Algorithmic Management and Fairness Peter Cappelli George W
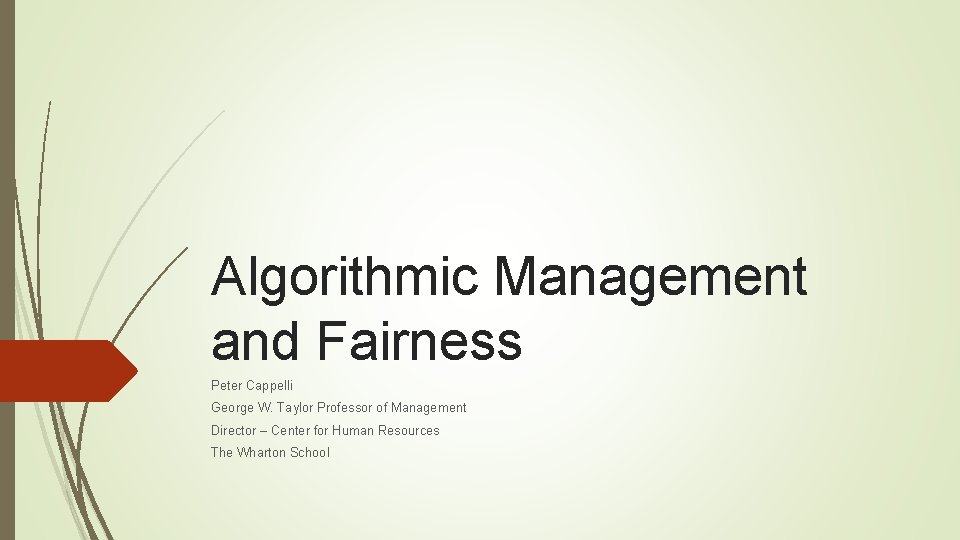
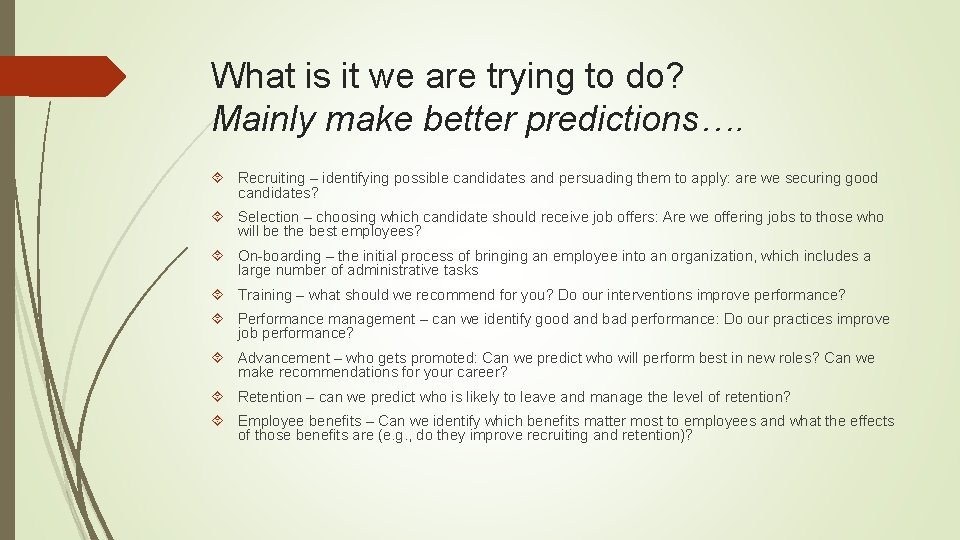
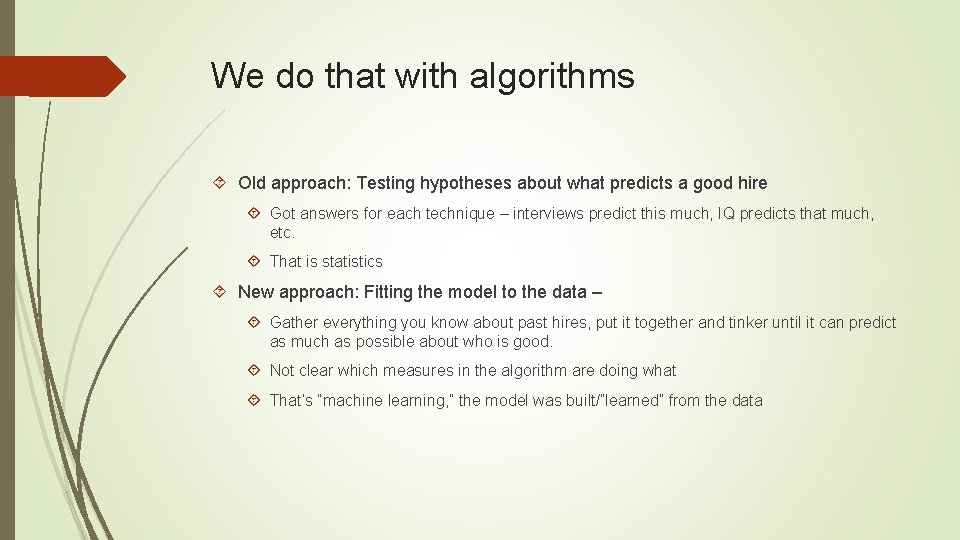
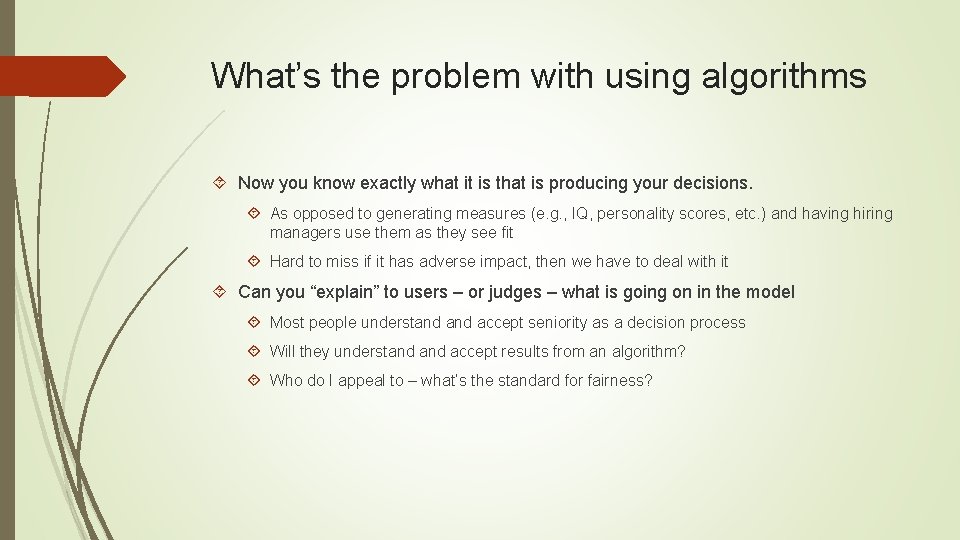
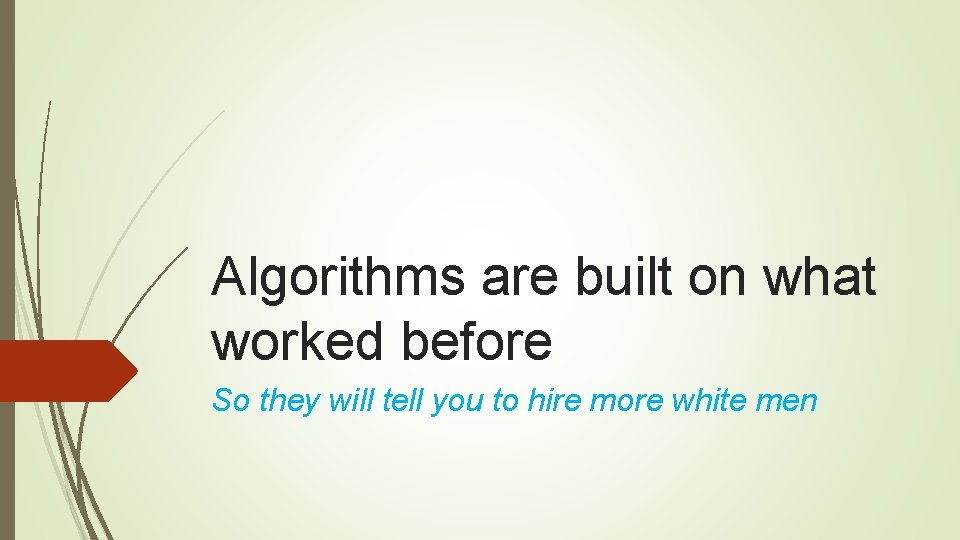
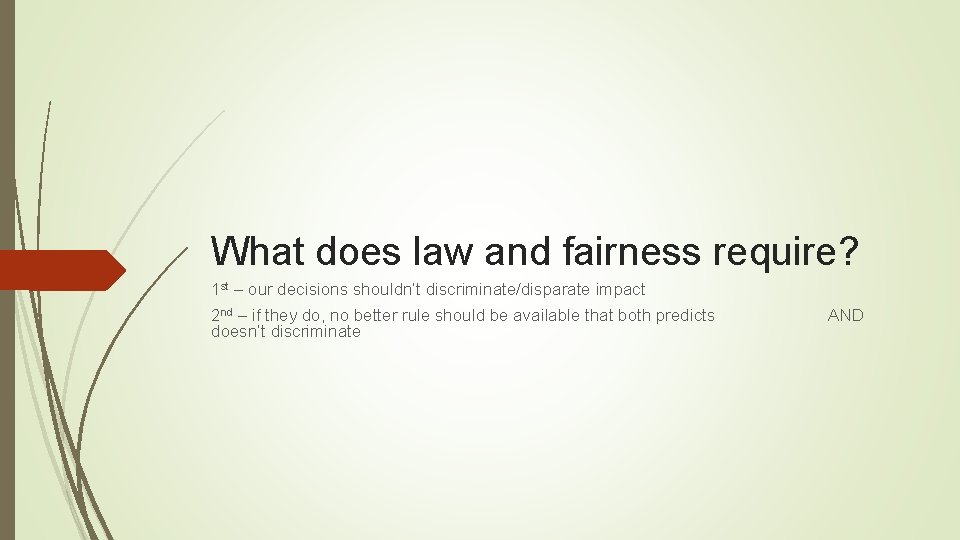
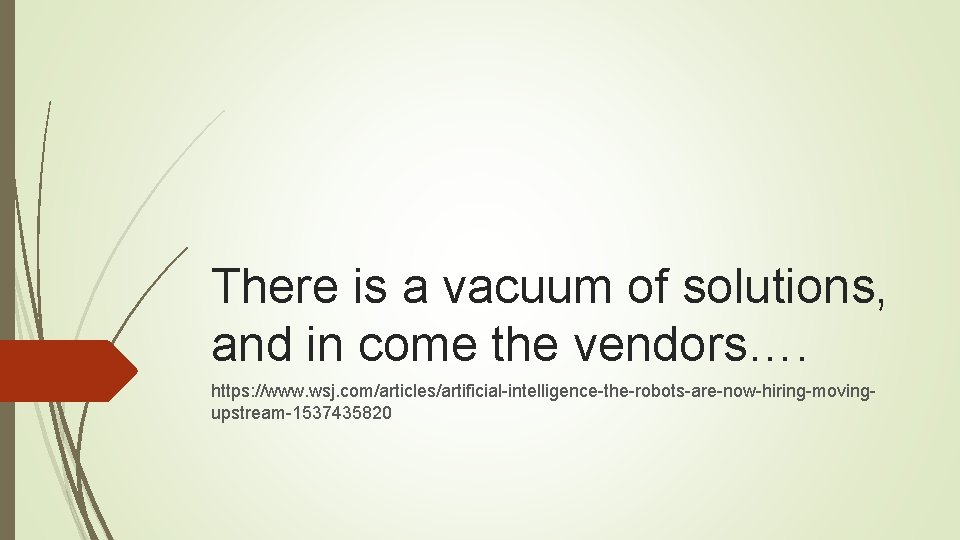
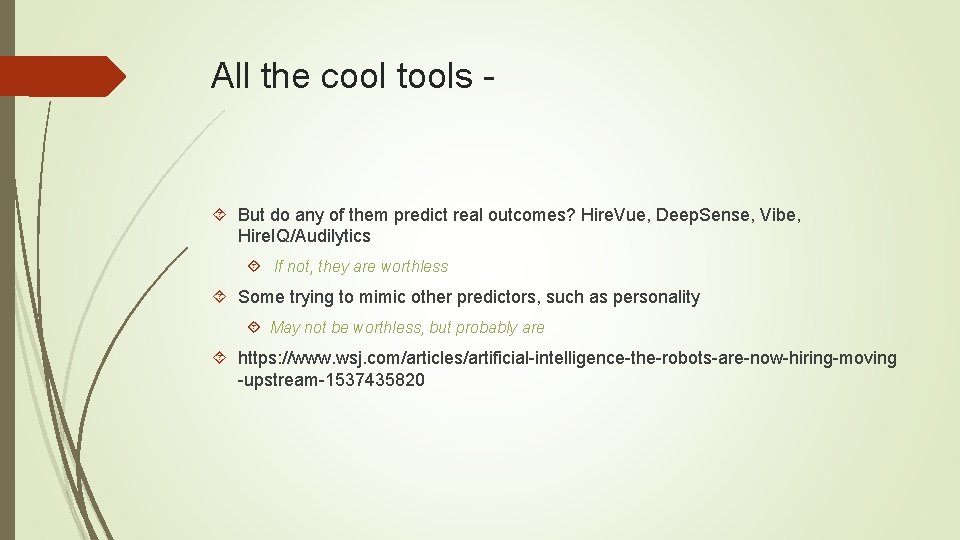
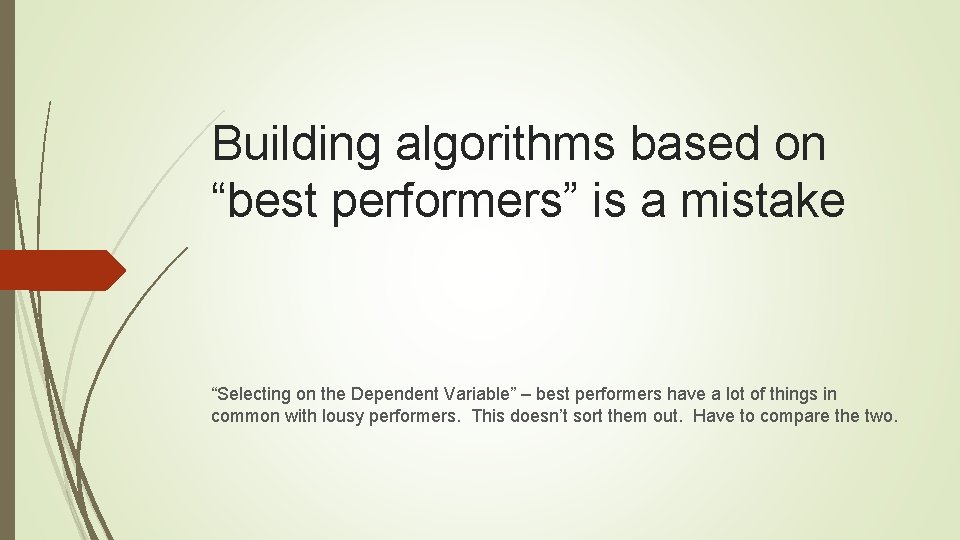
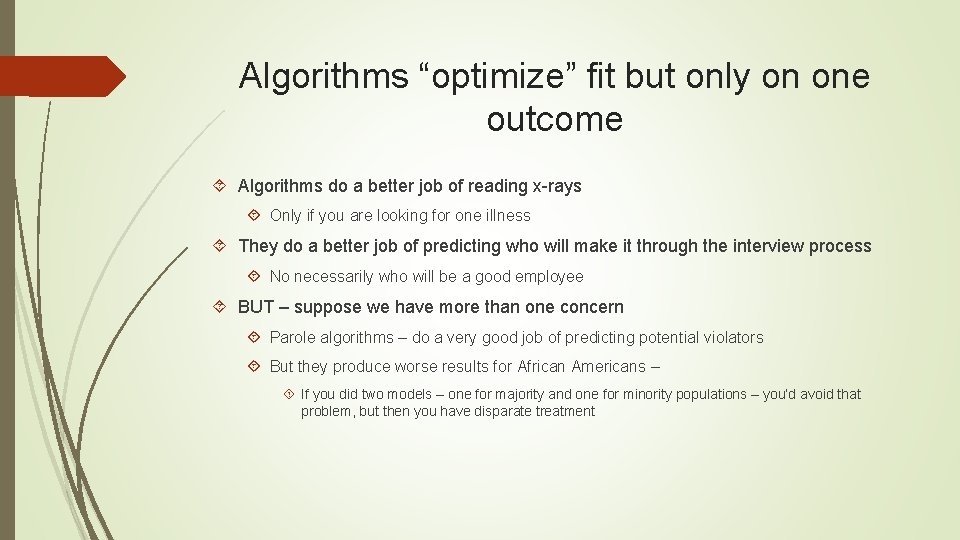
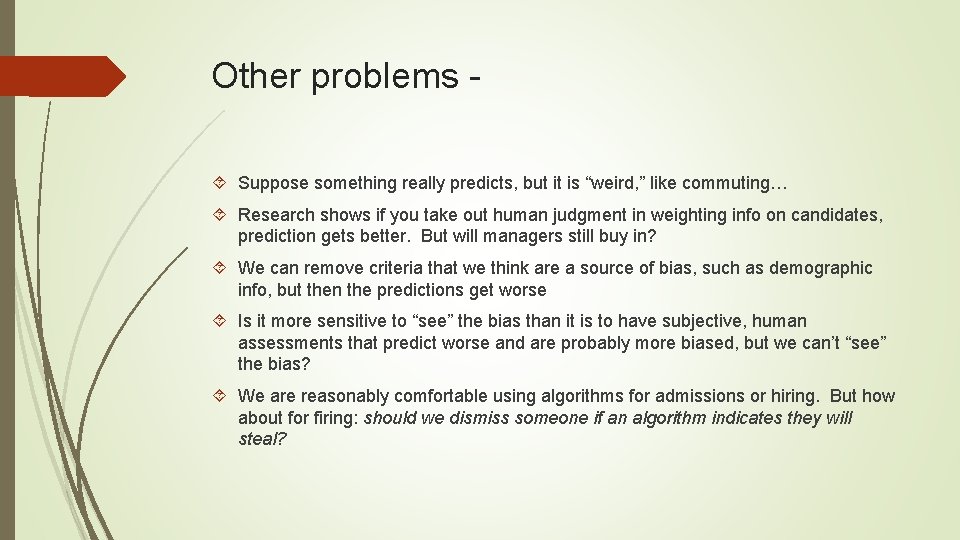
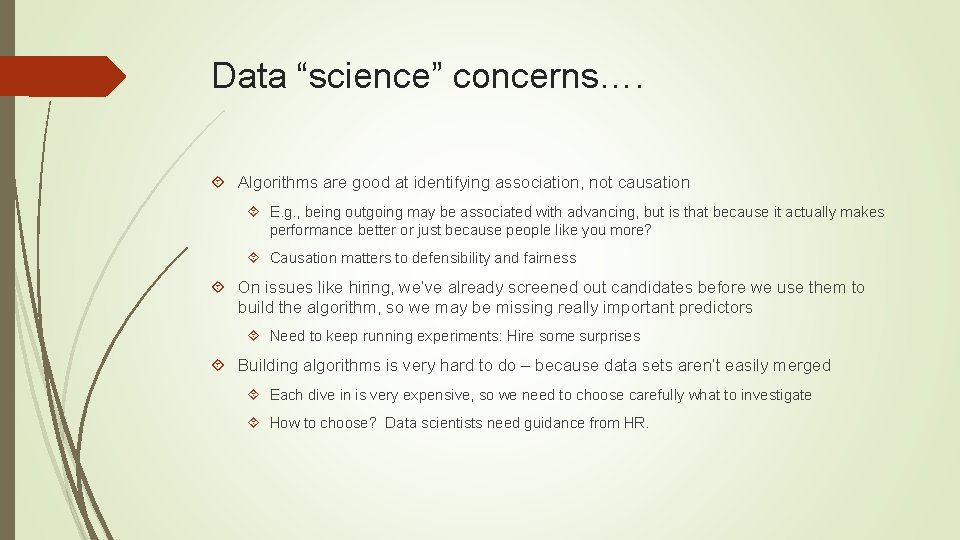
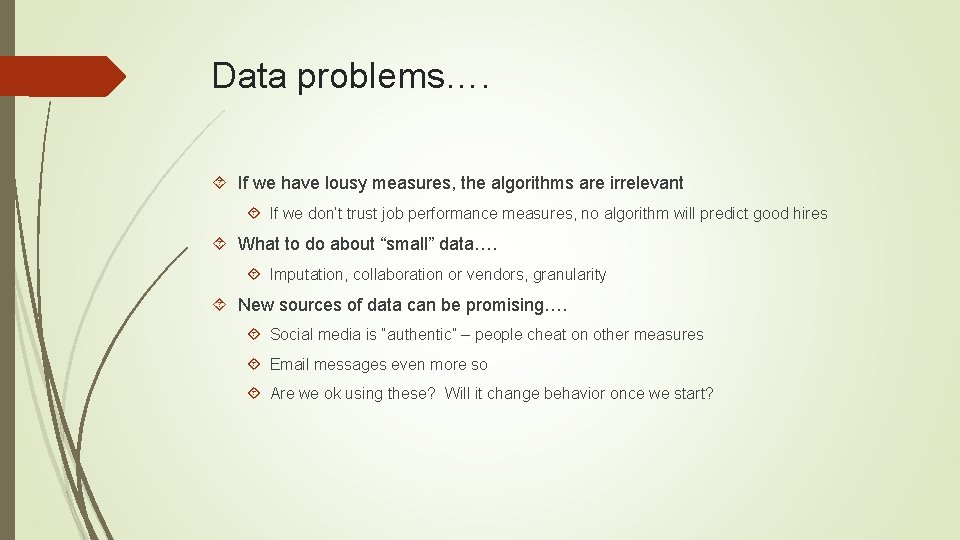
