WWC Standards Evaluating SingleCase Design Evidence With Visual
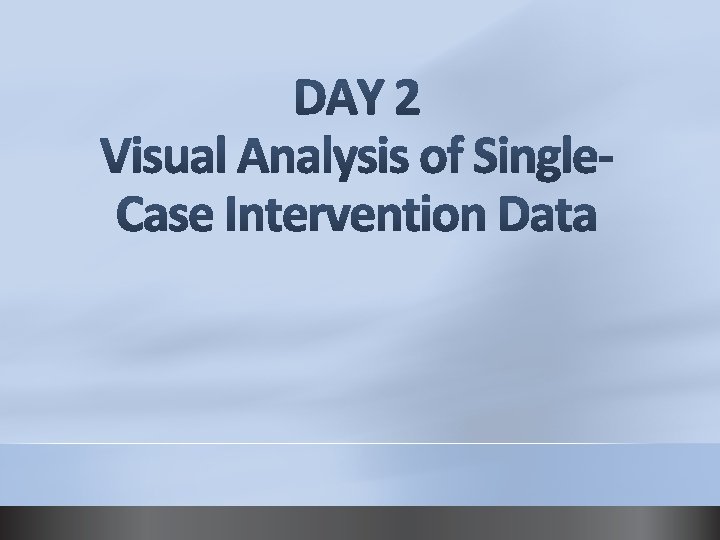
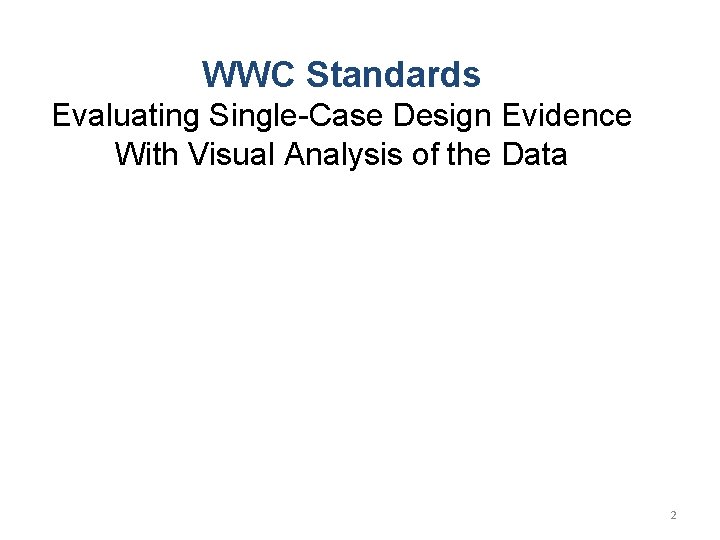
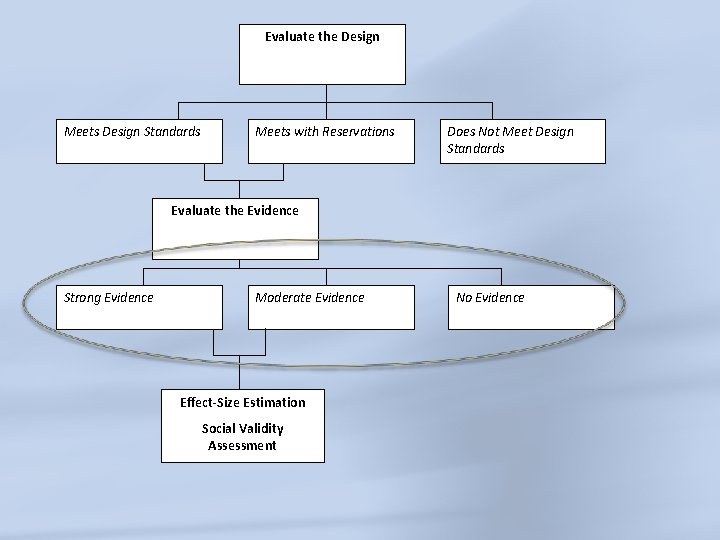
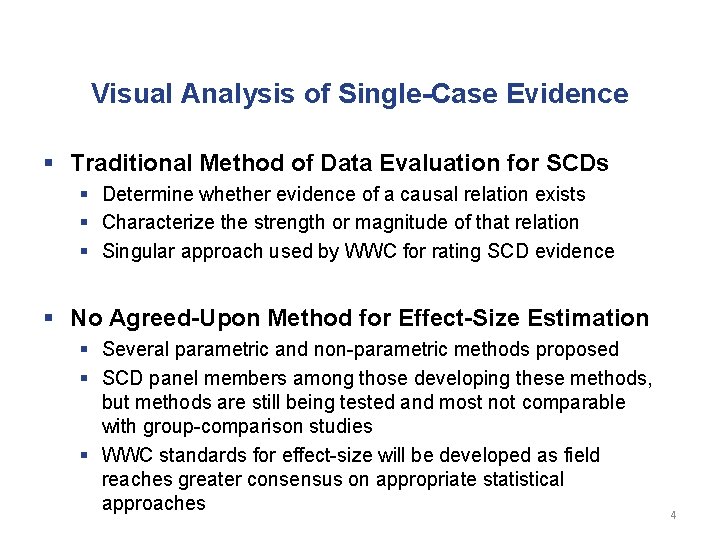
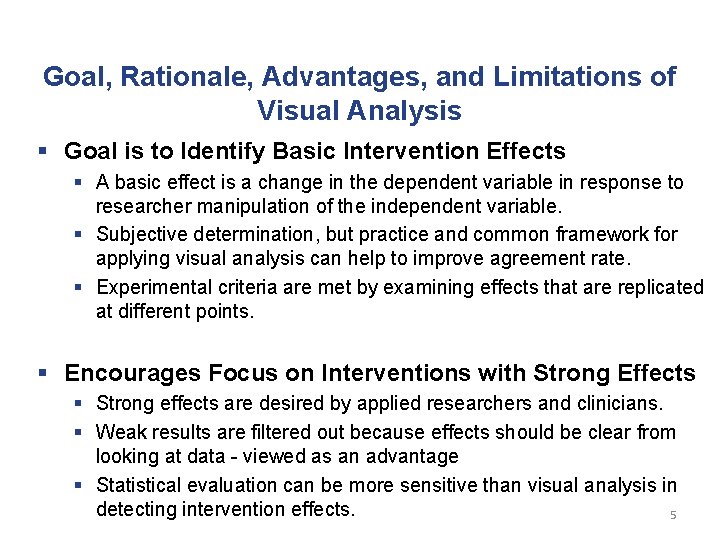
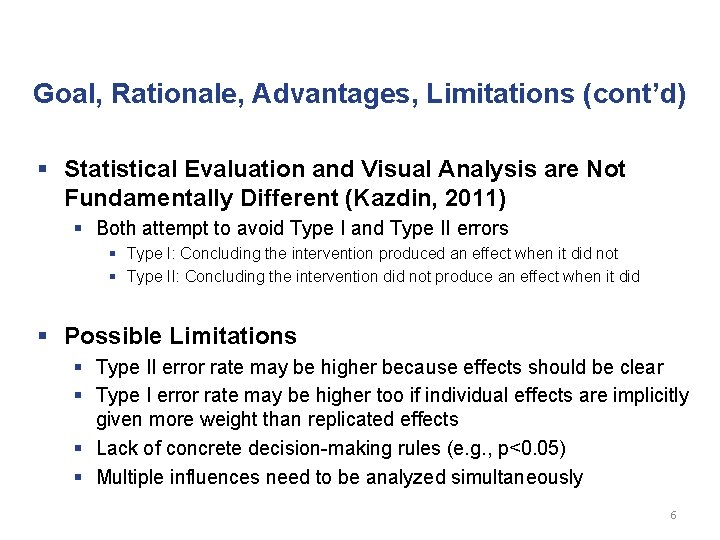
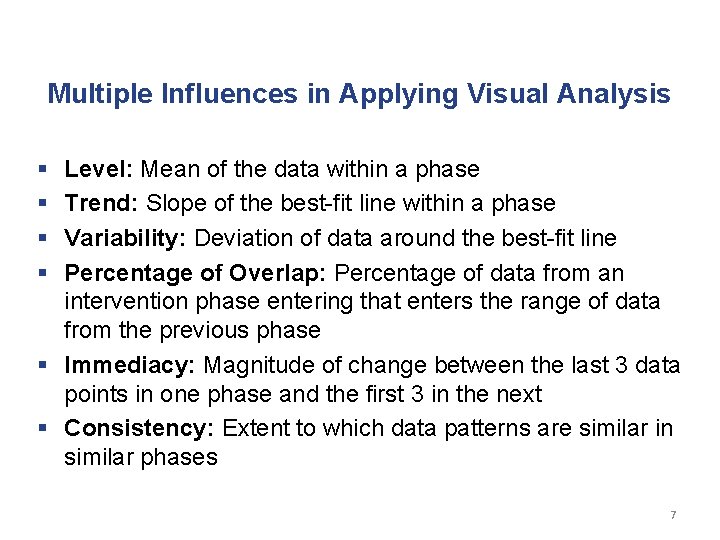
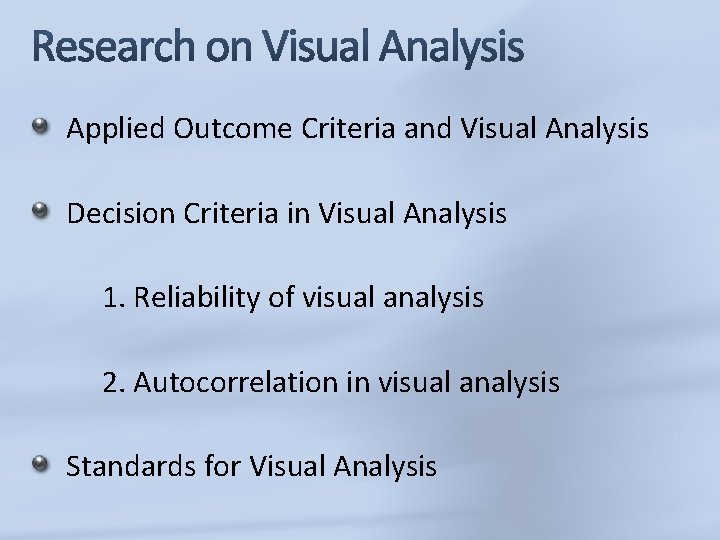
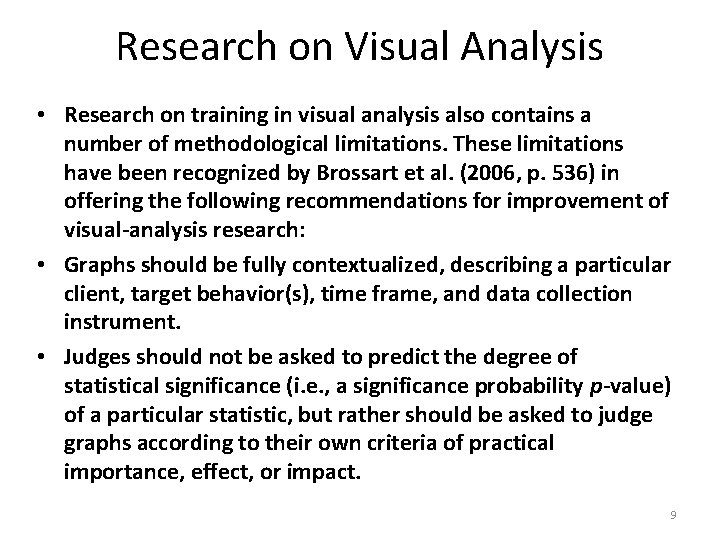
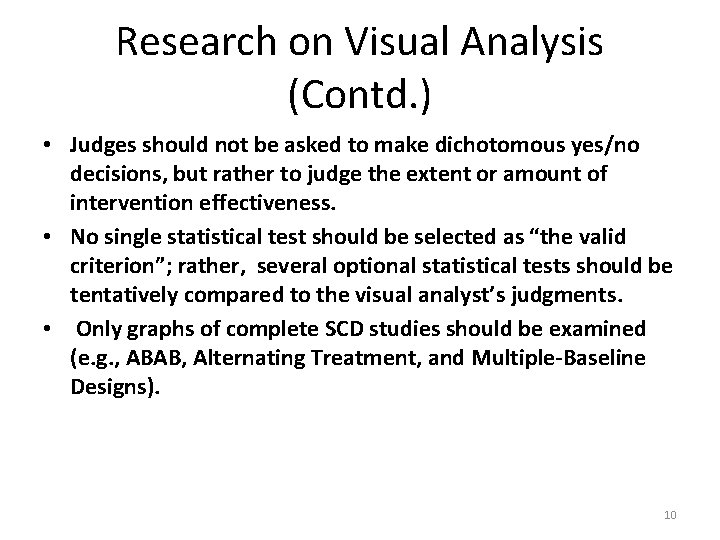
- Slides: 10
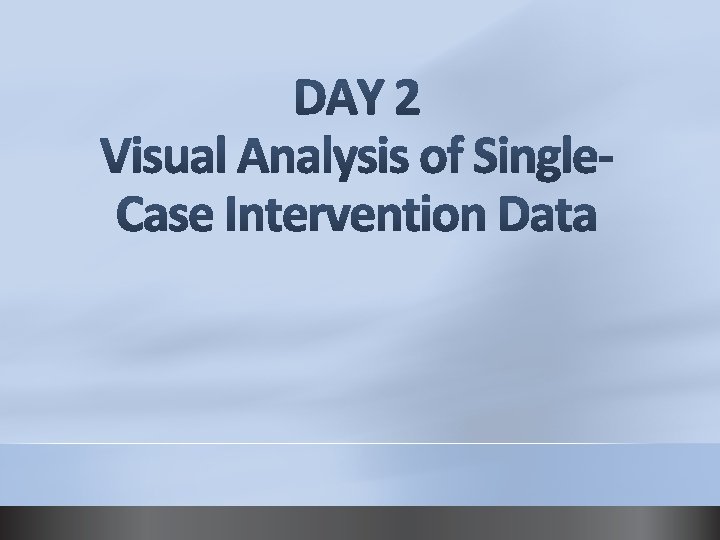
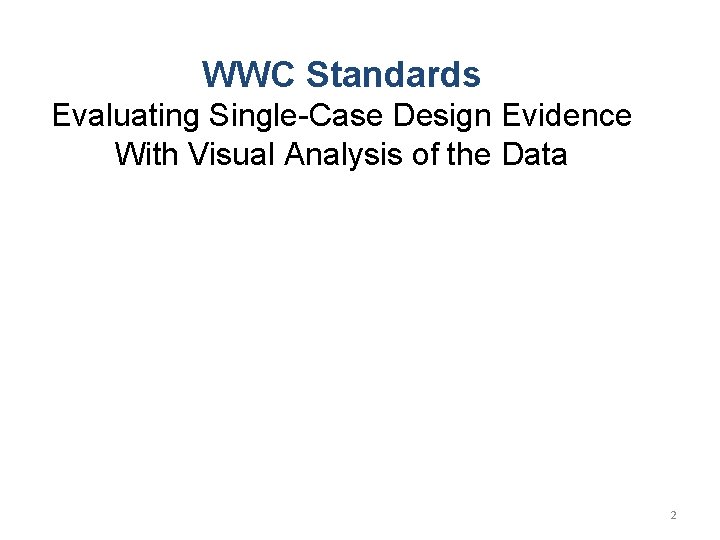
WWC Standards Evaluating Single-Case Design Evidence With Visual Analysis of the Data 2
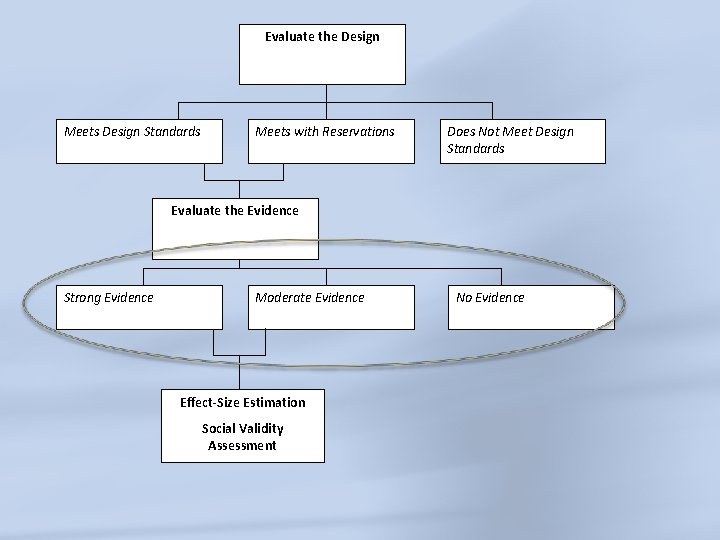
