Areas of Research Causal Discovery Integration Sofia Triantafillou
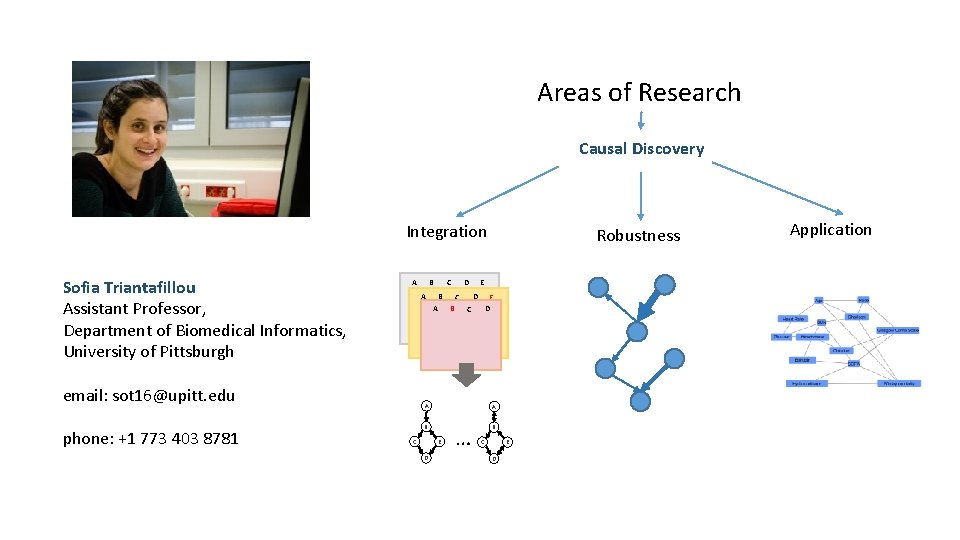
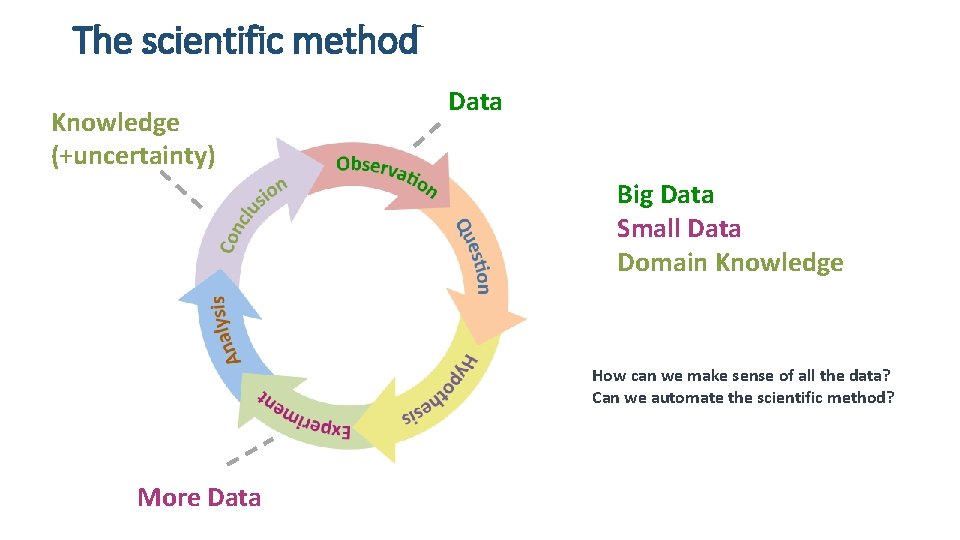
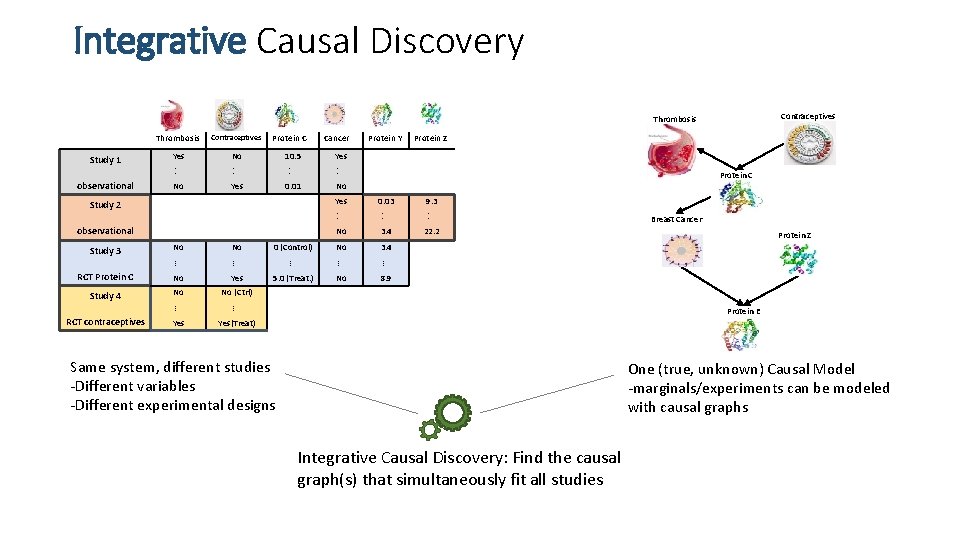
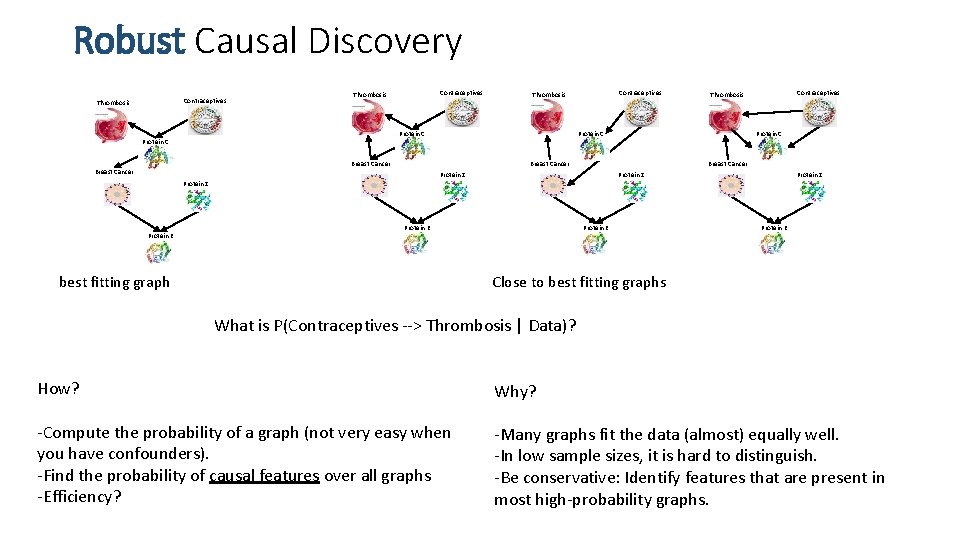
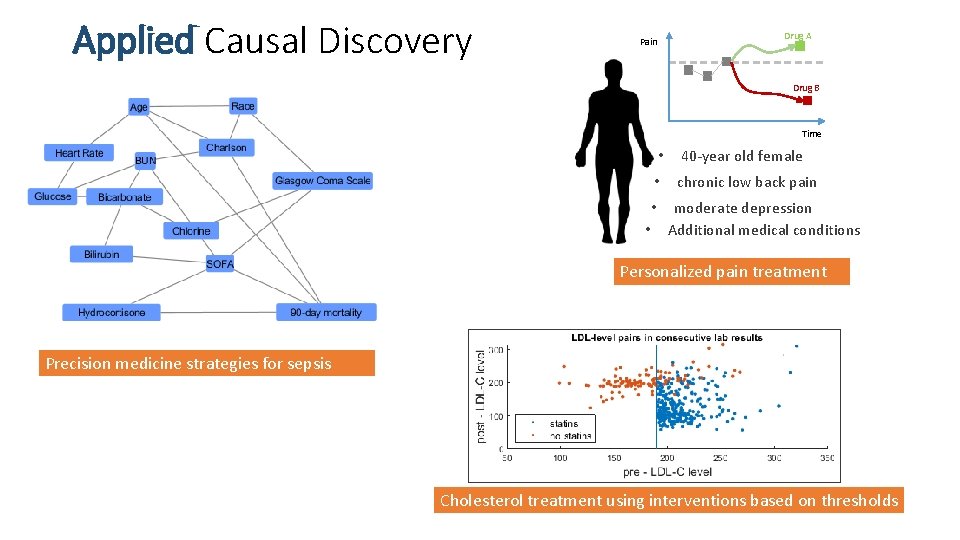
- Slides: 5
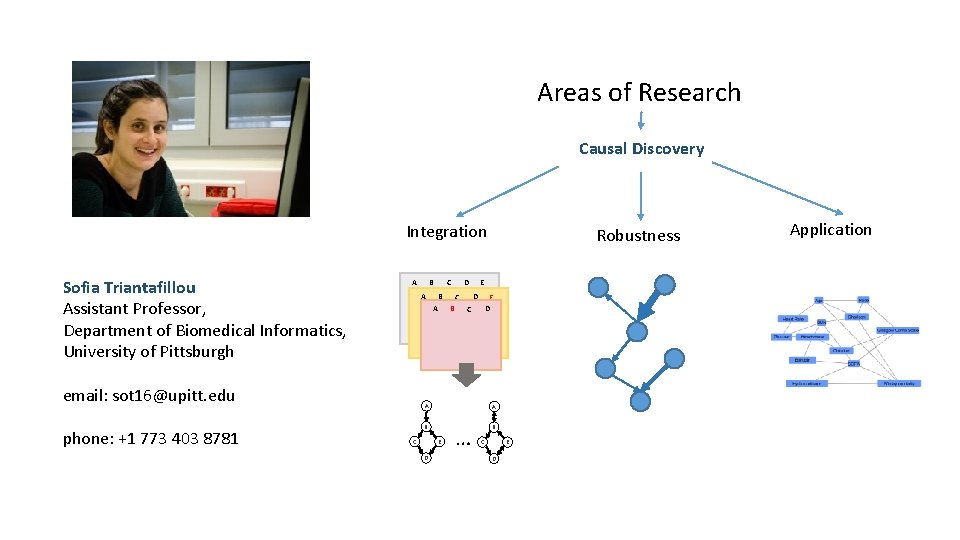
Areas of Research Causal Discovery Integration Sofia Triantafillou Assistant Professor, Department of Biomedical Informatics, University of Pittsburgh A C B A email: sot 16@upitt. edu