- Slides: 13
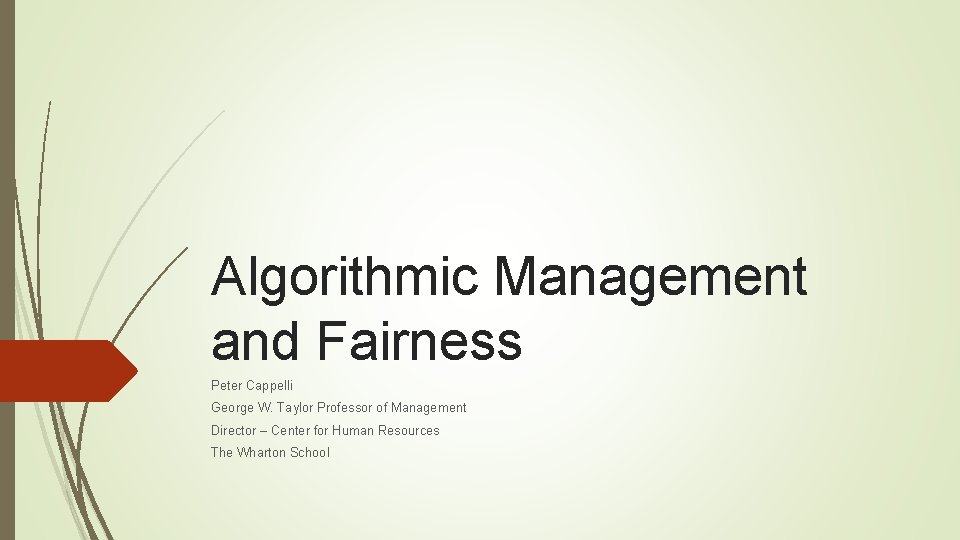
Algorithmic Management and Fairness Peter Cappelli George W. Taylor Professor of Management Director – Center for Human Resources The Wharton School
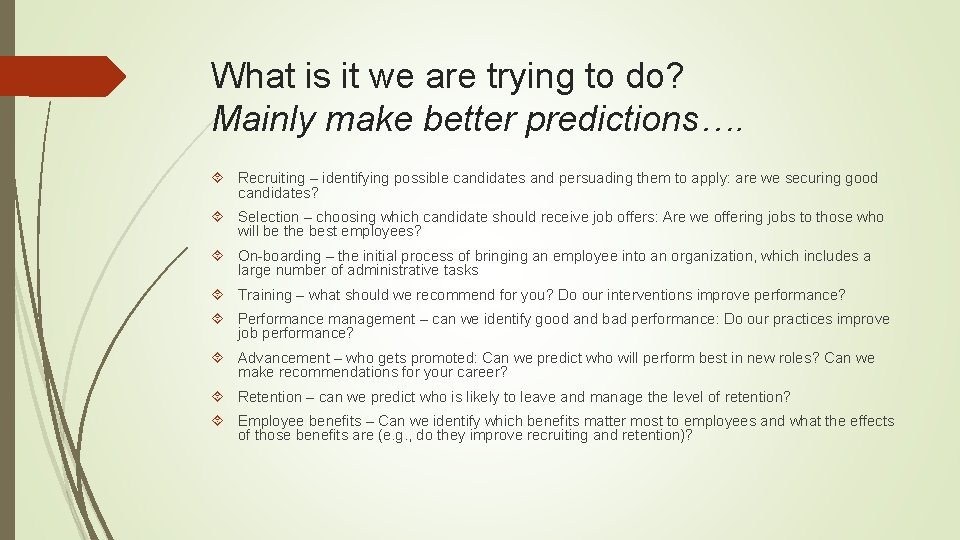
What is it we are trying to do? Mainly make better predictions…. Recruiting – identifying possible candidates and persuading them to apply: are we securing good candidates? Selection – choosing which candidate should receive job offers: Are we offering jobs to those who will be the best employees? On-boarding – the initial process of bringing an employee