SAMPLING TECHNIQUES Shamindra Nath Sanyal 1152022 SNS 1
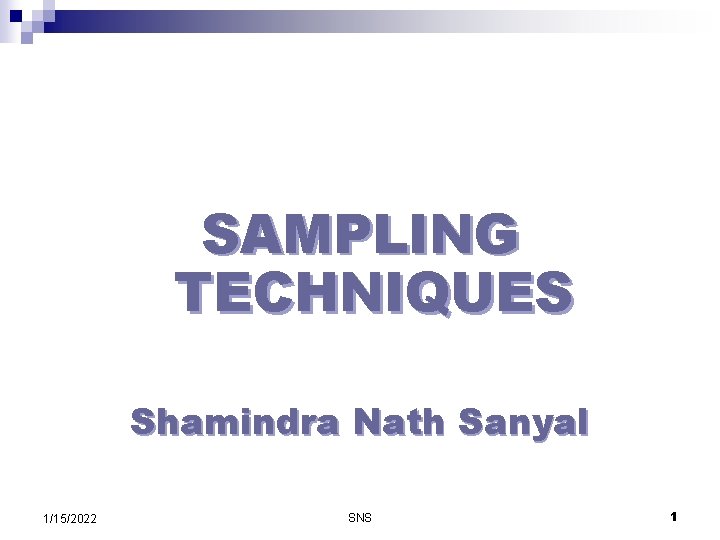
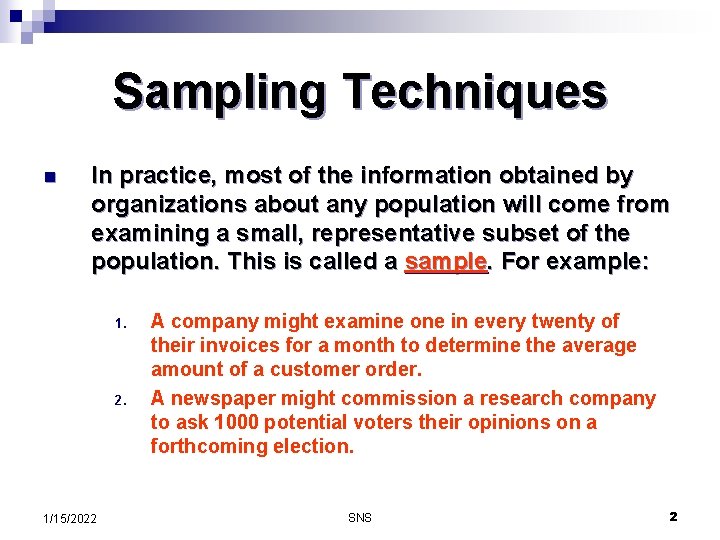
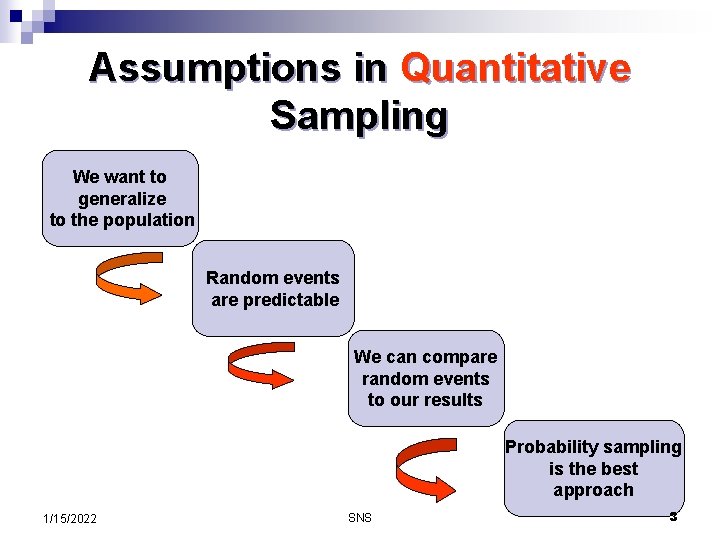
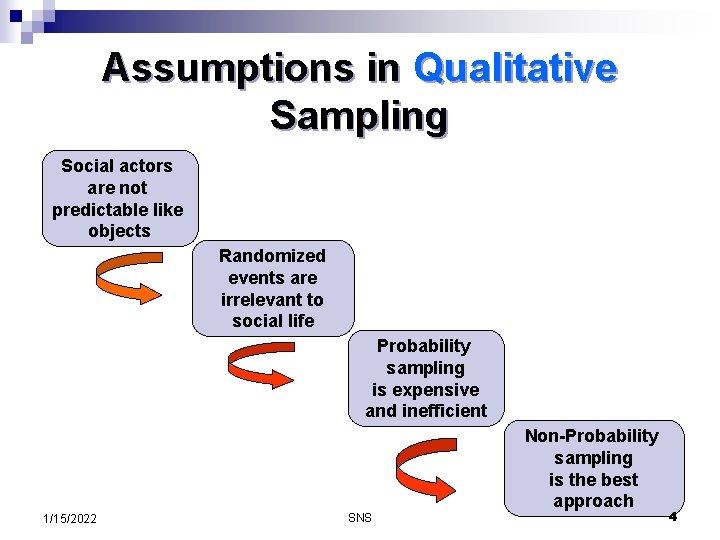
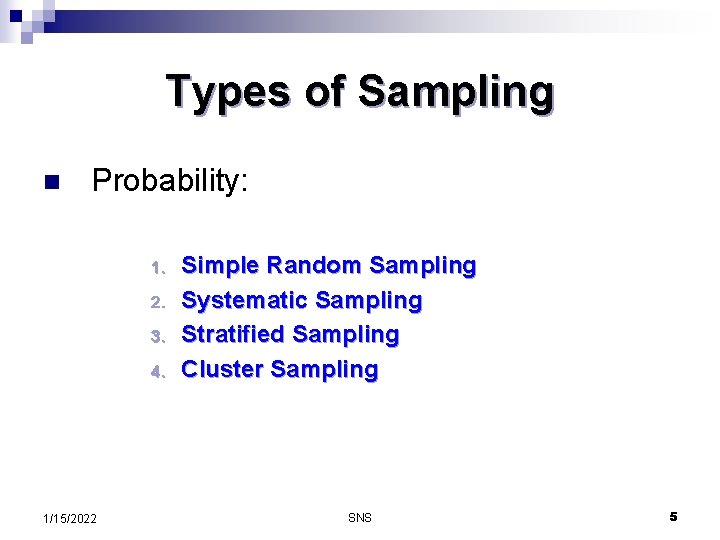
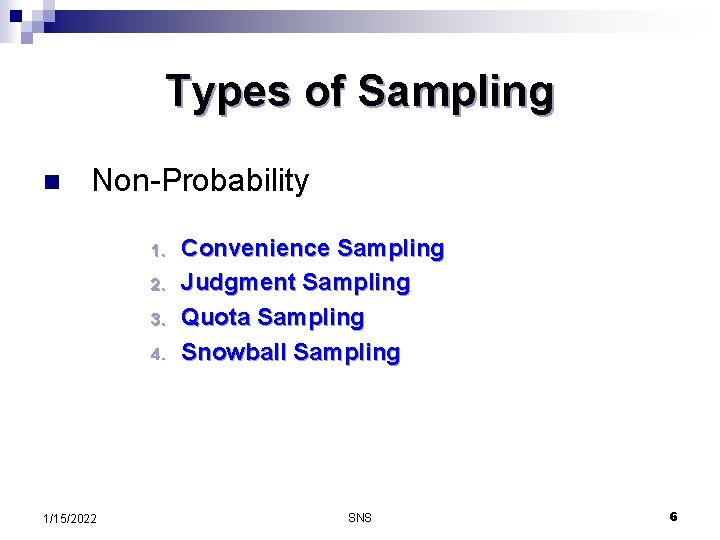
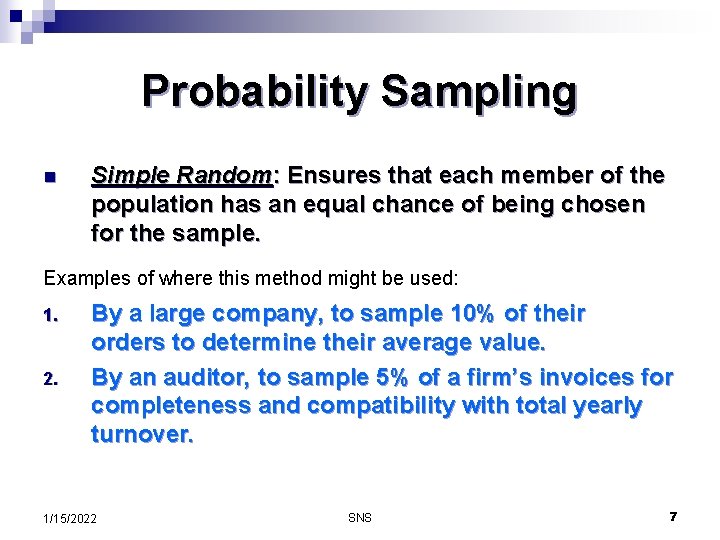
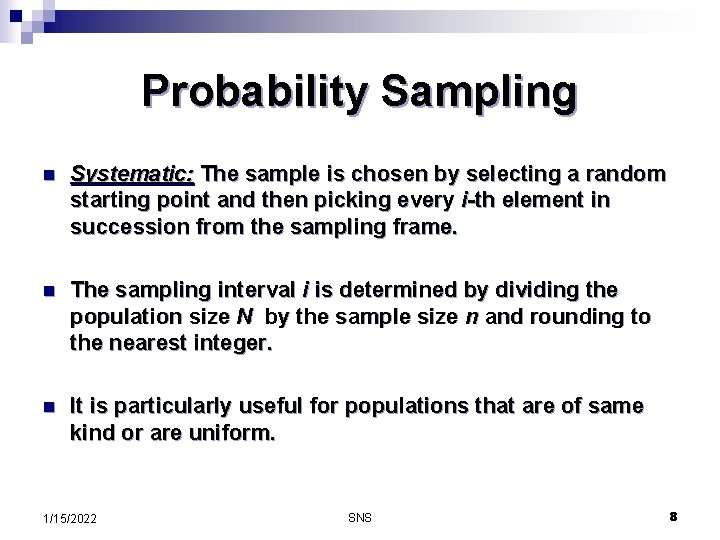
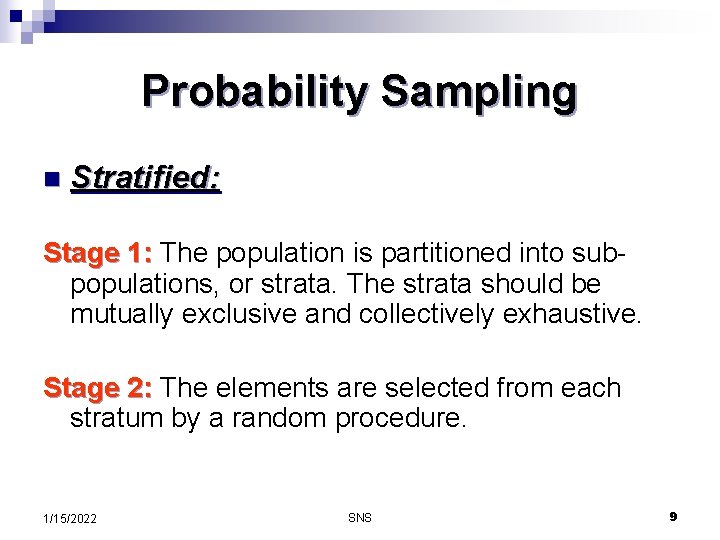
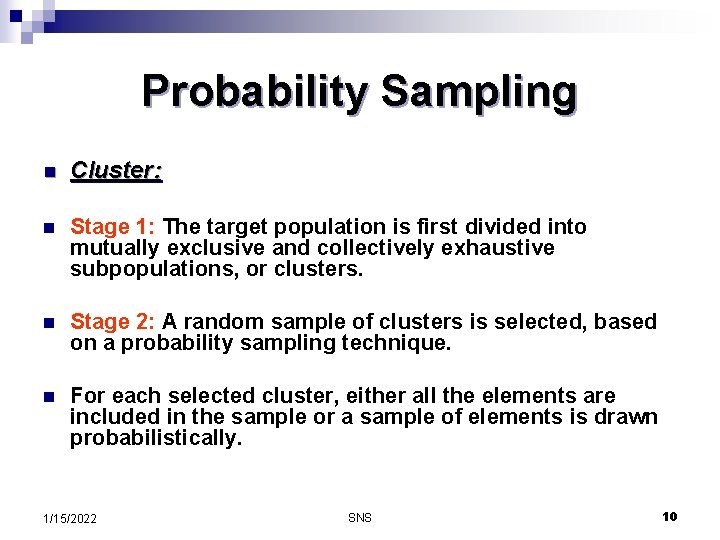
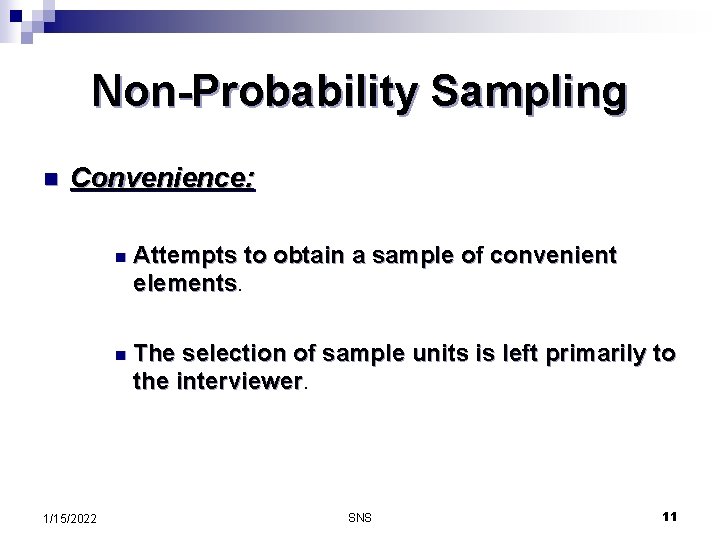
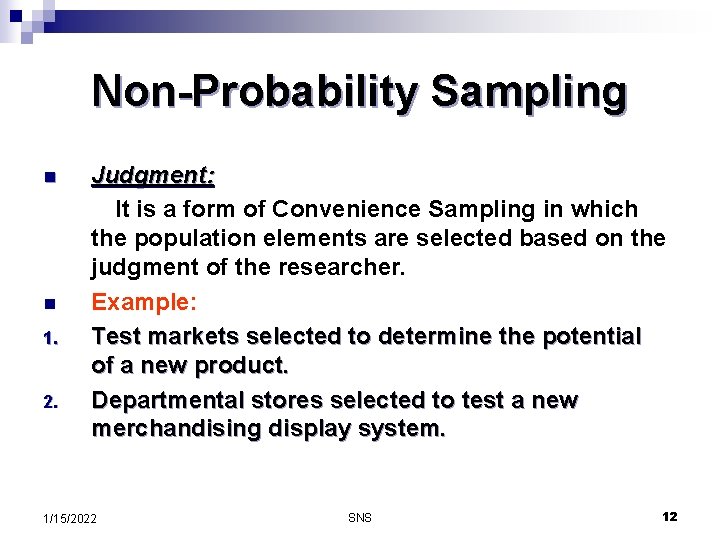
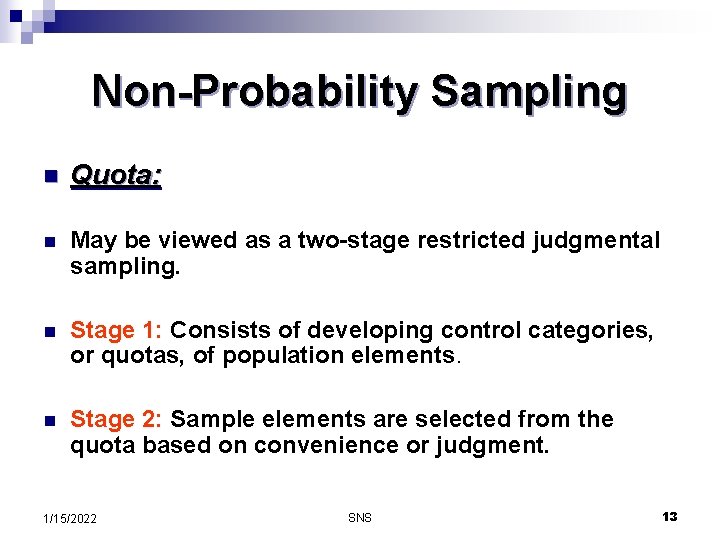
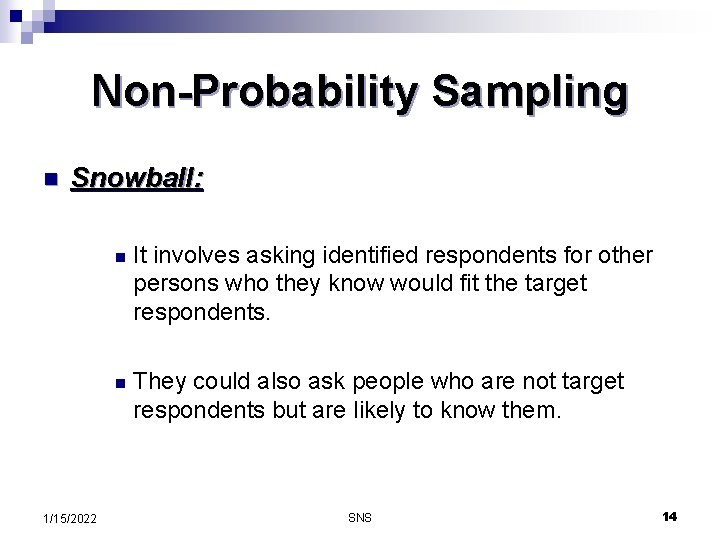
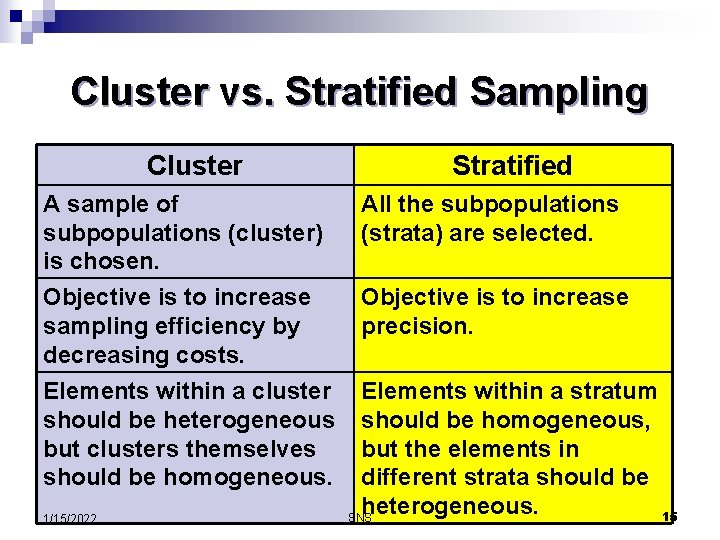
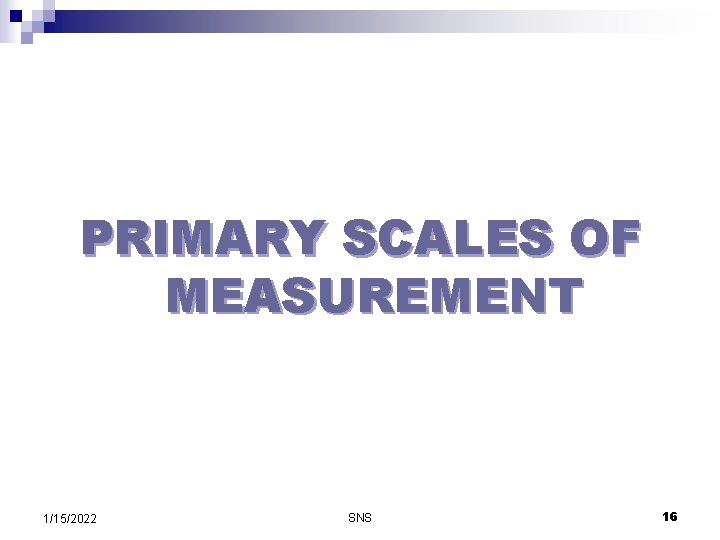
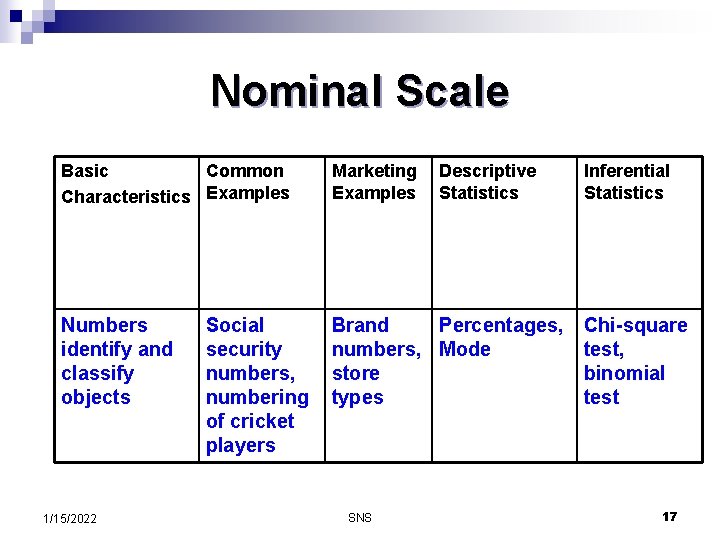
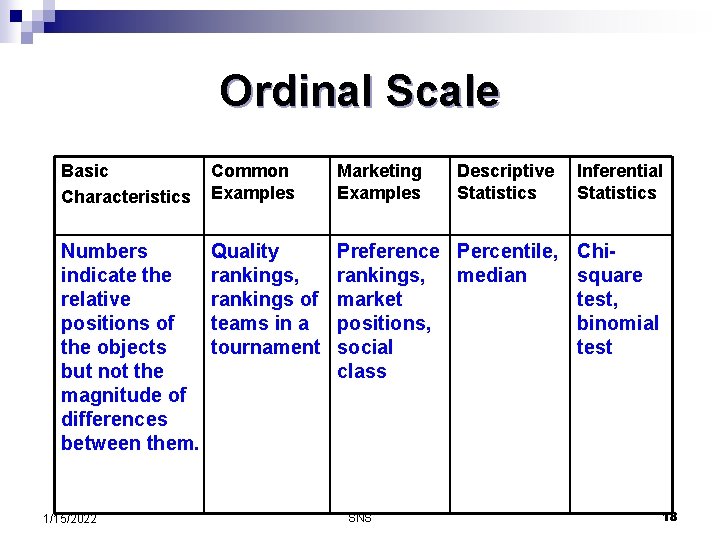
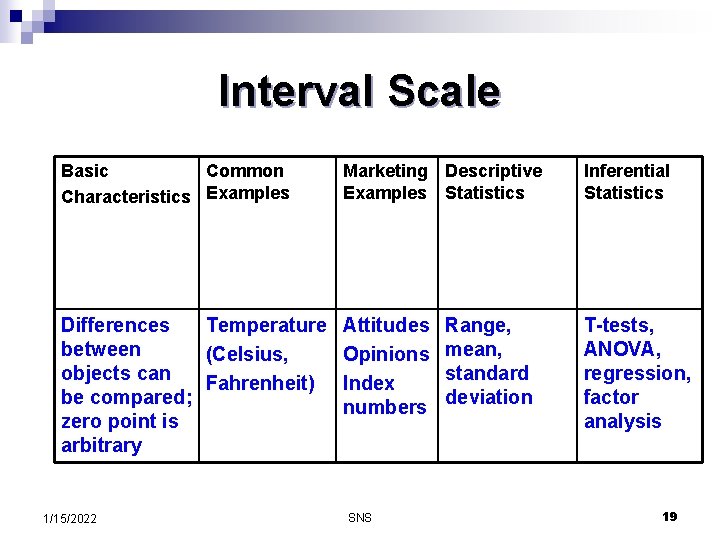
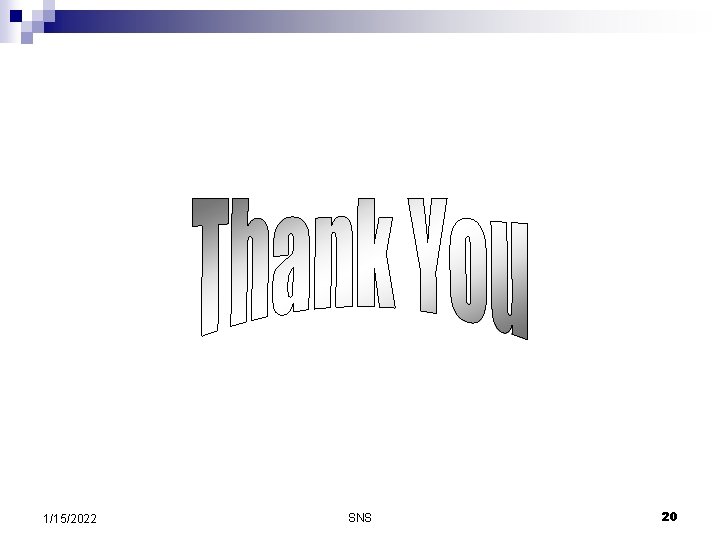
- Slides: 20
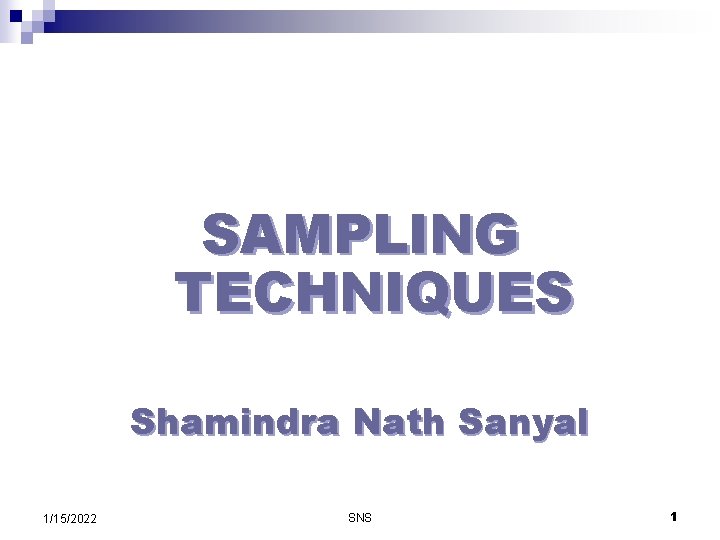
