Assessing Forecast Uncertainty in the National Digital Forecast
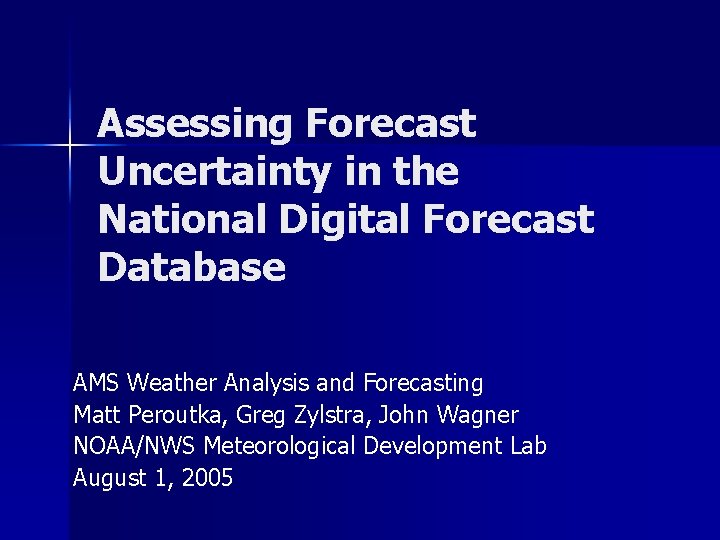
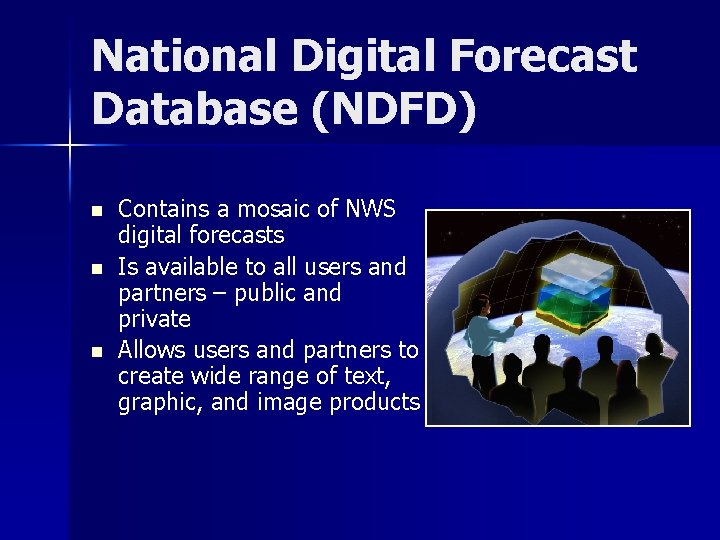
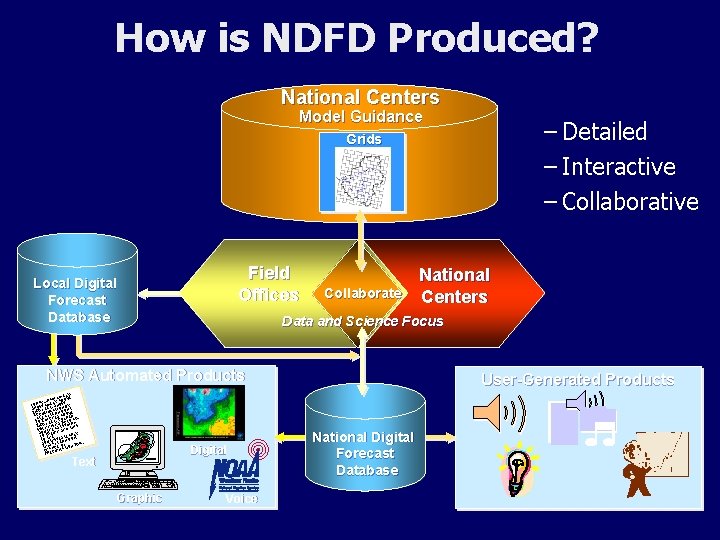
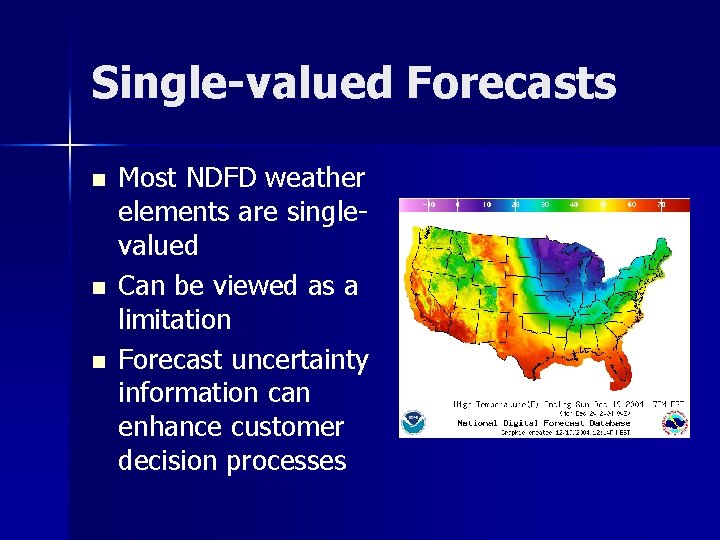
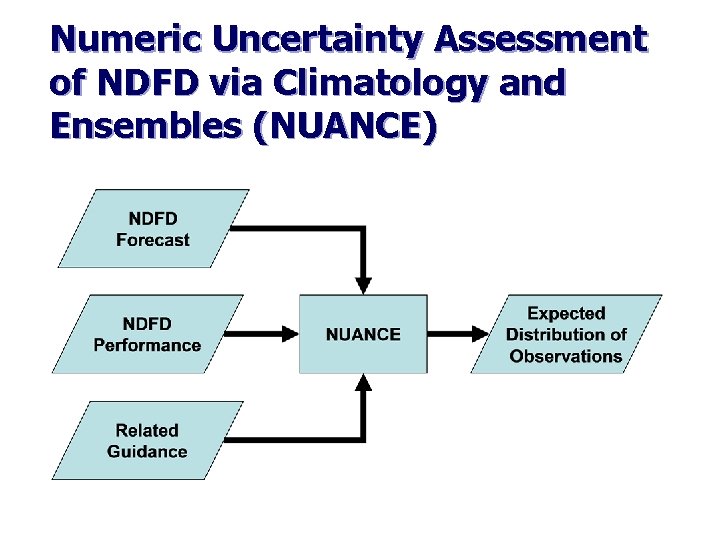
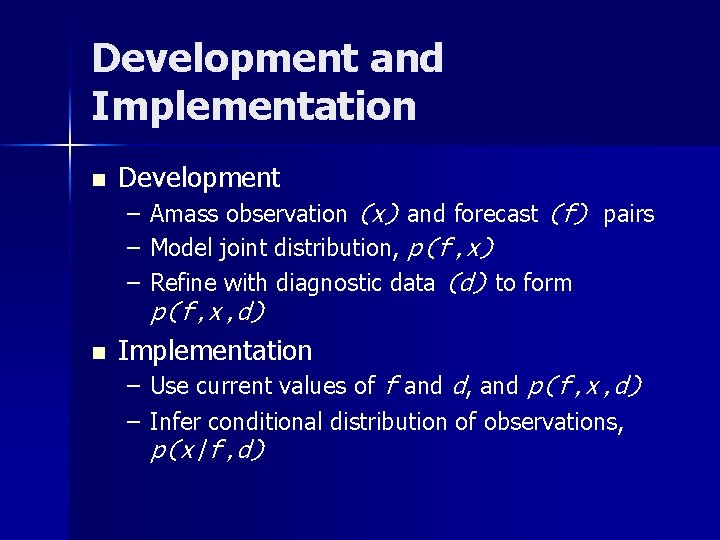
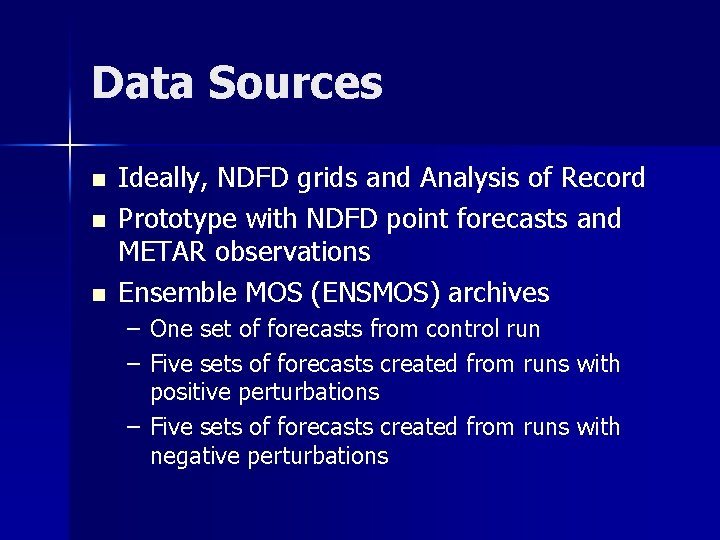
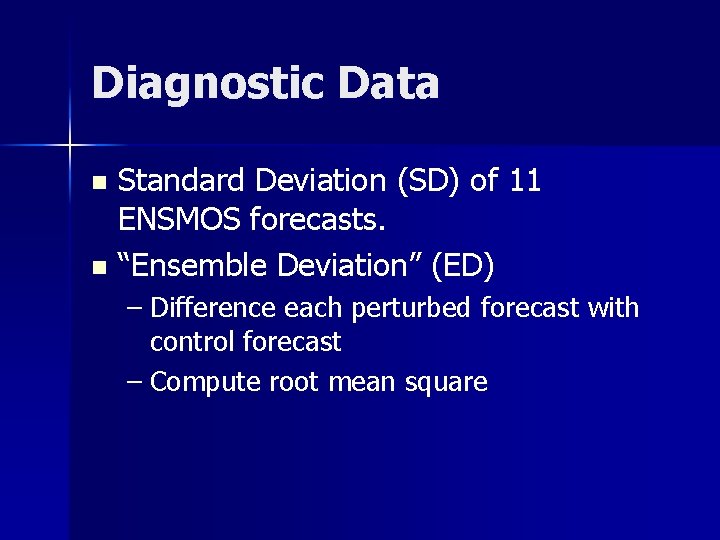
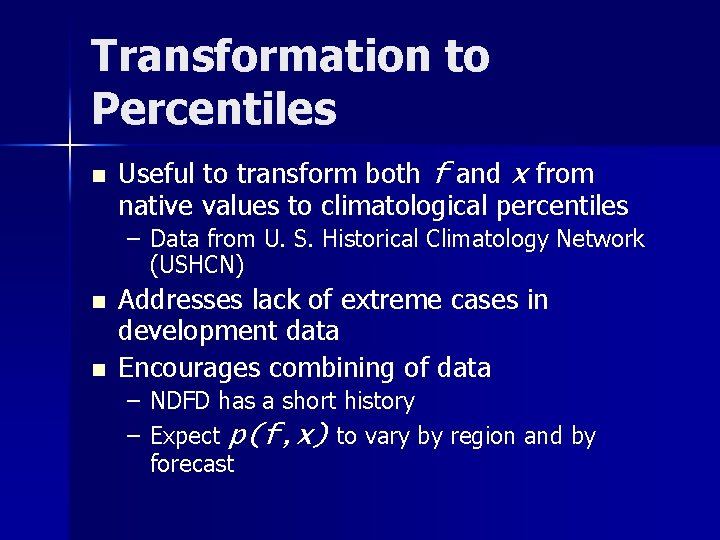
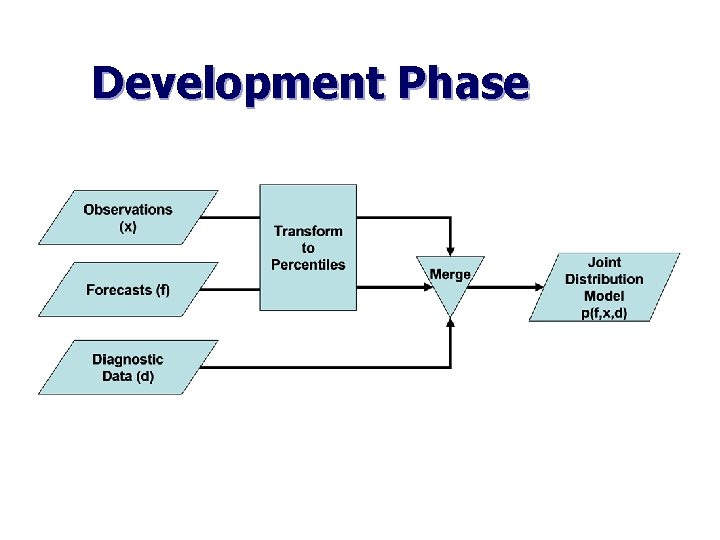
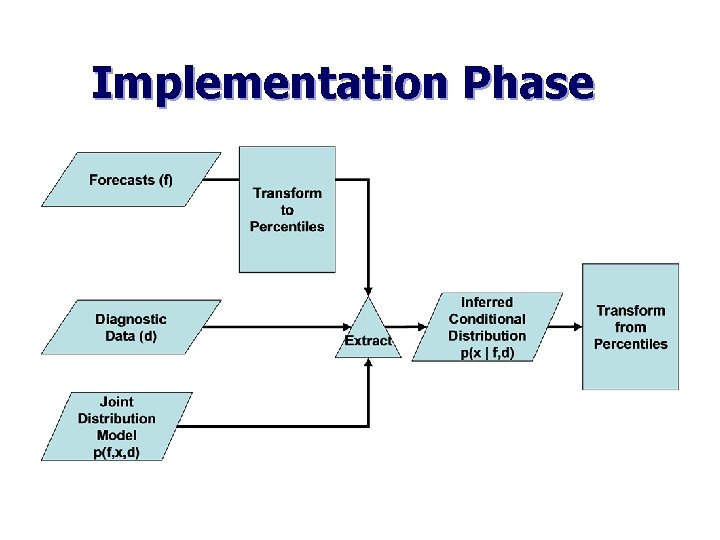
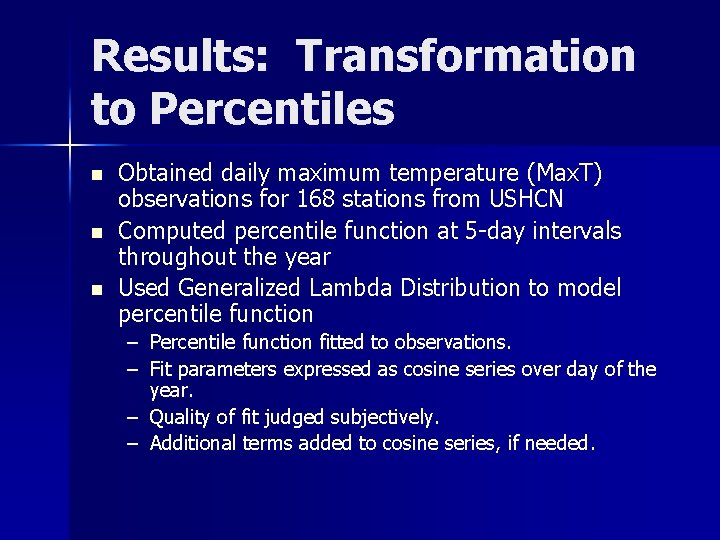
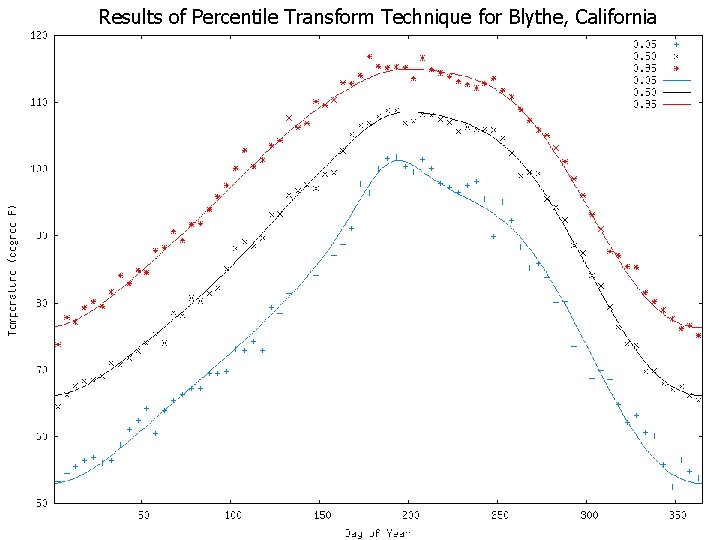
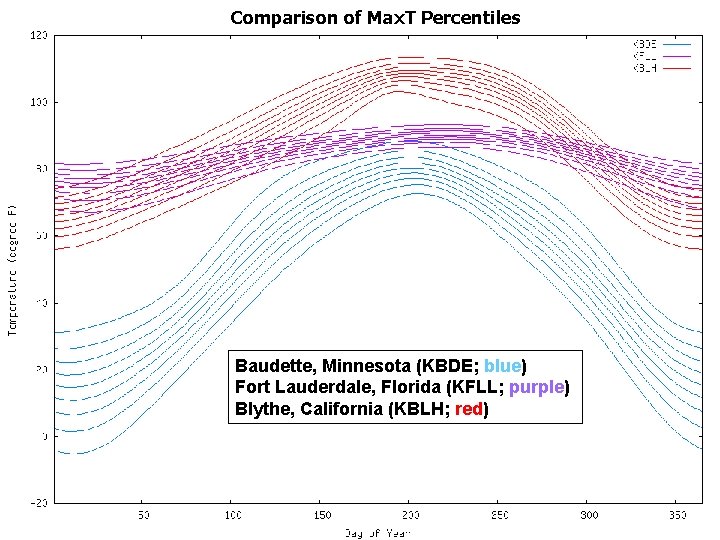
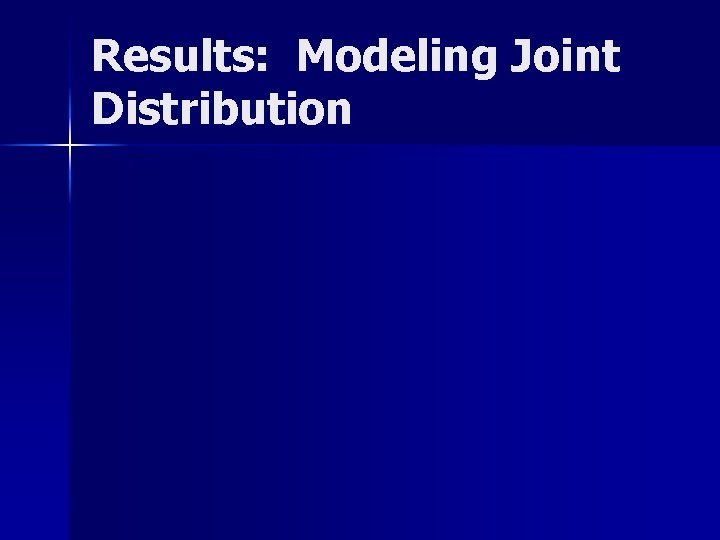
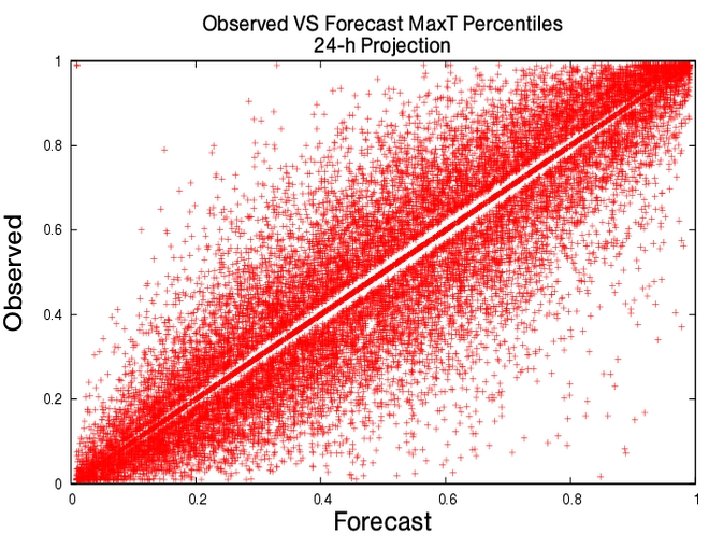
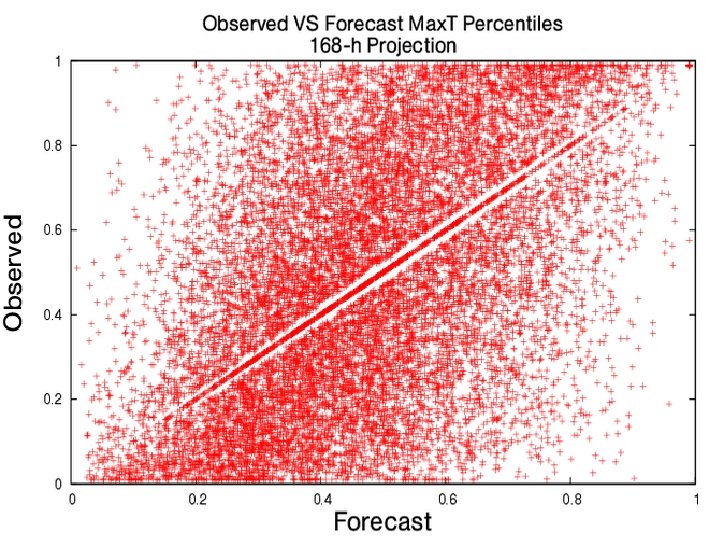
