TestRefine IR Research Hypothese Cheng Xiang Zhai Department
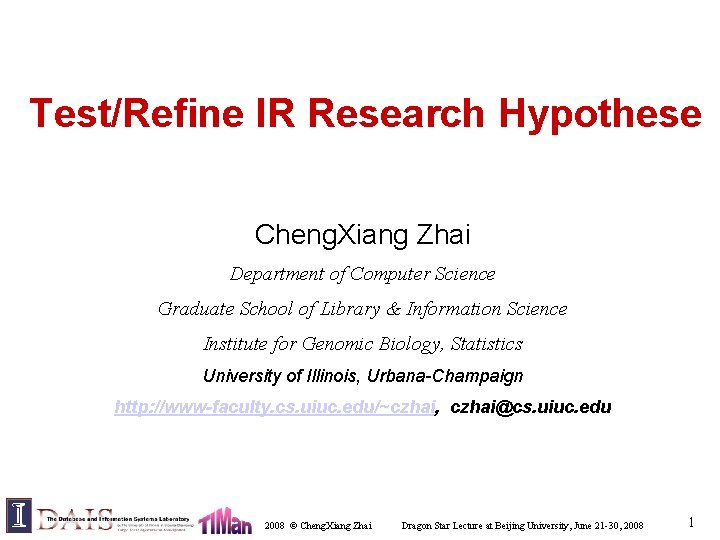
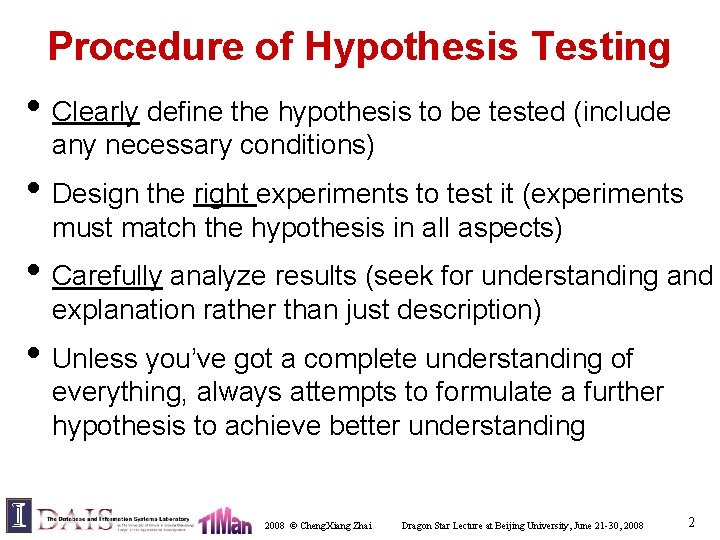
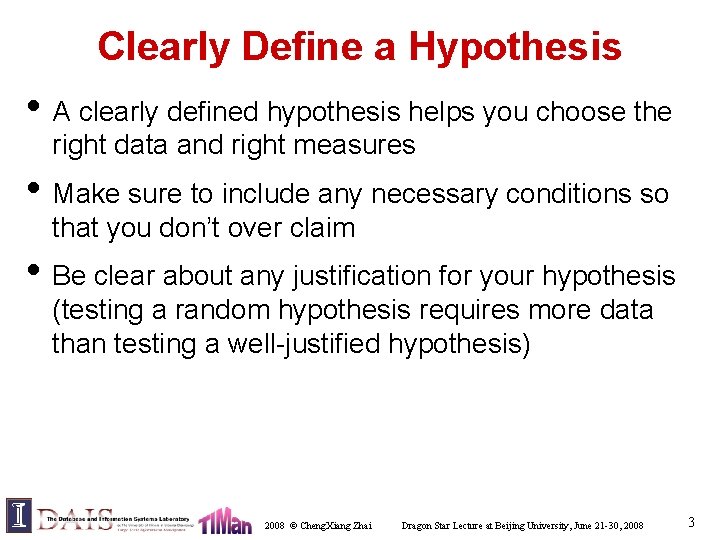
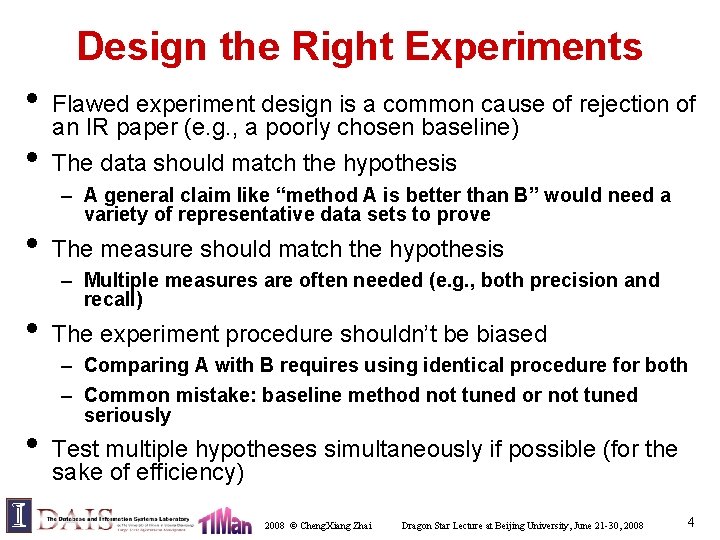
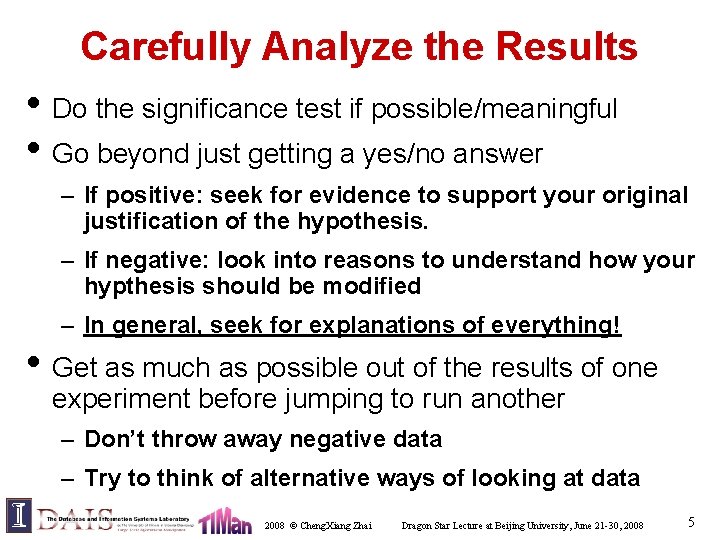
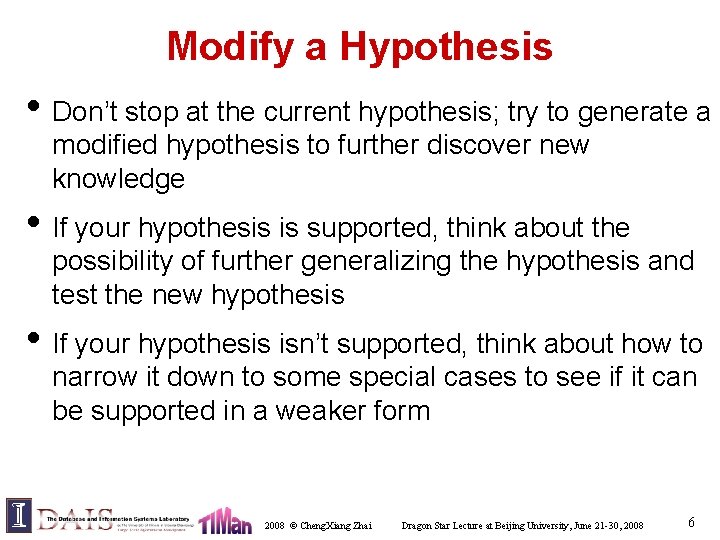
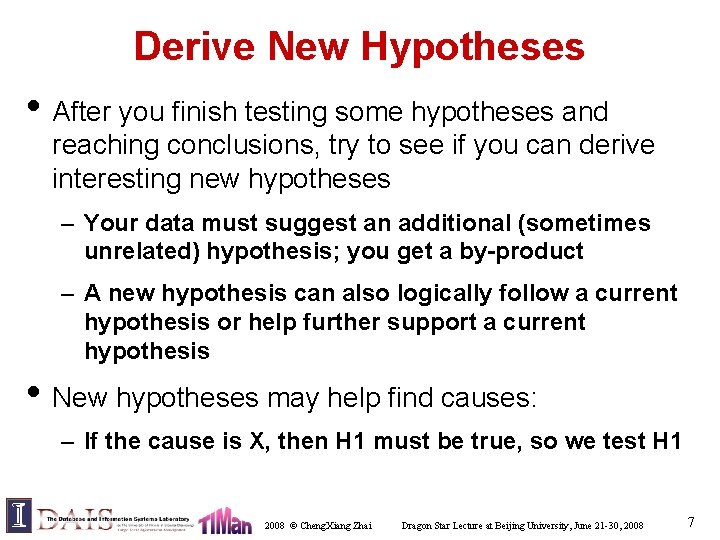
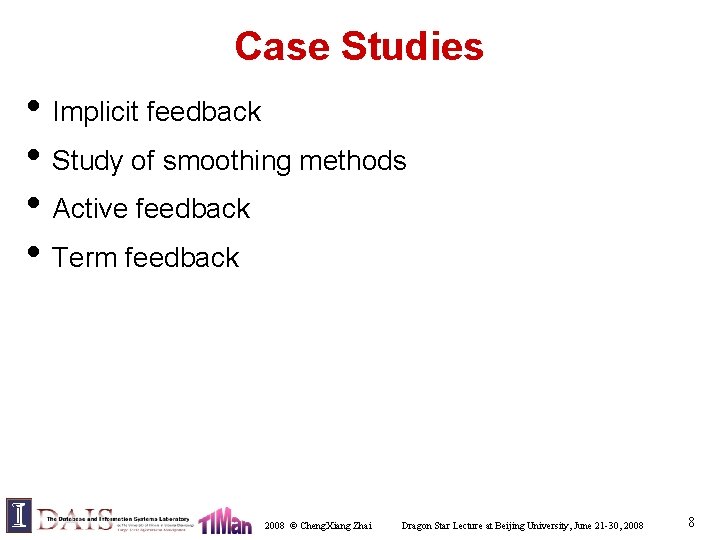
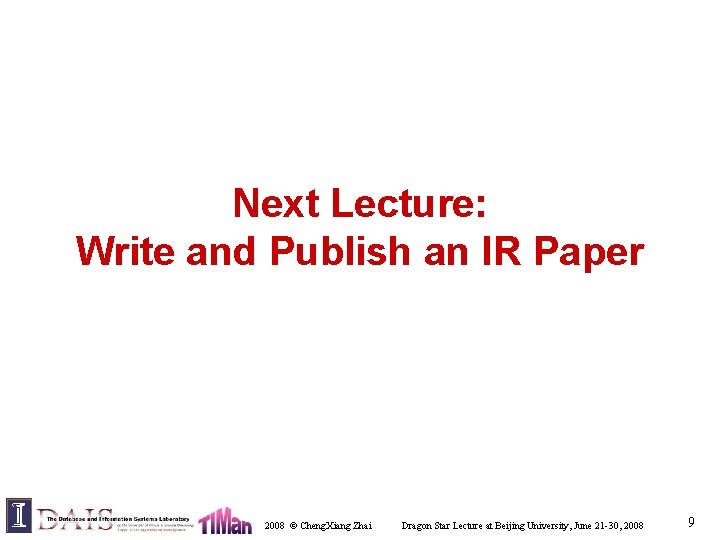
- Slides: 9
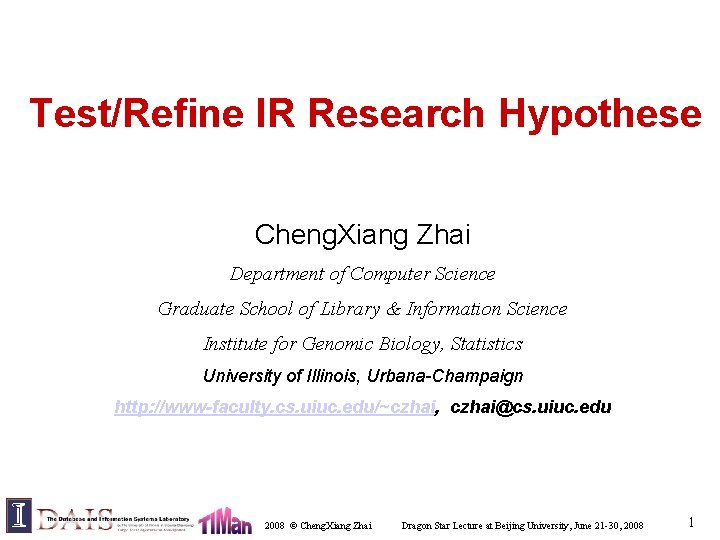
Test/Refine IR Research Hypothese Cheng. Xiang Zhai Department of Computer Science Graduate School of Library & Information Science Institute for Genomic Biology, Statistics University of Illinois, Urbana-Champaign