Ce NCOOS Regional DMAC Updates Patrick Daniel Information
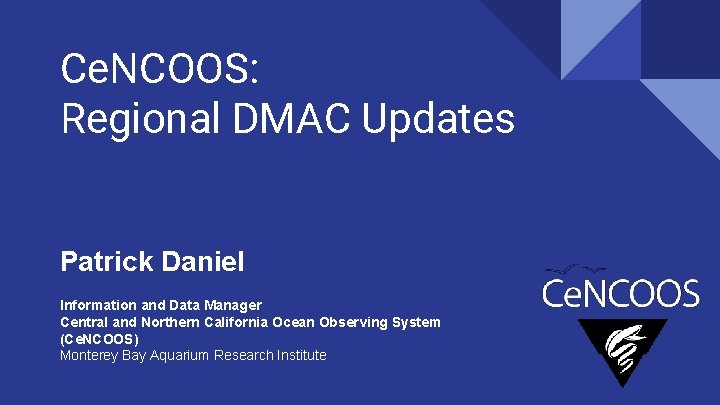
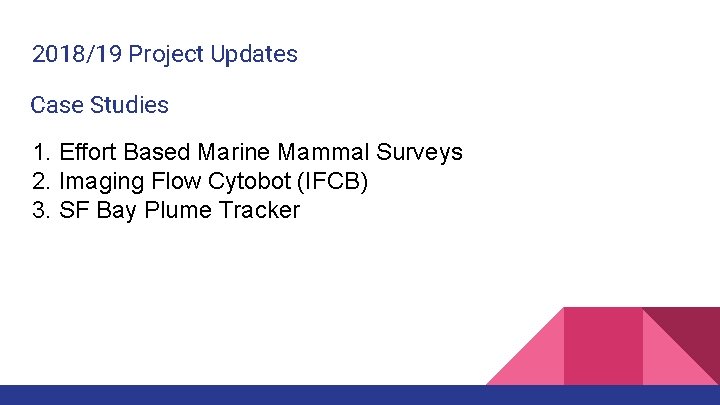
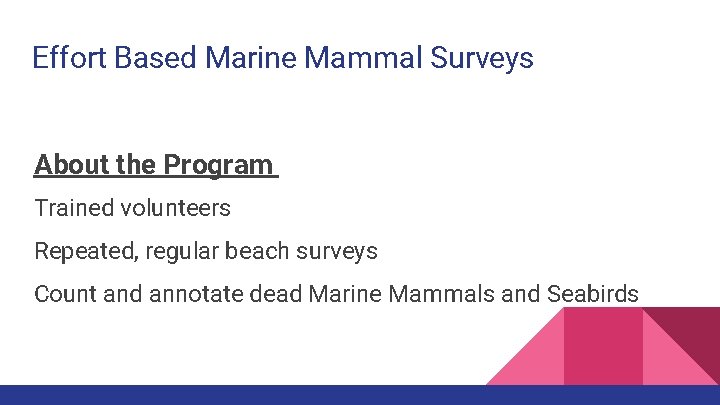
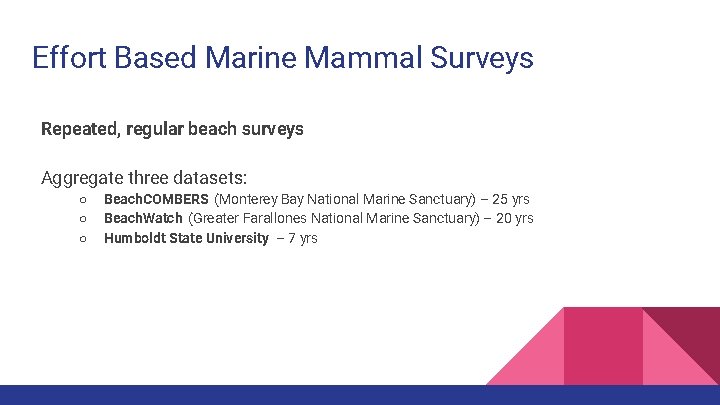
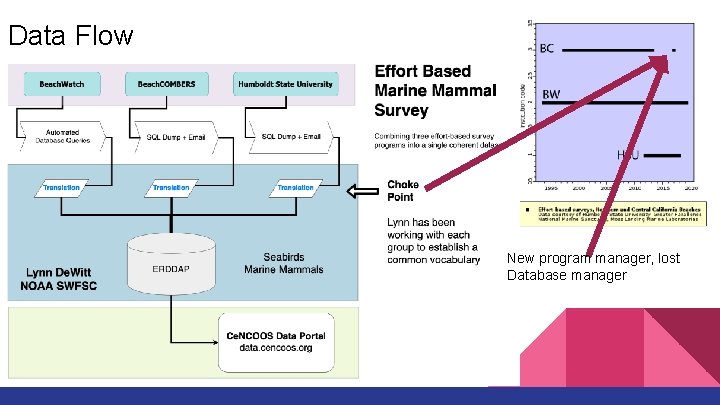
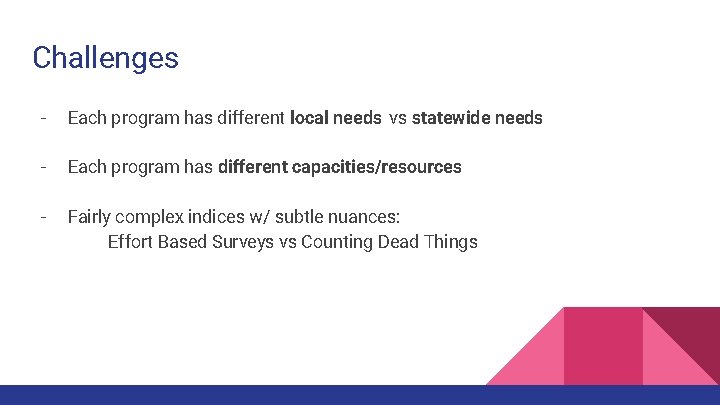
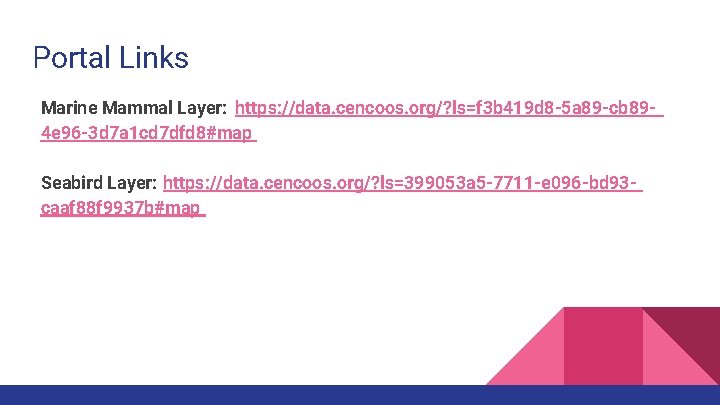
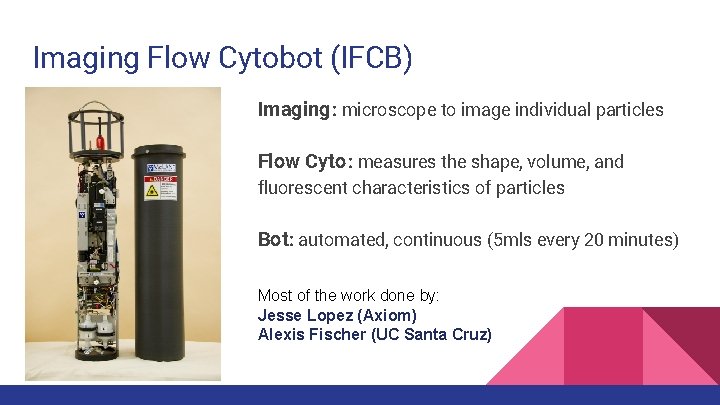
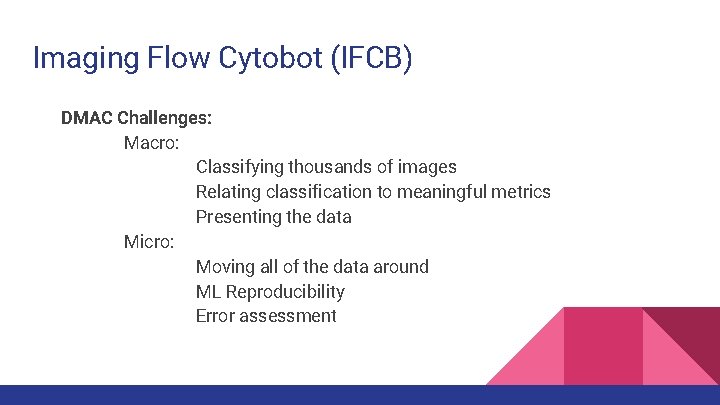
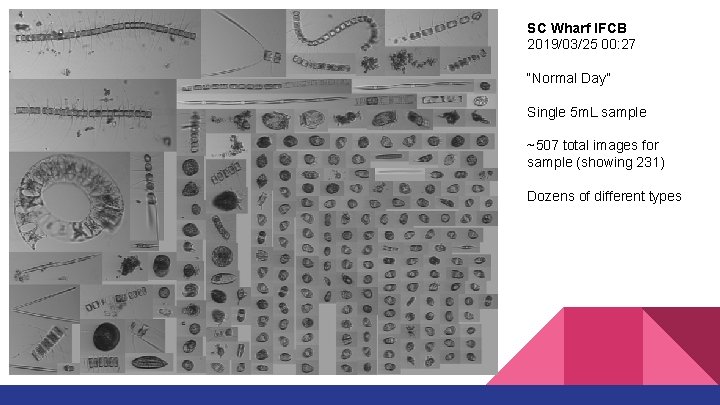
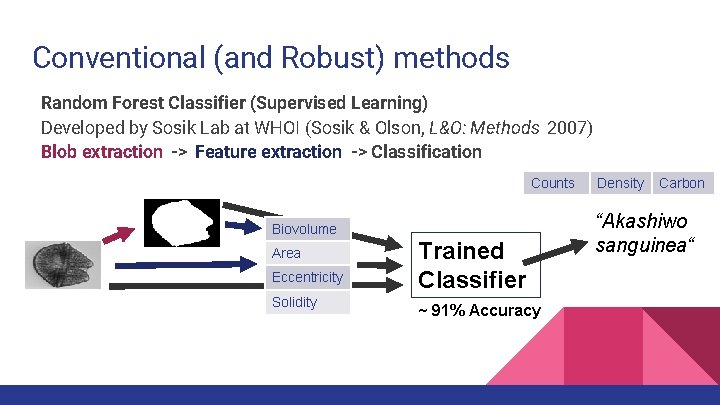
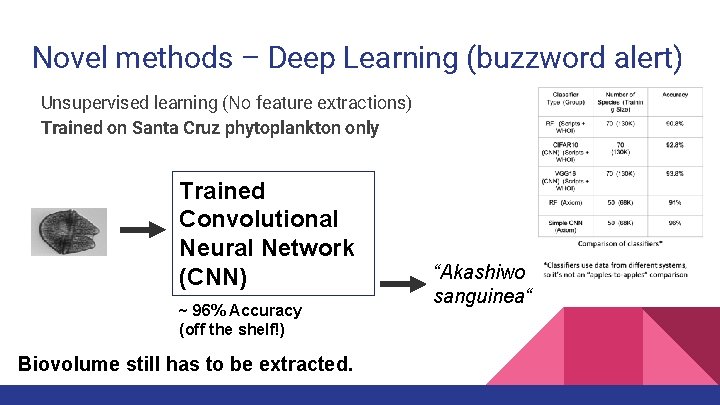
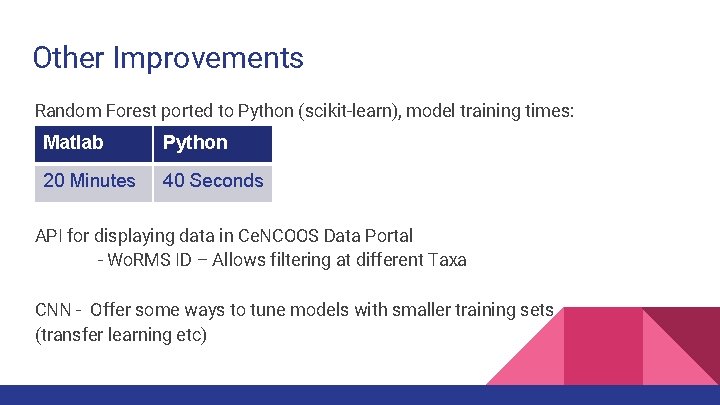
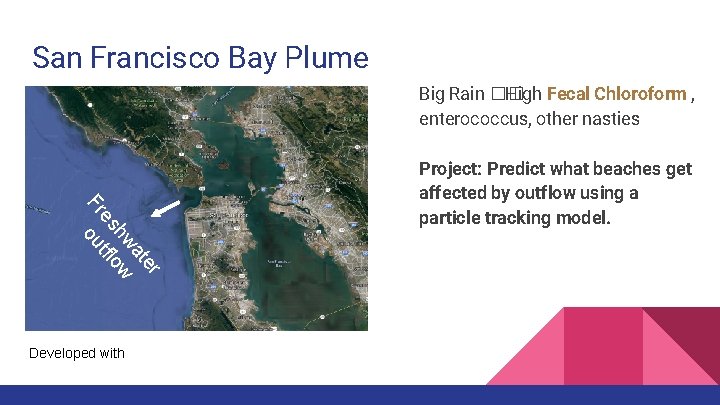