into an organization, which includes a large number of administrative tasks Training – what should we recommend for you? Do our interventions improve performance? Performance management – can we identify good and bad performance: Do our practices improve job performance? Advancement – who gets promoted: Can we predict who will perform best in new roles? Can we make recommendations for your career? Retention – can we predict who is likely to leave and manage the level of retention? Employee benefits – Can we identify which benefits matter most to employees and what the effects of those benefits are (e. g. , do they improve recruiting and retention)?
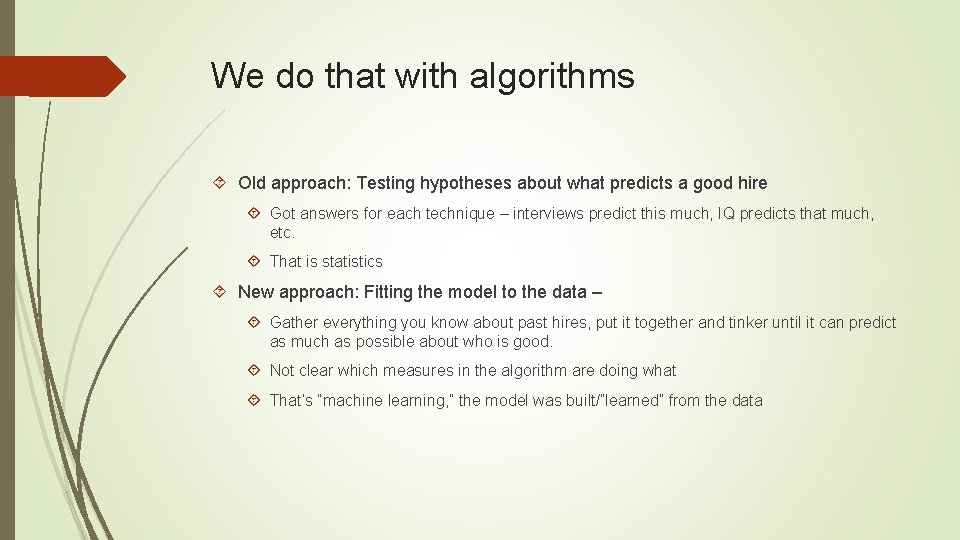
We do that with algorithms Old approach: Testing hypotheses about what predicts a good hire Got answers for each technique – interviews predict this much, IQ predicts that much, etc. That is statistics New approach: Fitting the model to the data – Gather everything you know about past hires, put it together and tinker until it can predict as much as possible about who is good. Not clear which measures in the algorithm are doing what That’s “machine learning, ” the model was built/”learned” from the data