http: //www-faculty. cs. uiuc. edu/~czhai, czhai@cs. uiuc. edu 2008 © Cheng. Xiang Zhai Dragon Star Lecture at Beijing University, June 21 -30, 2008 1
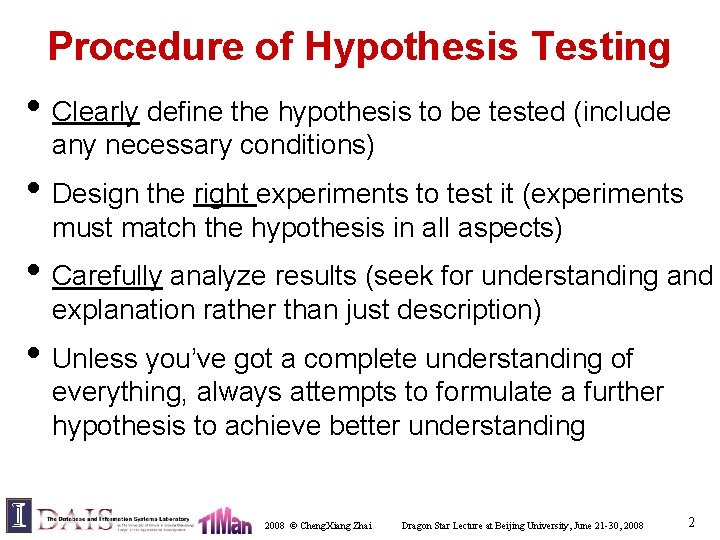
Procedure of Hypothesis Testing • Clearly define the hypothesis to be tested (include any necessary conditions) • Design the right experiments to test it (experiments must match the hypothesis in all aspects) • Carefully analyze results (seek for understanding and explanation rather than just description) • Unless you’ve got a complete understanding of everything, always attempts to formulate a further hypothesis to achieve better understanding 2008 © Cheng. Xiang Zhai Dragon Star Lecture at Beijing University, June 21 -30, 2008 2