phone: +1 773 403 8781 B A D E D C B E D C A A B C E D Robustness … B C E D Application
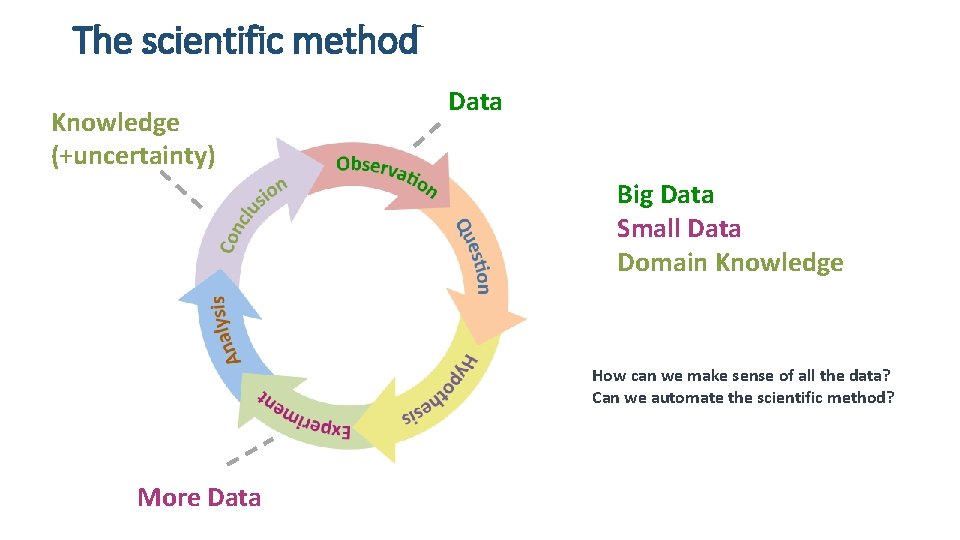
The scientific method Knowledge (+uncertainty) Data Big Data Small Data Domain Knowledge How can we make sense of all the data? Can we automate the scientific method? More Data
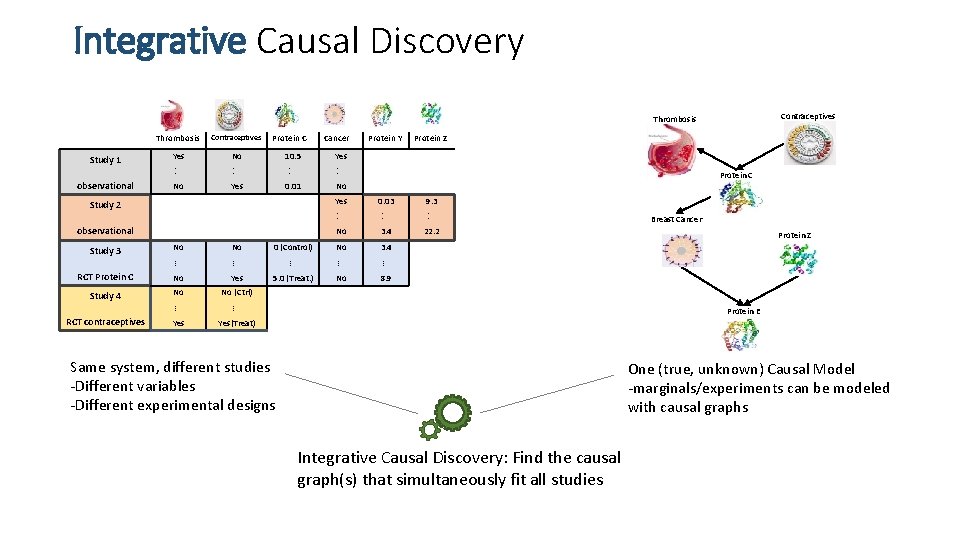
Integrative Causal Discovery Contraceptives Thrombosis Study 1 Contraceptives Yes No Protein C 10. 5 Yes … … … No Yes 0. 01 No Study 2 Protein C Yes 0. 03 9. 