Systematically Fair Modeling of Perioperative Cognitive Outcomes A
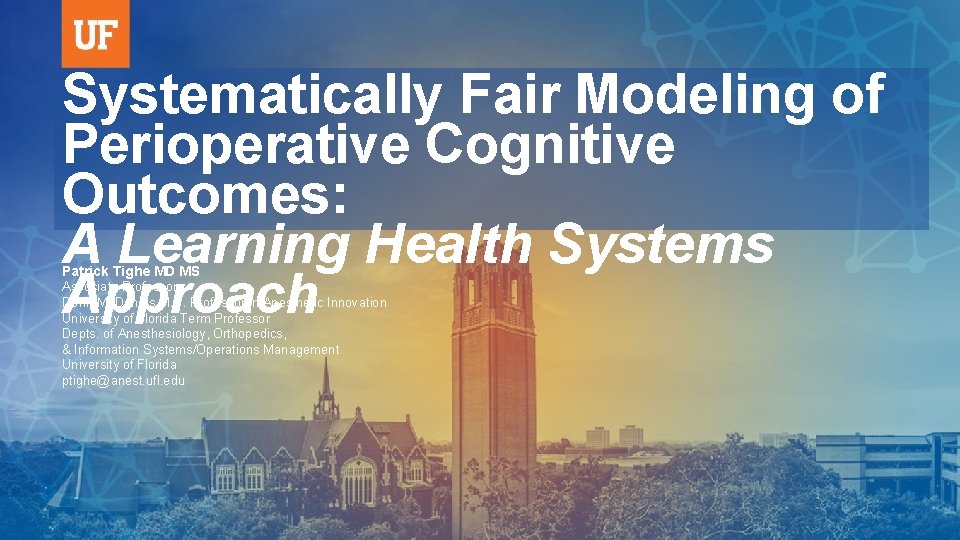
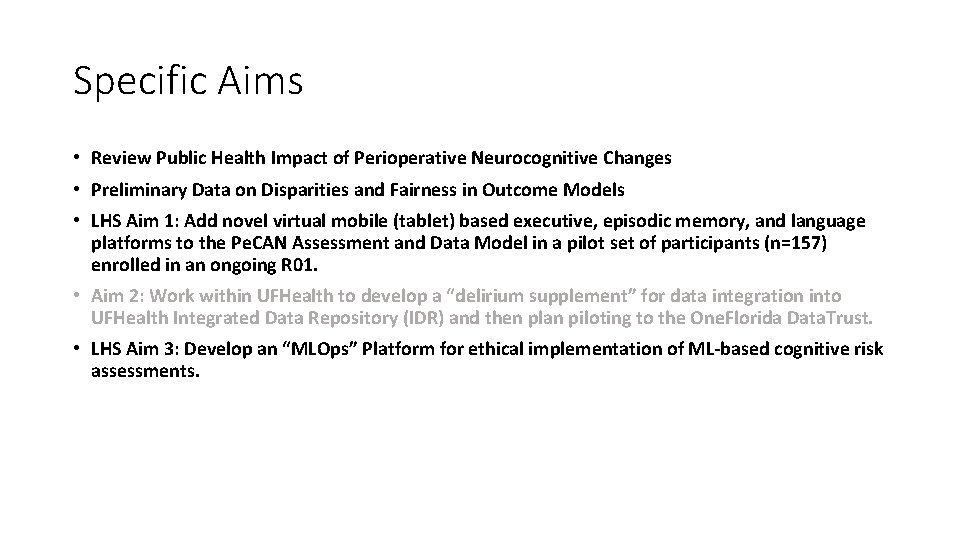
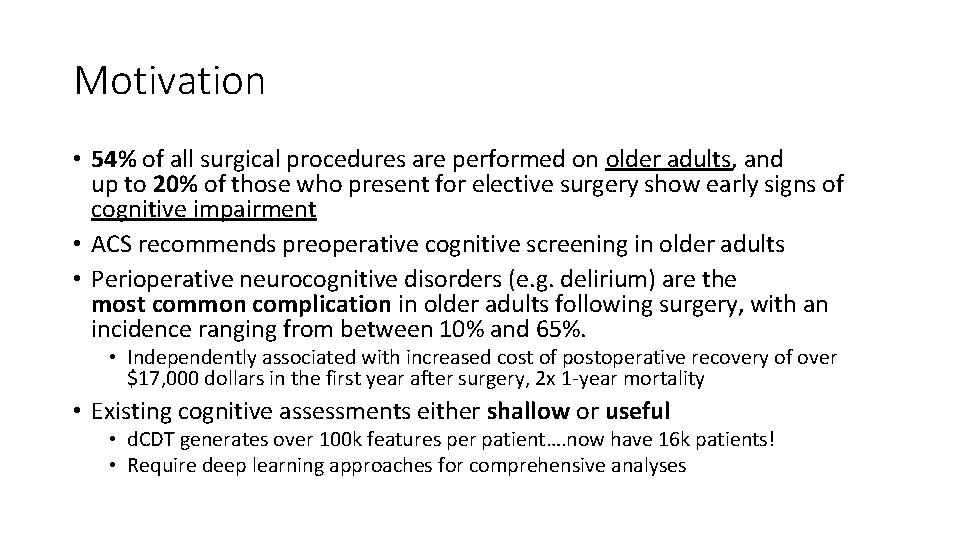
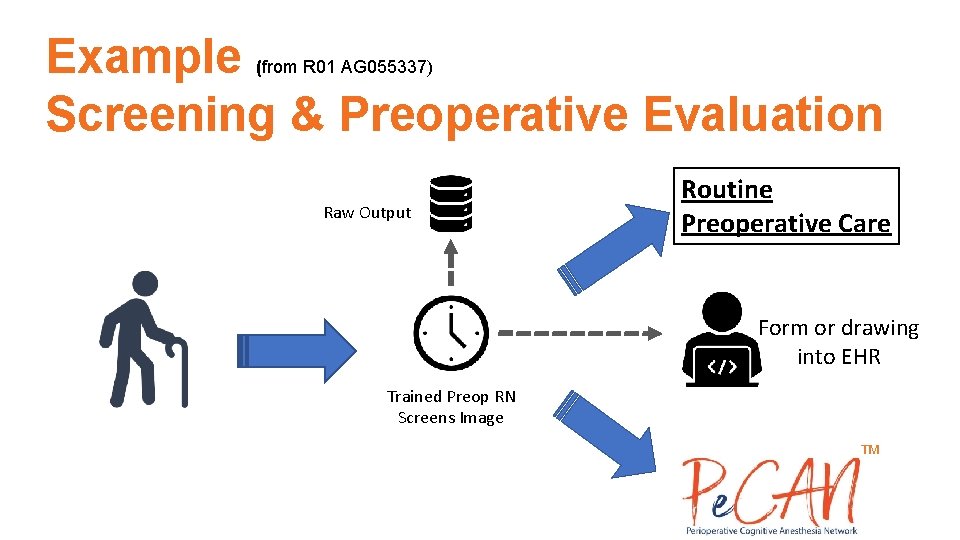
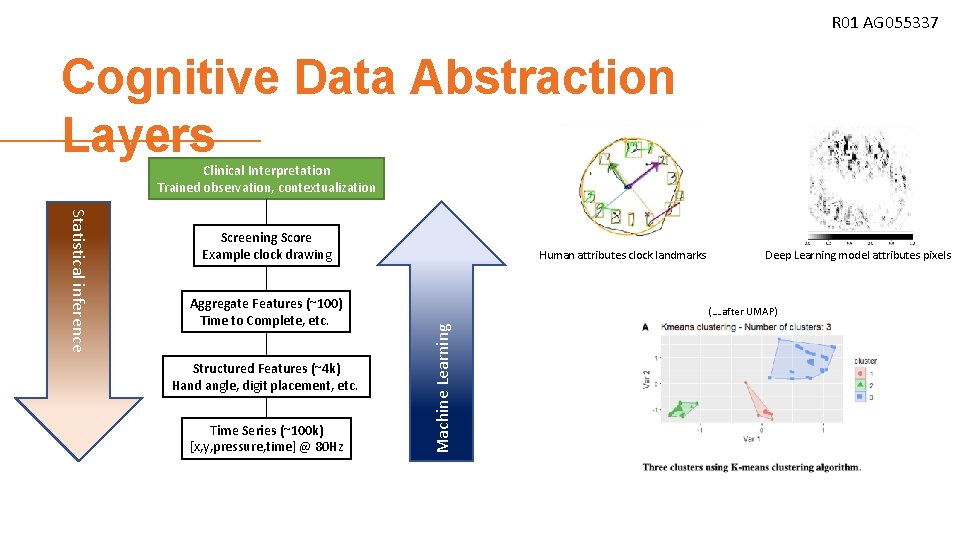
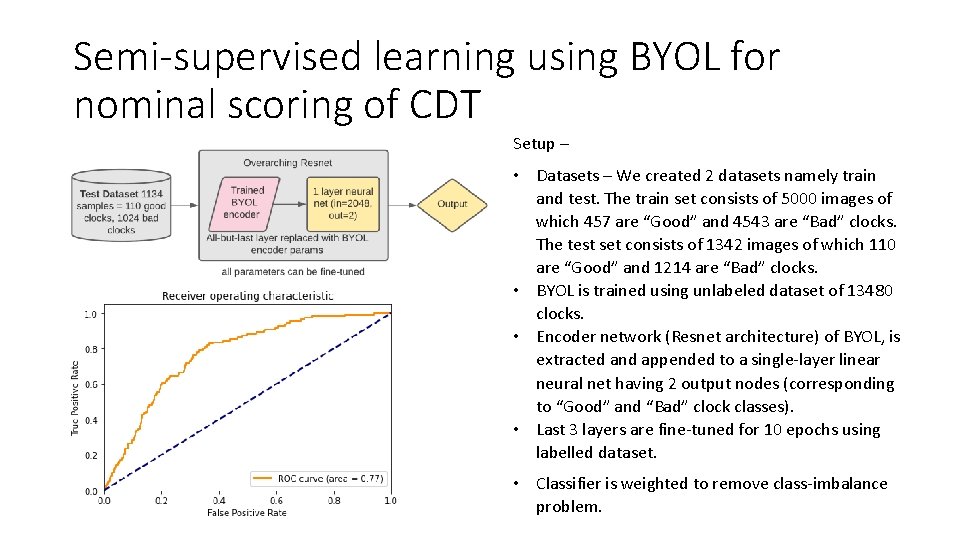
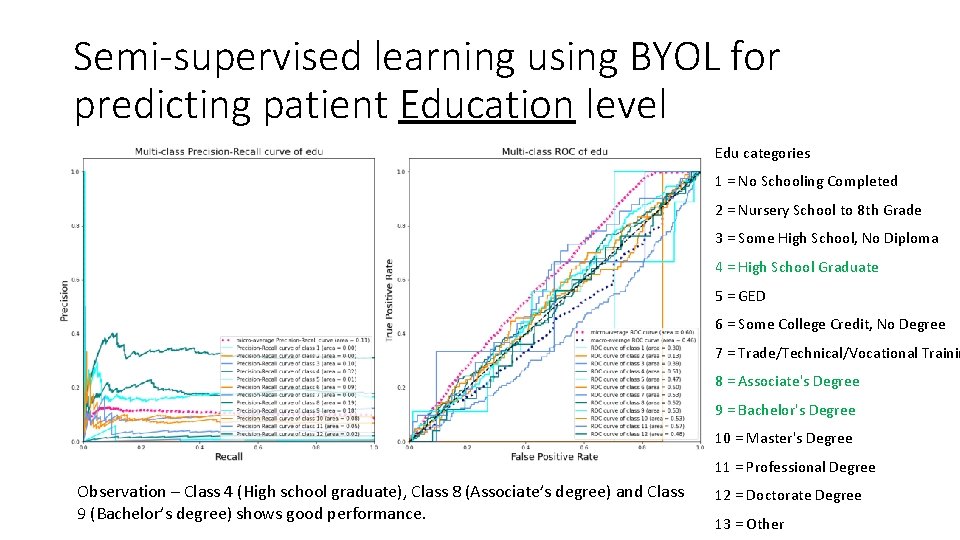
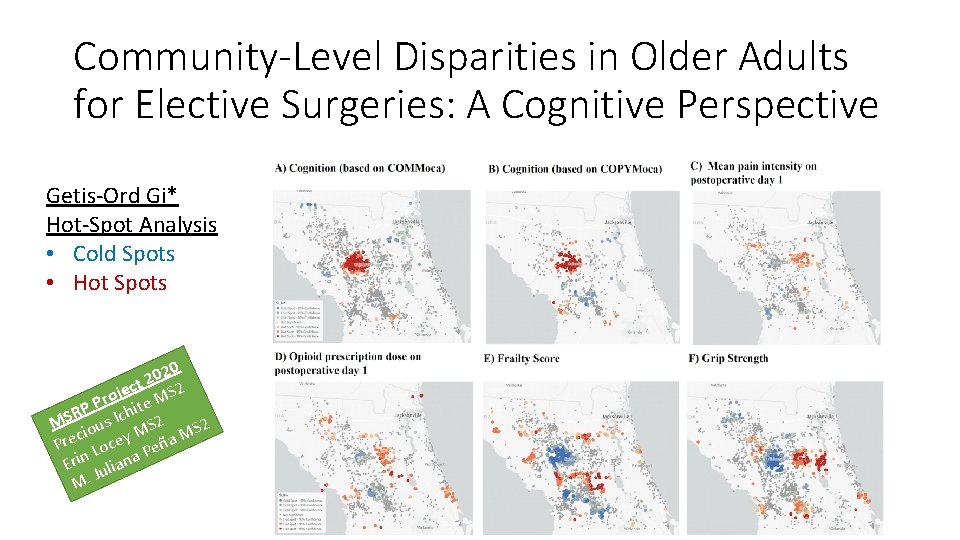
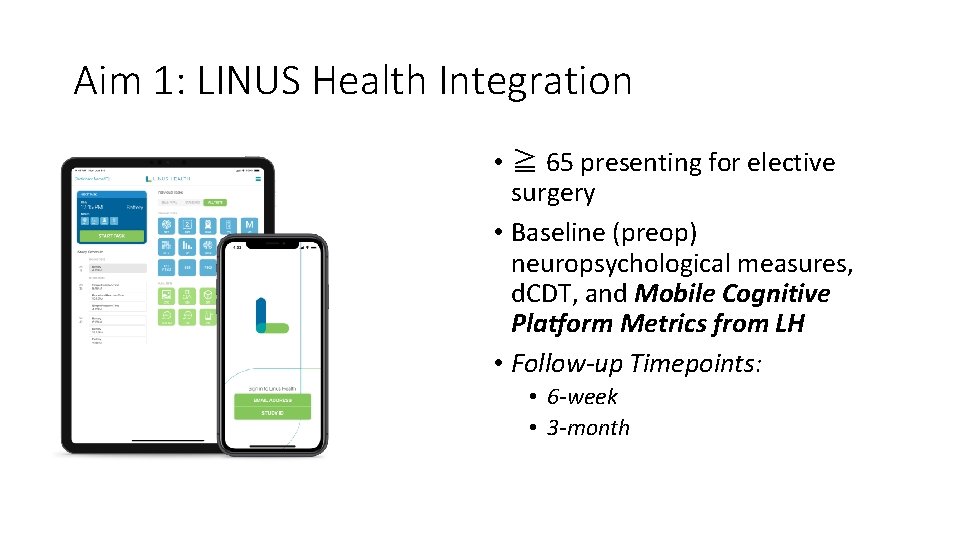
