PAKDD Panel What Next Ramakrishnan Srikant What Next
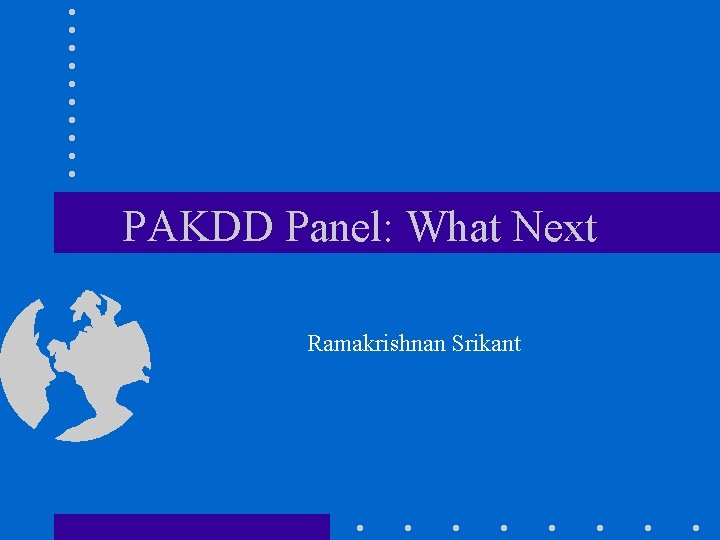
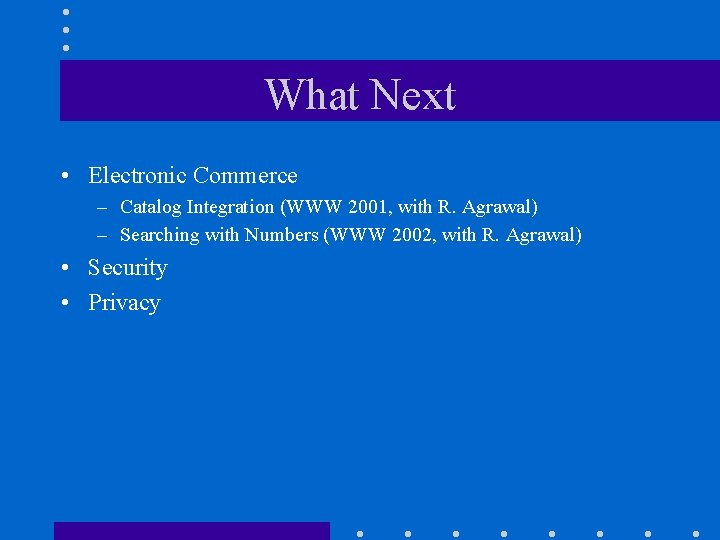
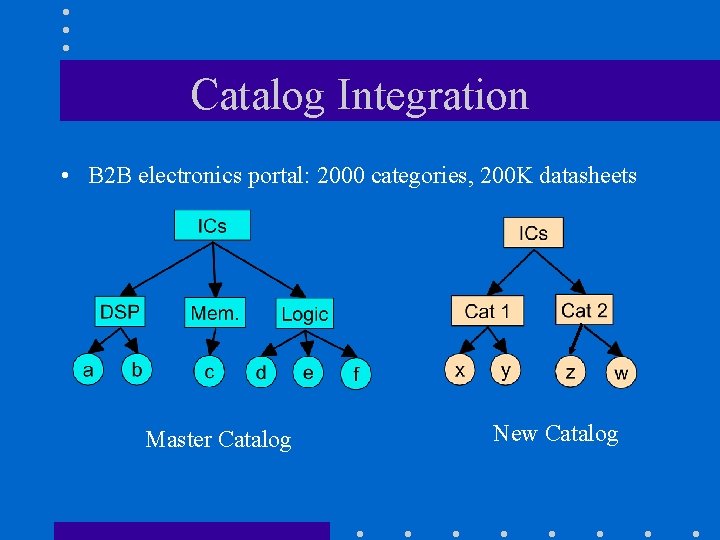
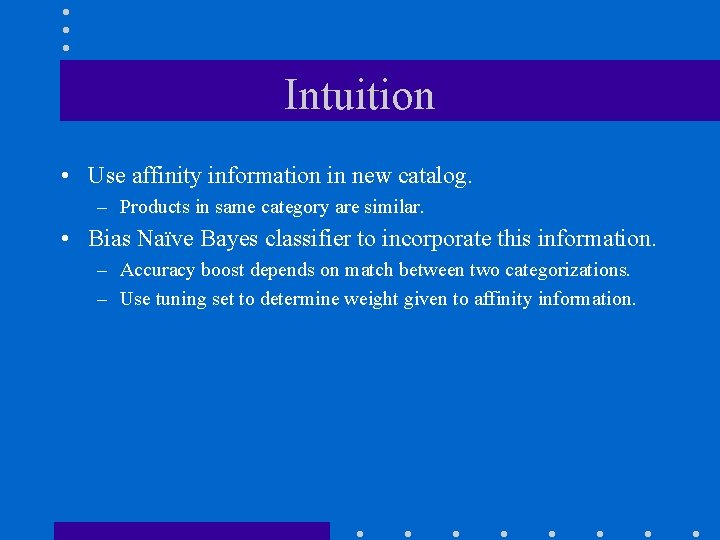