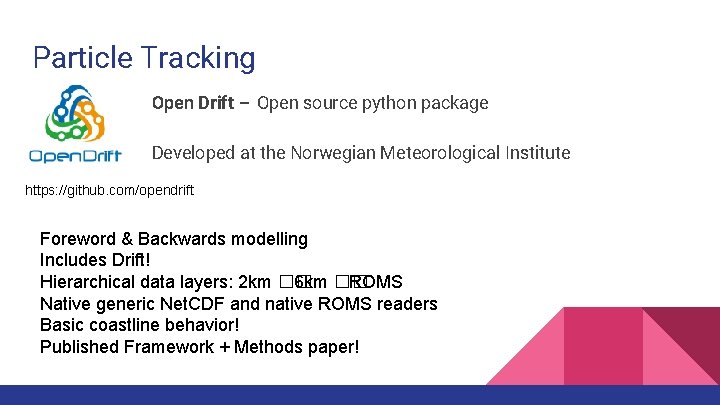
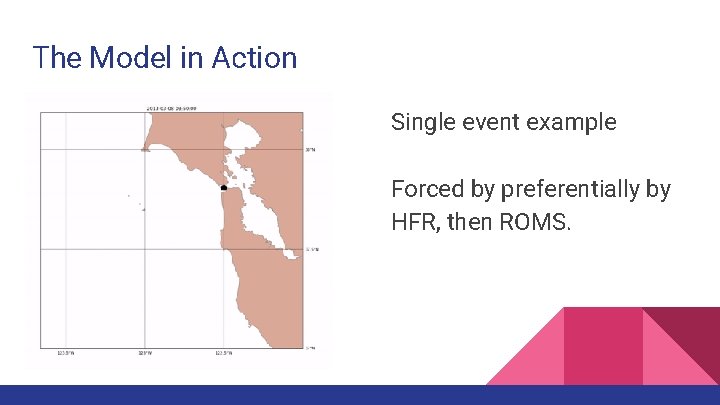
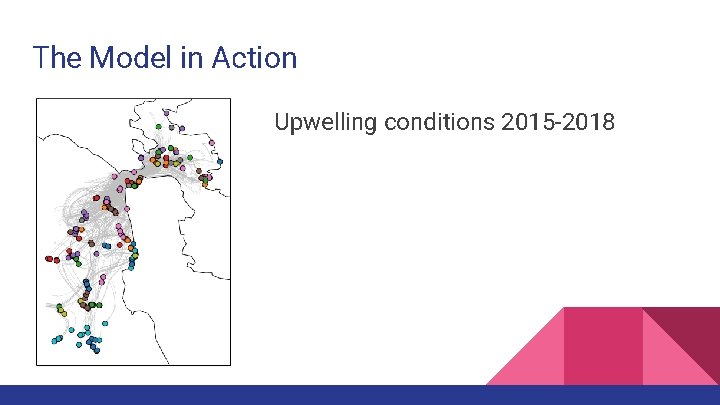
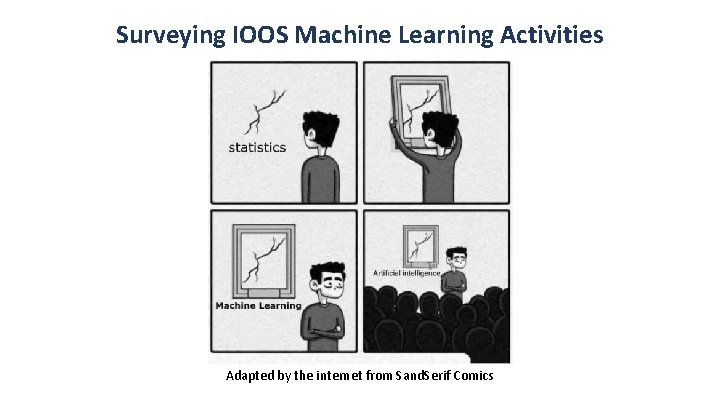
- Slides: 18
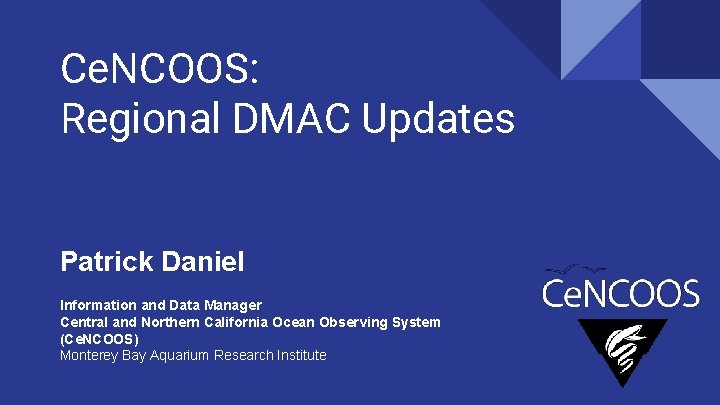
Ce. NCOOS: Regional DMAC Updates Patrick Daniel Information and Data Manager Central and Northern California Ocean Observing System (Ce. NCOOS) Monterey Bay Aquarium Research Institute
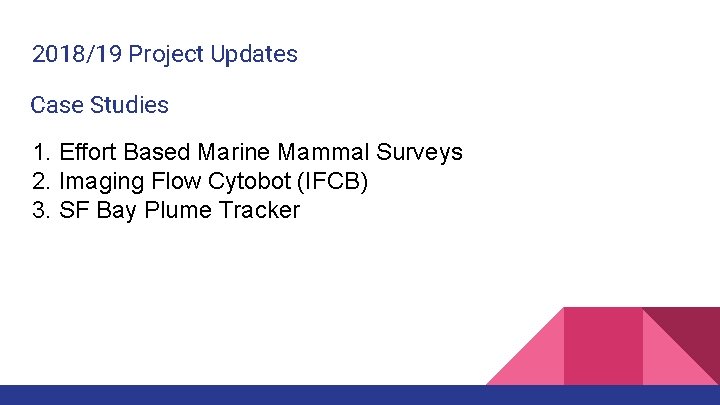
2018/19 Project Updates Case Studies 1. Effort Based Marine Mammal Surveys 2. Imaging Flow Cytobot (IFCB) 3. SF Bay Plume Tracker
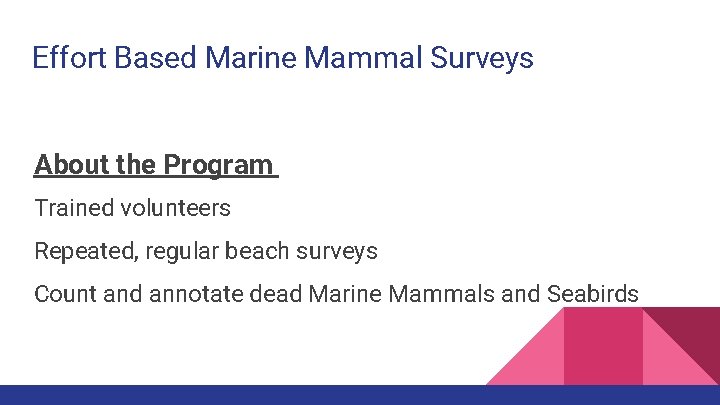
