A Customer Lifetime Value Framework for Customer Selection
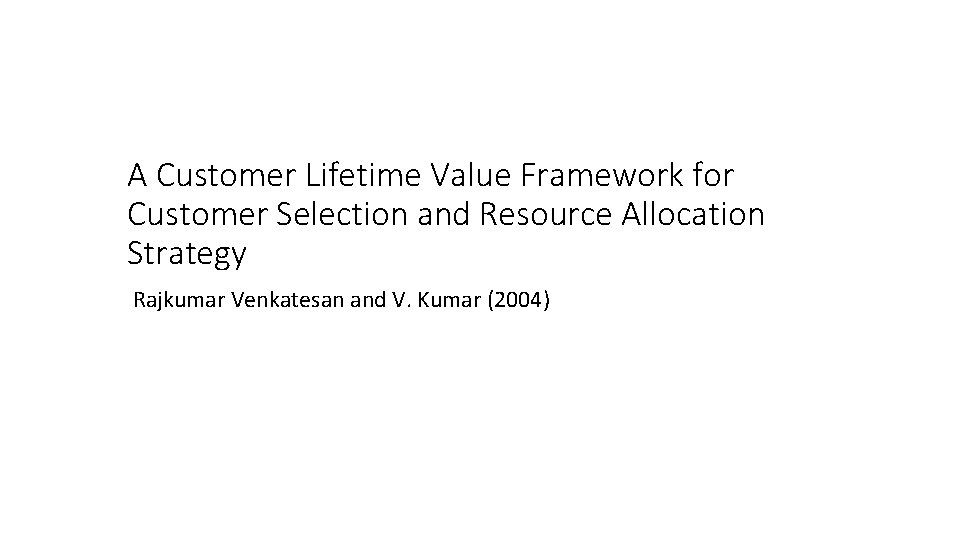
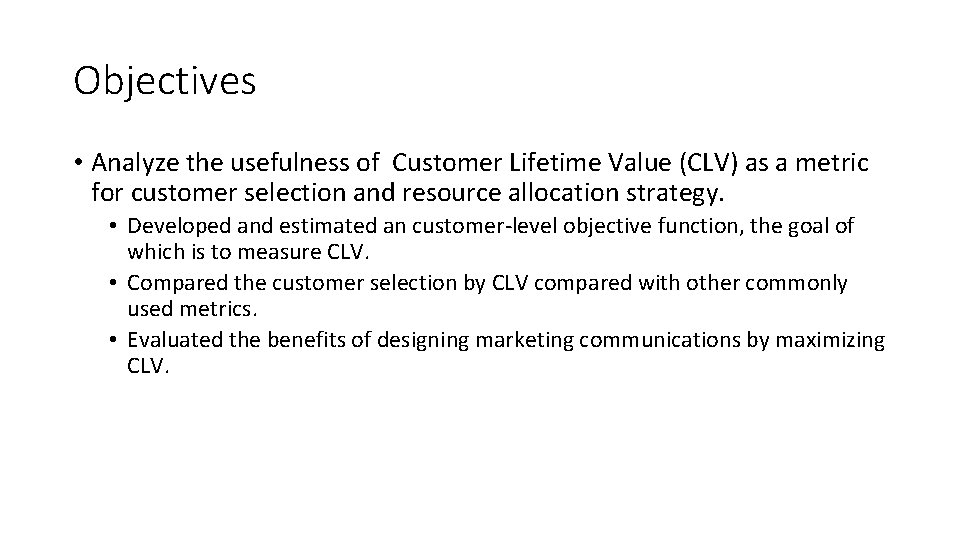
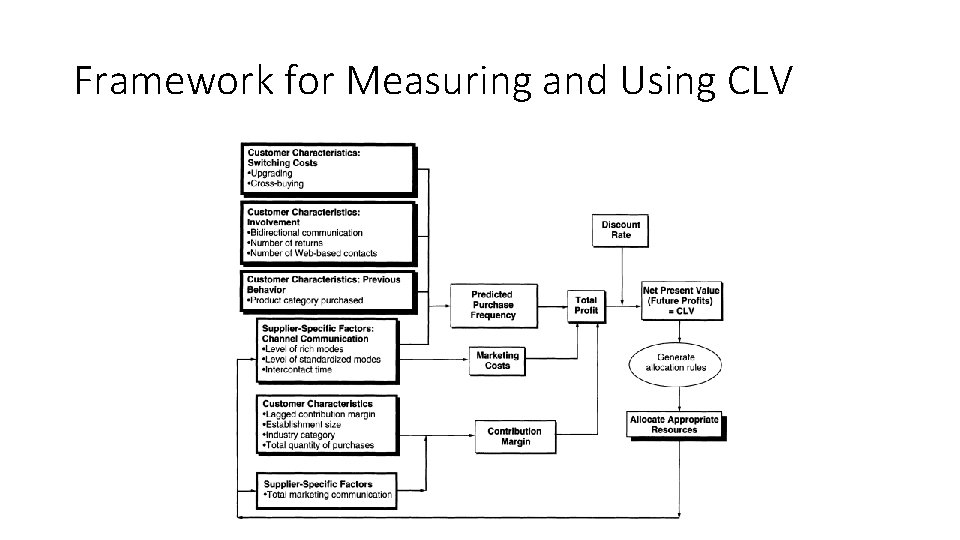
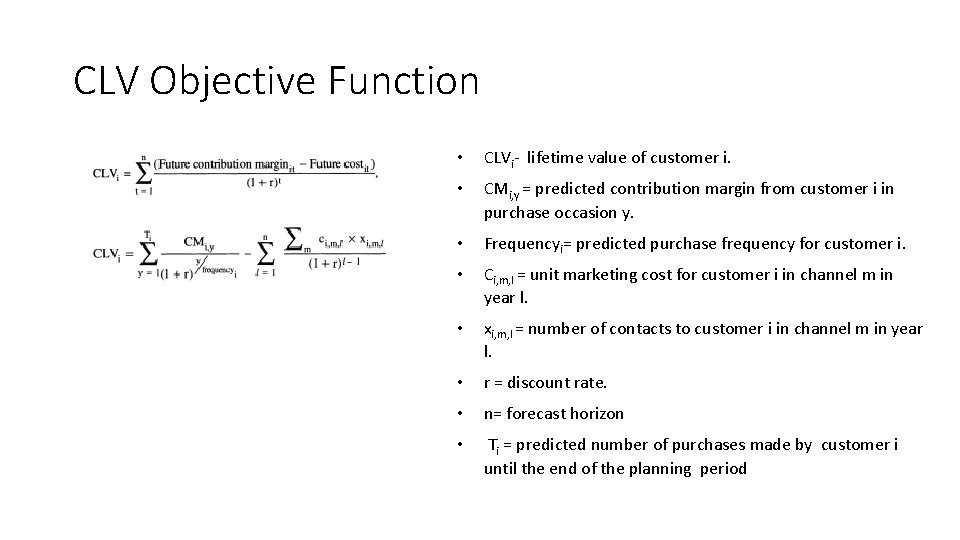
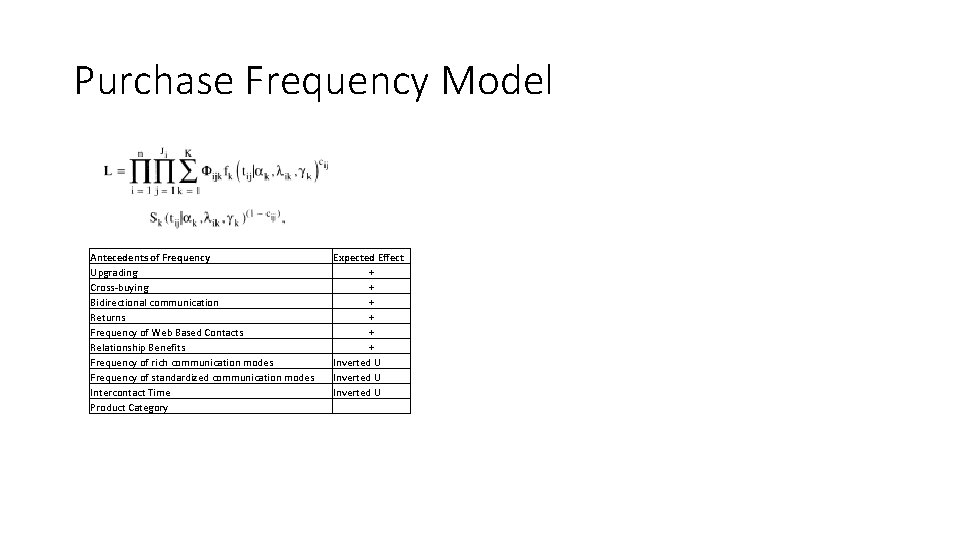
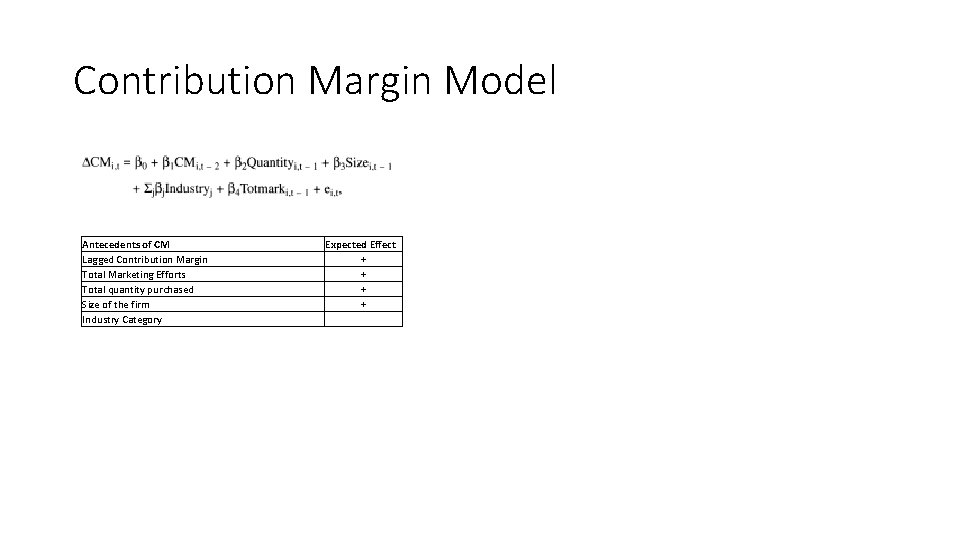
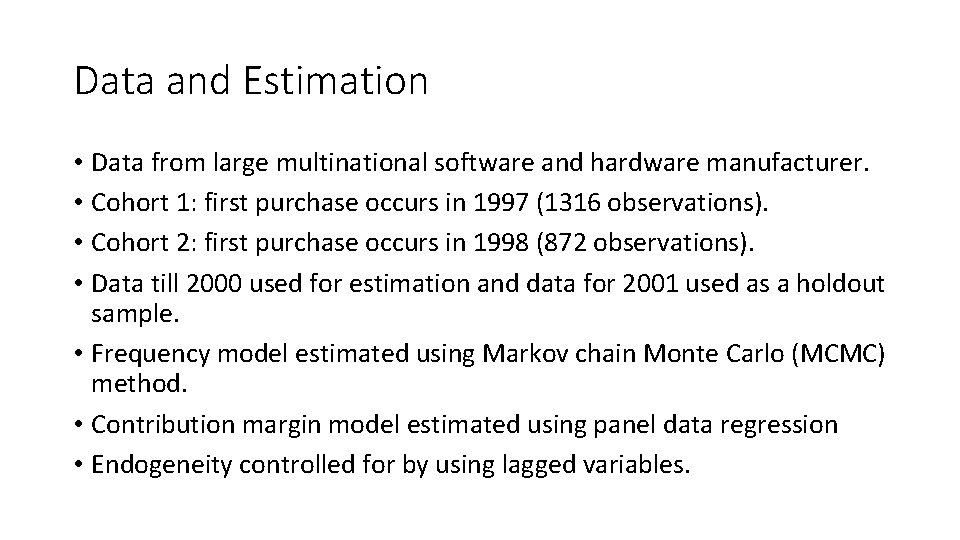
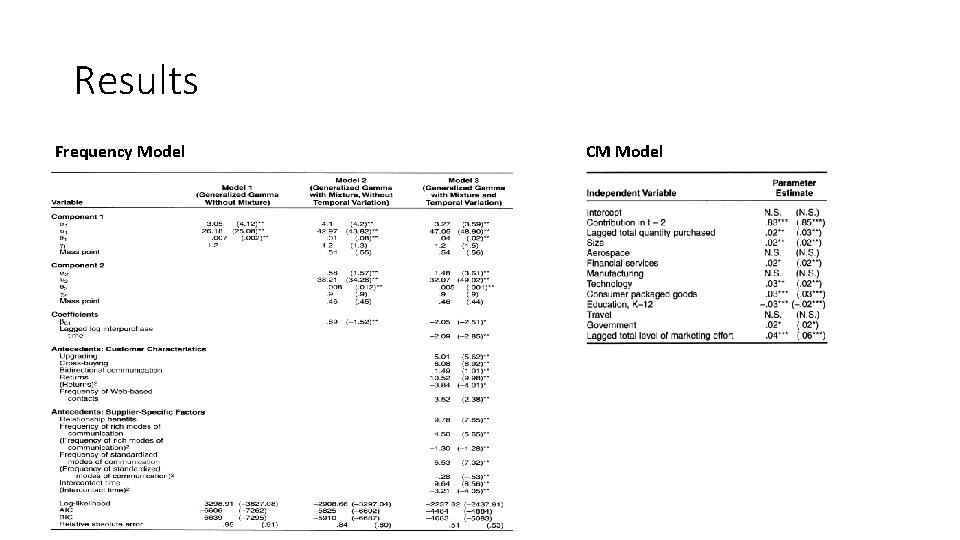
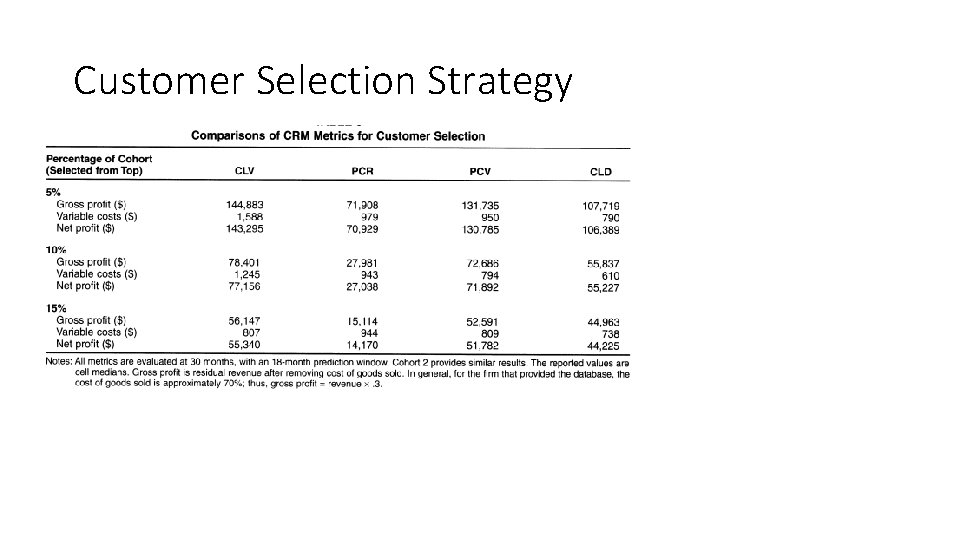
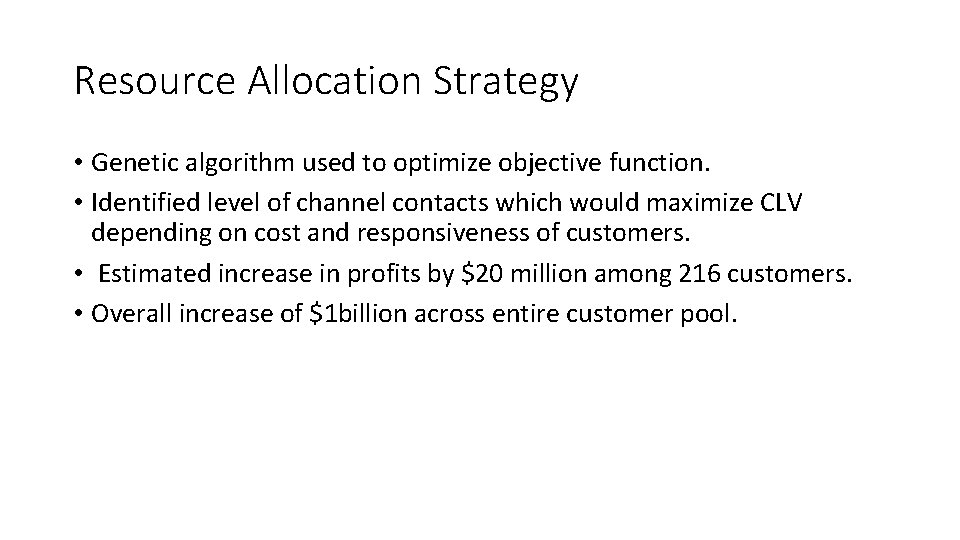