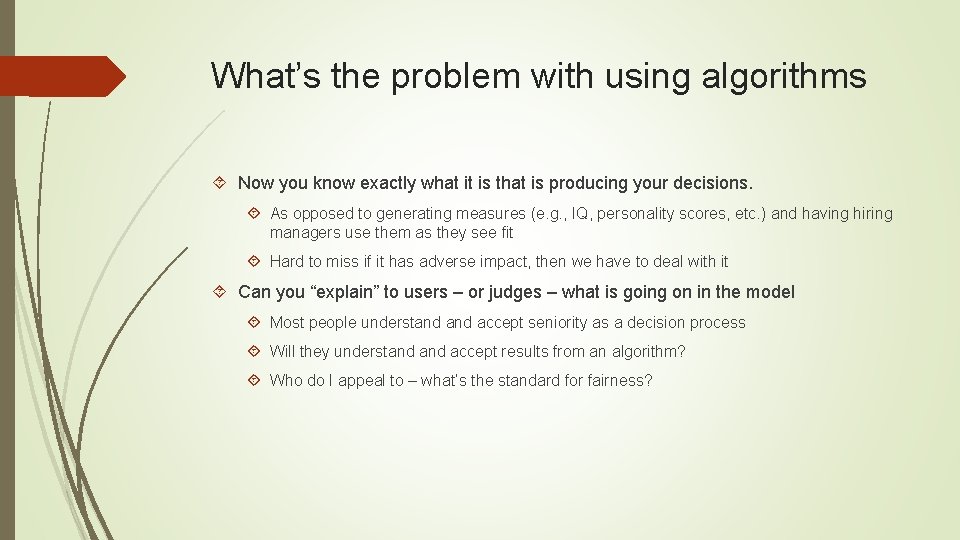
What’s the problem with using algorithms Now you know exactly what it is that is producing your decisions. As opposed to generating measures (e. g. , IQ, personality scores, etc. ) and having hiring managers use them as they see fit Hard to miss if it has adverse impact, then we have to deal with it Can you “explain” to users – or judges – what is going on in the model Most people understand accept seniority as a decision process Will they understand accept results from an algorithm? Who do I appeal to – what’s the standard for fairness?