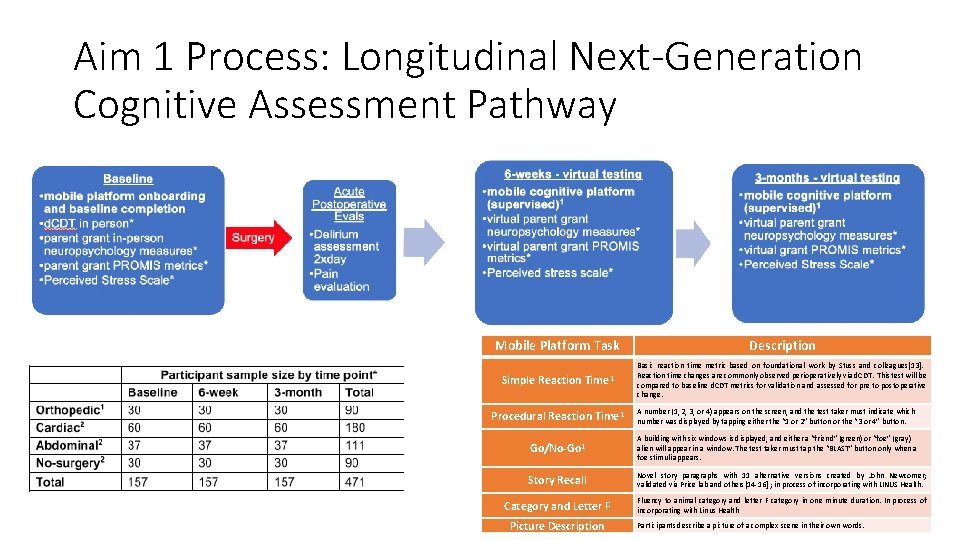
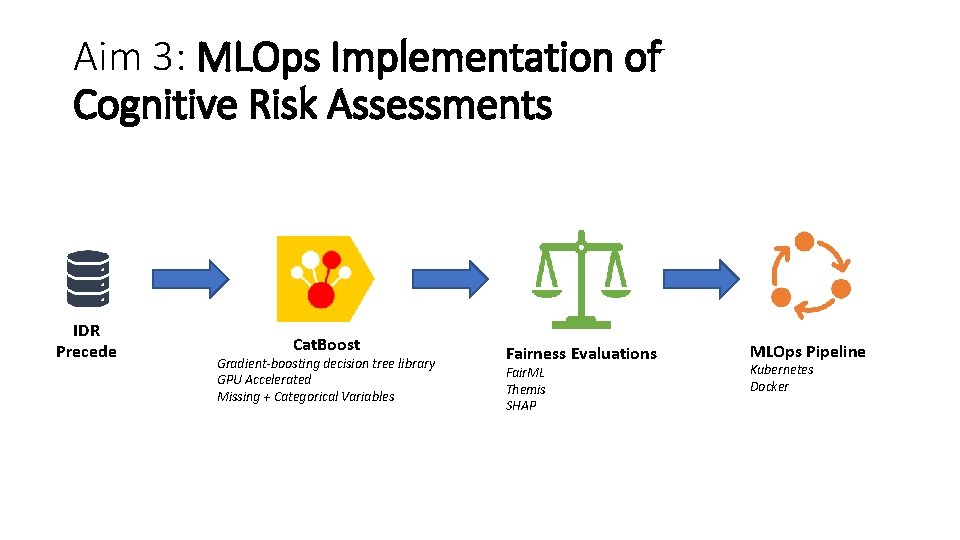
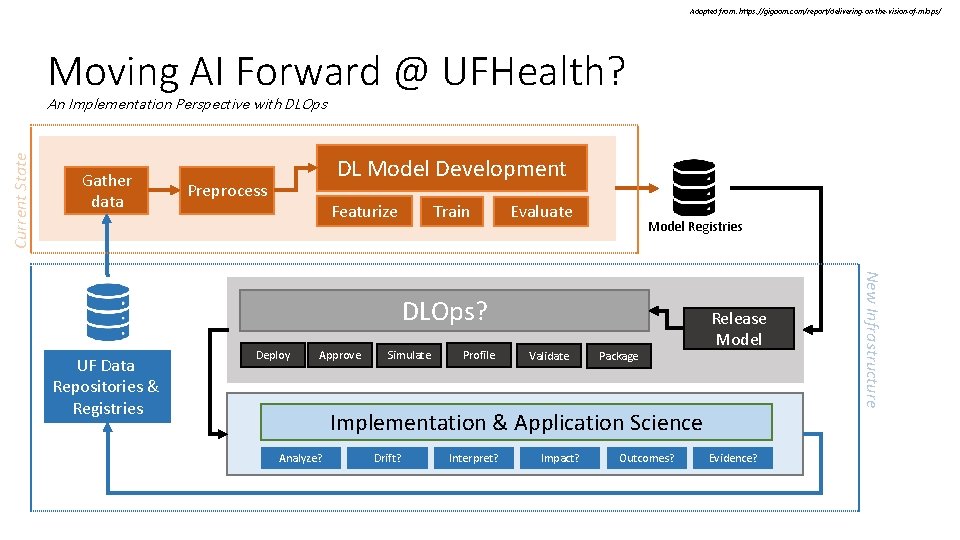
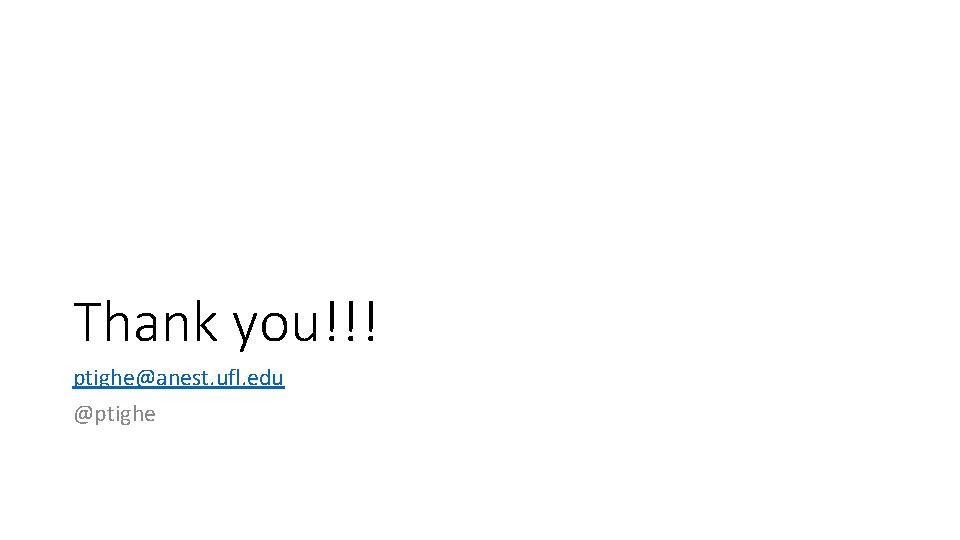
- Slides: 13
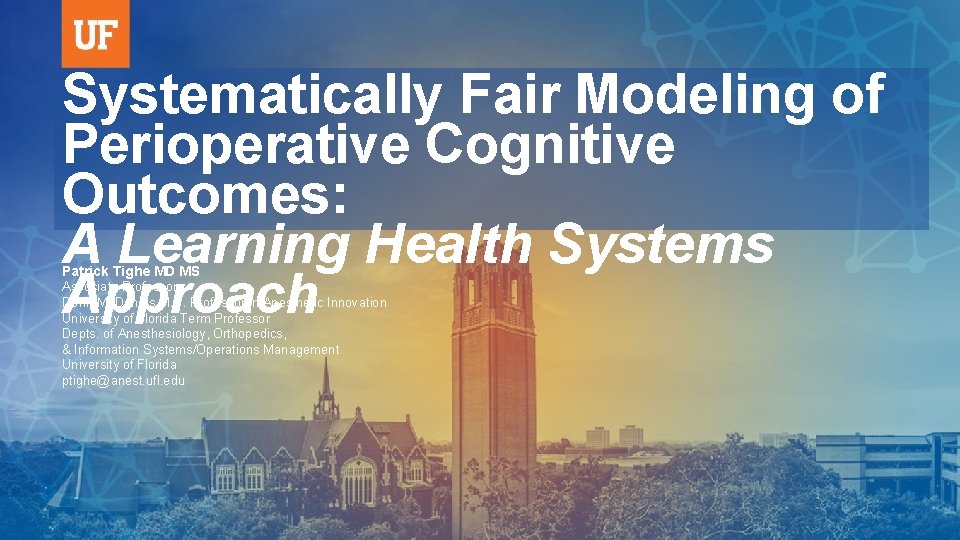
Systematically Fair Modeling of Perioperative Cognitive Outcomes: A Learning Health Systems Approach Patrick Tighe MD MS Associate Professor Donn M. Dennis M. D. Professor in Anesthetic Innovation University