The use of Optical Character Recognition OCR software
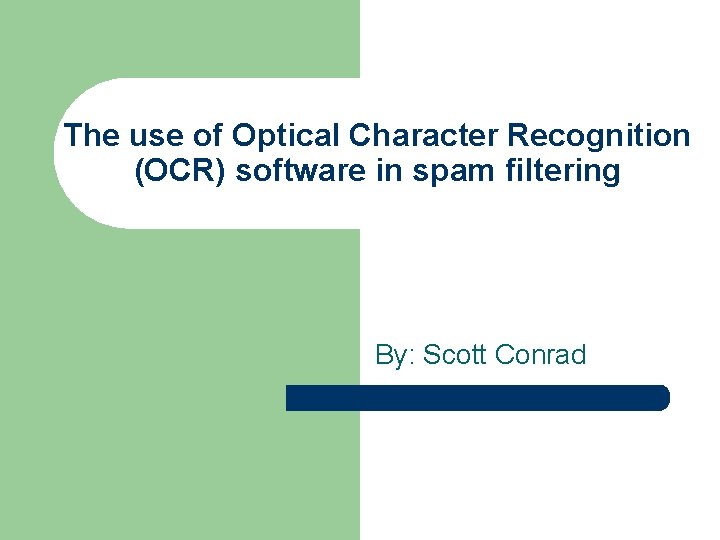
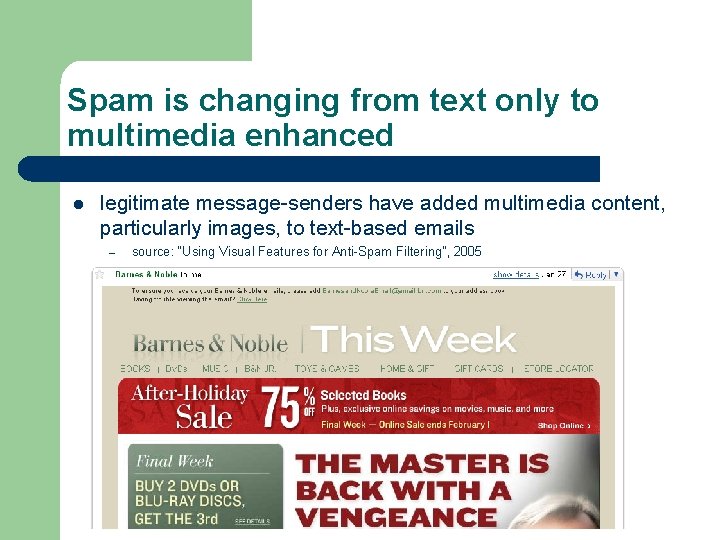
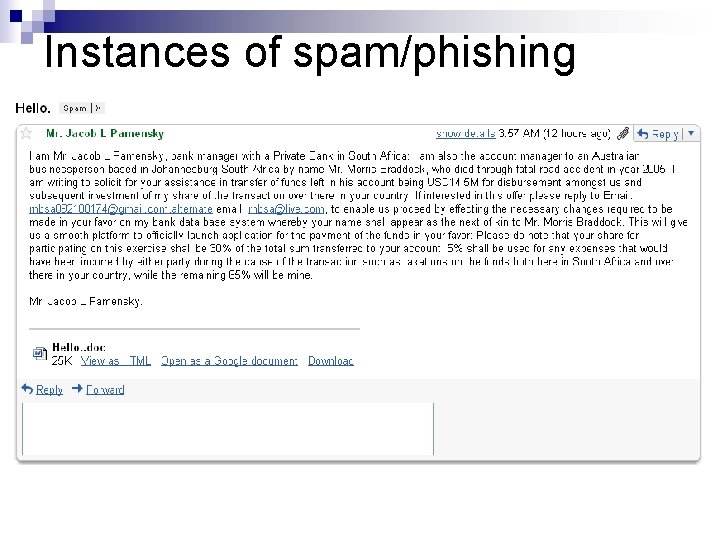
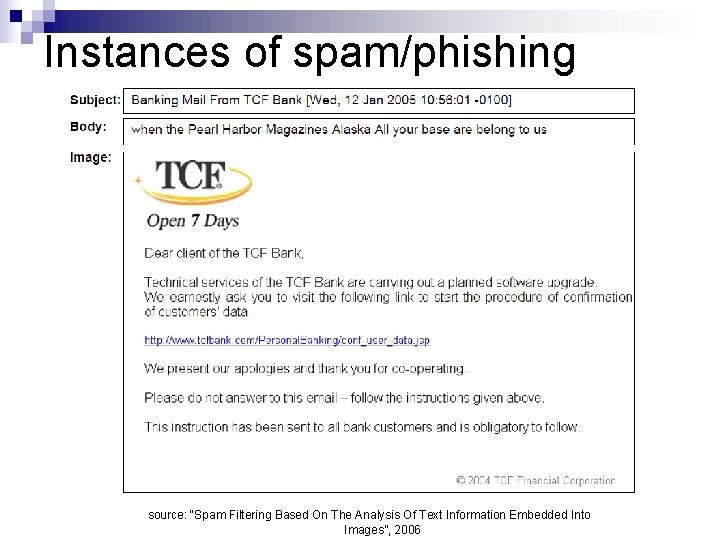
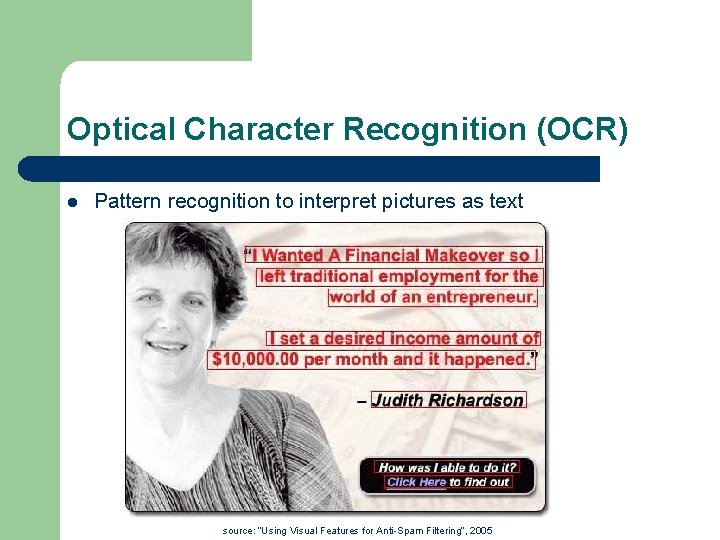
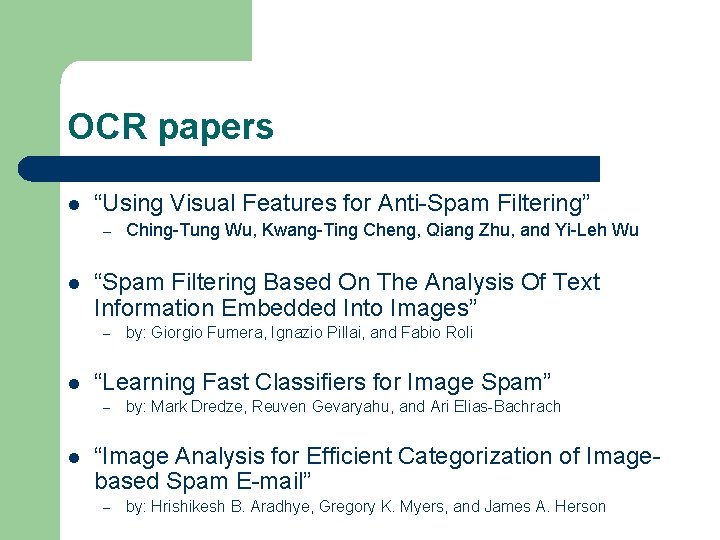
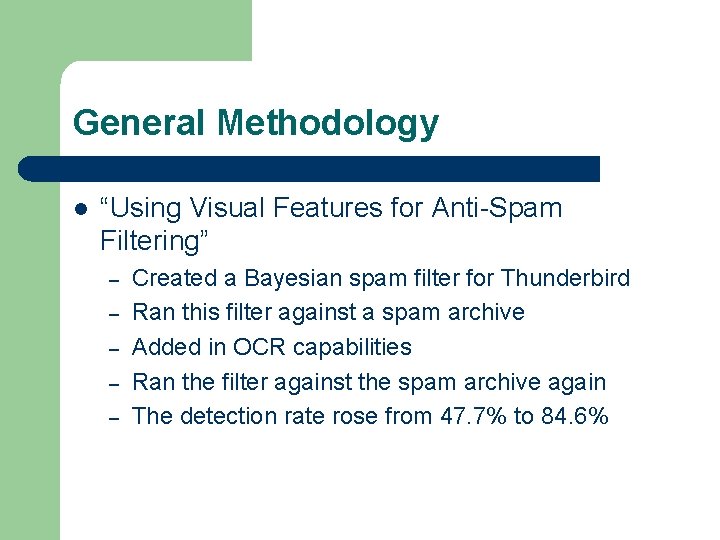
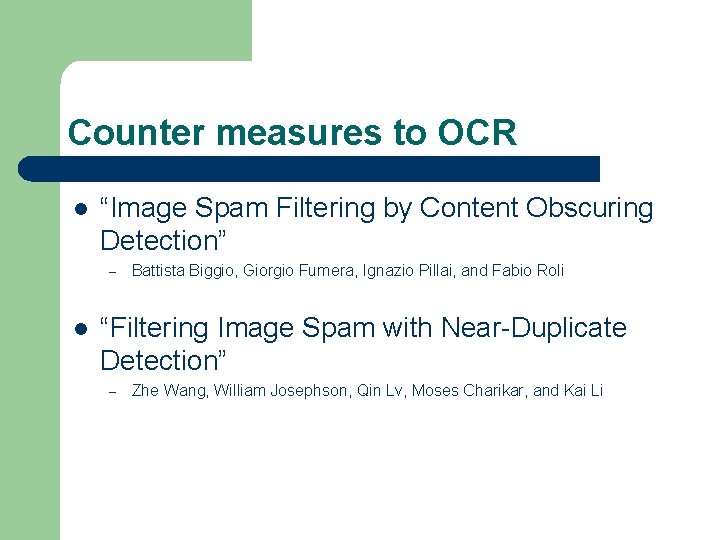