SAMPLING TECHNIQUES Shamindra Nath Sanyal 1/15/2022 SNS 1
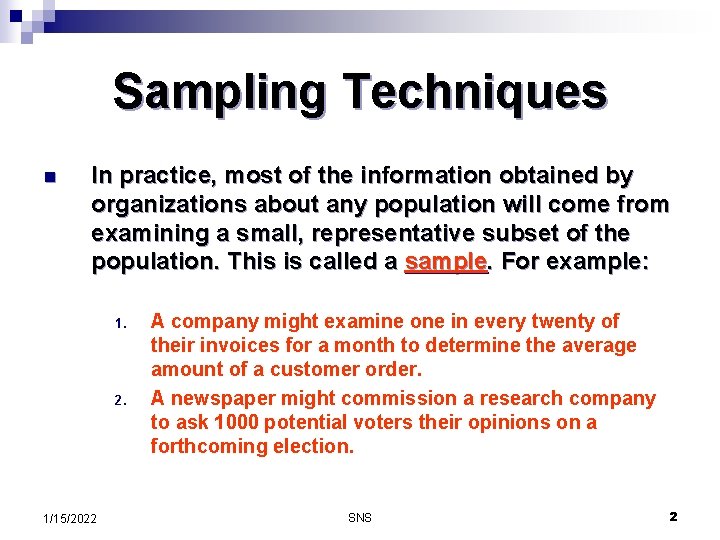
Sampling Techniques n In practice, most of the information obtained by organizations about any population will come from examining a small, representative subset of the population. This is called a sample. For example: 1. 2. 1/15/2022 A company might examine one in every twenty of their invoices for a month to determine the average amount of a customer order. A newspaper might commission a research company to ask 1000 potential voters their opinions on a forthcoming election. SNS 2
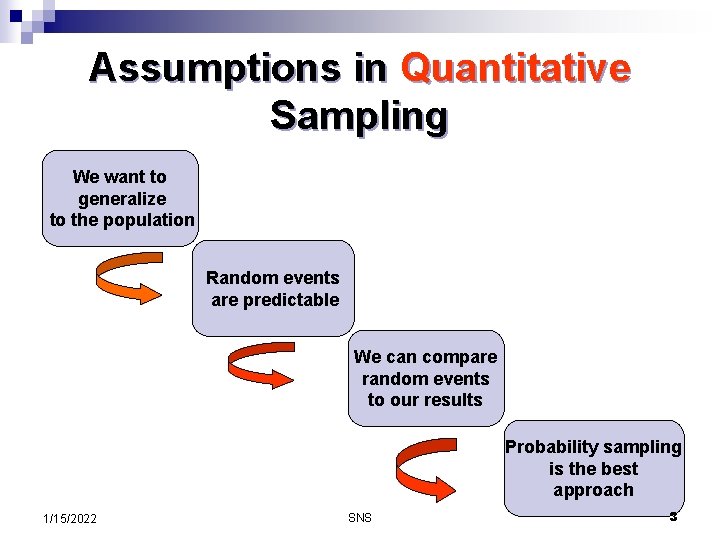
Assumptions in Quantitative Sampling We want to generalize to the population Random events are predictable We can compare random events to our results Probability sampling is the best approach 1/15/2022 SNS 3
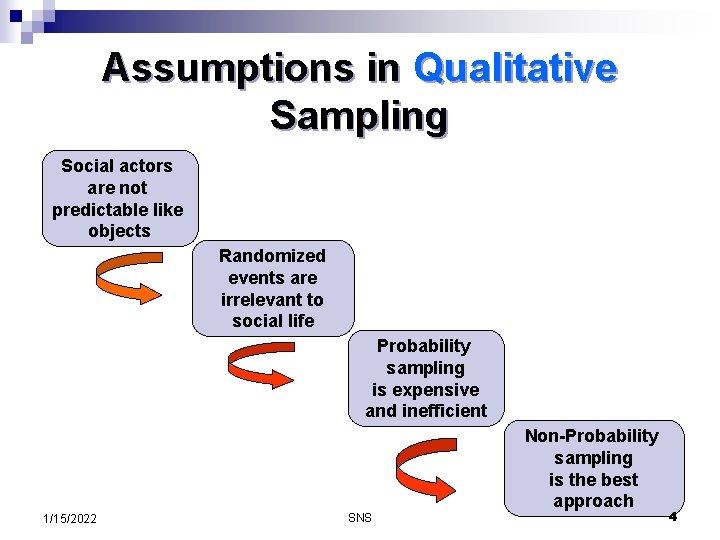