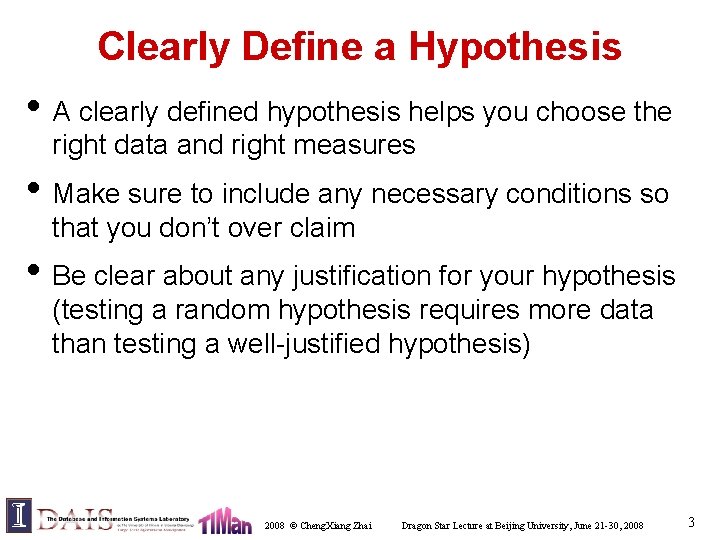
Clearly Define a Hypothesis • A clearly defined hypothesis helps you choose the right data and right measures • Make sure to include any necessary conditions so that you don’t over claim • Be clear about any justification for your hypothesis (testing a random hypothesis requires more data than testing a well-justified hypothesis) 2008 © Cheng. Xiang Zhai Dragon Star Lecture at Beijing University, June 21 -30, 2008 3
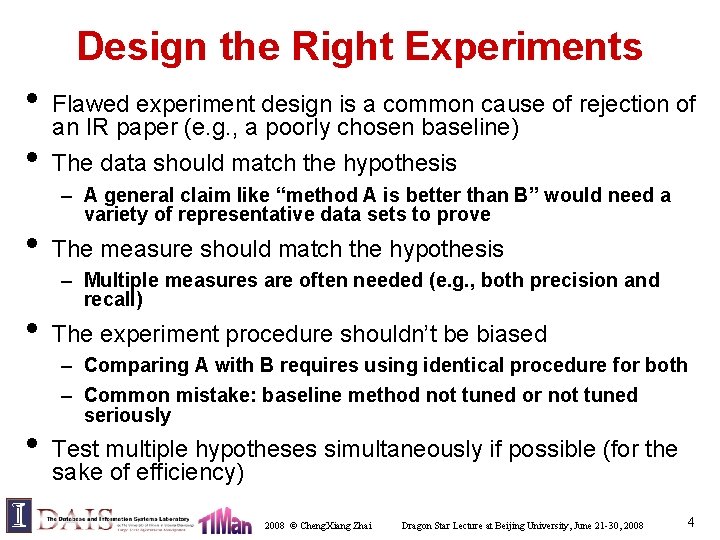
Design the Right Experiments • • • Flawed experiment design is a common cause of rejection of an IR paper (e. g. , a poorly chosen baseline) The