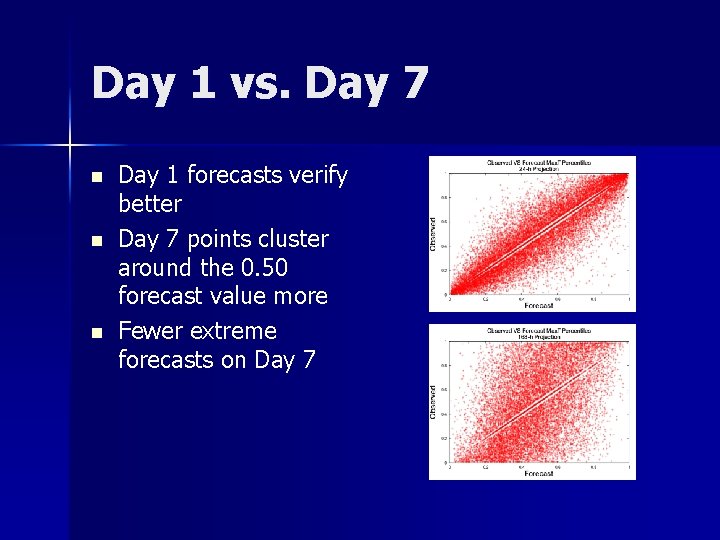
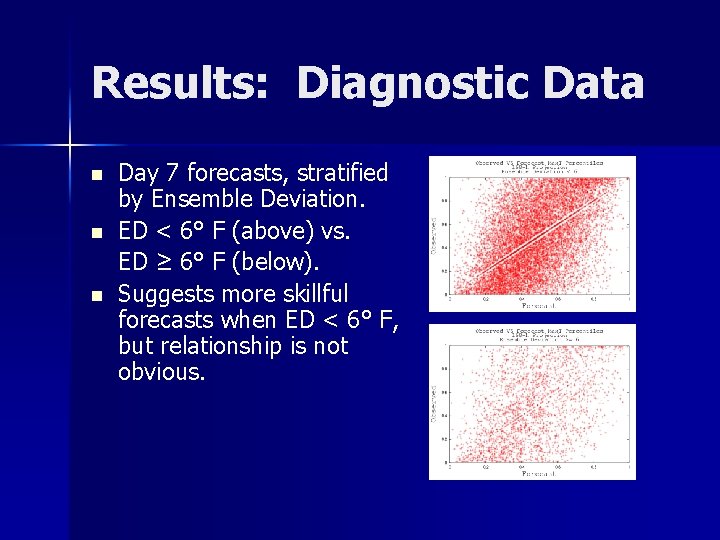
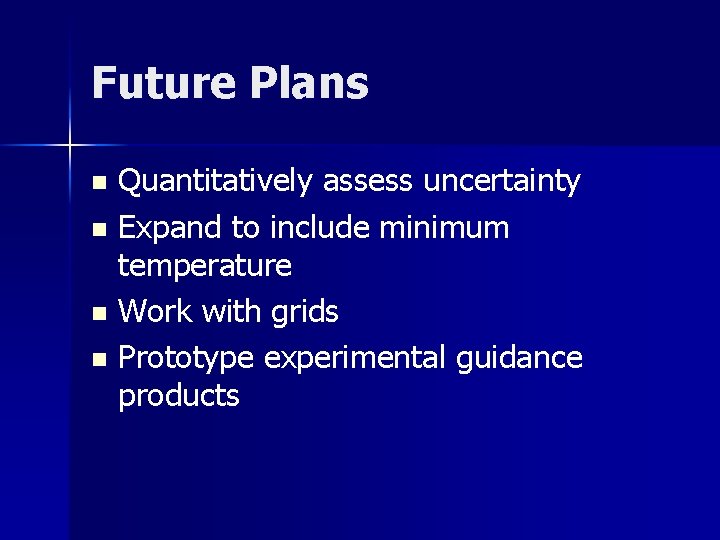
- Slides: 20
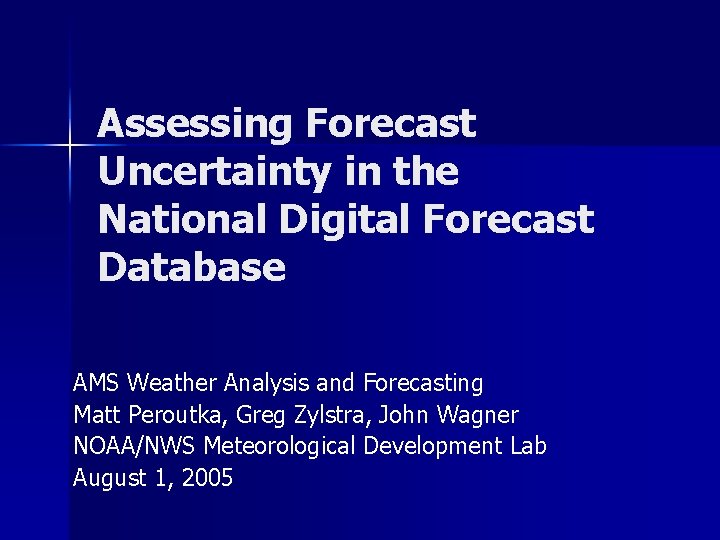
Assessing Forecast Uncertainty in the National Digital Forecast Database AMS Weather Analysis and Forecasting Matt Peroutka, Greg Zylstra, John Wagner NOAA/NWS Meteorological Development Lab August 1, 2005
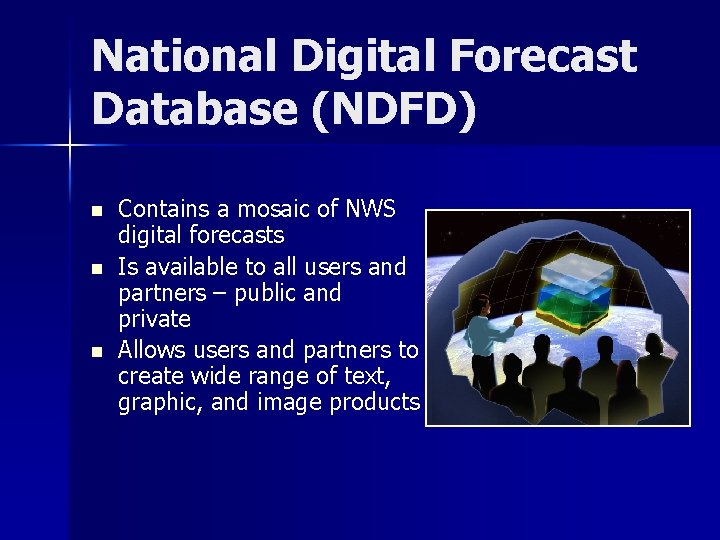
National Digital Forecast Database (NDFD) n n n Contains a mosaic of NWS digital forecasts Is available to all users and partners – public and private Allows users and partners to create wide range of text, graphic, and image products
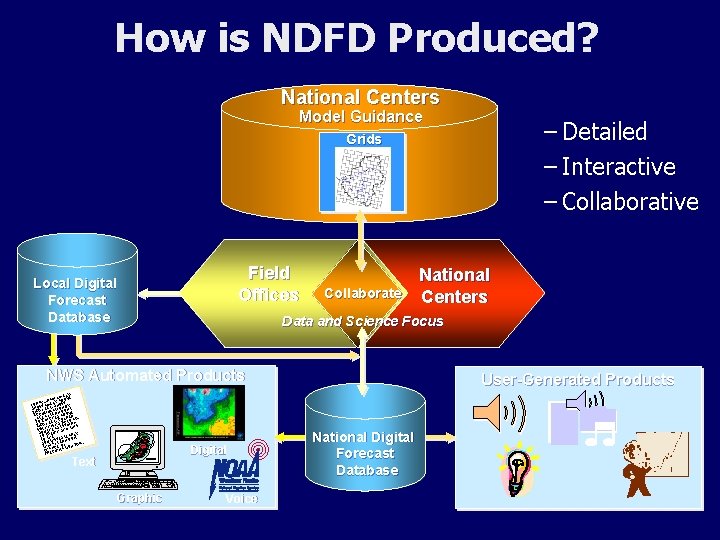