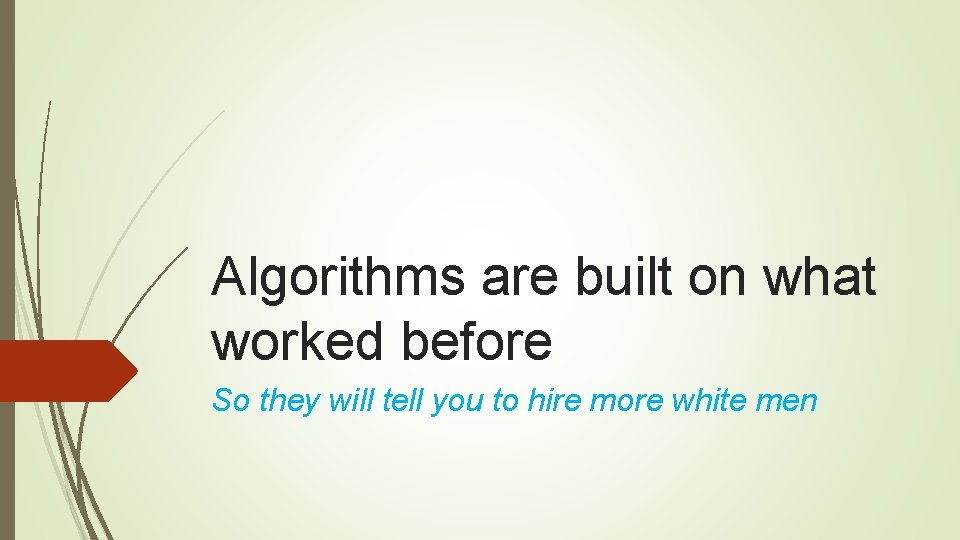
Algorithms are built on what worked before So they will tell you to hire more white men
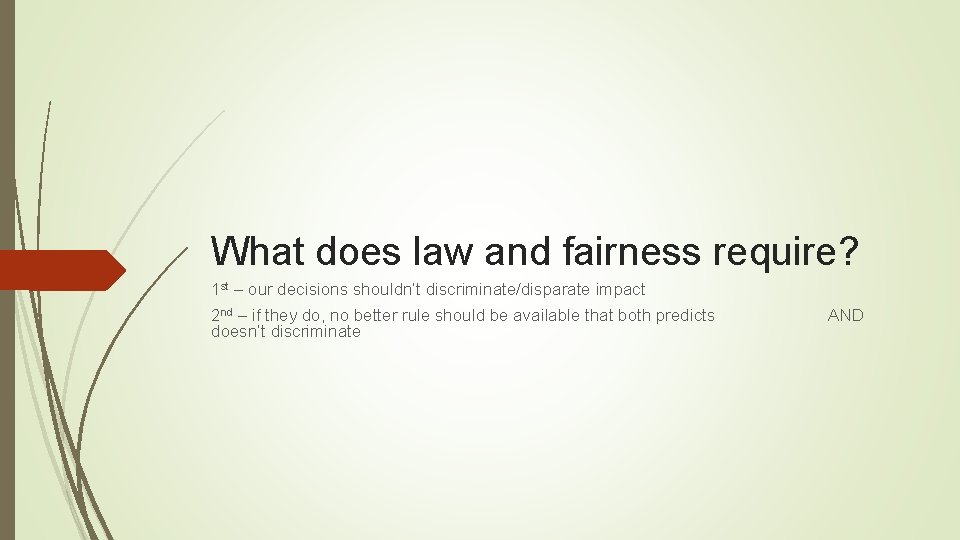
What does law and fairness require? 1 st – our decisions shouldn’t discriminate/disparate impact 2 nd – if they do, no better rule should be available that both predicts doesn’t discriminate AND
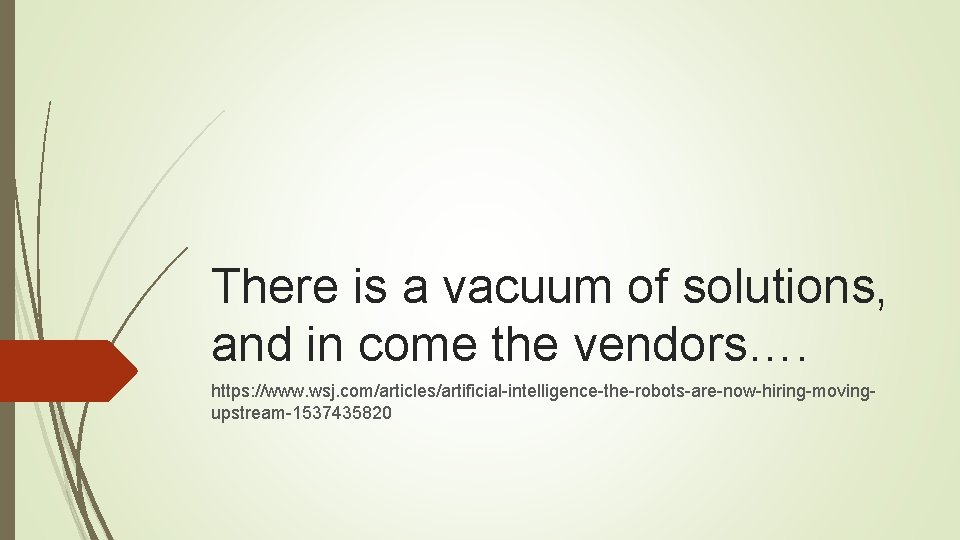
There is a vacuum of solutions, and in come the vendors…. https: //www. wsj. com/articles/artificial-intelligence-the-robots-are-now-hiring-movingupstream-1537435820
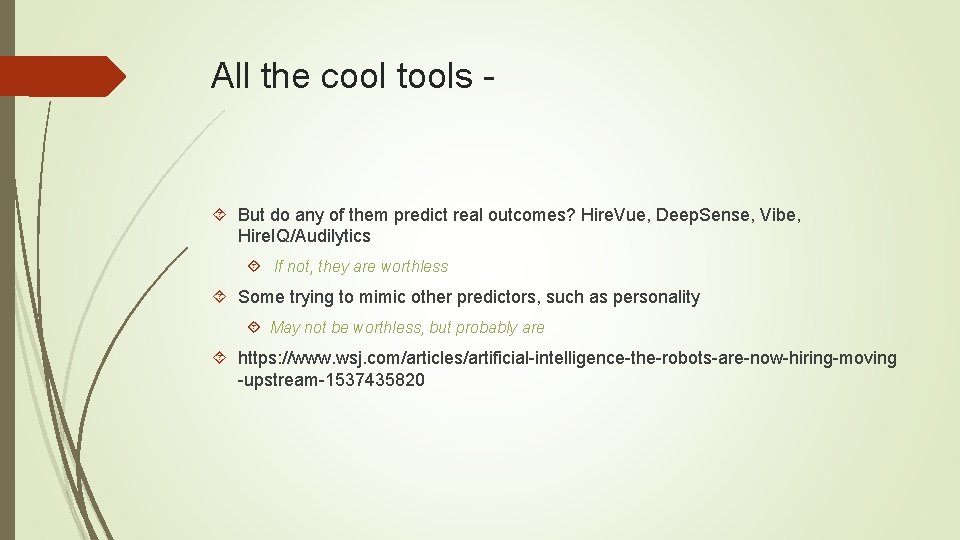
All the cool tools - But do any of them predict real outcomes? Hire. Vue, Deep. Sense, Vibe, Hire. IQ/Audilytics If not, they are worthless Some trying to mimic other predictors, such as personality May not be worthless, but probably are https: //www. wsj. com/articles/artificial-intelligence-the-robots-are-now-hiring-moving -upstream-1537435820
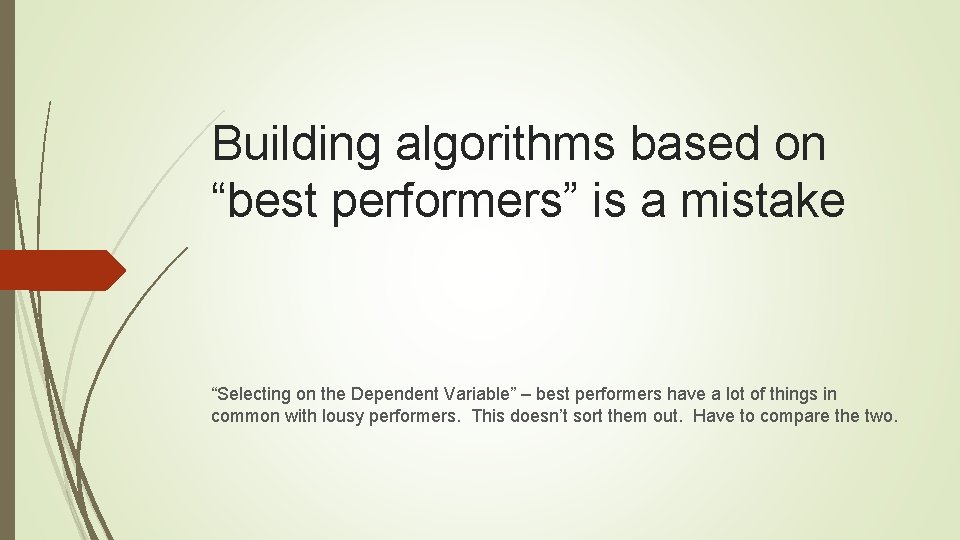
Building algorithms based on “best performers” is a mistake “Selecting on the Dependent Variable” – best performers have a lot of things in common with lousy performers. This doesn’t sort them out. Have to compare the two.
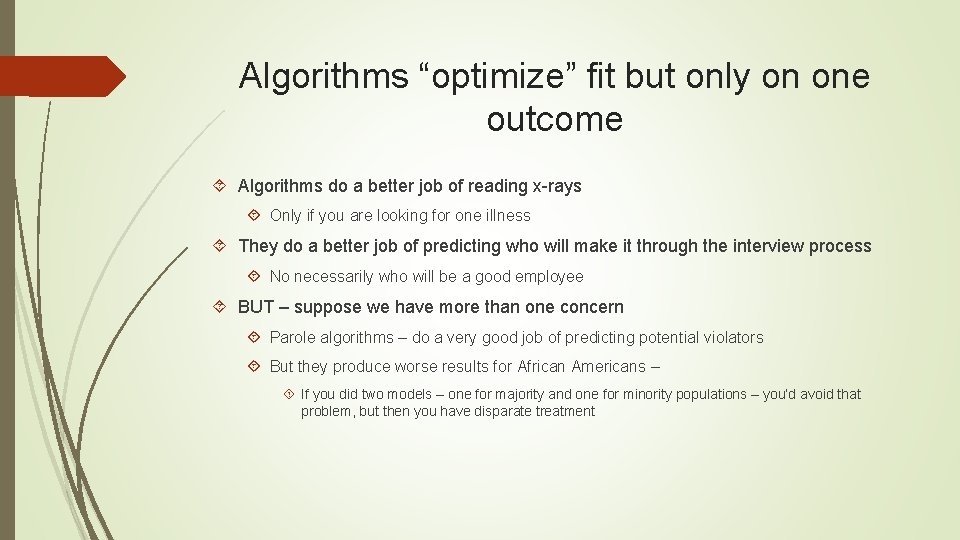