Evaluate the Design Meets Design Standards Meets with Reservations Does Not Meet Design Standards Evaluate the Evidence Strong Evidence Moderate Evidence Effect-Size Estimation Social Validity Assessment No Evidence
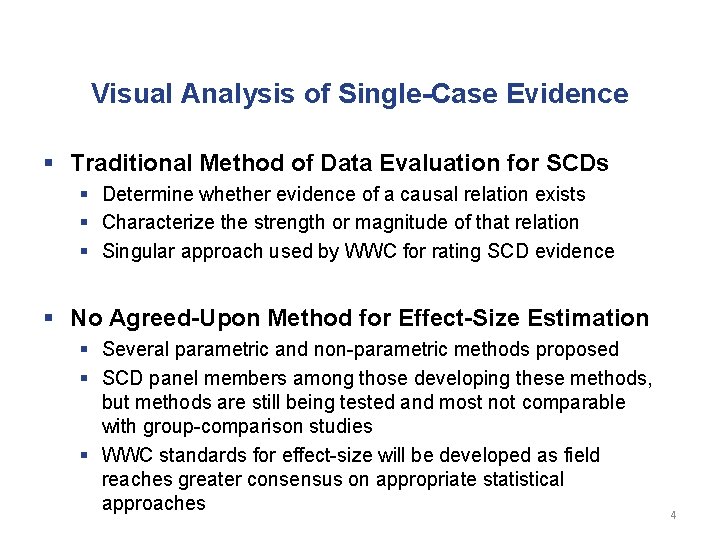
Visual Analysis of Single-Case Evidence § Traditional Method of Data Evaluation for SCDs § Determine whether evidence of a causal relation exists § Characterize the strength or magnitude of that relation § Singular approach used by WWC for rating SCD evidence § No Agreed-Upon Method for Effect-Size Estimation § Several parametric and non-parametric methods proposed § SCD panel members among those developing these methods, but methods are still being tested and most not comparable with group-comparison studies § WWC standards for effect-size will be developed as field reaches greater consensus on appropriate statistical approaches 4