of Florida Term Professor Depts. of Anesthesiology, Orthopedics, & Information Systems/Operations Management University of Florida ptighe@anest. ufl. edu
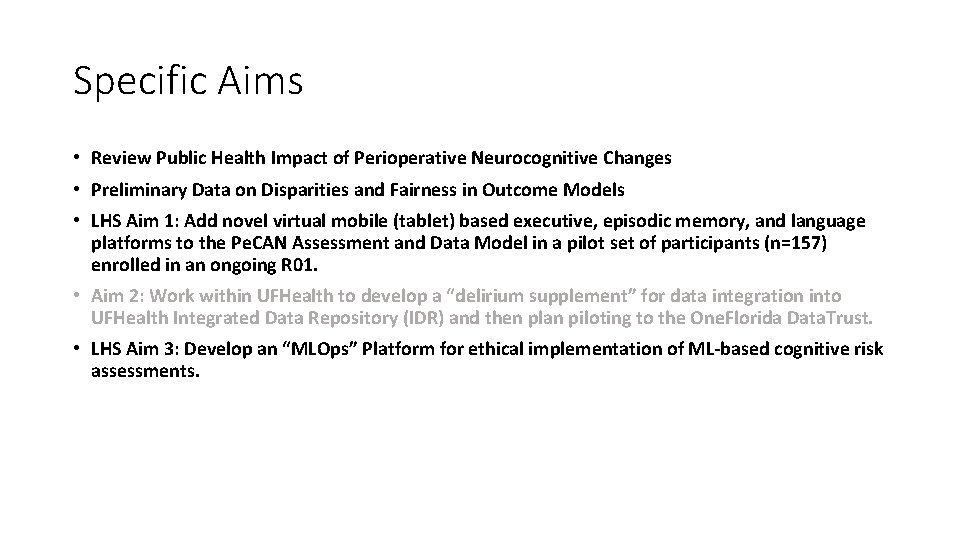
Specific Aims • Review Public Health Impact of Perioperative Neurocognitive Changes • Preliminary Data on Disparities and Fairness in Outcome Models • LHS Aim 1: Add novel virtual mobile (tablet) based executive, episodic memory, and language platforms to the Pe. CAN Assessment and Data Model in a pilot set of participants (n=157) enrolled in an ongoing R 01. • Aim 2: Work within UFHealth to develop a “delirium supplement” for data integration into UFHealth Integrated Data Repository (IDR) and then plan piloting to the One. Florida Data. Trust. • LHS Aim 3: Develop an “MLOps” Platform for ethical implementation of ML-based cognitive risk assessments.