Algorithms “optimize” fit but only on one outcome Algorithms do a better job of reading x-rays Only if you are looking for one illness They do a better job of predicting who will make it through the interview process No necessarily who will be a good employee BUT – suppose we have more than one concern Parole algorithms – do a very good job of predicting potential violators But they produce worse results for African Americans – If you did two models – one for majority and one for minority populations – you’d avoid that problem, but then you have disparate treatment
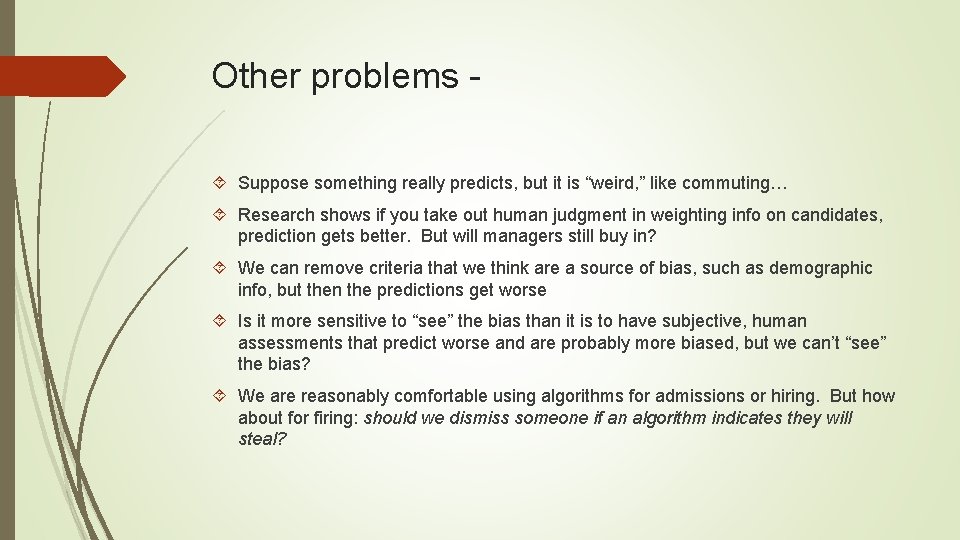
Other problems Suppose something really predicts, but it is “weird, ” like commuting… Research shows if you take out human judgment in weighting info on candidates, prediction gets better. But will managers still buy in? We can remove criteria that we think are a source of bias, such as demographic info, but then the predictions get worse Is it more sensitive to “see” the bias than it is to have subjective, human assessments that predict worse and are probably more biased, but we can’t “see” the bias? We are reasonably comfortable using algorithms for admissions or hiring. But how about for firing: should we dismiss someone if an algorithm indicates they will steal?
