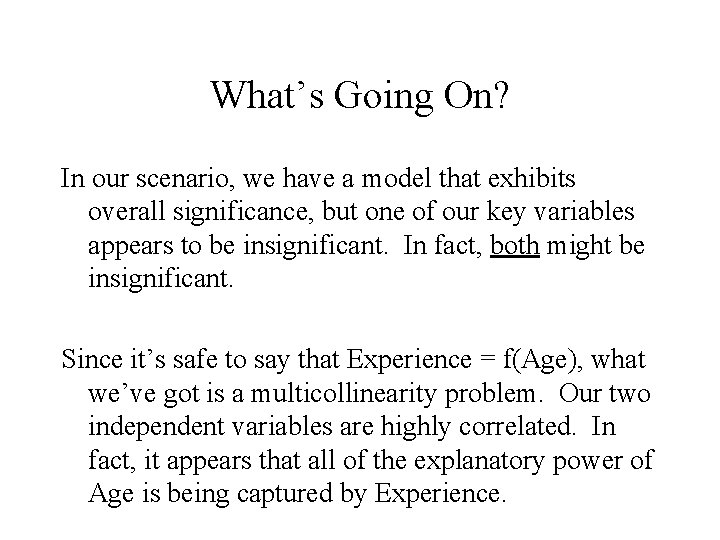
What’s Going On? In our scenario, we have a model that exhibits overall significance, but one of our key variables appears to be insignificant. In fact, both might be insignificant. Since it’s safe to say that Experience = f(Age), what we’ve got is a multicollinearity problem. Our two independent variables are highly correlated. In fact, it appears that all of the explanatory power of Age is being captured by Experience.
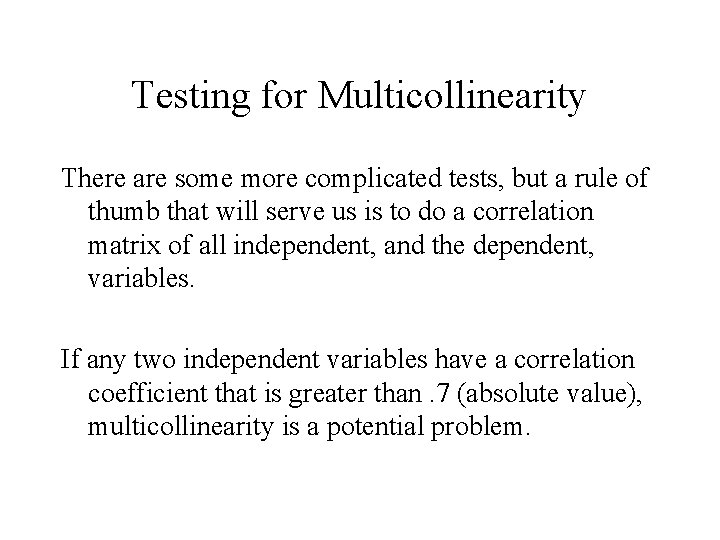
Testing for Multicollinearity There are some more complicated tests, but a rule of thumb that will serve us is to do a correlation matrix of all independent, and the dependent, variables. If any two independent variables have a correlation coefficient that is greater than. 7 (absolute value), multicollinearity is a potential problem.
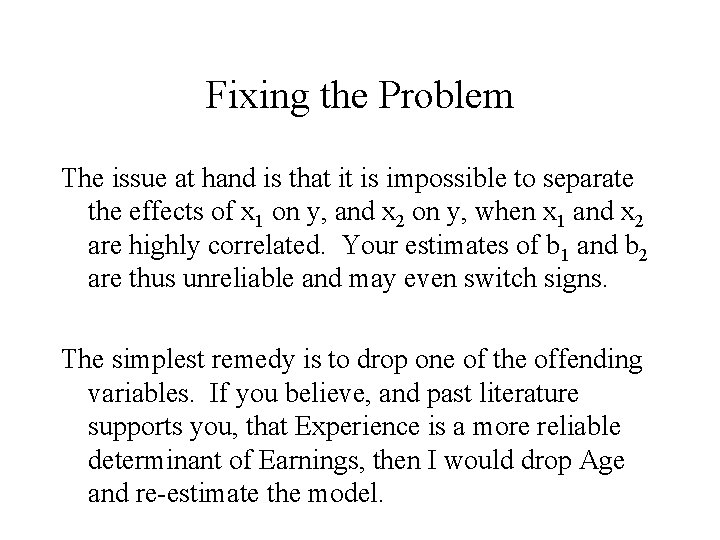
Fixing the Problem The issue at hand is that it is impossible to separate the effects of x 1 on y, and x 2 on y, when x 1 and x 2 are highly correlated. Your estimates of b 1 and b 2 are thus unreliable and may even switch signs. The simplest remedy is to drop one of the offending variables. If you believe, and past literature supports you, that Experience is a more reliable determinant of Earnings, then I would drop Age and re-estimate the model.