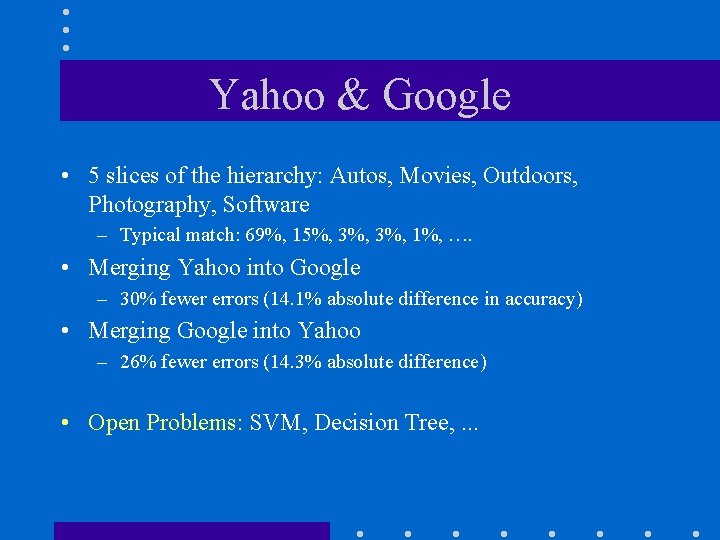
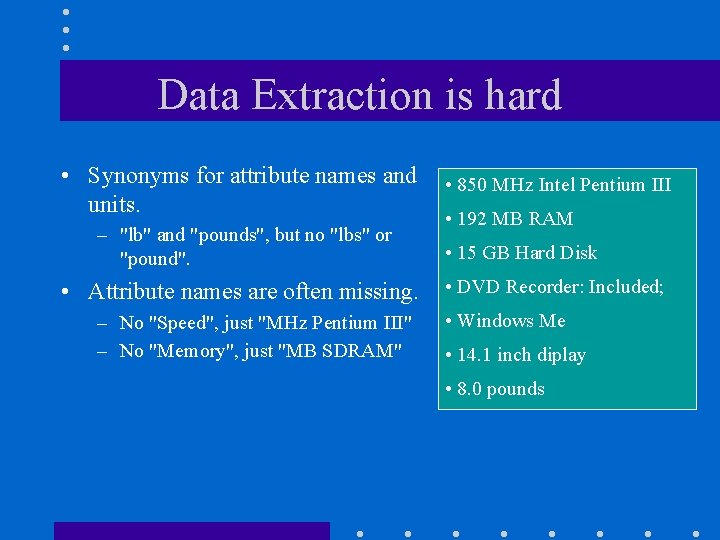
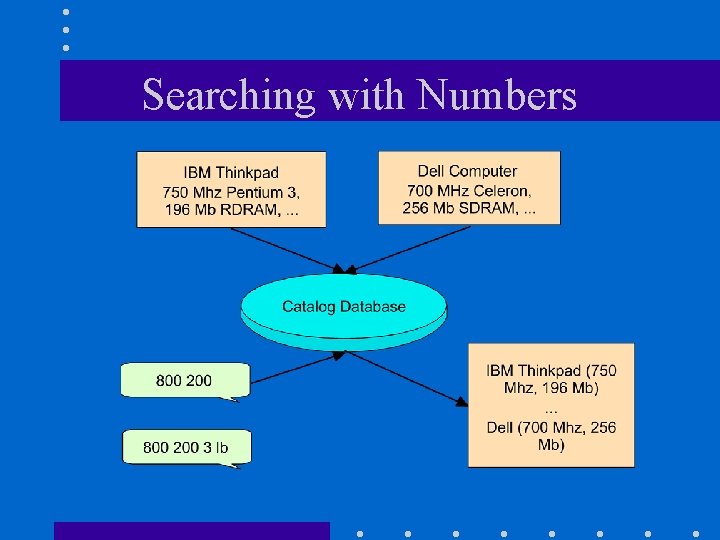
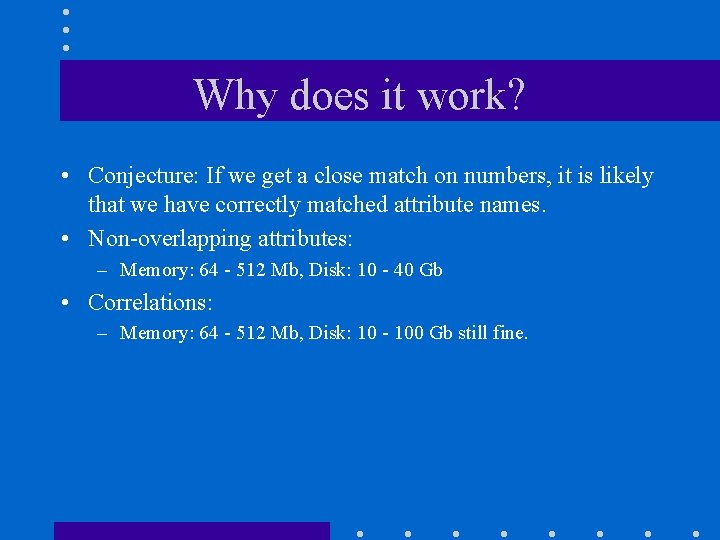
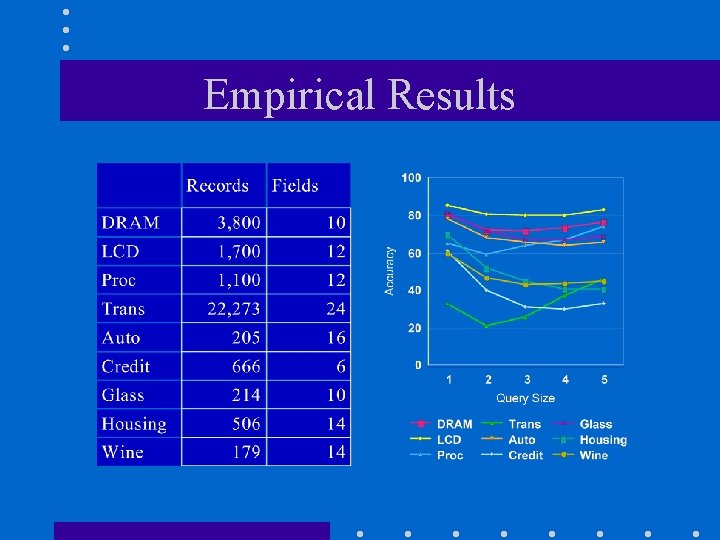