data should match the hypothesis – A general claim like “method A is better than B” would need a variety of representative data sets to prove The measure should match the hypothesis – Multiple measures are often needed (e. g. , both precision and recall) The experiment procedure shouldn’t be biased – Comparing A with B requires using identical procedure for both – Common mistake: baseline method not tuned or not tuned seriously Test multiple hypotheses simultaneously if possible (for the sake of efficiency) 2008 © Cheng. Xiang Zhai Dragon Star Lecture at Beijing University, June 21 -30, 2008 4
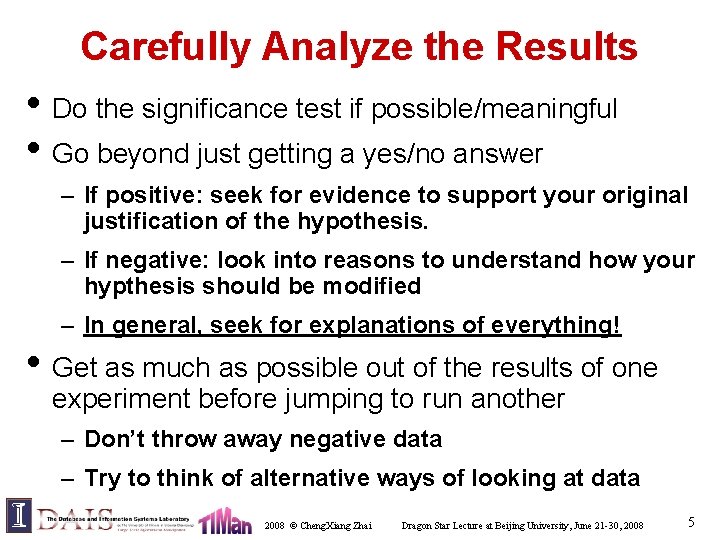