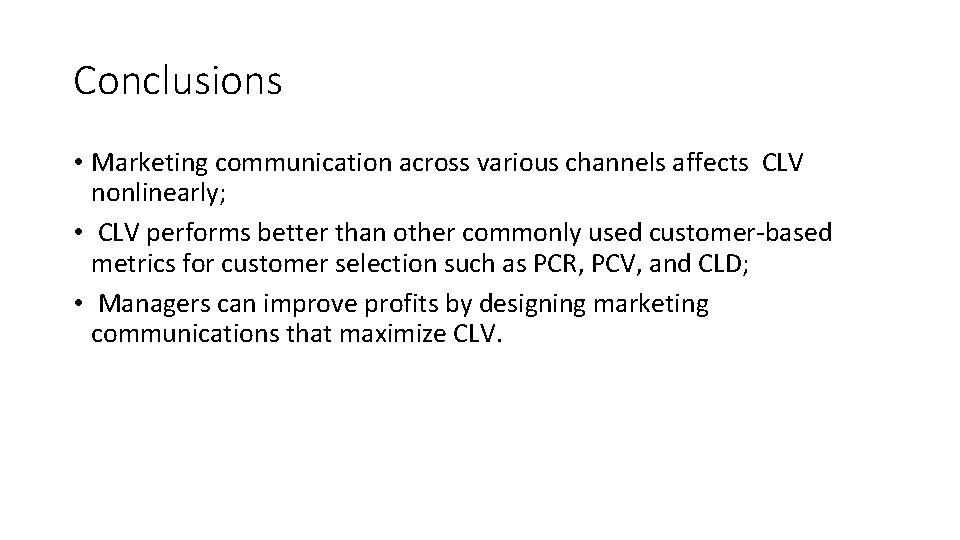
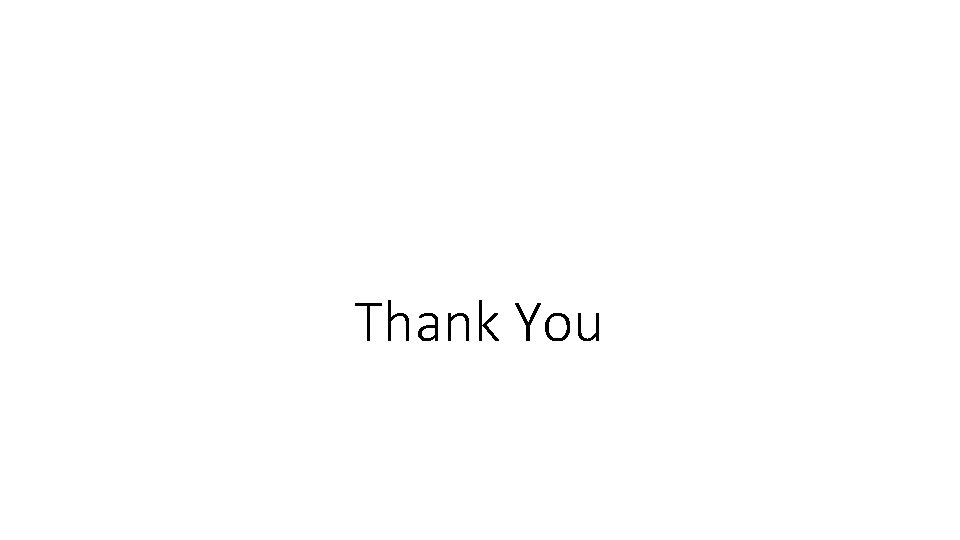
- Slides: 12
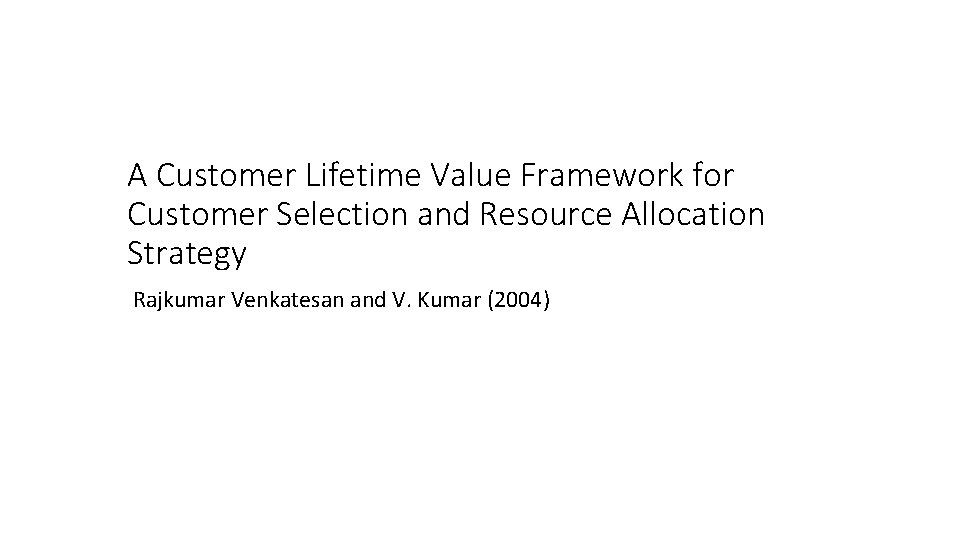
A Customer Lifetime Value Framework for Customer Selection and Resource Allocation Strategy Rajkumar Venkatesan and V. Kumar (2004)
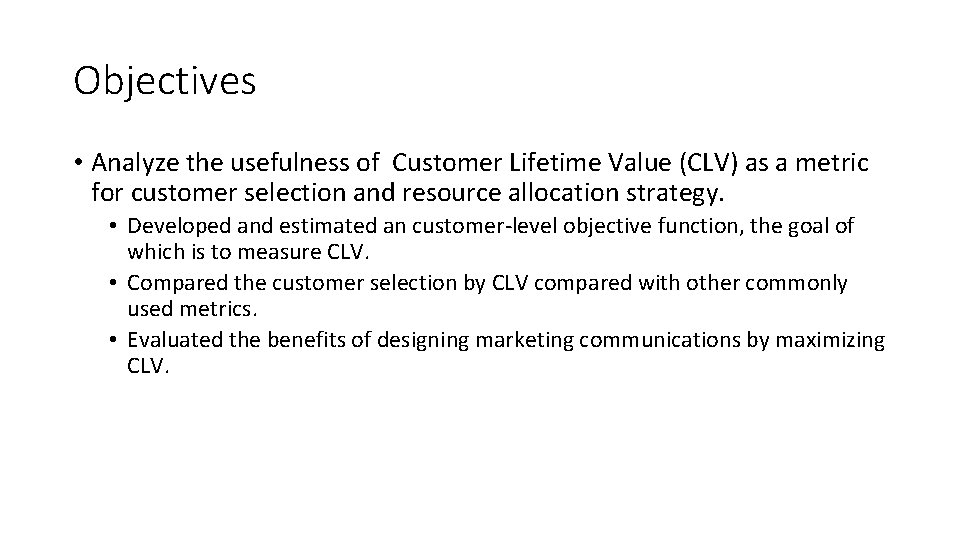
Objectives • Analyze the usefulness of Customer Lifetime Value (CLV) as a metric for customer selection and resource allocation strategy. • Developed and estimated an customer-level