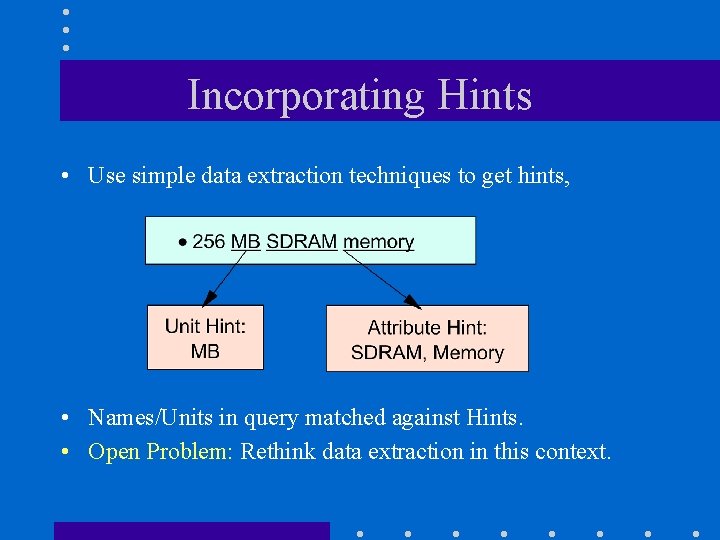
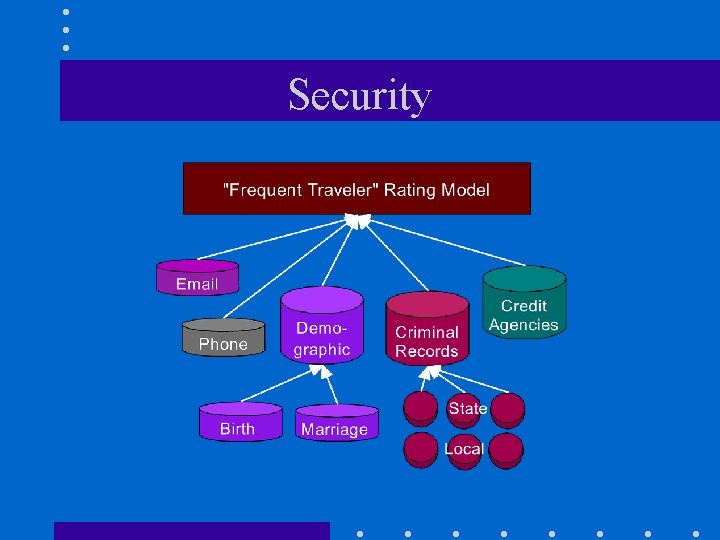
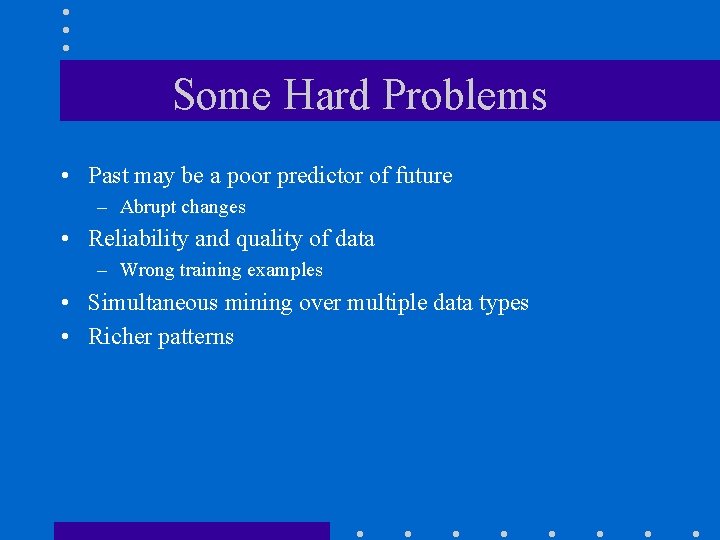
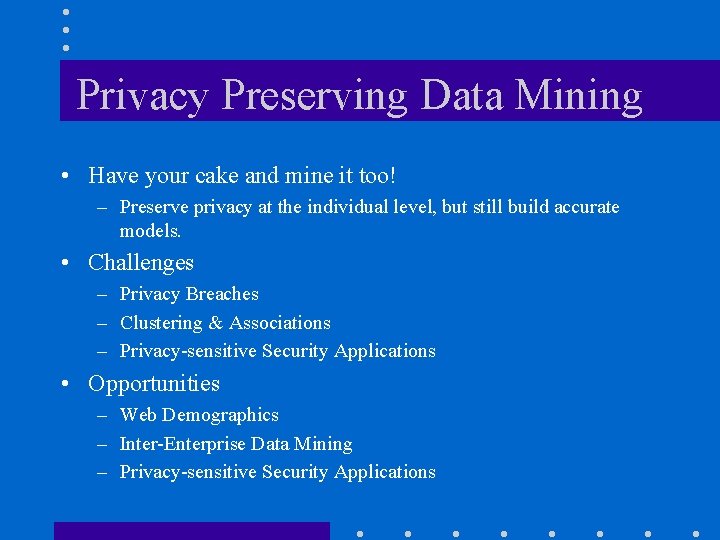
- Slides: 13