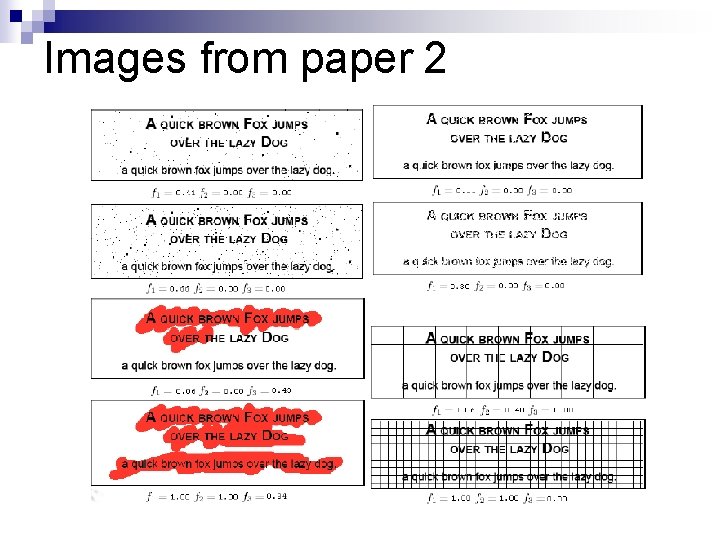
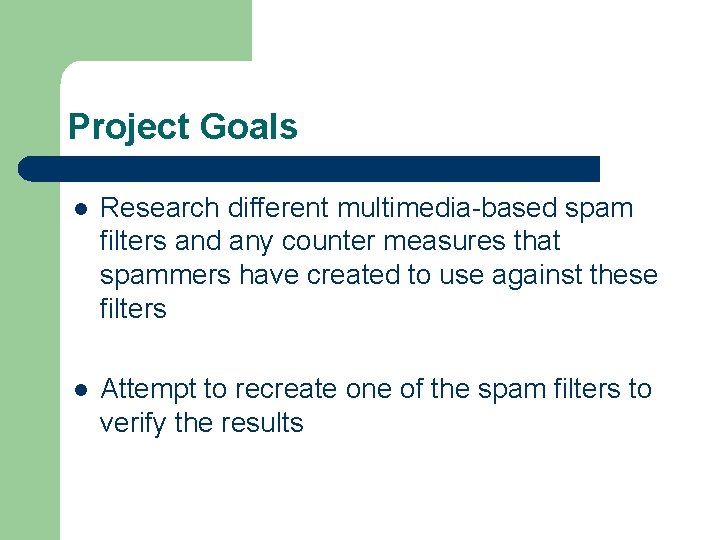
- Slides: 10
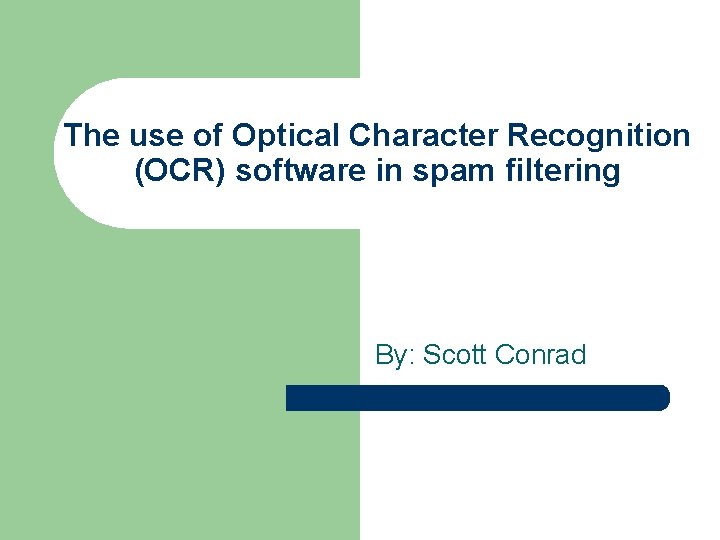
The use of Optical Character Recognition (OCR) software in spam filtering By: Scott Conrad
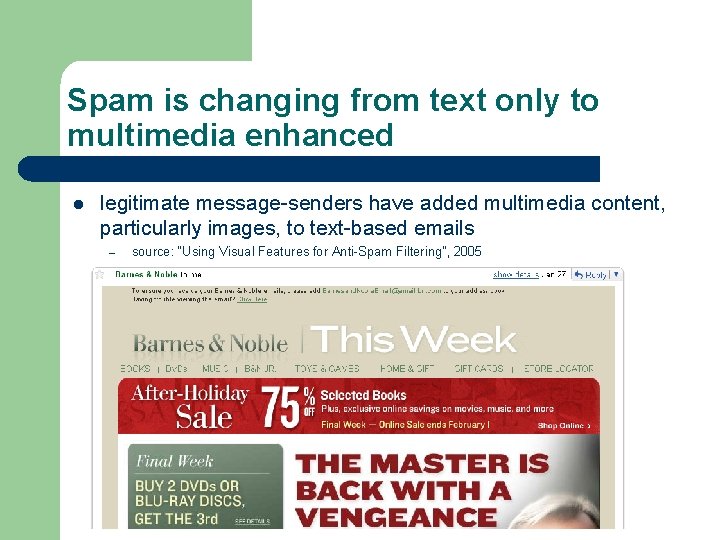
Spam is changing from text only to multimedia enhanced l legitimate message-senders have added multimedia content, particularly images, to