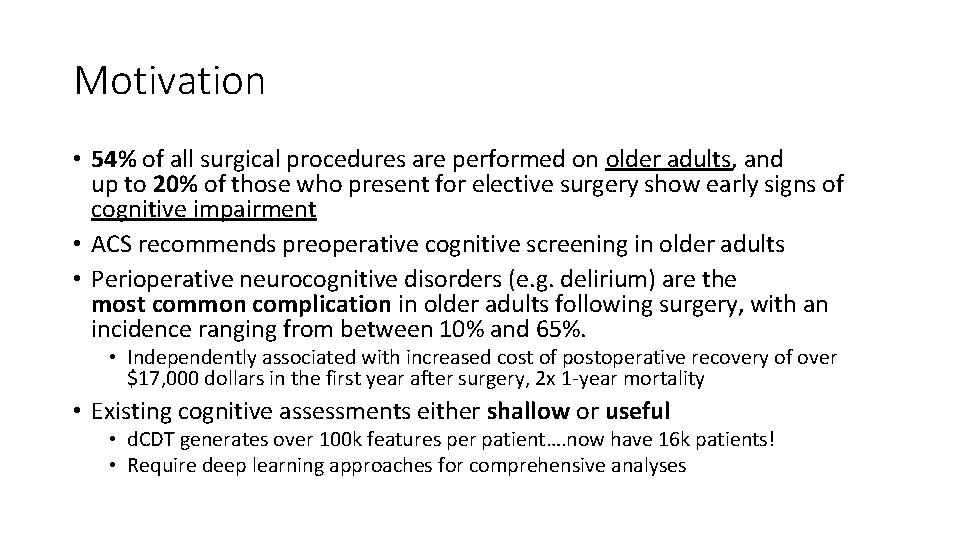
Motivation • 54% of all surgical procedures are performed on older adults, and up to 20% of those who present for elective surgery show early signs of cognitive impairment • ACS recommends preoperative cognitive screening in older adults • Perioperative neurocognitive disorders (e. g. delirium) are the most common complication in older adults following surgery, with an incidence ranging from between 10% and 65%. • Independently associated with increased cost of postoperative recovery of over $17, 000 dollars in the first year after surgery, 2 x 1 -year mortality • Existing cognitive assessments either shallow or useful • d. CDT generates over 100 k features per patient…. now have 16 k patients! • Require deep learning approaches for comprehensive analyses
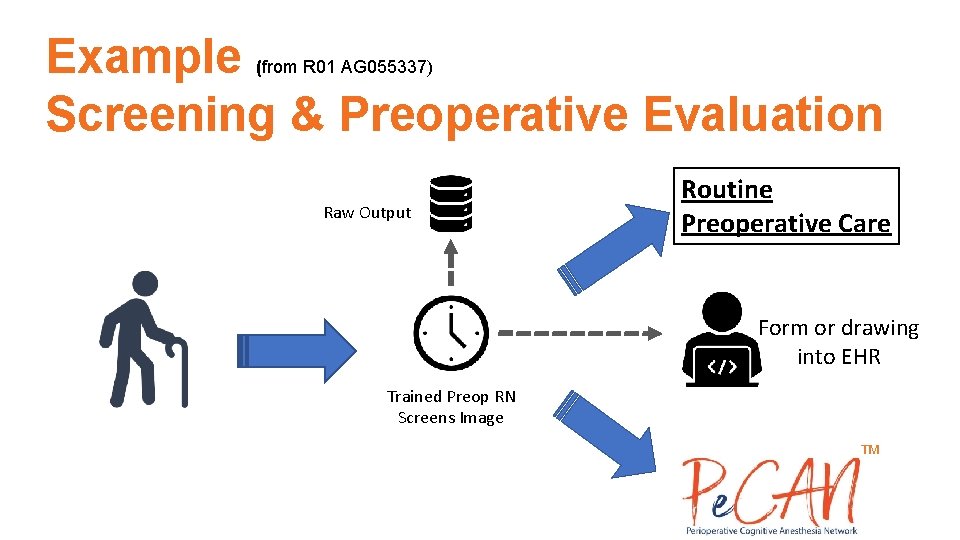
Example Screening & Preoperative Evaluation (from R 01 AG 055337) Raw Output Routine Preoperative Care Form or drawing into EHR Trained Preop RN Screens Image TM
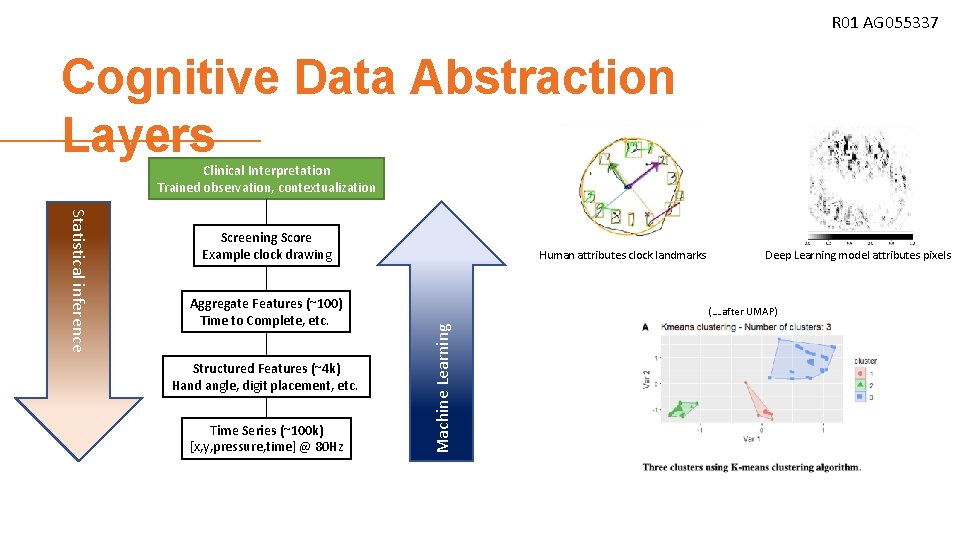
R 01 AG 055337 Cognitive Data Abstraction Layers Clinical Interpretation Trained observation, contextualization Aggregate Features (~100) Time to Complete, etc. Structured Features (~4 k) Hand angle, digit placement, etc. Time Series (~100 k) [x, y, pressure, time] @ 80 Hz Human attributes clock landmarks Deep Learning model attributes pixels (…. after UMAP) Machine Learning Statistical inference Screening Score Example clock drawing
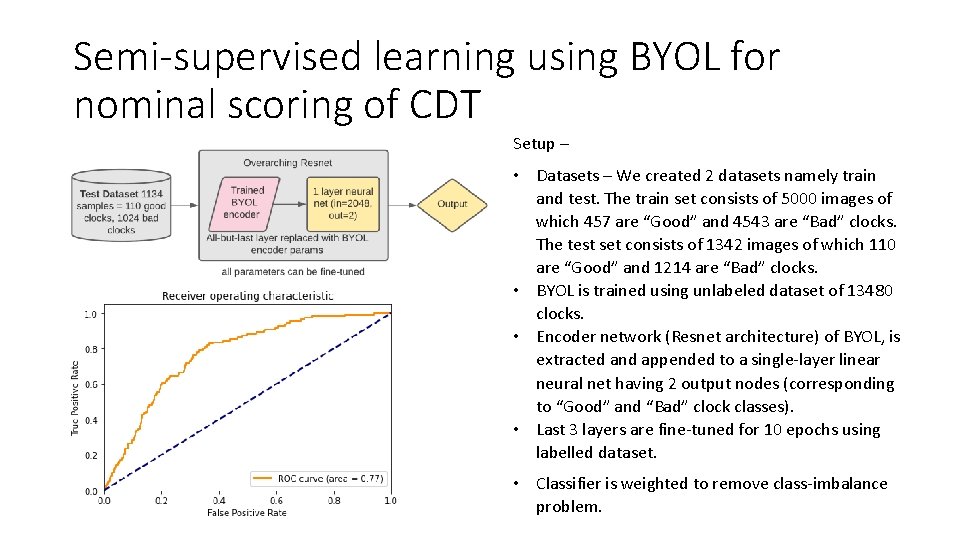