Effort Based Marine Mammal Surveys About the Program Trained volunteers Repeated, regular beach surveys Count and annotate dead Marine Mammals and Seabirds
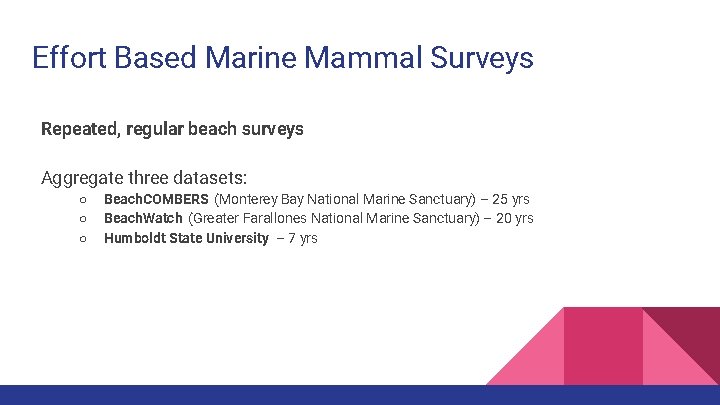
Effort Based Marine Mammal Surveys Repeated, regular beach surveys Aggregate three datasets: ○ ○ ○ Beach. COMBERS (Monterey Bay National Marine Sanctuary) – 25 yrs Beach. Watch (Greater Farallones National Marine Sanctuary) – 20 yrs Humboldt State University – 7 yrs
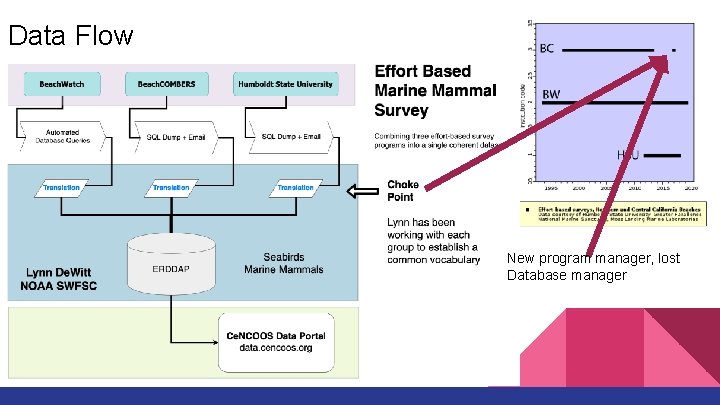
Data Flow New program manager, lost Database manager
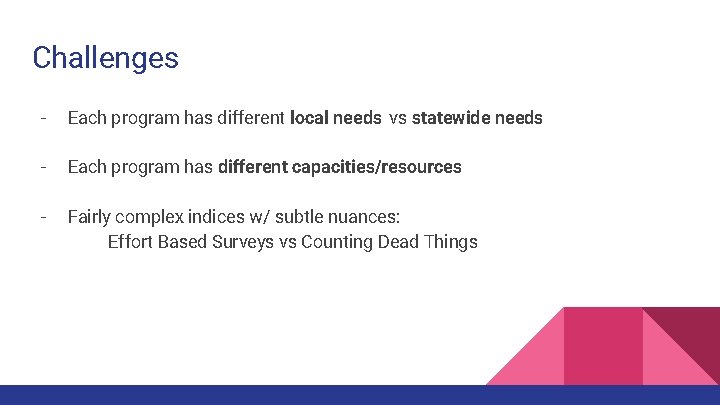