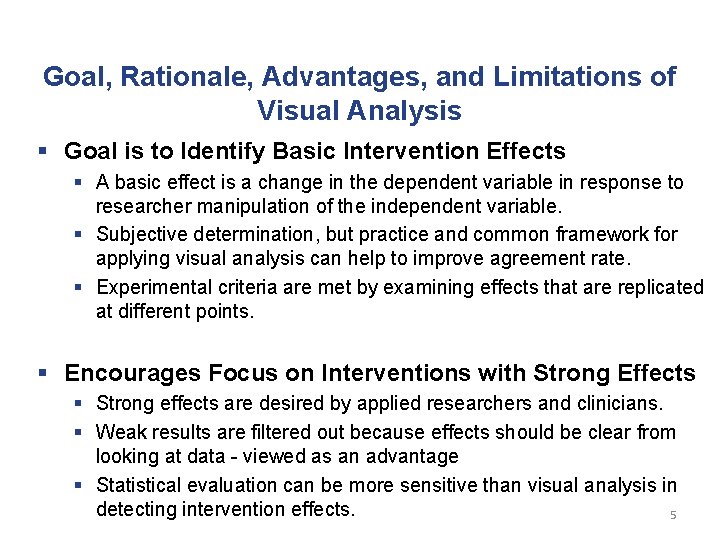
Goal, Rationale, Advantages, and Limitations of Visual Analysis § Goal is to Identify Basic Intervention Effects § A basic effect is a change in the dependent variable in response to researcher manipulation of the independent variable. § Subjective determination, but practice and common framework for applying visual analysis can help to improve agreement rate. § Experimental criteria are met by examining effects that are replicated at different points. § Encourages Focus on Interventions with Strong Effects § Strong effects are desired by applied researchers and clinicians. § Weak results are filtered out because effects should be clear from looking at data - viewed as an advantage § Statistical evaluation can be more sensitive than visual analysis in detecting intervention effects. 5