Semi-supervised learning using BYOL for nominal scoring of CDT Setup – • Datasets – We created 2 datasets namely train and test. The train set consists of 5000 images of which 457 are “Good” and 4543 are “Bad” clocks. The test set consists of 1342 images of which 110 are “Good” and 1214 are “Bad” clocks. • BYOL is trained using unlabeled dataset of 13480 clocks. • Encoder network (Resnet architecture) of BYOL, is extracted and appended to a single-layer linear neural net having 2 output nodes (corresponding to “Good” and “Bad” clock classes). • Last 3 layers are fine-tuned for 10 epochs using labelled dataset. • Classifier is weighted to remove class-imbalance problem.
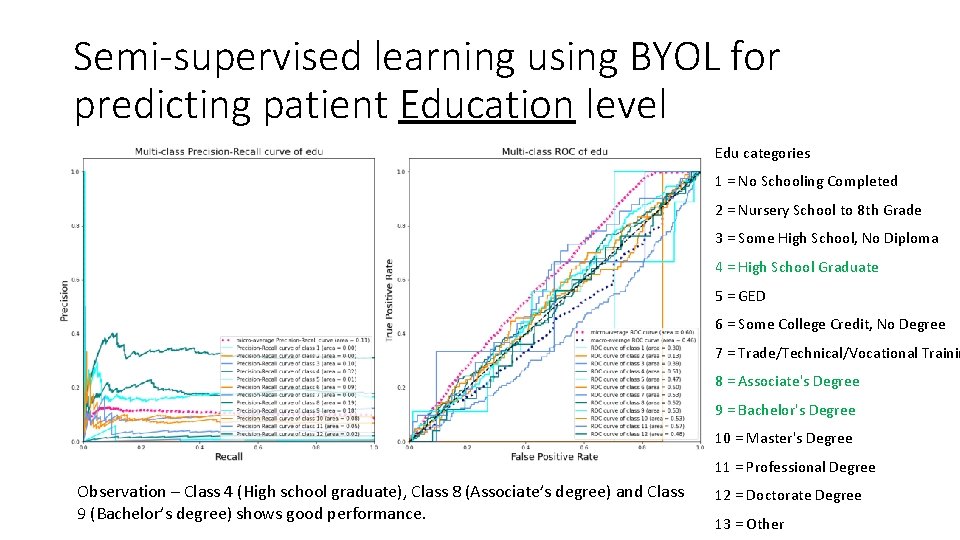
Semi-supervised learning using BYOL for predicting patient Education level Edu categories 1 = No Schooling Completed 2 = Nursery School to 8 th Grade 3 = Some High School, No Diploma 4 = High School Graduate 5 = GED 6 = Some College Credit, No Degree 7 = Trade/Technical/Vocational Trainin 8 = Associate's Degree 9 = Bachelor's Degree 10 = Master's Degree 11 = Professional Degree Observation – Class 4 (High school graduate), Class 8 (Associate’s degree) and Class 9 (Bachelor’s degree) shows good performance. 12 = Doctorate Degree 13 = Other
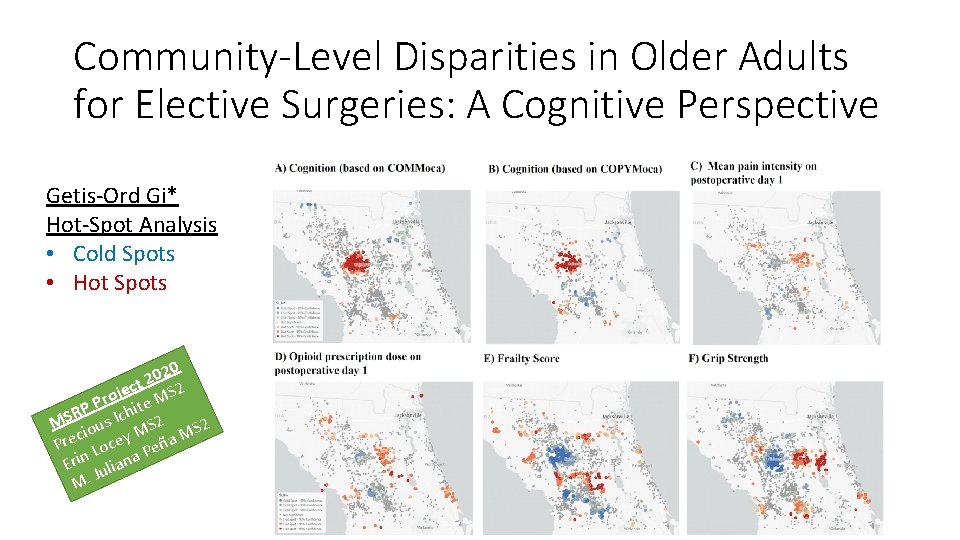
Community-Level Disparities in Older Adults for Elective Surgeries: A Cognitive Perspective Getis-Ord Gi* Hot-Spot Analysis • Cold Spots • Hot Spots 20 0 2 ct S 2 e j o Pr ite M P h R MS ious Ic MS 2 M c y Pre Loce Peña Erin uliana J M.