Carefully Analyze the Results • Do the significance test if possible/meaningful • Go beyond just getting a yes/no answer – If positive: seek for evidence to support your original justification of the hypothesis. – If negative: look into reasons to understand how your hypthesis should be modified – In general, seek for explanations of everything! • Get as much as possible out of the results of one experiment before jumping to run another – Don’t throw away negative data – Try to think of alternative ways of looking at data 2008 © Cheng. Xiang Zhai Dragon Star Lecture at Beijing University, June 21 -30, 2008 5
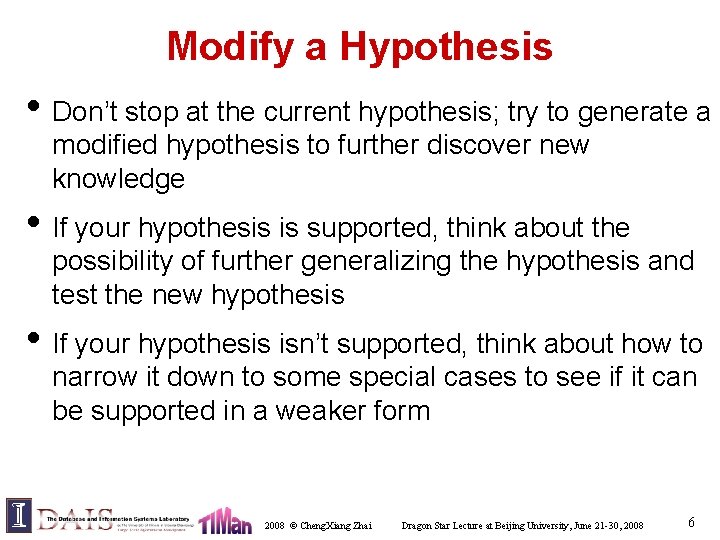