3 … … No Protein Z … No Protein Y No 3. 4 22. 2 0 (Control) No 3. 4 observational Study 3 Cancer … observational Thrombosis … … No Yes 5. 0 (Treat. ) No 8. 9 Study 4 No No (Ctrl) … … … RCT Protein C RCT contraceptives Yes(Treat) Breast Cancer Protein Z Protein E Same system, different studies -Different variables -Different experimental designs One (true, unknown) Causal Model -marginals/experiments can be modeled with causal graphs Integrative Causal Discovery: Find the causal graph(s) that simultaneously fit all studies
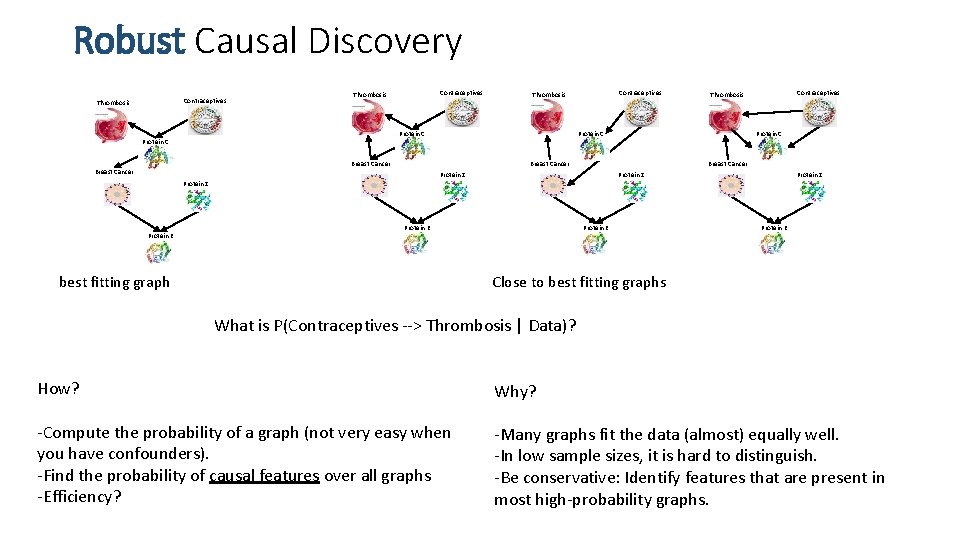
Robust Causal Discovery Contraceptives Thrombosis Breast Cancer Protein Z Protein E Protein C Breast Cancer Contraceptives Thrombosis Protein C Contraceptives Thrombosis Protein E Protein Z Protein E Close to best fitting graphs best fitting graph What is P(Contraceptives --> Thrombosis | Data)? How? Why? -Compute the probability of a graph (not very easy when you have confounders). -Find the probability of causal features over all graphs -Efficiency? -Many graphs fit the data (almost) equally well. -In low sample sizes, it is hard to distinguish. -Be conservative: Identify features that are present in most high-probability graphs.
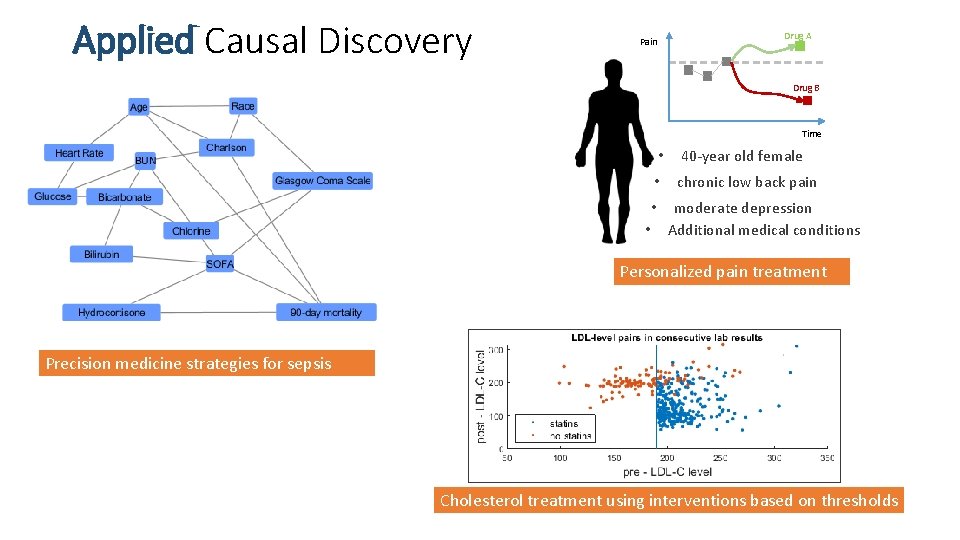
Applied Causal Discovery Drug A Pain Drug B Time • 40 -year old female • chronic low back pain • • moderate depression Additional medical conditions Personalized pain treatment Precision medicine strategies for sepsis Cholesterol treatment using interventions based on thresholds