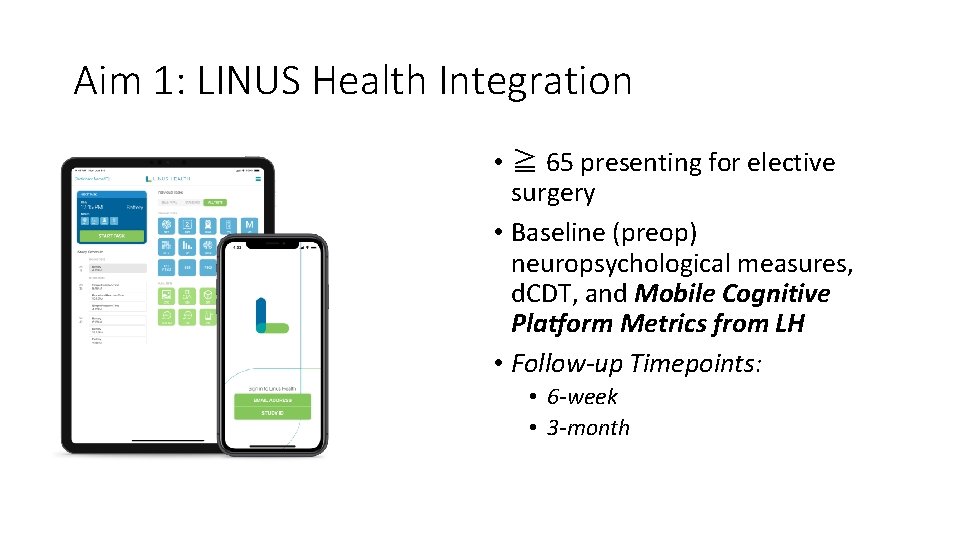
Aim 1: LINUS Health Integration • ≧ 65 presenting for elective surgery • Baseline (preop) neuropsychological measures, d. CDT, and Mobile Cognitive Platform Metrics from LH • Follow-up Timepoints: • 6 -week • 3 -month
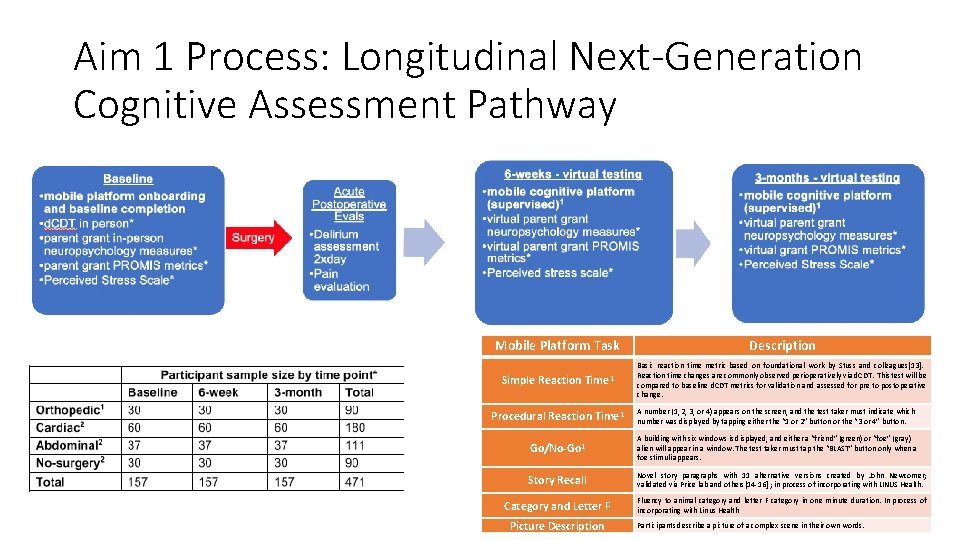
Aim 1 Process: Longitudinal Next-Generation Cognitive Assessment Pathway Mobile Platform Task Simple Reaction Time 1 Description Basic reaction time metric based on foundational work by Stuss and colleagues[13]. Reaction time changes are commonly observed perioperatively via d. CDT. This test will be compared to baseline d. CDT metrics for validation and assessed for pre to postoperative change. Procedural Reaction Time 1 A number (1, 2, 3, or 4) appears on the screen, and the test taker must indicate which number was displayed by tapping either the “ 1 or 2” button or the “ 3 or 4” button. Go/No-Go 1 A building with six windows is displayed, and either a “friend” (green) or “foe” (gray) alien will appear in a window. The test taker must tap the “BLAST” button only when a foe stimuli appears. Story Recall Novel story paragraphs with 11 alternative versions created by John Newcomer; validated via Price lab and others[14 -16] ; in process of incorporating with LINUS Health. Category and Letter F Fluency to animal category and letter F category in one minute duration. In process of incorporating with Linus Health Picture Description Participants describe a picture of a complex scene in their own words.