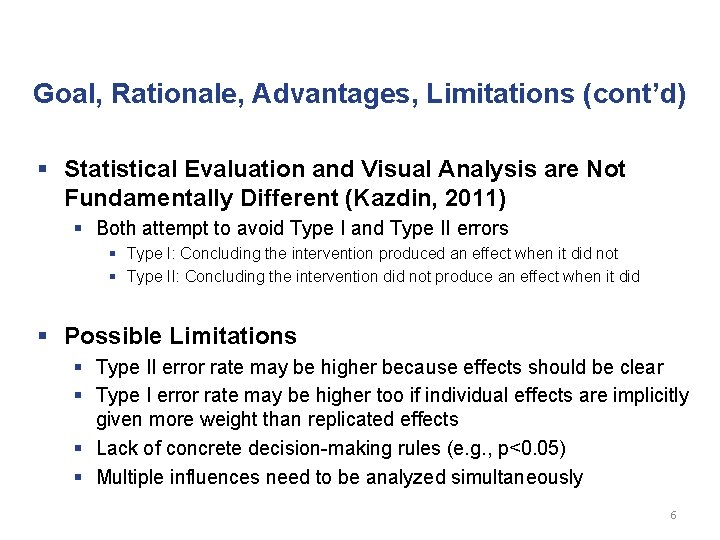
Goal, Rationale, Advantages, Limitations (cont’d) § Statistical Evaluation and Visual Analysis are Not Fundamentally Different (Kazdin, 2011) § Both attempt to avoid Type I and Type II errors § Type I: Concluding the intervention produced an effect when it did not § Type II: Concluding the intervention did not produce an effect when it did § Possible Limitations § Type II error rate may be higher because effects should be clear § Type I error rate may be higher too if individual effects are implicitly given more weight than replicated effects § Lack of concrete decision-making rules (e. g. , p<0. 05) § Multiple influences need to be analyzed simultaneously 6
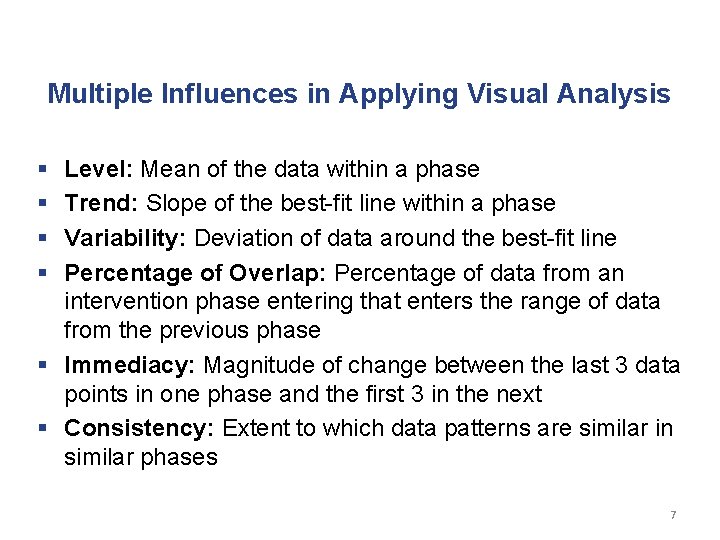
