Towards a Translation Assessment Assistant Tom Cheesman Assessment
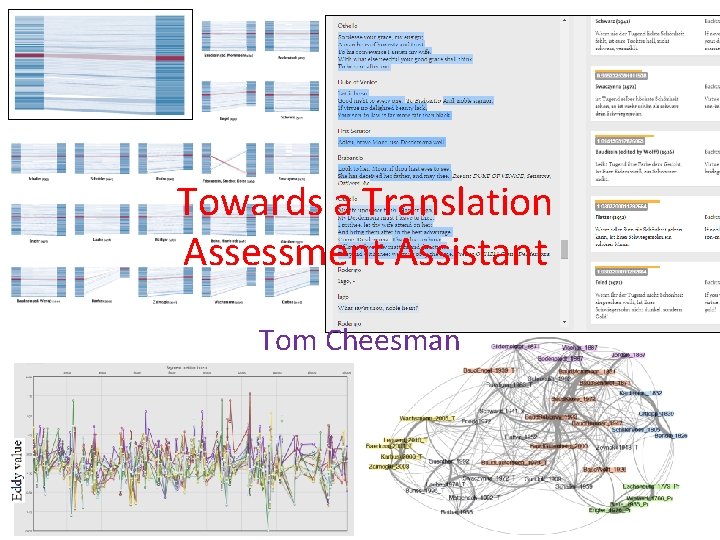
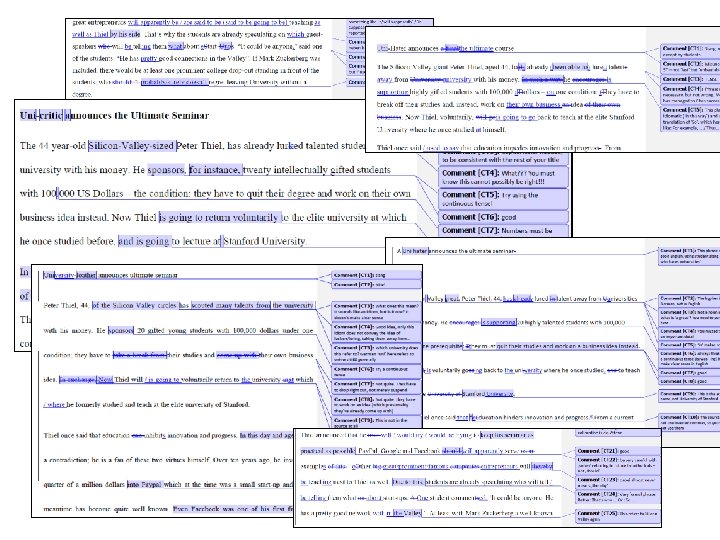
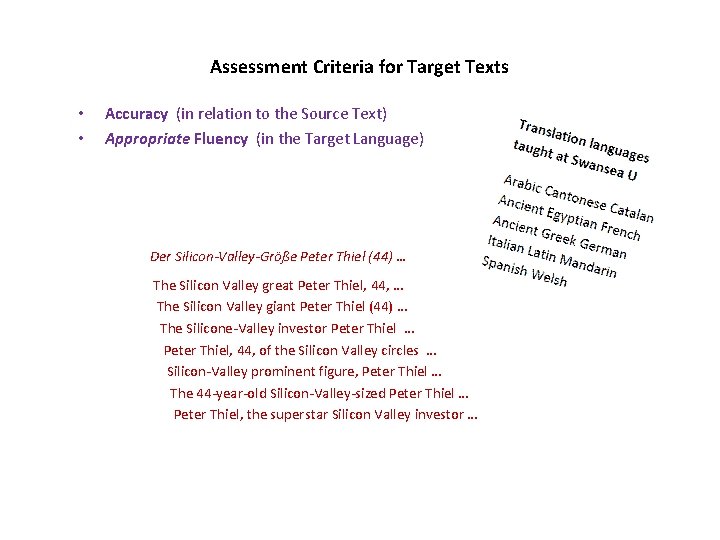
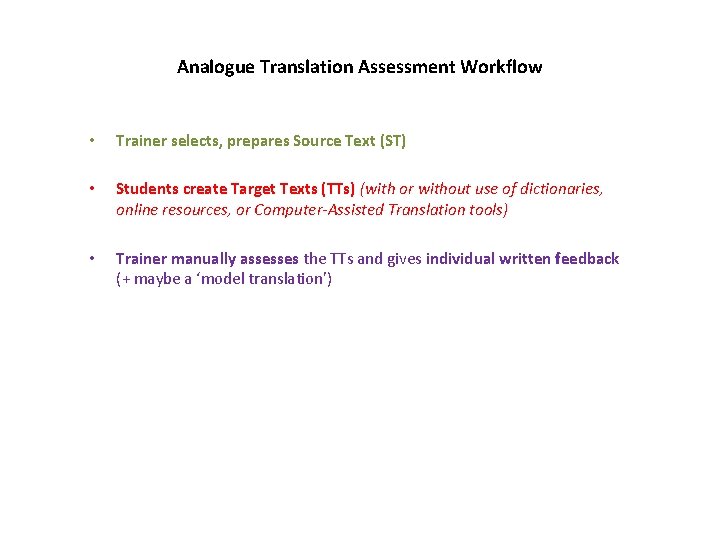
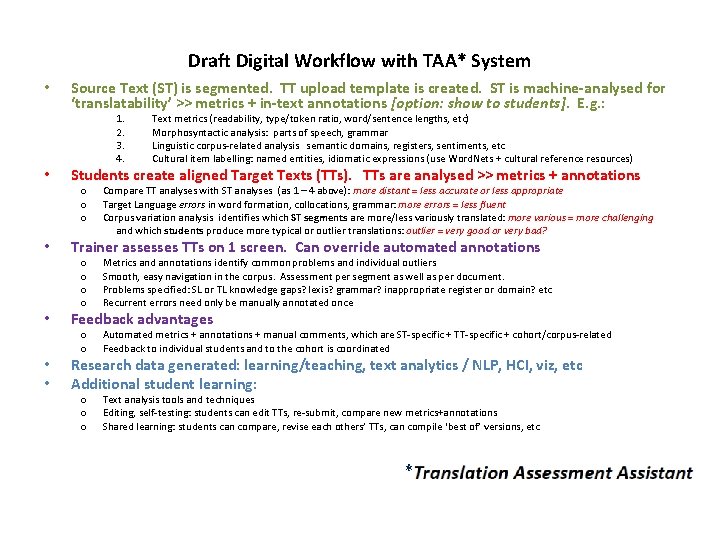
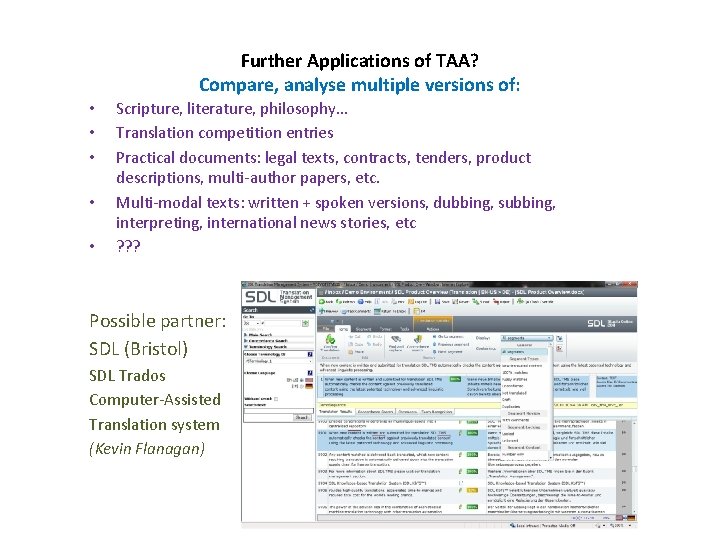
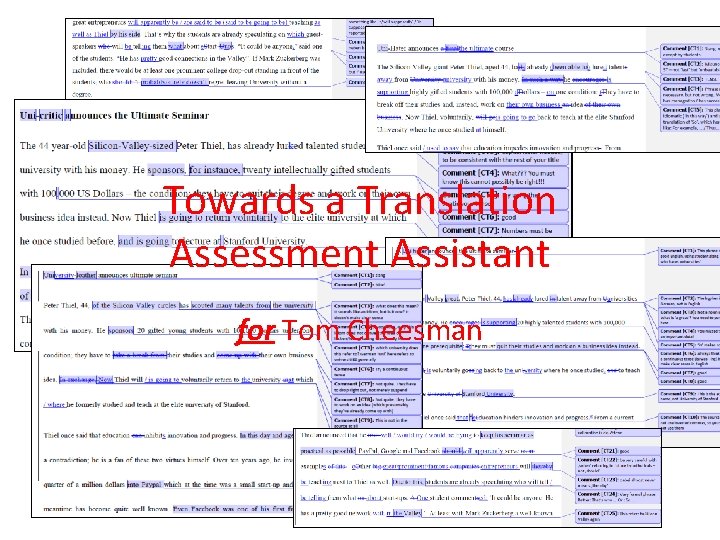
- Slides: 7
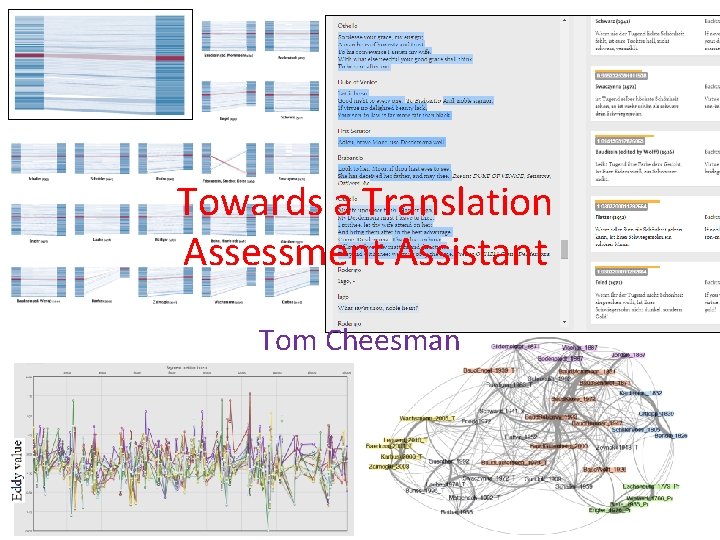
Towards a Translation Assessment Assistant Tom Cheesman
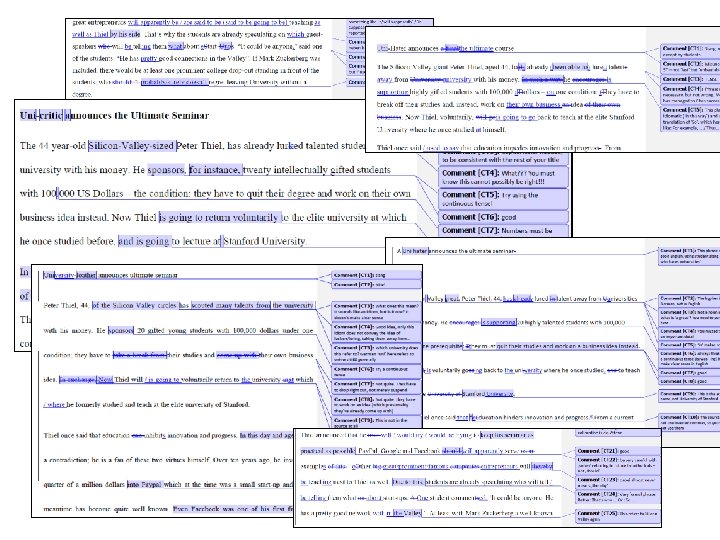
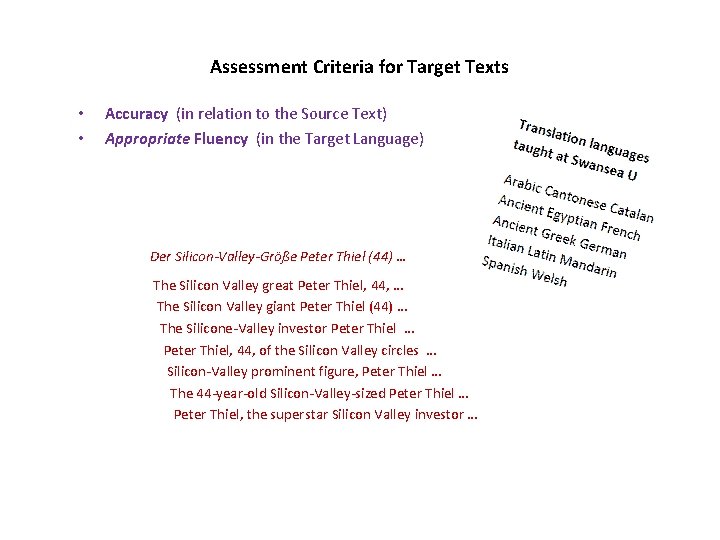
Assessment Criteria for Target Texts • • Accuracy (in relation to the Source Text) Appropriate Fluency (in the Target Language) Der Silicon-Valley-Größe Peter Thiel (44) … The Silicon Valley great Peter Thiel, 44, … The Silicon Valley giant Peter Thiel (44) … The