text-based emails – source: “Using Visual Features for Anti-Spam Filtering”, 2005
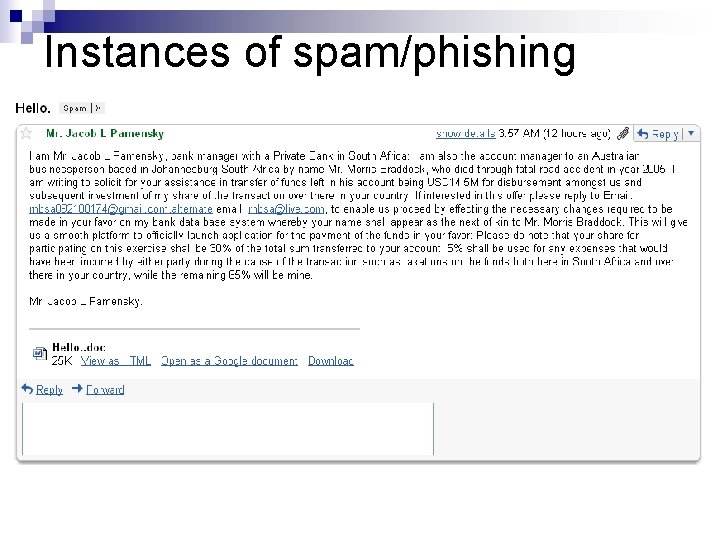
Instances of spam/phishing
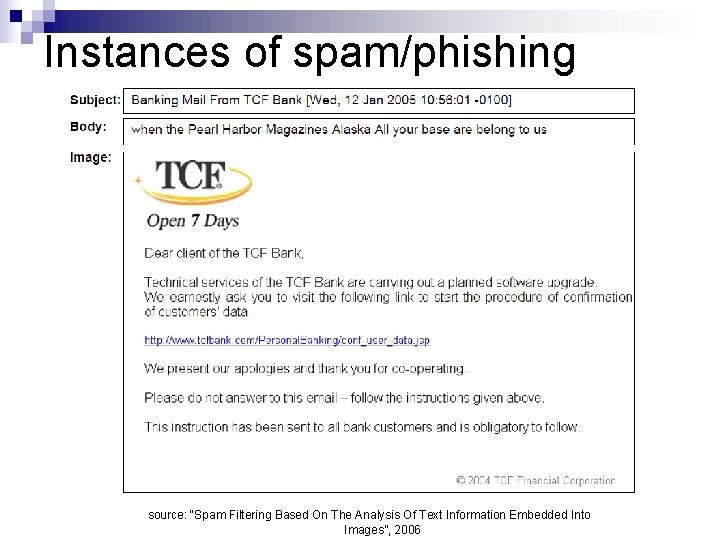
Instances of spam/phishing source: “Spam Filtering Based On The Analysis Of Text Information Embedded Into Images”, 2006
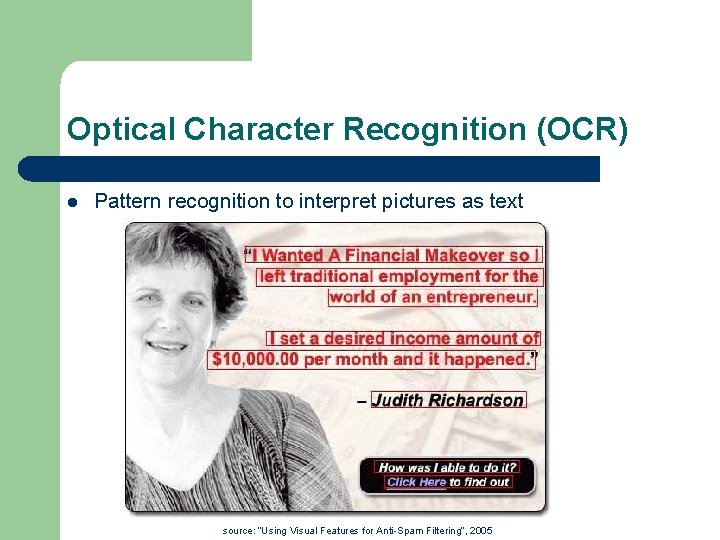