Challenges - Each program has different local needs vs statewide needs - Each program has different capacities/resources - Fairly complex indices w/ subtle nuances: Effort Based Surveys vs Counting Dead Things
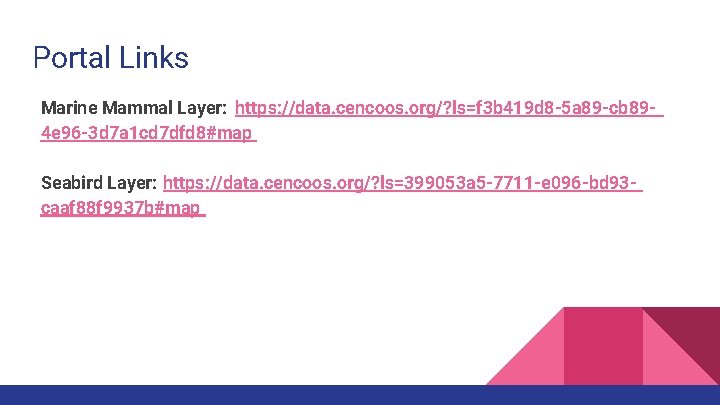
Portal Links Marine Mammal Layer: https: //data. cencoos. org/? ls=f 3 b 419 d 8 -5 a 89 -cb 894 e 96 -3 d 7 a 1 cd 7 dfd 8#map Seabird Layer: https: //data. cencoos. org/? ls=399053 a 5 -7711 -e 096 -bd 93 caaf 88 f 9937 b#map
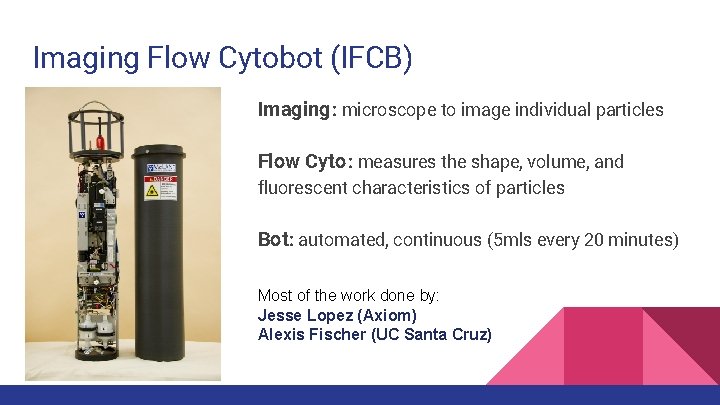
Imaging Flow Cytobot (IFCB) Imaging : microscope to image individual particles Flow Cyto : measures the shape, volume, and fluorescent