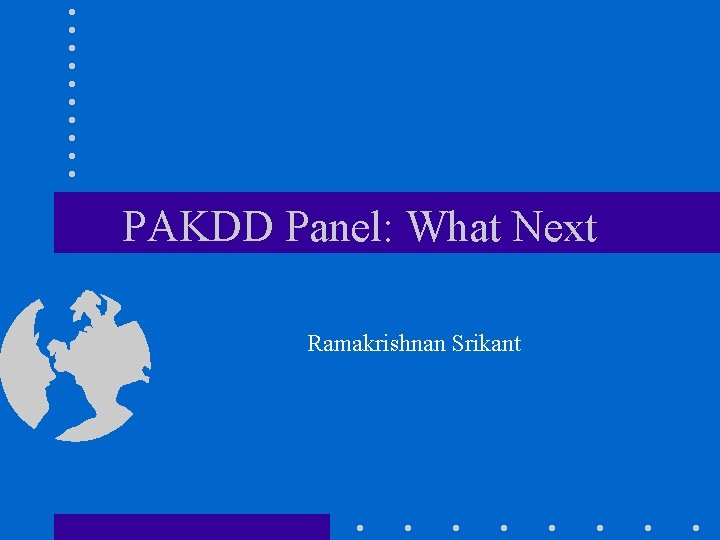
PAKDD Panel: What Next Ramakrishnan Srikant
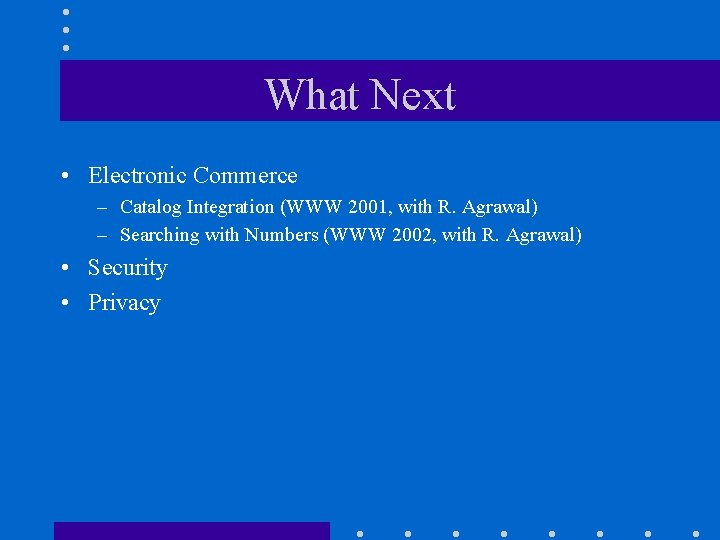
What Next • Electronic Commerce – Catalog Integration (WWW 2001, with R. Agrawal) – Searching with