Assumptions in Qualitative Sampling Social actors are not predictable like objects Randomized events are irrelevant to social life Probability sampling is expensive and inefficient 1/15/2022 SNS Non-Probability sampling is the best approach 4
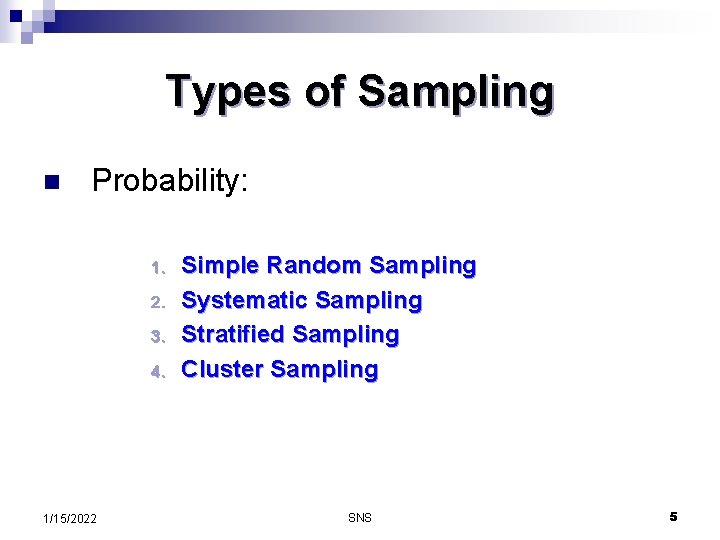
Types of Sampling n Probability: 1. 2. 3. 4. 1/15/2022 Simple Random Sampling Systematic Sampling Stratified Sampling Cluster Sampling SNS 5
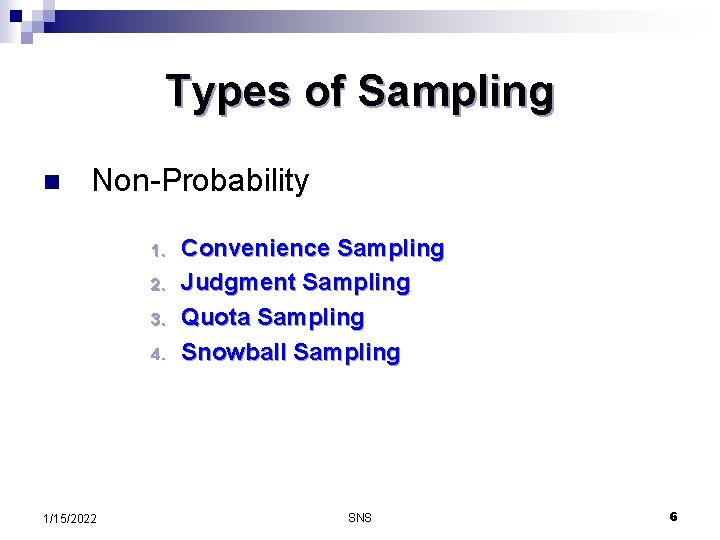
Types of Sampling n Non-Probability 1. 2. 3. 4. 1/15/2022 Convenience Sampling Judgment Sampling Quota Sampling Snowball Sampling SNS 6
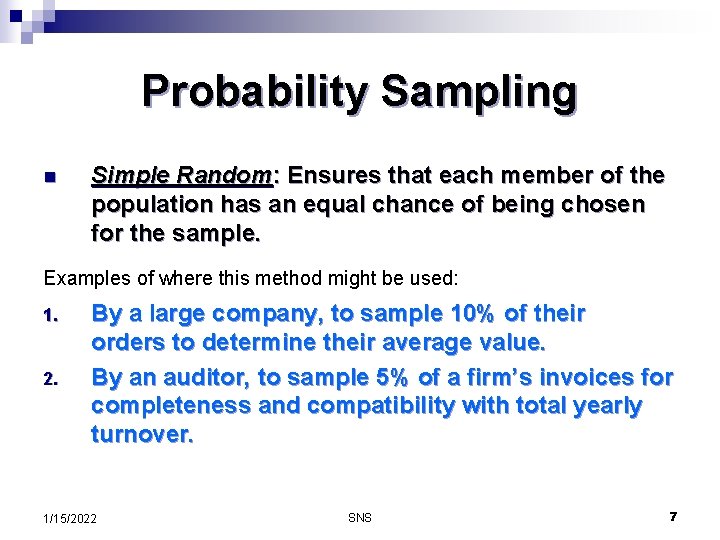
Probability Sampling n Simple Random: Ensures that each member of the population has an equal chance of being chosen for the sample. Examples of where this method might be used: 1. 2. By a large company, to sample 10% of their orders to determine their average value. By an auditor, to sample 5% of a firm’s invoices for completeness and compatibility with total yearly turnover. 1/15/2022 SNS 7