characteristics of particles Bot : automated, continuous (5 mls every 20 minutes) Most of the work done by: Jesse Lopez (Axiom) Alexis Fischer (UC Santa Cruz)
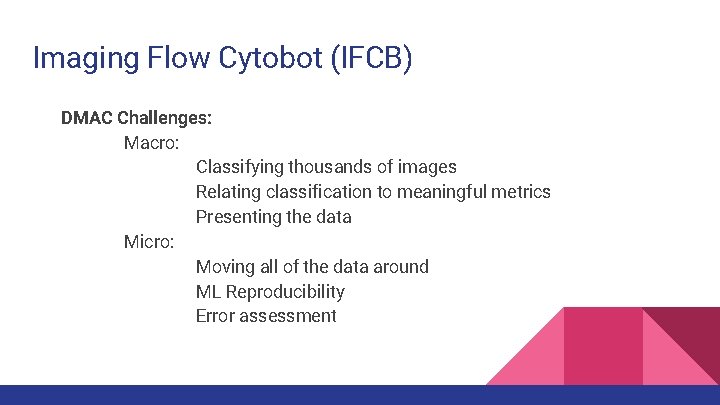
Imaging Flow Cytobot (IFCB) DMAC Challenges: Macro: Classifying thousands of images Relating classification to meaningful metrics Presenting the data Micro: Moving all of the data around ML Reproducibility Error assessment
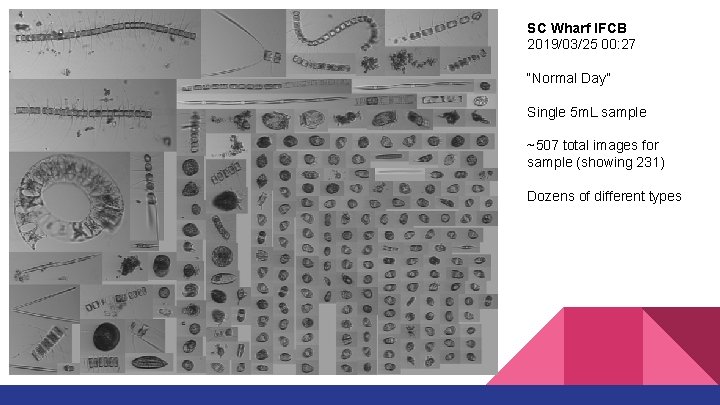
SC Wharf IFCB 2019/03/25 00: 27 “Normal Day” Single 5 m. L sample ~507 total images for sample (showing 231) Dozens of different types
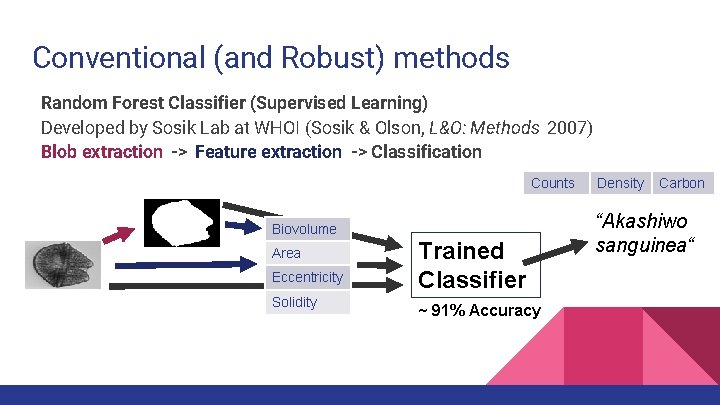