objective function, the goal of which is to measure CLV. • Compared the customer selection by CLV compared with other commonly used metrics. • Evaluated the benefits of designing marketing communications by maximizing CLV.
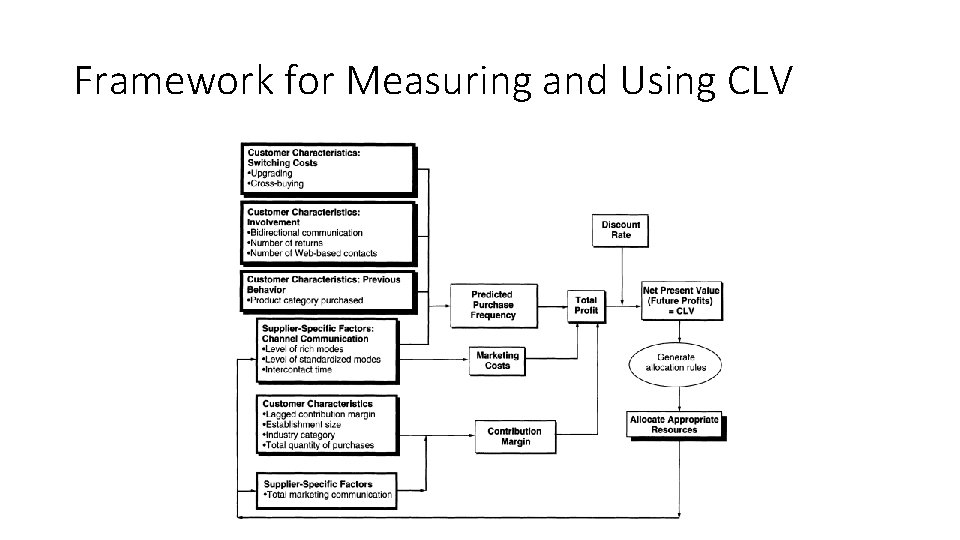
Framework for Measuring and Using CLV
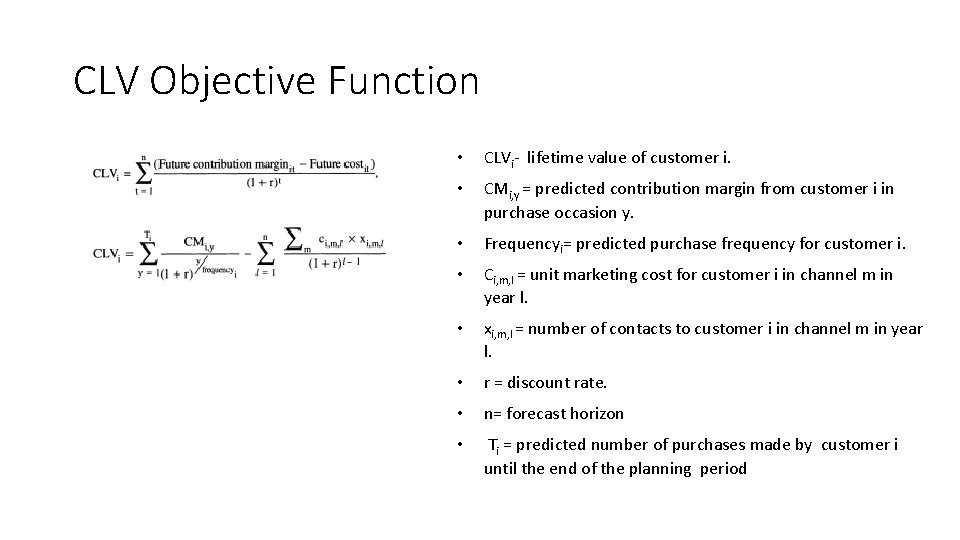
CLV Objective Function • CLVi- lifetime value of customer i. • CMi, y = predicted contribution margin from customer i in purchase occasion