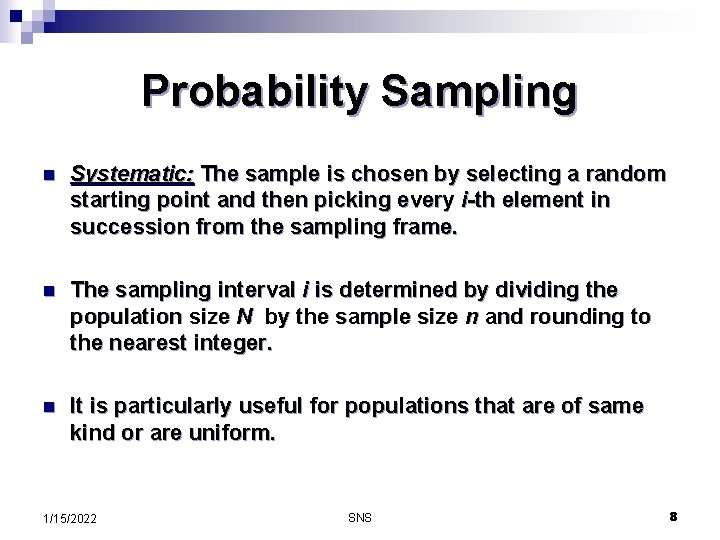
Probability Sampling n Systematic: The sample is chosen by selecting a random starting point and then picking every i-th element in succession from the sampling frame. n The sampling interval i is determined by dividing the population size N by the sample size n and rounding to the nearest integer. n It is particularly useful for populations that are of same kind or are uniform. 1/15/2022 SNS 8
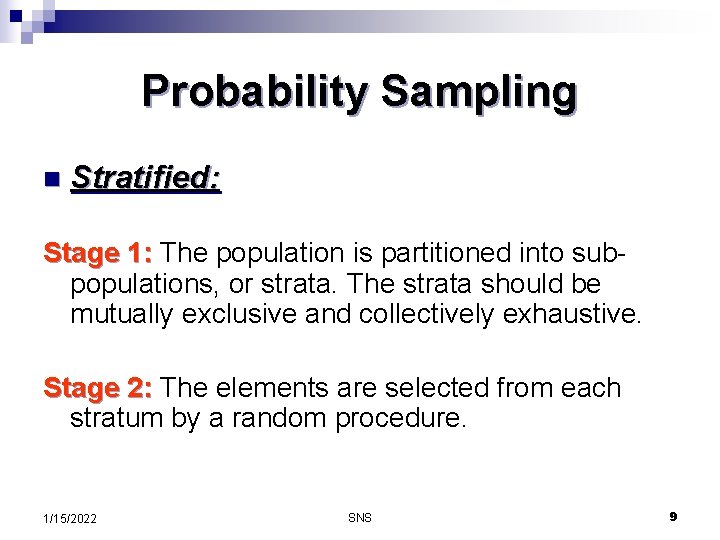
Probability Sampling n Stratified: Stage 1: The population is partitioned into subpopulations, or strata. The strata should be mutually exclusive and collectively exhaustive. Stage 2: The elements are selected from each stratum by a random procedure. 1/15/2022 SNS 9
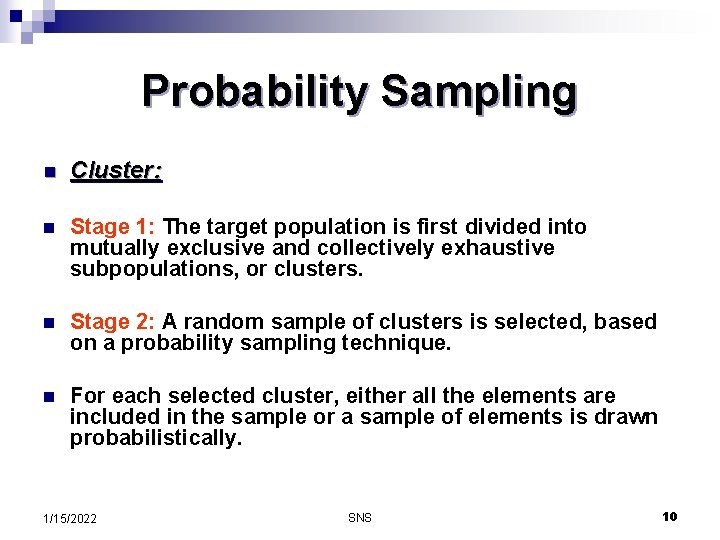