Modify a Hypothesis • Don’t stop at the current hypothesis; try to generate a modified hypothesis to further discover new knowledge • If your hypothesis is supported, think about the possibility of further generalizing the hypothesis and test the new hypothesis • If your hypothesis isn’t supported, think about how to narrow it down to some special cases to see if it can be supported in a weaker form 2008 © Cheng. Xiang Zhai Dragon Star Lecture at Beijing University, June 21 -30, 2008 6
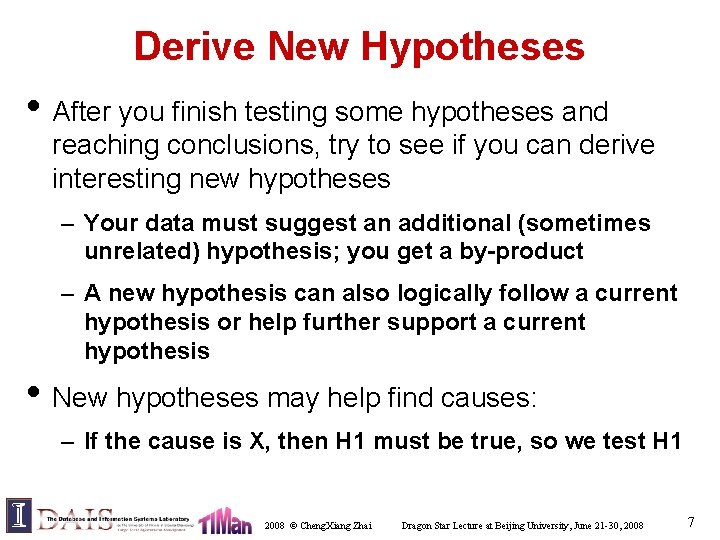
Derive New Hypotheses • After you finish testing some hypotheses and reaching conclusions, try to see if you can derive interesting new hypotheses – Your data must suggest an additional (sometimes unrelated) hypothesis; you get a by-product – A