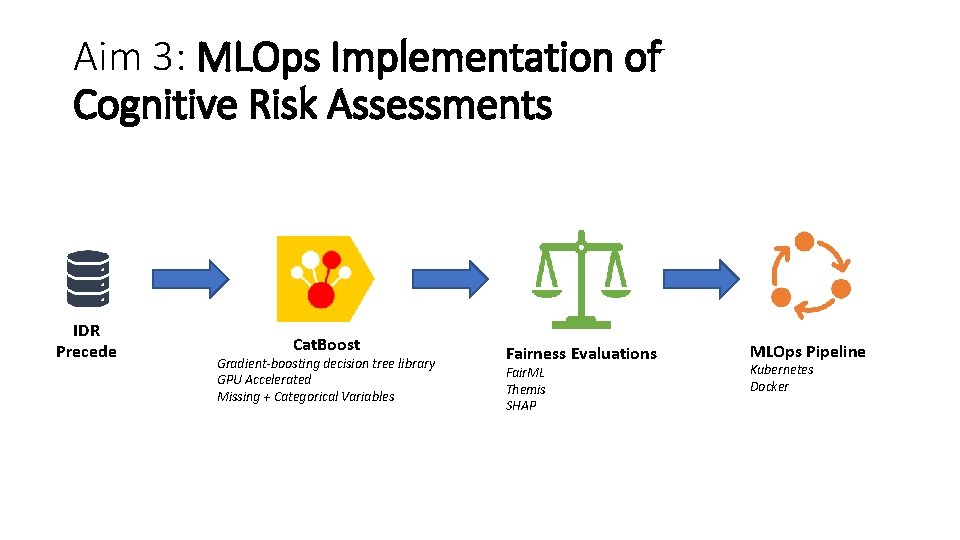
Aim 3: MLOps Implementation of Cognitive Risk Assessments IDR Precede Cat. Boost Gradient-boosting decision tree library GPU Accelerated Missing + Categorical Variables Fairness Evaluations Fair. ML Themis SHAP MLOps Pipeline Kubernetes Docker
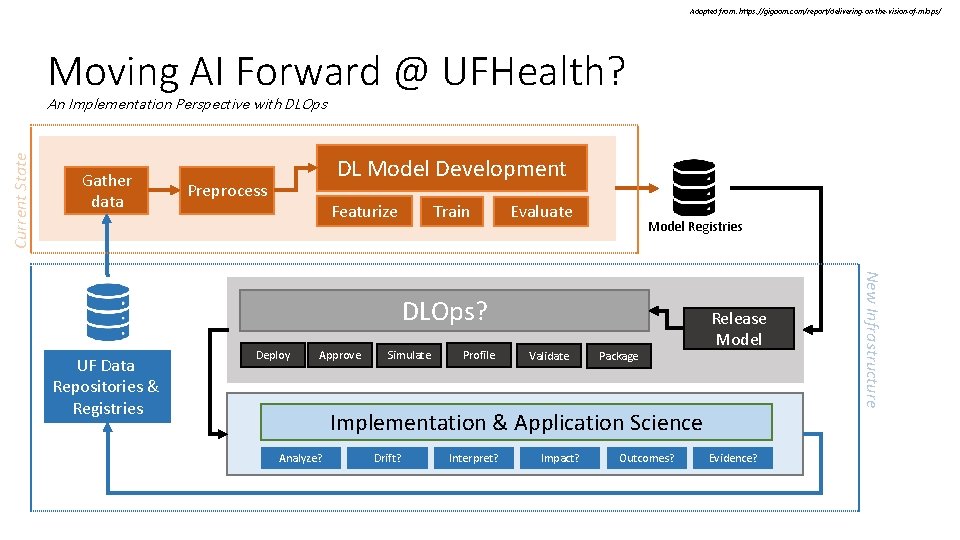
Adapted from: https: //gigaom. com/report/delivering-on-the-vision-of-mlops/ Moving AI Forward @ UFHealth? Current State An Implementation Perspective with DLOps Gather data DL Model Development Preprocess Featurize Train Evaluate Model Registries UF Data Repositories & Registries Deploy Approve Simulate Profile Validate Package Release Model Implementation & Application Science Analyze? Drift? Interpret? Impact? Outcomes? Evidence? New Infrastructure DLOps?
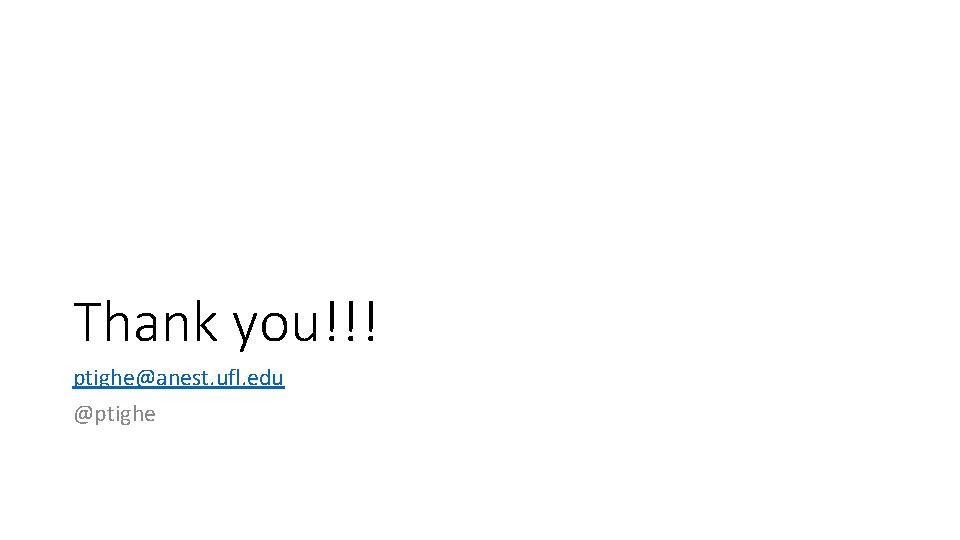
Thank you!!! ptighe@anest. ufl. edu @ptighe
Relational modeling vs dimensional modeling
Helen c. erickson
Thinking humanly: the cognitive modeling approach
Foul is fair and fair is foul literary device
Significa fair play
Oxymoron in macbeth
Examples of fair is foul and foul is fair in macbeth
Who says fair is foul and foul is fair
Sonnet modern
Syntax literary devices
Cognitive and non cognitive religious language
A set of systematically interrelated concepts
Understanding data and ways to systematically collect data
Perioperative case study