Silicone-Valley investor Peter Thiel … Peter Thiel, 44, of the Silicon Valley circles … Silicon-Valley prominent figure, Peter Thiel … The 44 -year-old Silicon-Valley-sized Peter Thiel … Peter Thiel, the superstar Silicon Valley investor …
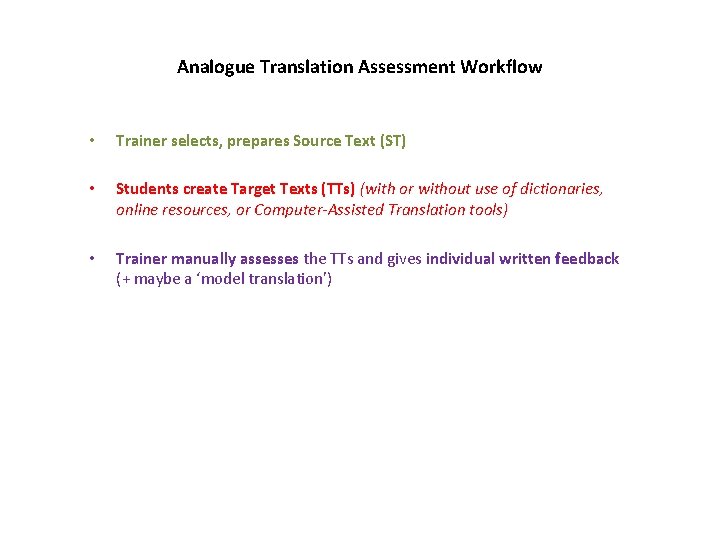
Analogue Translation Assessment Workflow • Trainer selects, prepares Source Text (ST) • Students create Target Texts (TTs) (with or without use of dictionaries, online resources, or Computer-Assisted Translation tools) • Trainer manually assesses the TTs and gives individual written feedback (+ maybe a ‘model translation’)
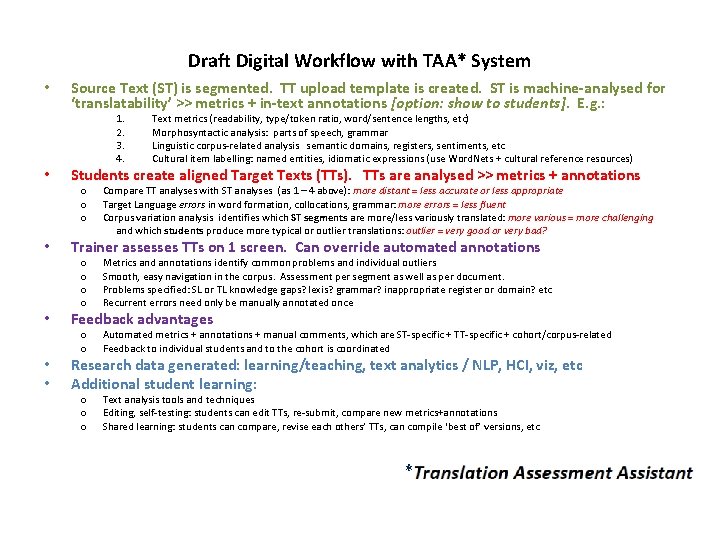
Draft Digital Workflow with TAA* System • Source Text (ST) is segmented. TT upload template is created. ST is machine-analysed for ‘translatability’ >> metrics + in-text annotations [option: show to students]. E. g. : 1. 