Multiple Influences in Applying Visual Analysis § § Level: Mean of the data within a phase Trend: Slope of the best-fit line within a phase Variability: Deviation of data around the best-fit line Percentage of Overlap: Percentage of data from an intervention phase entering that enters the range of data from the previous phase § Immediacy: Magnitude of change between the last 3 data points in one phase and the first 3 in the next § Consistency: Extent to which data patterns are similar in similar phases 7
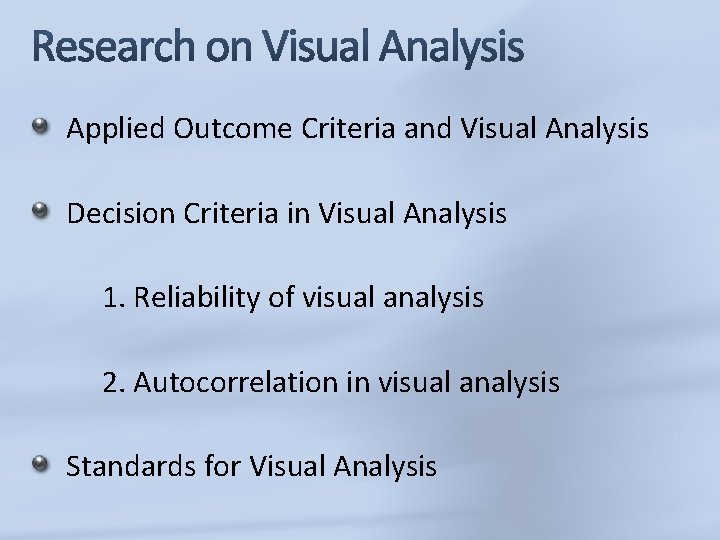
Applied Outcome Criteria and Visual Analysis Decision Criteria in Visual Analysis 1. Reliability of visual analysis 2. Autocorrelation in visual analysis Standards for Visual Analysis
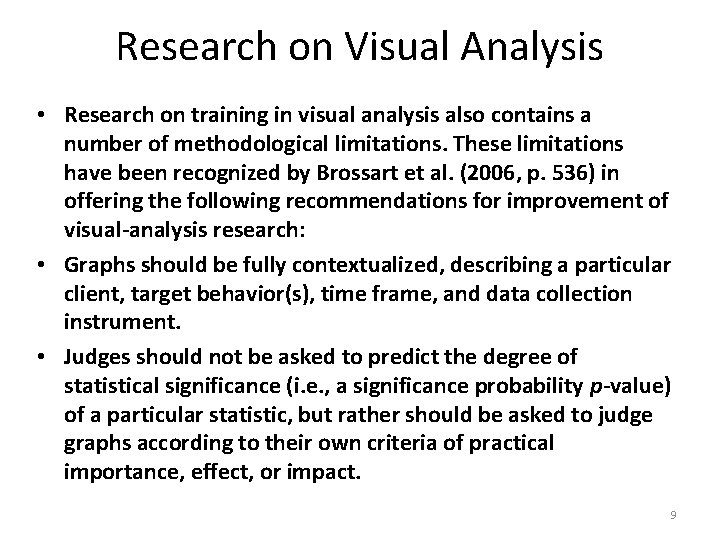