Probability Sampling n Cluster: n Stage 1: The target population is first divided into mutually exclusive and collectively exhaustive subpopulations, or clusters. n Stage 2: A random sample of clusters is selected, based on a probability sampling technique. n For each selected cluster, either all the elements are included in the sample or a sample of elements is drawn probabilistically. 1/15/2022 SNS 10
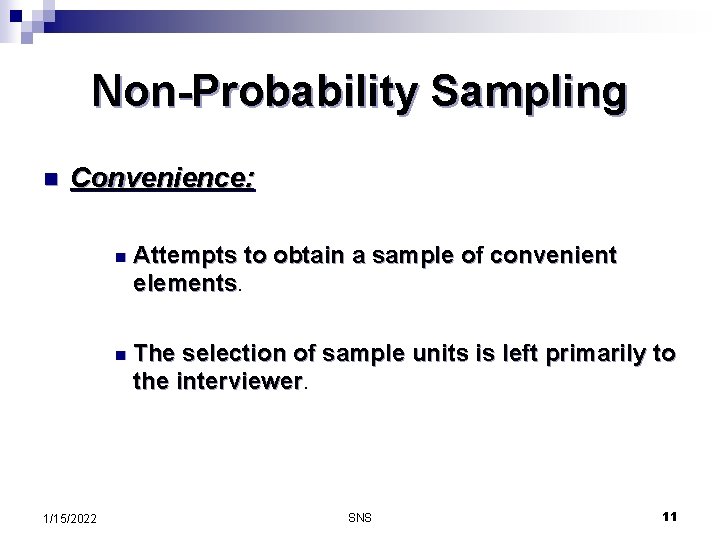
Non-Probability Sampling n Convenience: 1/15/2022 n Attempts to obtain a sample of convenient elements n The selection of sample units is left primarily to the interviewer SNS 11
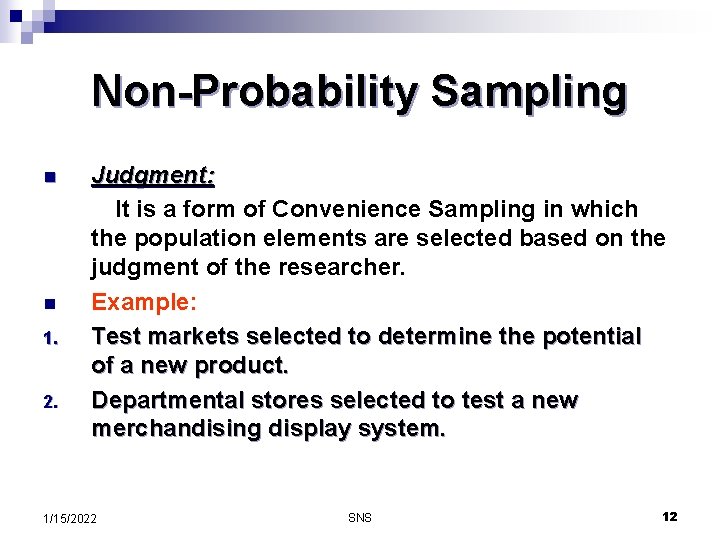
Non-Probability Sampling n n 1. 2. Judgment: It is a form of Convenience Sampling in which the population elements are selected based on the judgment of the researcher. Example: Test markets selected to determine the potential of a new product. Departmental stores selected to test a new merchandising display system. 1/15/2022 SNS 12
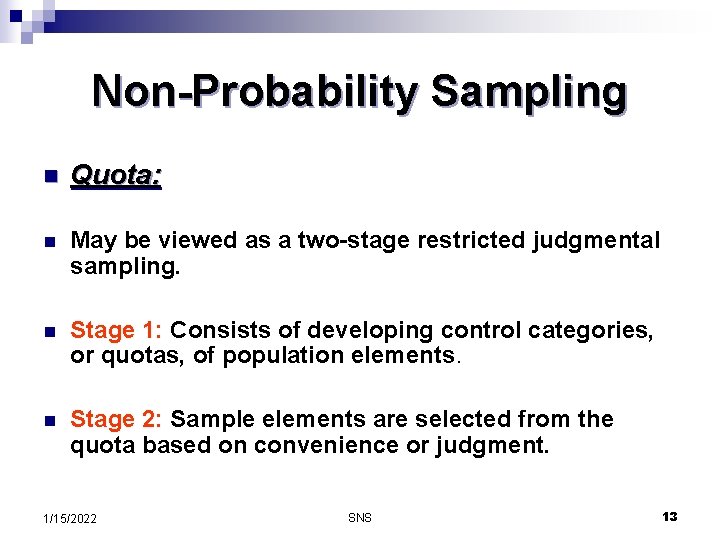