Conventional (and Robust) methods Random Forest Classifier (Supervised Learning) Developed by Sosik Lab at WHOI (Sosik & Olson, L&O: Methods 2007) Blob extraction -> Feature extraction -> Classification Counts Biovolume Area Eccentricity Solidity Trained Classifier ~ 91% Accuracy Density Carbon “Akashiwo sanguinea“
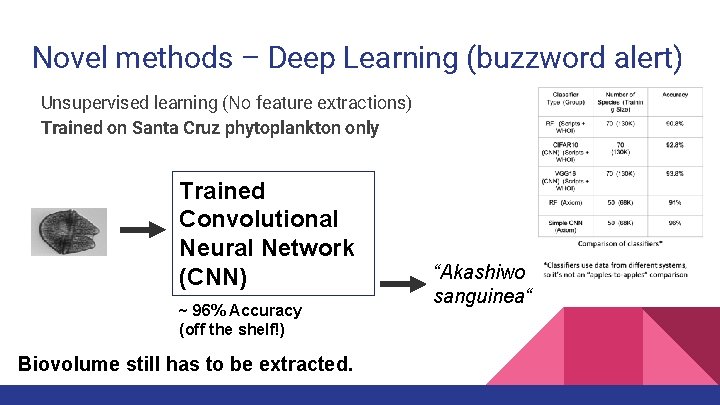
Novel methods – Deep Learning (buzzword alert) Unsupervised learning (No feature extractions) Trained on Santa Cruz phytoplankton only Trained Convolutional Neural Network (CNN) ~ 96% Accuracy (off the shelf!) Biovolume still has to be extracted. “Akashiwo sanguinea“