How is NDFD Produced? National Centers Model Guidance – Detailed – Interactive – Collaborative Grids Field Offices Local Digital Forecast Database Collaborate National Centers Data and Science Focus NWS Automated Products LY. LIKEOVE B. RAIN LY A AY. . TODOW LIKE. SNOWBY SN 0 FEET ATION N 1 250 CUMULERNOOOVE AC E AFT ES AB DER LAT 2 INCH. COL TO 40. TO 0 FEETHS 35 D 5 250 H HIG ST WINTING WITUTHEAH SHIF SO 10 MP Y ARL TO THE STE N. TO UTHWE RNOO SO IS AFTEOF 0%. 7 N H E T ANC ATIO CH ECIPIT PR Digital Text Graphic Voice User-Generated Products National Digital Forecast Database
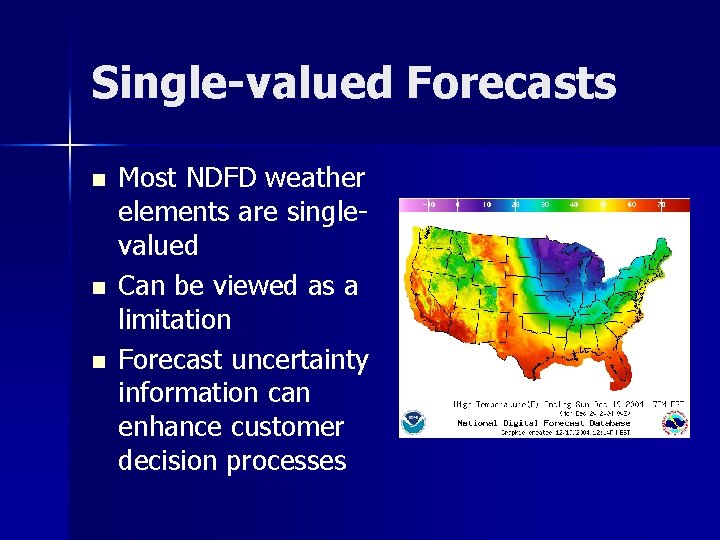
Single-valued Forecasts n n n Most NDFD weather elements are singlevalued Can be viewed as a limitation Forecast uncertainty information can enhance customer decision processes
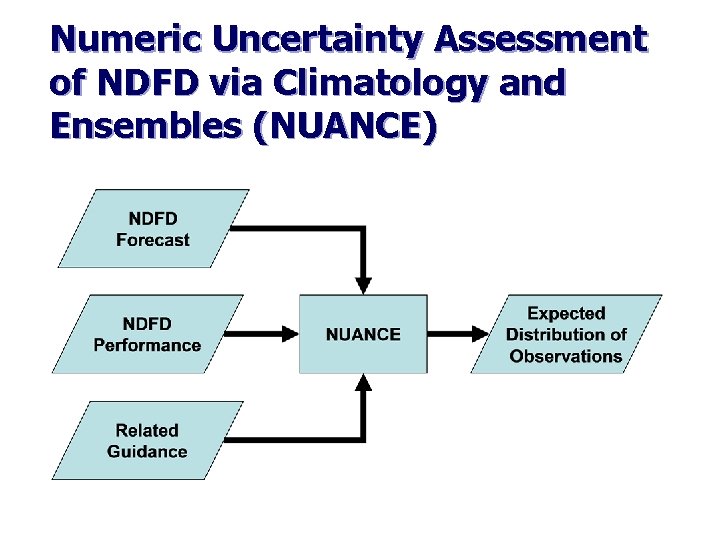