y. • Frequencyi= predicted purchase frequency for customer i. • Ci, m, l = unit marketing cost for customer i in channel m in year l. • xi, m, l = number of contacts to customer i in channel m in year l. • r = discount rate. • n= forecast horizon • Ti = predicted number of purchases made by customer i until the end of the planning period
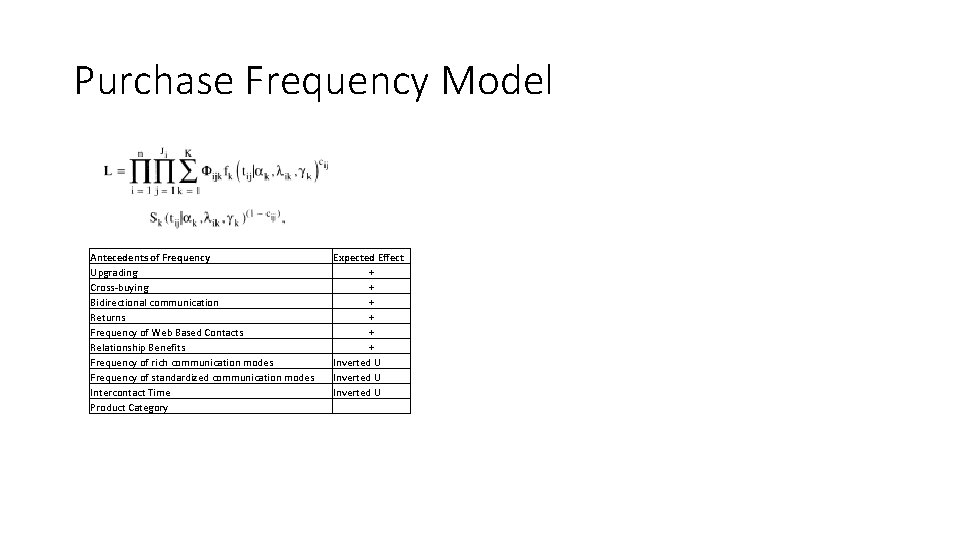
Purchase Frequency Model Antecedents of Frequency Upgrading Cross-buying Bidirectional communication