Non-Probability Sampling n Quota: n May be viewed as a two-stage restricted judgmental sampling. n Stage 1: Consists of developing control categories, or quotas, of population elements. n Stage 2: Sample elements are selected from the quota based on convenience or judgment. 1/15/2022 SNS 13
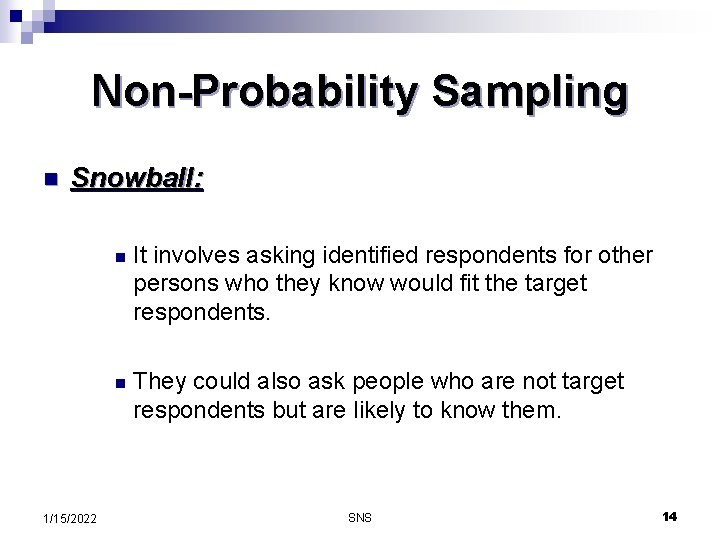
Non-Probability Sampling n Snowball: 1/15/2022 n It involves asking identified respondents for other persons who they know would fit the target respondents. n They could also ask people who are not target respondents but are likely to know them. SNS 14
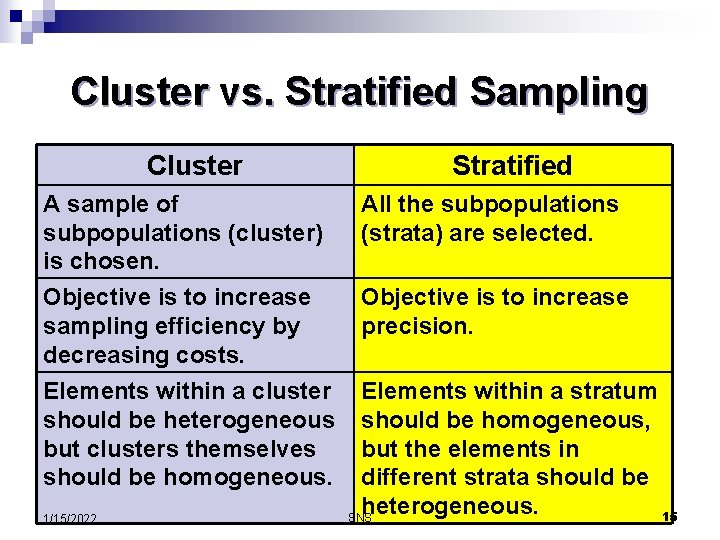
Cluster vs. Stratified Sampling Cluster A sample of subpopulations (cluster) is chosen. Objective is to increase sampling efficiency by decreasing costs. Elements within a cluster should be heterogeneous but clusters themselves should be homogeneous. 