Optical Character Recognition (OCR) l Pattern recognition to interpret pictures as text source: “Using Visual Features for Anti-Spam Filtering”, 2005
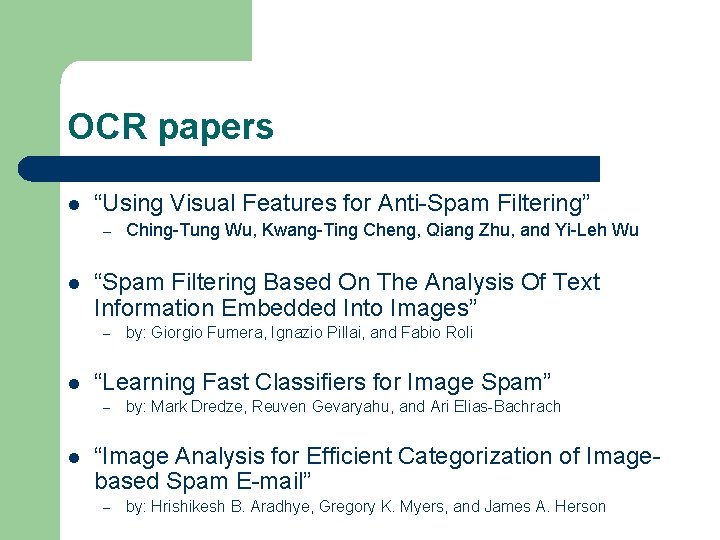
OCR papers l “Using Visual Features for Anti-Spam Filtering” – l “Spam Filtering Based On The Analysis Of Text Information Embedded Into Images” – l by: Giorgio Fumera, Ignazio Pillai, and Fabio Roli “Learning Fast Classifiers for Image Spam” – l Ching-Tung