Numeric Uncertainty Assessment of NDFD via Climatology and Ensembles (NUANCE)
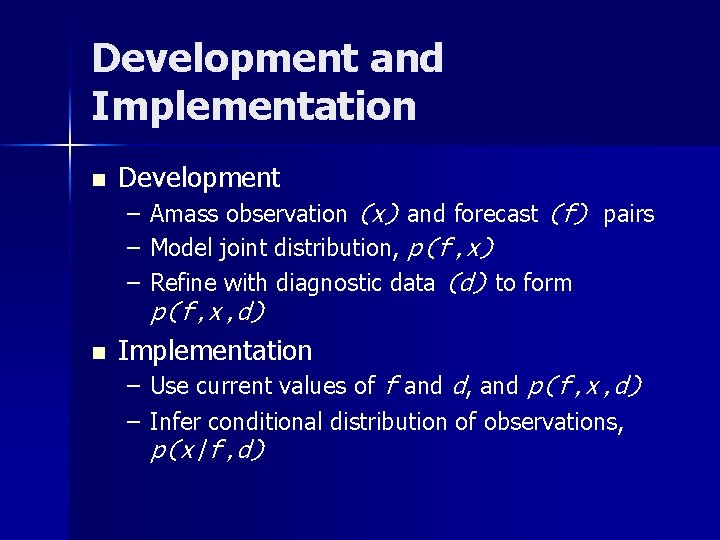
Development and Implementation n Development – Amass observation (x) and forecast (f) pairs – Model joint distribution, p(f, x) – Refine with diagnostic data (d) to form p(f, x, d) n Implementation – Use current values of f and d, and p(f, x, d) – Infer conditional distribution of observations, p(x|f, d)
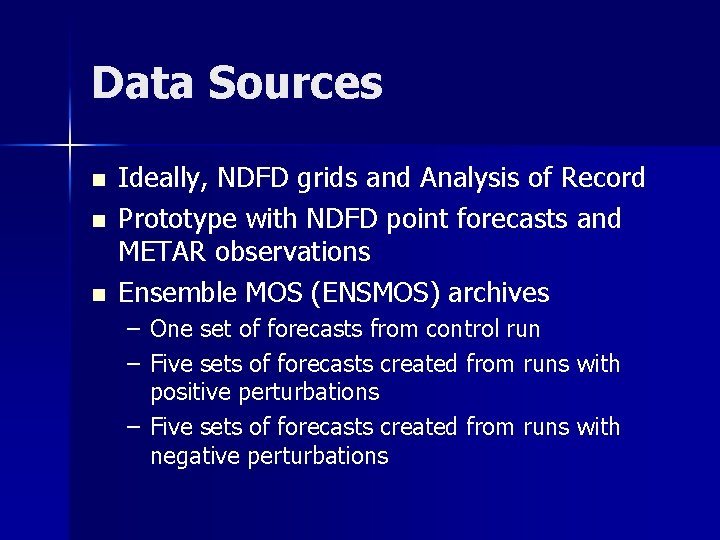
Data Sources n n n Ideally, NDFD grids and Analysis of Record Prototype with NDFD point forecasts and METAR observations Ensemble MOS (ENSMOS) archives – One set of forecasts from control run – Five sets of forecasts created from runs with positive perturbations – Five sets of forecasts created from runs with negative perturbations