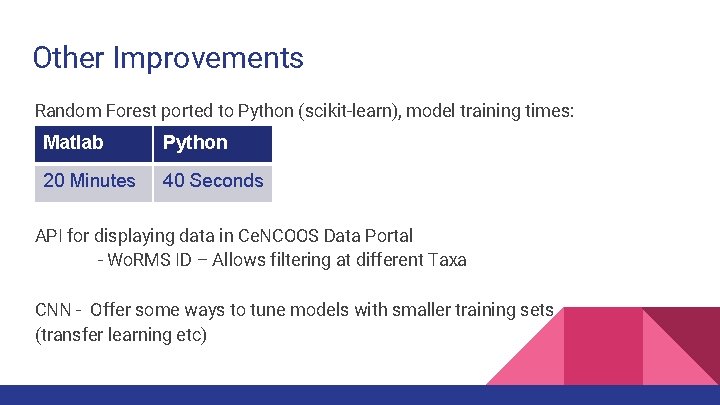
Other Improvements Random Forest ported to Python (scikit-learn), model training times: Matlab Python 20 Minutes 40 Seconds API for displaying data in Ce. NCOOS Data Portal - Wo. RMS ID – Allows filtering at different Taxa CNN - Offer some ways to tune models with smaller training sets (transfer learning etc)
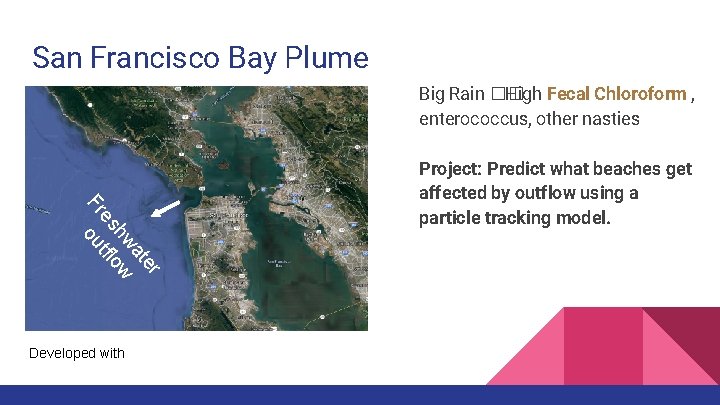
San Francisco Bay Plume Big Rain �� High Fecal Chloroform , enterococcus, other nasties er at hw w es tflo Fr ou Developed with Project: Predict what beaches get affected by outflow using a particle tracking model.