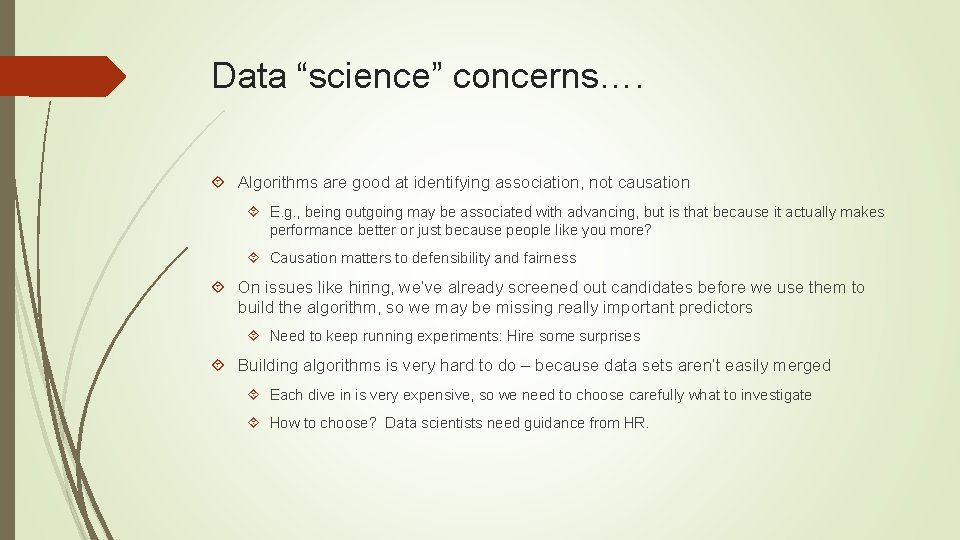
Data “science” concerns…. Algorithms are good at identifying association, not causation E. g. , being outgoing may be associated with advancing, but is that because it actually makes performance better or just because people like you more? Causation matters to defensibility and fairness On issues like hiring, we’ve already screened out candidates before we use them to build the algorithm, so we may be missing really important predictors Need to keep running experiments: Hire some surprises Building algorithms is very hard to do – because data sets aren’t easily merged Each dive in is very expensive, so we need to choose carefully what to investigate How to choose? Data scientists need guidance from HR.
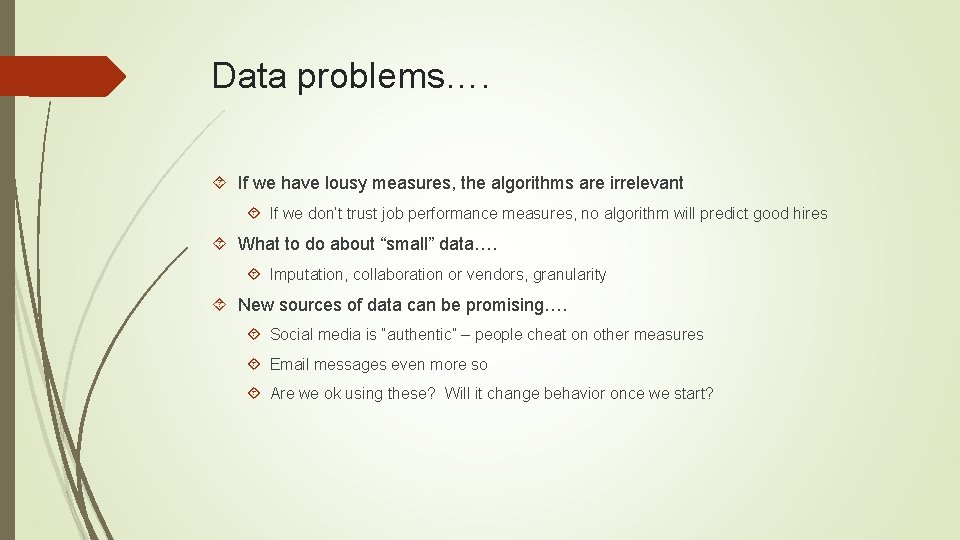
Data problems…. If we have lousy measures, the algorithms are irrelevant If we don’t trust job performance measures, no algorithm will predict good hires What to do about “small” data…. Imputation, collaboration or vendors, granularity New sources of data can be promising…. Social media is “authentic” – people cheat on other measures Email messages even more so Are we ok using these? Will it change behavior once we start?
Sei cappelli per pensare scuola primaria
Algorithmic graph theory and perfect graphs
Ib global contexts
Fairness and diversity in the workplace
"john locke institute"
Fairness and flawless ceo
George washington vs king george iii
George washington vs king george iii venn diagram
Algorithmic trading singapore
Mealy asm chart
Algorithmic thinking gcse
Algorithmic nuggets in content delivery
Algorithmic cost modelling
Notasi phi