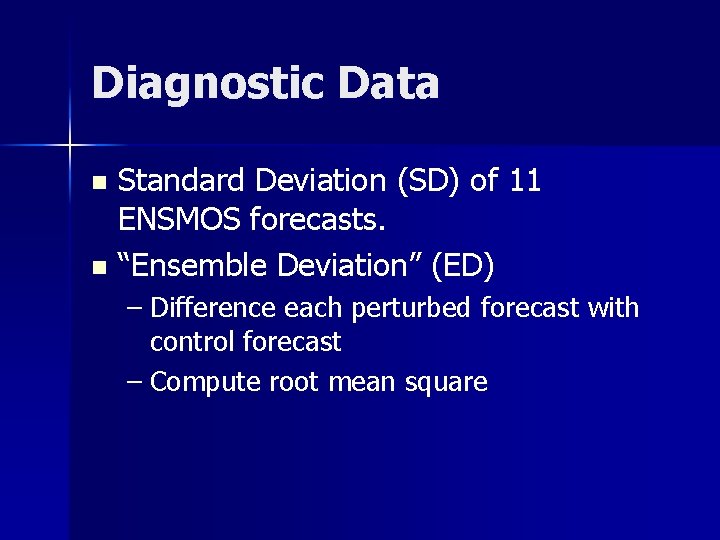
Diagnostic Data Standard Deviation (SD) of 11 ENSMOS forecasts. n “Ensemble Deviation” (ED) n – Difference each perturbed forecast with control forecast – Compute root mean square
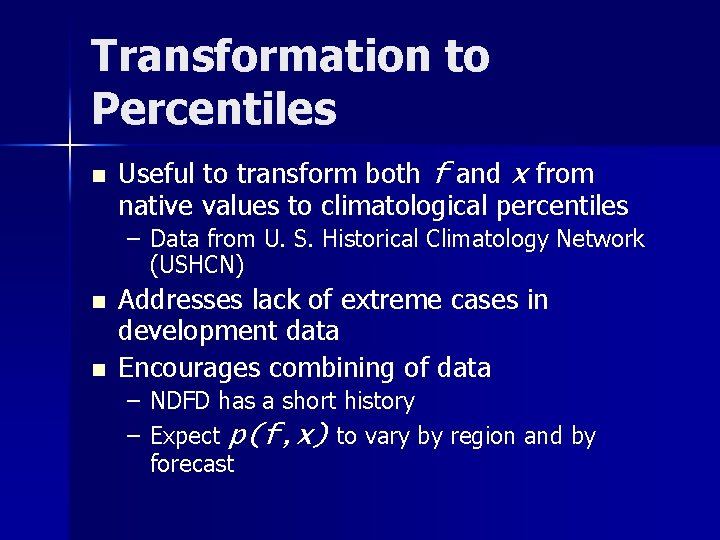
Transformation to Percentiles n Useful to transform both f and x from native values to climatological percentiles – Data from U. S. Historical Climatology Network (USHCN) n n Addresses lack of extreme cases in development data Encourages combining of data – NDFD has a short history – Expect p(f, x) to vary by region and by forecast
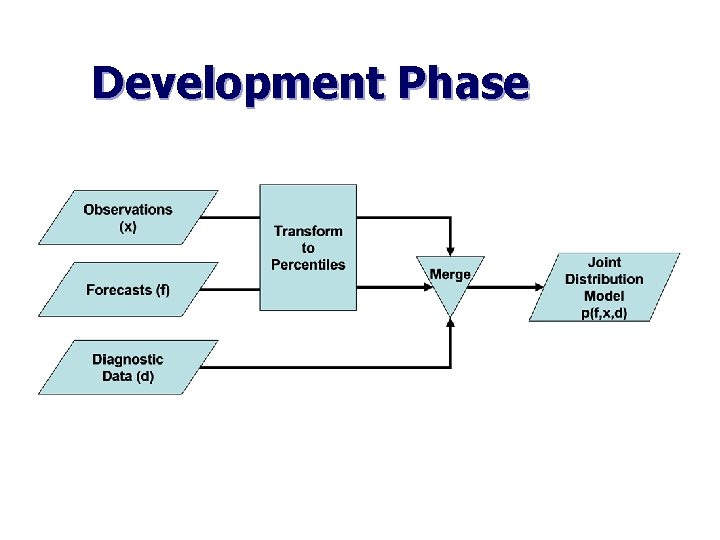
Development Phase
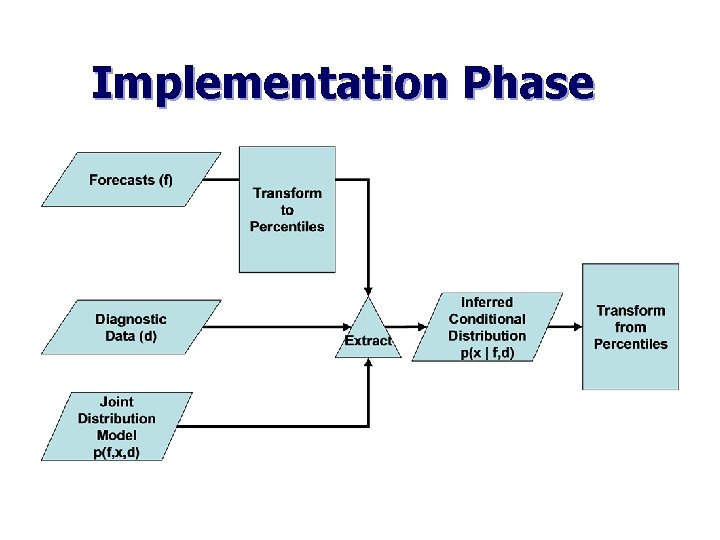
Implementation Phase
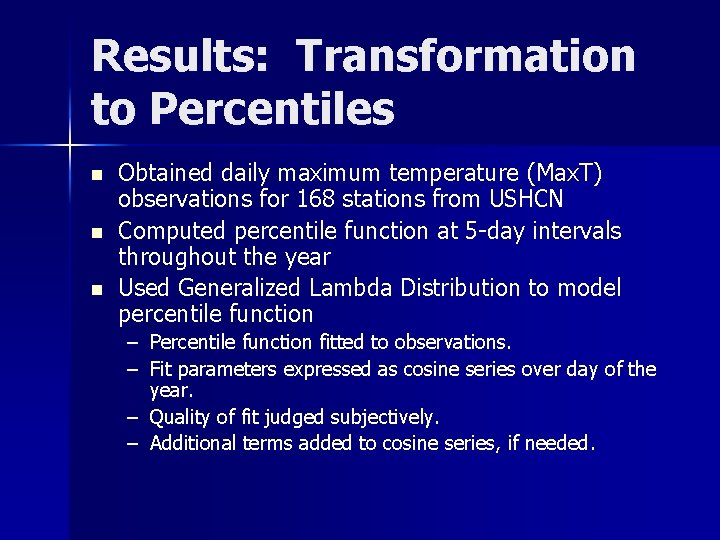