Returns Frequency of Web Based Contacts Relationship Benefits Frequency of rich communication modes Frequency of standardized communication modes Intercontact Time Product Category Expected Effect + + + Inverted U
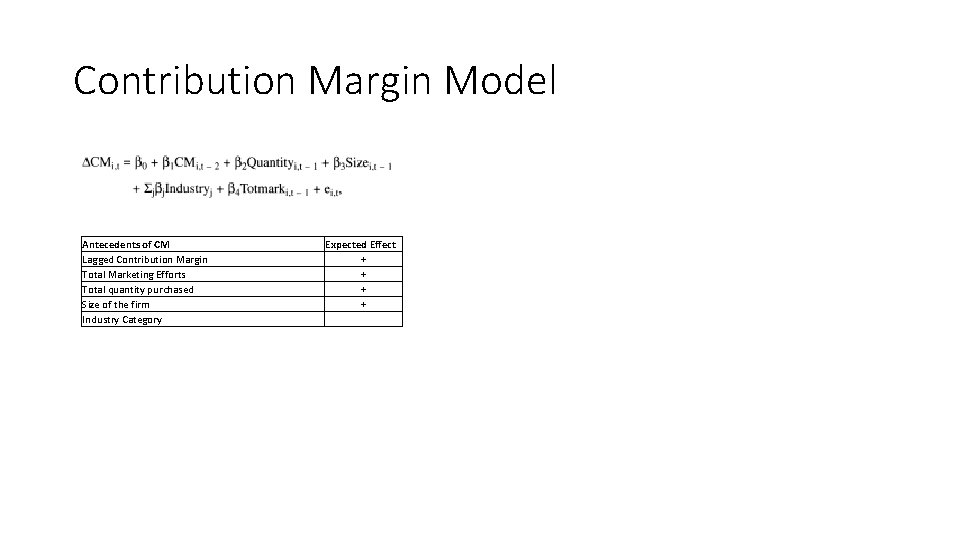
Contribution Margin Model Antecedents of CM Lagged Contribution Margin Total Marketing Efforts Total quantity purchased Size of the firm Industry Category Expected Effect + +
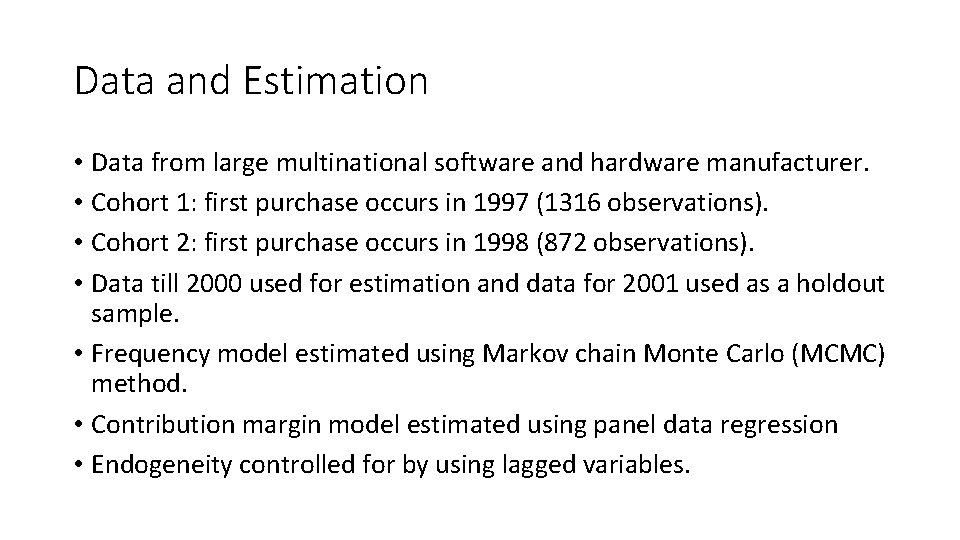
Data and Estimation • Data from large multinational software and hardware manufacturer. • Cohort 1: first purchase occurs in 1997 (1316 observations). • Cohort 2: first purchase occurs in 1998 (872 observations). • Data till 2000 used for estimation and data for 2001 used as a holdout sample. • Frequency model estimated using Markov chain Monte Carlo (MCMC) method. • Contribution margin model estimated using panel data regression • Endogeneity controlled for by using lagged variables.