Research on Visual Analysis • Research on training in visual analysis also contains a number of methodological limitations. These limitations have been recognized by Brossart et al. (2006, p. 536) in offering the following recommendations for improvement of visual-analysis research: • Graphs should be fully contextualized, describing a particular client, target behavior(s), time frame, and data collection instrument. • Judges should not be asked to predict the degree of statistical significance (i. e. , a significance probability p-value) of a particular statistic, but rather should be asked to judge graphs according to their own criteria of practical importance, effect, or impact. 9
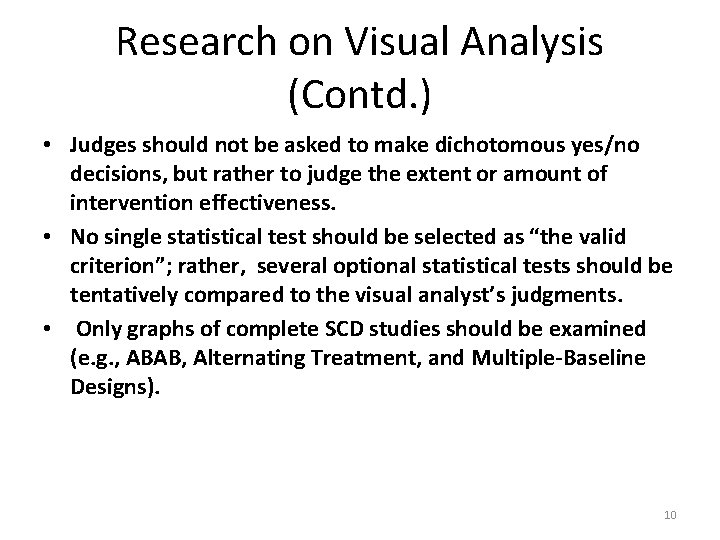
Research on Visual Analysis (Contd. ) • Judges should not be asked to make dichotomous yes/no decisions, but rather to judge the extent or amount of intervention effectiveness. • No single statistical test should be selected as “the valid criterion”; rather, several optional statistical tests should be tentatively compared to the visual analyst’s judgments. • Only graphs of complete SCD studies should be examined (e. g. , ABAB, Alternating Treatment, and Multiple-Baseline Designs). 10
Wwc practice guides
Secondary sources
Primary evidence vs secondary evidence
Secondary sources
Primary evidence vs secondary evidence
Primary evidence vs secondary evidence
Why are fibers class evidence
Class evidence vs individual evidence
Explain how class evidence may be useful.
Class and individual evidence
The absence of evidence is not the evidence of absence