Results: Transformation to Percentiles n n n Obtained daily maximum temperature (Max. T) observations for 168 stations from USHCN Computed percentile function at 5 -day intervals throughout the year Used Generalized Lambda Distribution to model percentile function – Percentile function fitted to observations. – Fit parameters expressed as cosine series over day of the year. – Quality of fit judged subjectively. – Additional terms added to cosine series, if needed.
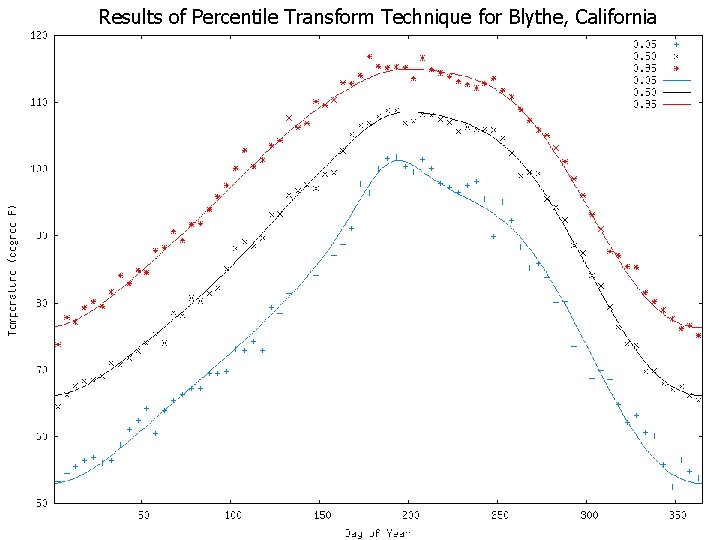
Results of Percentile Transform Technique for Blythe, California
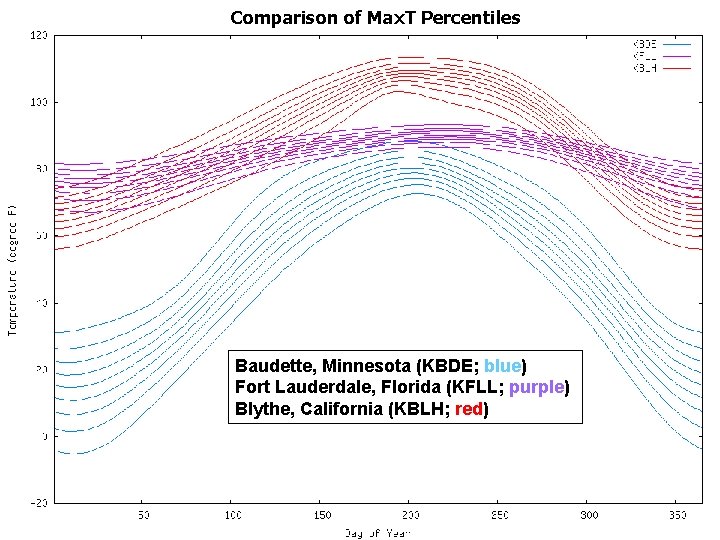
Comparison of Max. T Percentiles Baudette, Minnesota (KBDE; blue) Fort Lauderdale, Florida (KFLL; purple) Blythe, California (KBLH; red)