Wu, Kwang-Ting Cheng, Qiang Zhu, and Yi-Leh Wu by: Mark Dredze, Reuven Gevaryahu, and Ari Elias-Bachrach “Image Analysis for Efficient Categorization of Imagebased Spam E-mail” – by: Hrishikesh B. Aradhye, Gregory K. Myers, and James A. Herson
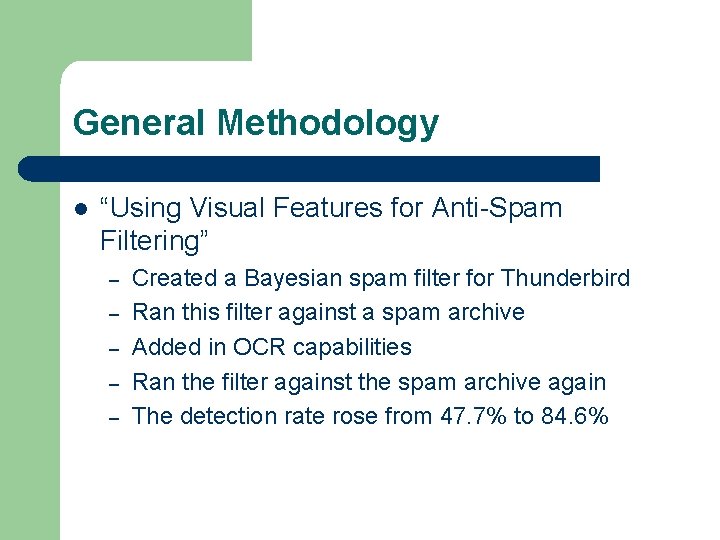
General Methodology l “Using Visual Features for Anti-Spam Filtering” – – – Created a Bayesian spam filter