1/15/2022 Stratified All the subpopulations (strata) are selected. Objective is to increase precision. Elements within a stratum should be homogeneous, but the elements in different strata should be heterogeneous. 15 SNS
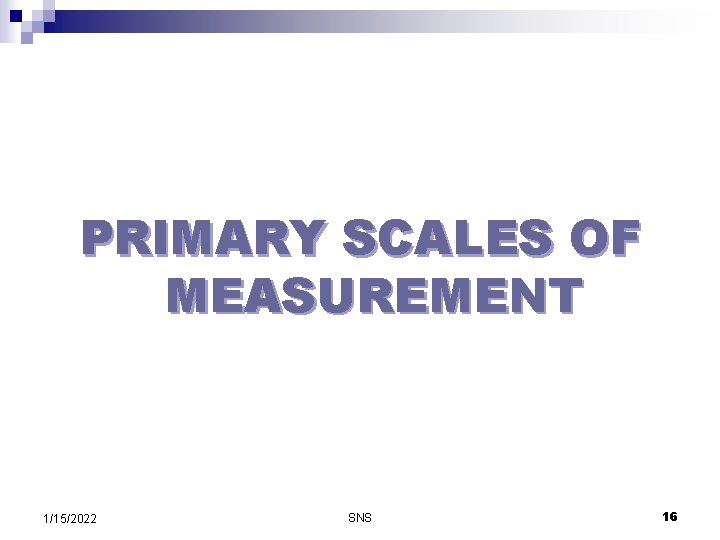
PRIMARY SCALES OF MEASUREMENT 1/15/2022 SNS 16
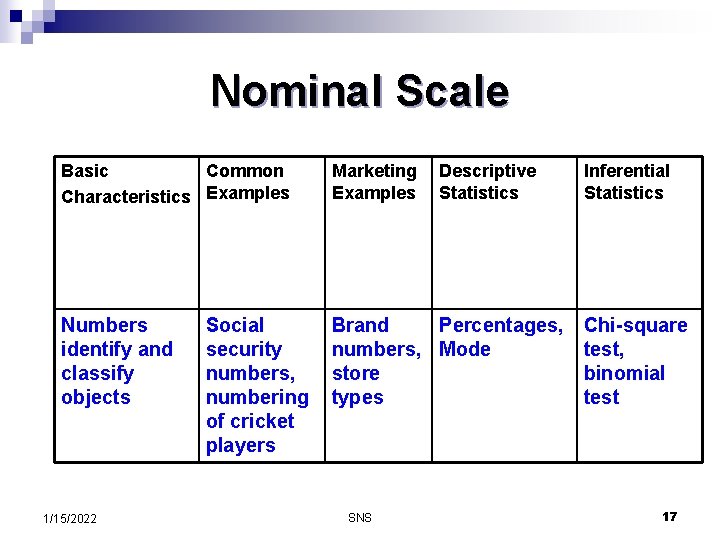
Nominal Scale Basic Common Characteristics Examples Marketing Examples Numbers identify and classify objects Brand Percentages, numbers, Mode store types 1/15/2022 Social security numbers, numbering of cricket players SNS Descriptive Statistics Inferential Statistics Chi-square test, binomial test 17
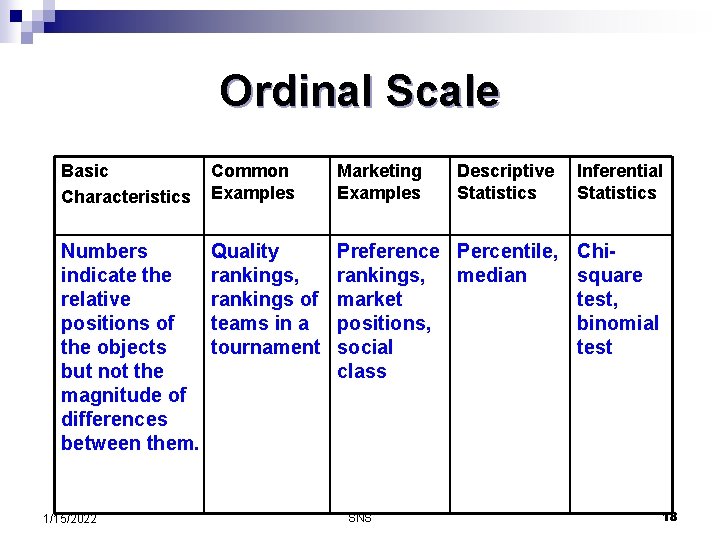
Ordinal Scale Basic Characteristics Common Examples Marketing Examples Numbers indicate the relative positions of the objects but not the magnitude of differences between them. Quality rankings, rankings of teams in a tournament Preference Percentile, Chirankings, median square market test, positions, binomial social test class 1/15/2022 SNS Descriptive Statistics Inferential Statistics 18