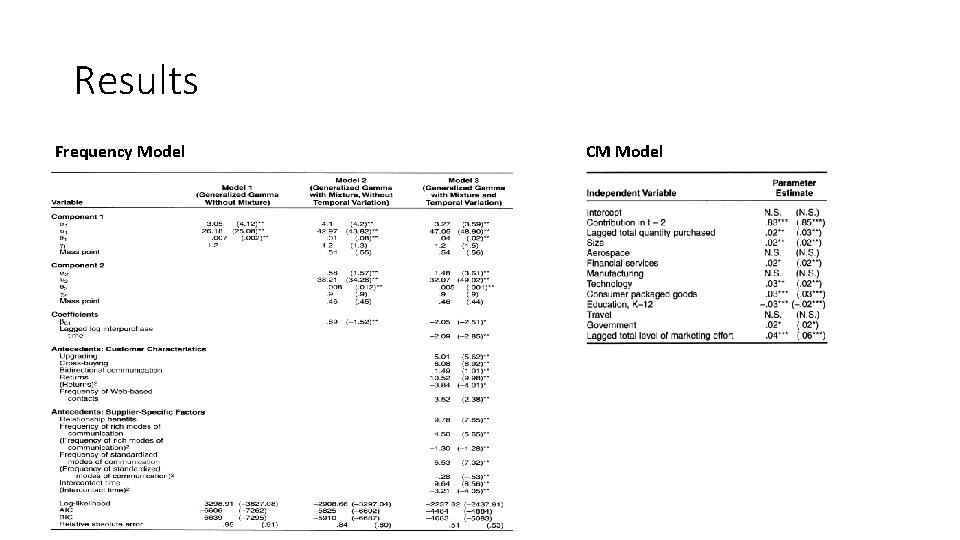
Results Frequency Model CM Model
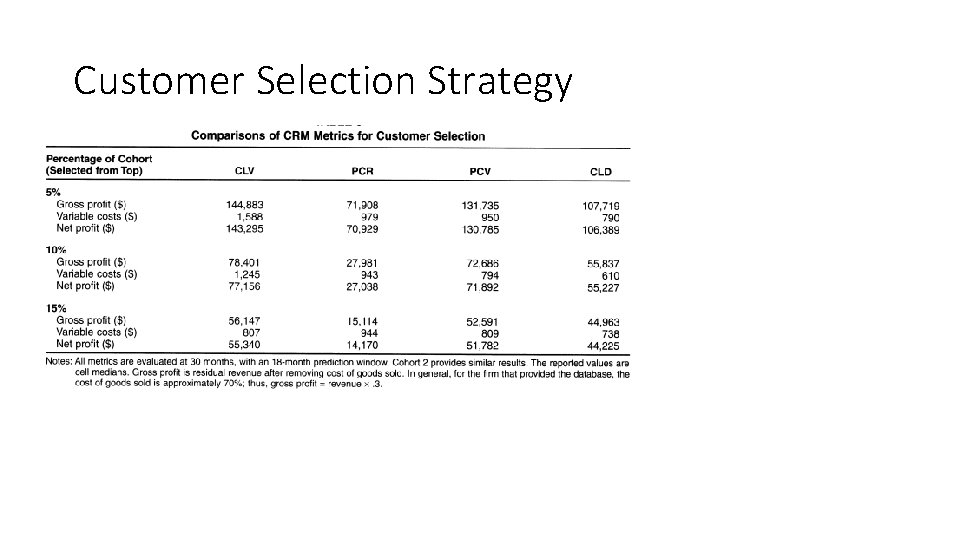
Customer Selection Strategy
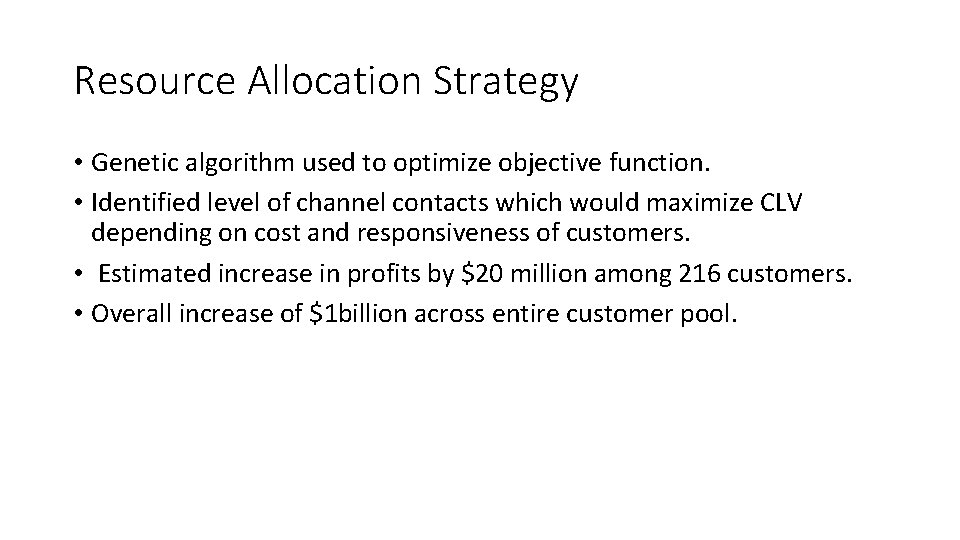
Resource Allocation Strategy • Genetic algorithm used to optimize objective function. • Identified level of channel contacts which would maximize CLV depending on cost and responsiveness of customers. • Estimated increase in profits by $20 million among 216 customers. • Overall increase of $1 billion across entire customer pool.