for Thunderbird Ran this filter against a spam archive Added in OCR capabilities Ran the filter against the spam archive again The detection rate rose from 47. 7% to 84. 6%
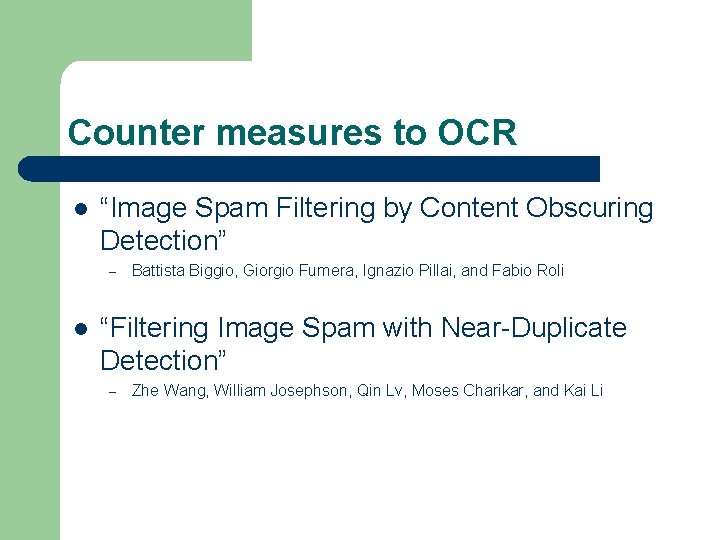
Counter measures to OCR l “Image Spam Filtering by Content Obscuring Detection” – l Battista Biggio, Giorgio Fumera, Ignazio Pillai, and Fabio Roli “Filtering Image Spam with Near-Duplicate