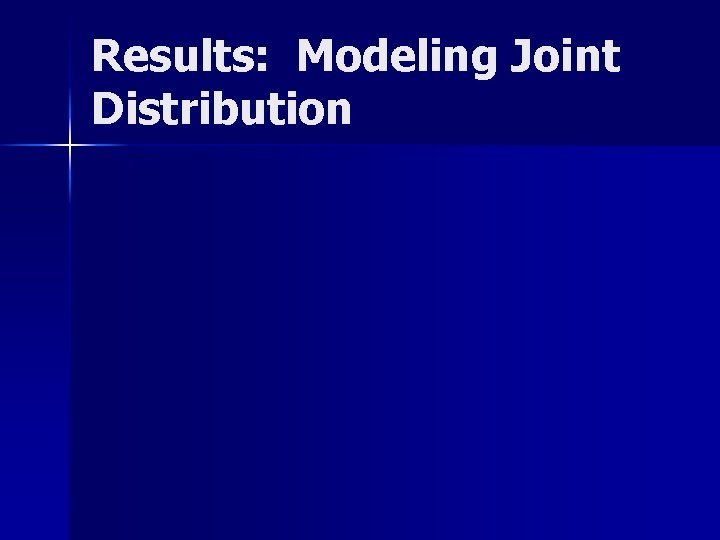
Results: Modeling Joint Distribution
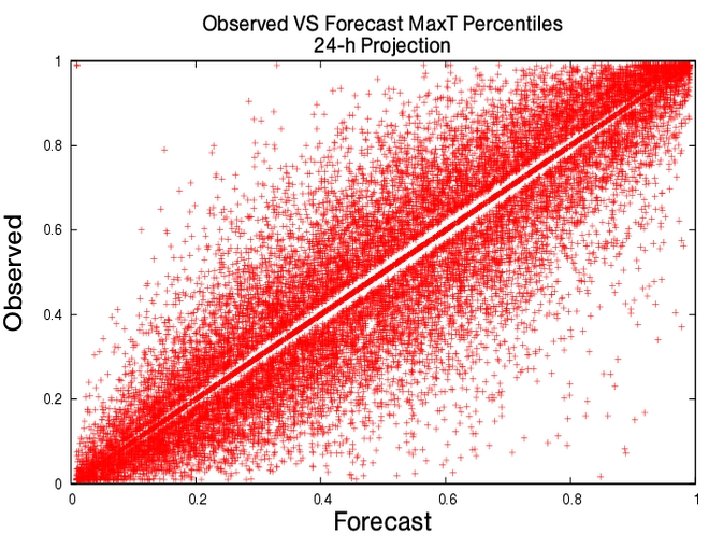
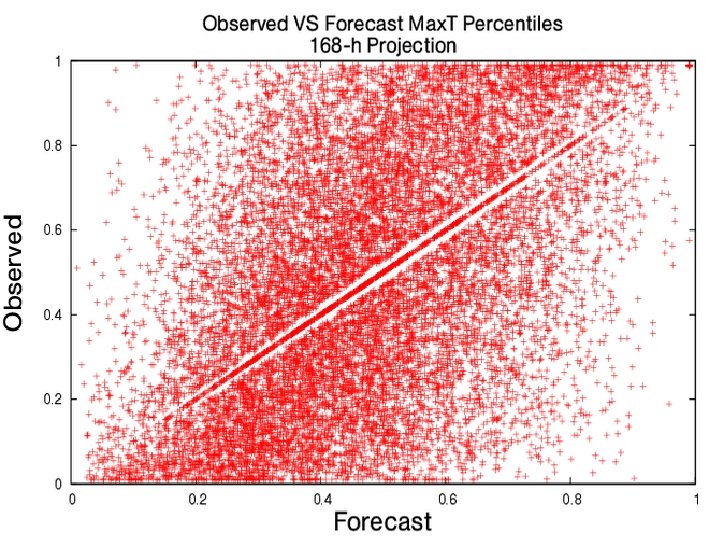
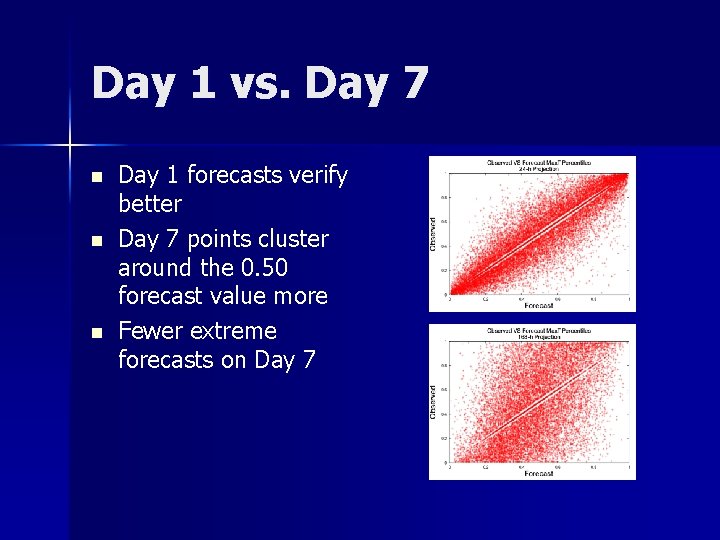
Day 1 vs. Day 7 n n n Day 1 forecasts verify better Day 7 points cluster around the 0. 50 forecast value more Fewer extreme forecasts on Day 7
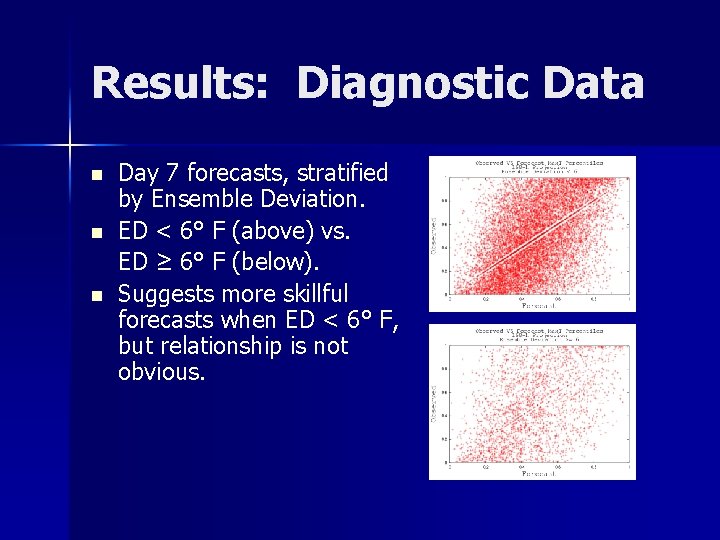
Results: Diagnostic Data n n n Day 7 forecasts, stratified by Ensemble Deviation. ED < 6° F (above) vs. ED ≥ 6° F (below). Suggests more skillful forecasts when ED < 6° F, but relationship is not obvious.
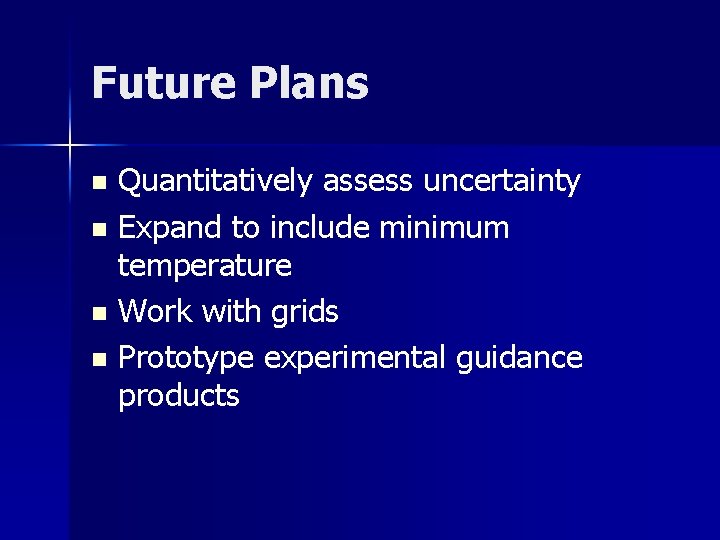
Future Plans Quantitatively assess uncertainty n Expand to include minimum temperature n Work with grids n Prototype experimental guidance products n
Imt 556
Btec sport unit 3
Unit 18 assessing children's development support needs
Assessing grammar effectively
Career stage 2 proficient teacher
Assessing need for hrd
What is informal assessment
Manual for assessing safety hardware
Cultural dynamics in assessing global markets
Assessing a new venture's financial strength and viability
Assessing cardiorespiratory fitness
Assessing leadership and measuring its effects
Many new drivers first fender bender is a backing collision
Module 4 topic 1 assessing and managing risk
A nine box matrix requires assessing employees on ________.
Oecd maps
Core multiple measures
While assessing the risks of material misstatement
Amc orange county
Assessing health status chapter 22
Assessing motivation to change