new hypothesis can also logically follow a current hypothesis or help further support a current hypothesis • New hypotheses may help find causes: – If the cause is X, then H 1 must be true, so we test H 1 2008 © Cheng. Xiang Zhai Dragon Star Lecture at Beijing University, June 21 -30, 2008 7
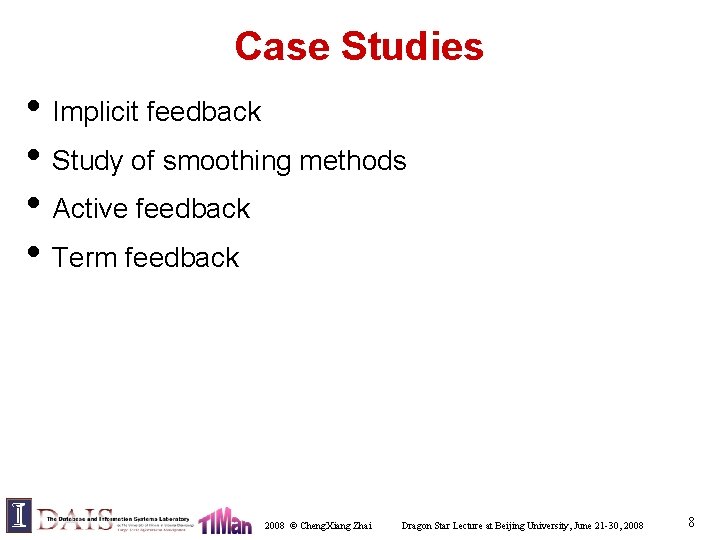
Case Studies • Implicit feedback • Study of smoothing methods • Active feedback • Term feedback 2008 © Cheng. Xiang Zhai Dragon Star Lecture at Beijing University, June 21 -30, 2008 8
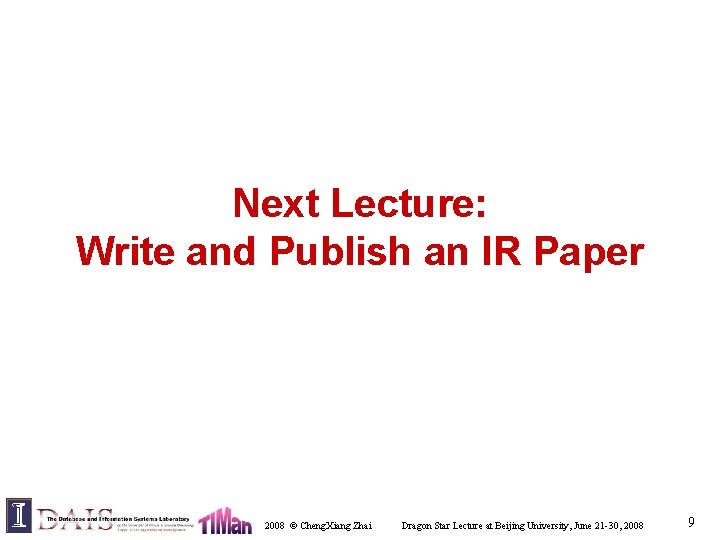
Next Lecture: Write and Publish an IR Paper 2008 © Cheng. Xiang Zhai Dragon Star Lecture at Beijing University, June 21 -30, 2008 9