Detection” – Zhe Wang, William Josephson, Qin Lv, Moses Charikar, and Kai Li
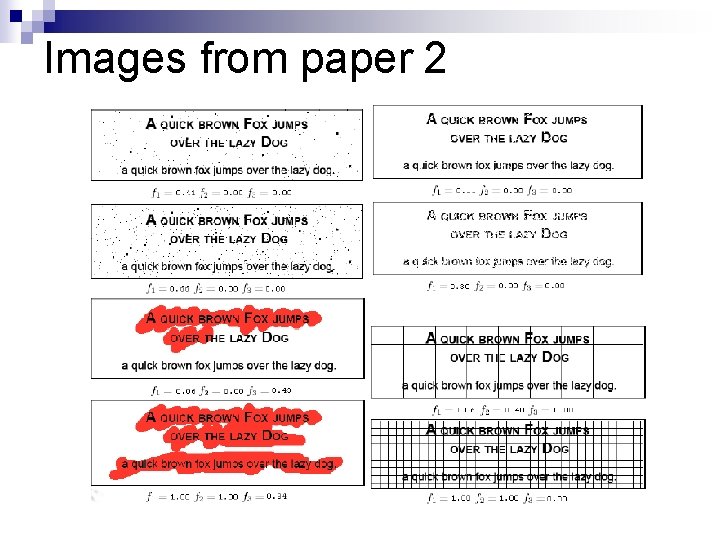
Images from paper 2
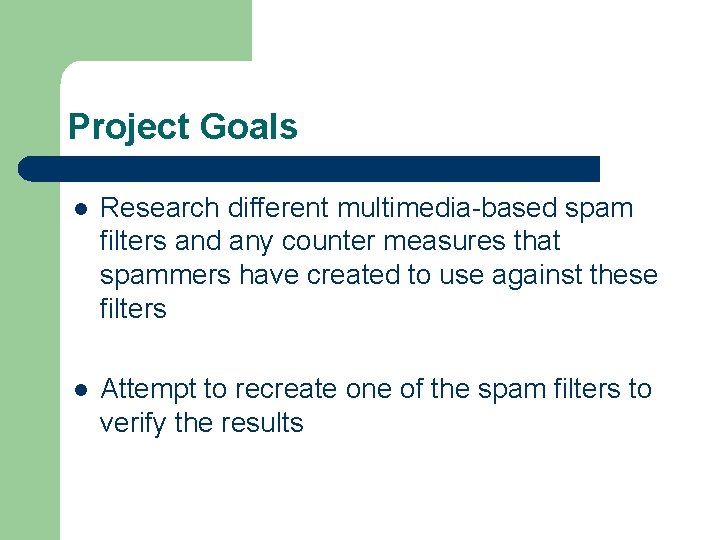
Project Goals l Research different multimedia-based spam filters and any counter measures that spammers have created to use against these filters l Attempt to recreate one of the spam filters to verify the results