2. 3. 4. • Students create aligned Target Texts (TTs). TTs are analysed >> metrics + annotations o o o • • Text metrics (readability, type/token ratio, word/sentence lengths, etc) Morphosyntactic analysis: parts of speech, grammar Linguistic corpus-related analysis semantic domains, registers, sentiments, etc Cultural item labelling: named entities, idiomatic expressions (use Word. Nets + cultural reference resources) Compare TT analyses with ST analyses (as 1 – 4 above): more distant = less accurate or less appropriate Target Language errors in word formation, collocations, grammar: more errors = less fluent Corpus variation analysis identifies which ST segments are more/less variously translated: more various = more challenging and which students produce more typical or outlier translations: outlier = very good or very bad? Trainer assesses TTs on 1 screen. Can override automated annotations o o Metrics and annotations identify common problems and individual outliers Smooth, easy navigation in the corpus. Assessment per segment as well as per document. Problems specified: SL or TL knowledge gaps? lexis? grammar? inappropriate register or domain? etc Recurrent errors need only be manually annotated once o o Automated metrics + annotations + manual comments, which are ST-specific + TT-specific + cohort/corpus-related Feedback to individual students and to the cohort is coordinated o o o Text analysis tools and techniques Editing, self-testing: students can edit TTs, re-submit, compare new metrics+annotations Shared learning: students can compare, revise each others’ TTs, can compile ‘best of’ versions, etc Feedback advantages Research data generated: learning/teaching, text analytics / NLP, HCI, viz, etc Additional student learning: *
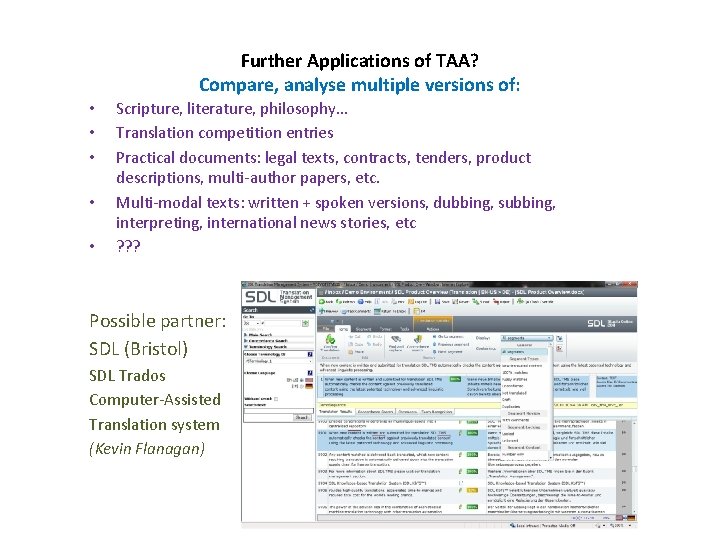
Further Applications of TAA? Compare, analyse multiple versions of: • • • Scripture, literature, philosophy… Translation competition entries Practical documents: legal texts, contracts, tenders, product descriptions, multi-author papers, etc. Multi-modal texts: written + spoken versions, dubbing, subbing, interpreting, international news stories, etc ? ? ? Possible partner: SDL (Bristol) SDL Trados Computer-Assisted Translation system (Kevin Flanagan)
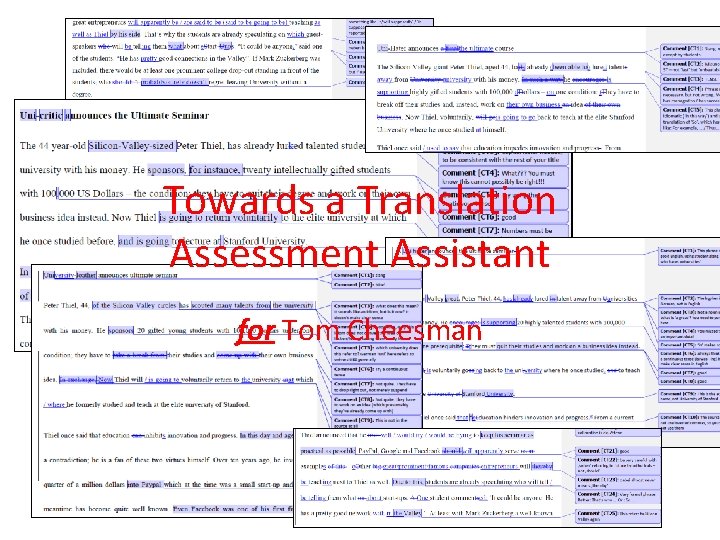
Towards a Translation Assessment Assistant for Tom Cheesman