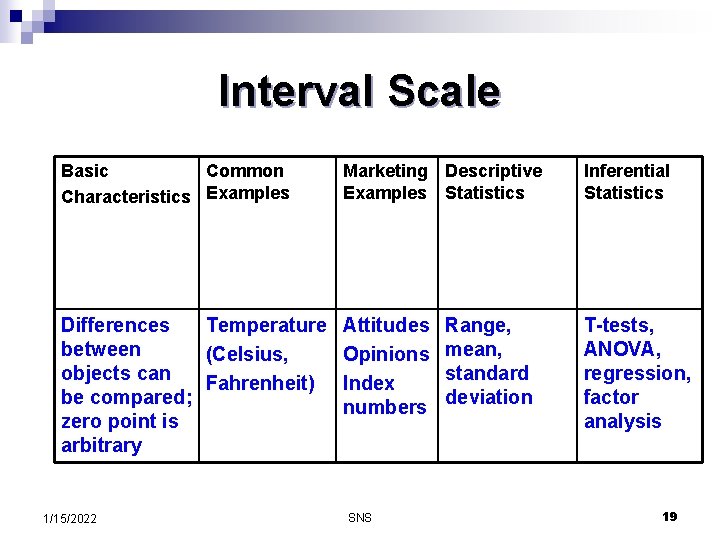
Interval Scale Basic Common Characteristics Examples Marketing Descriptive Examples Statistics Differences Temperature Attitudes Range, between (Celsius, Opinions mean, objects can standard Fahrenheit) Index be compared; numbers deviation zero point is arbitrary 1/15/2022 SNS Inferential Statistics T-tests, ANOVA, regression, factor analysis 19
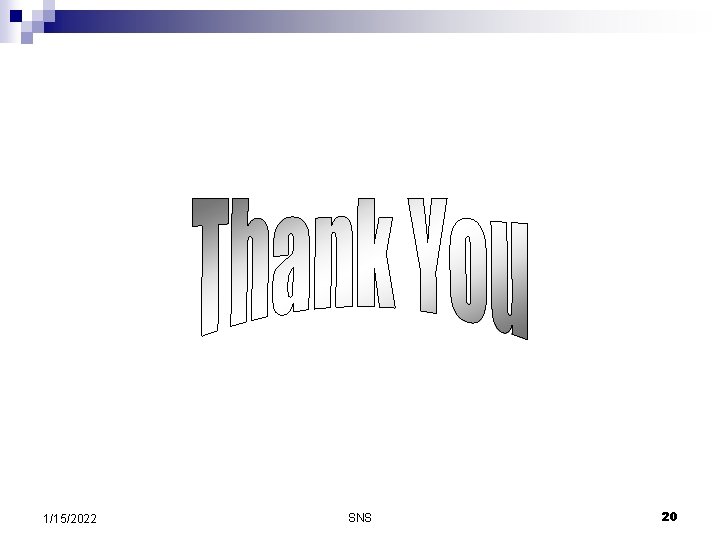
1/15/2022 SNS 20
Shamindra nath sanyal
Yatindra nath singh
Dr p umanath ias
Bose einstein condensate definition chemistry
Arindam nath
Kedarnath gupta dubai
Doll's eye
Dr reshmi nath
Somatic nervous system (sns)
Sns vision
Sns. gov. pt
Narin acapella
Psns and sns
Scottish neighbourhood statistics
Matplotlib bokeh
Cns and sns
Postganglionic vs preganglionic
Sns workshop
Sns
Aws batch icon
I was not aware