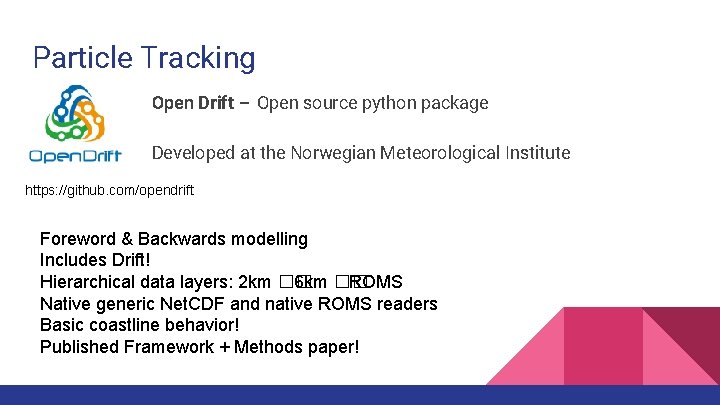
Particle Tracking Open Drift – Open source python package Developed at the Norwegian Meteorological Institute https: //github. com/opendrift Foreword & Backwards modelling Includes Drift! Hierarchical data layers: 2 km �� 6 km �� ROMS Native generic Net. CDF and native ROMS readers Basic coastline behavior! Published Framework + Methods paper!
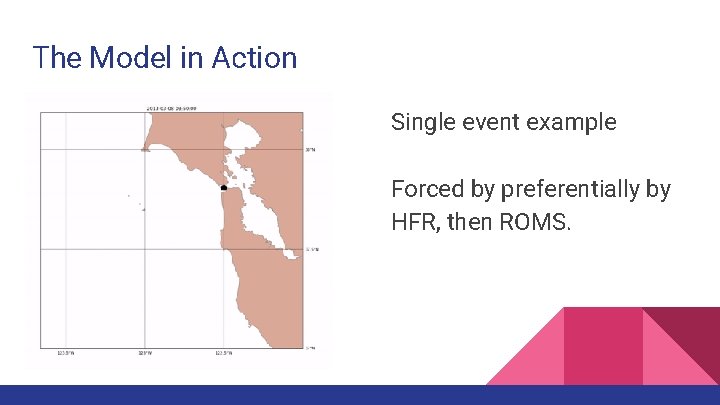
The Model in Action Single event example Forced by preferentially by HFR, then ROMS.
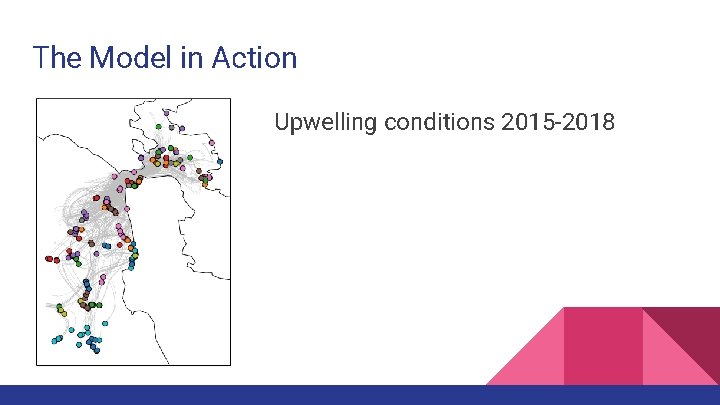
The Model in Action Upwelling conditions 2015 -2018
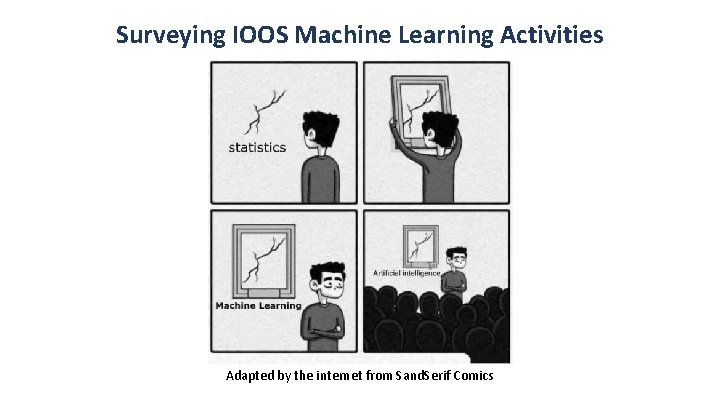
Surveying IOOS Machine Learning Activities Adapted by the internet from Sand. Serif Comics
Dmac
Dmac
Soa dmac
Dmac code
Upstu
Microsoft forefront client security updates
Dsc updates today
Https://twitter.com/search?f=users&q=david%20melin
Mrp inputs
It is the writable phrase of www with interactive data
Pauloh wa updates
Updates windows
+notion +trial
Visio 2010 upgrade
"mail" "blogs" "wikis" "updates" "calendar"
Sage knowledgesync
Incremental updates
Linearization formula
Regional automated property information database