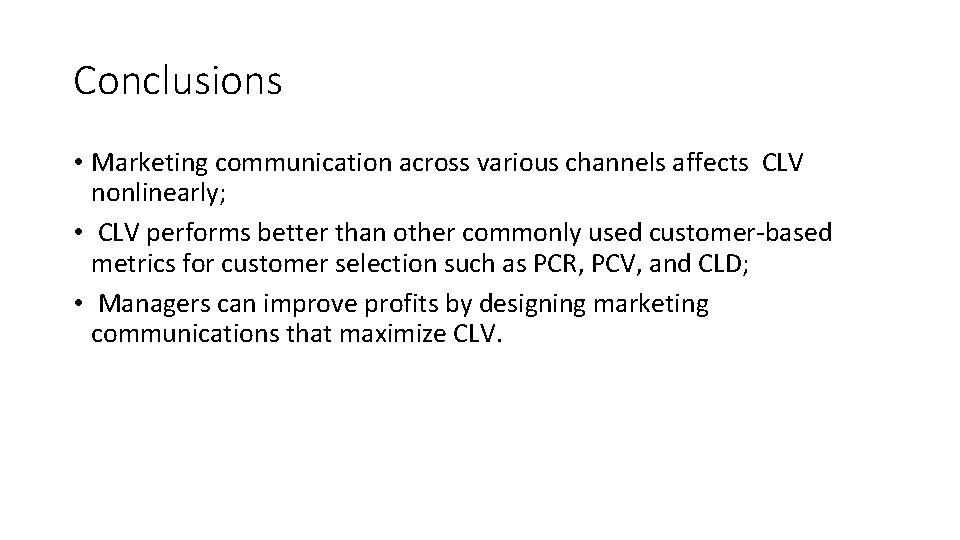
Conclusions • Marketing communication across various channels affects CLV nonlinearly; • CLV performs better than other commonly used customer-based metrics for customer selection such as PCR, PCV, and CLD; • Managers can improve profits by designing marketing communications that maximize CLV.
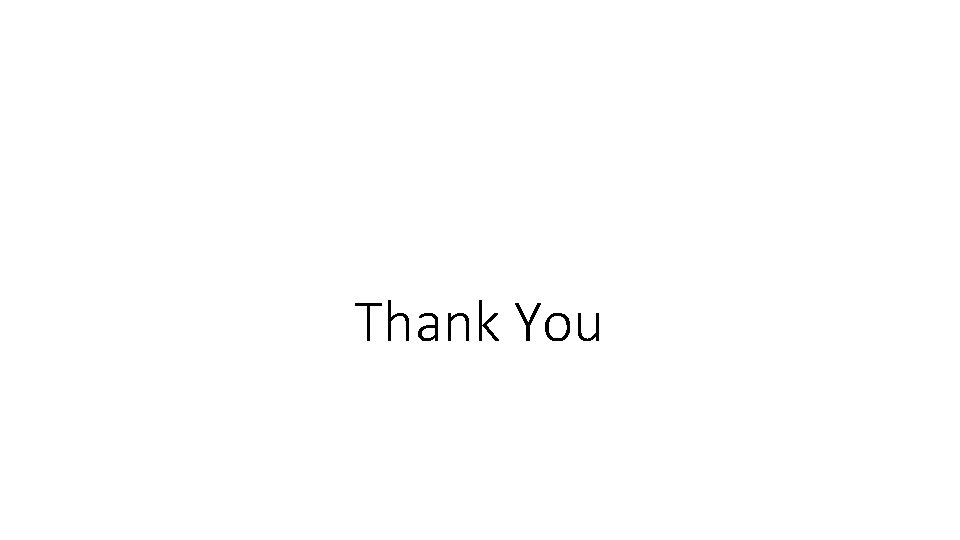
Thank You
Starbucks clv
Lifetime customer profitability analysis
Contoh value creation adalah
Balancing selection vs stabilizing selection
Similarities
K selection r selection
Natural selection vs artificial selection
Difference between continuous and discontinuous variation
5 points of natural selection
Clumped dispersion
Natural selection vs artificial selection
Two way selection and multiway selection in c
Multiway selection in c