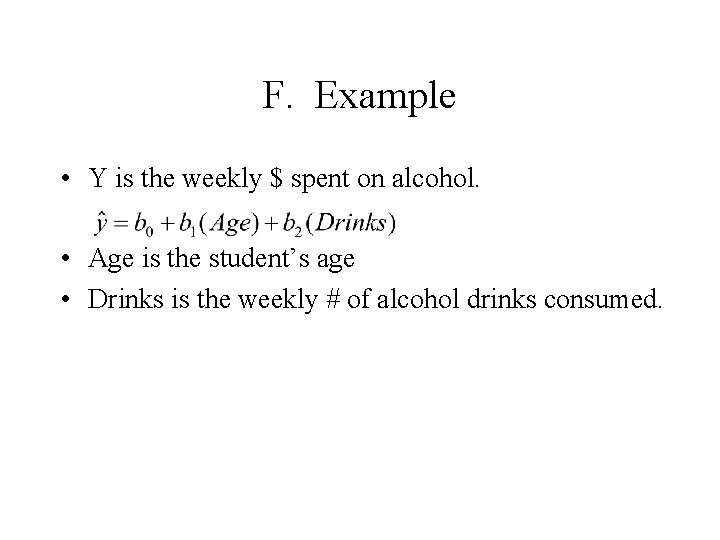
F. Example • Y is the weekly $ spent on alcohol. • Age is the student’s age • Drinks is the weekly # of alcohol drinks consumed.
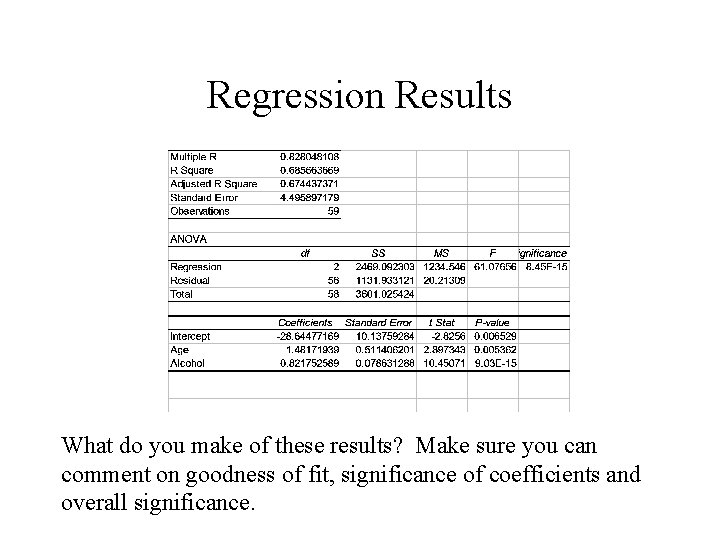
Regression Results What do you make of these results? Make sure you can comment on goodness of fit, significance of coefficients and overall significance.
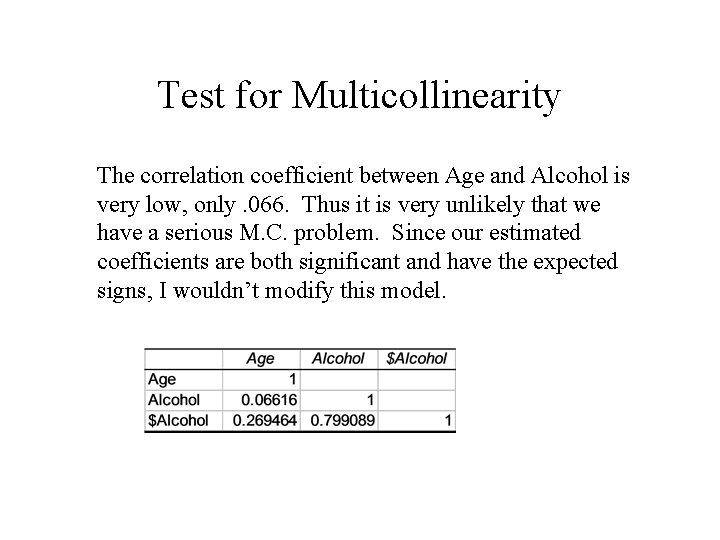
Test for Multicollinearity The correlation coefficient between Age and Alcohol is very low, only. 066. Thus it is very unlikely that we have a serious M. C. problem. Since our estimated coefficients are both significant and have the expected signs, I wouldn’t modify this model.