Numbers (WWW 2002, with R. Agrawal) • Security • Privacy
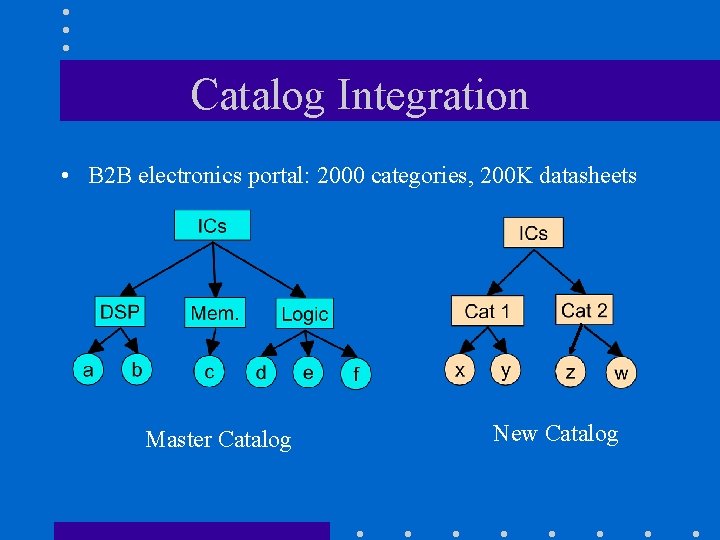
Catalog Integration • B 2 B electronics portal: 2000 categories, 200 K datasheets Master Catalog New Catalog
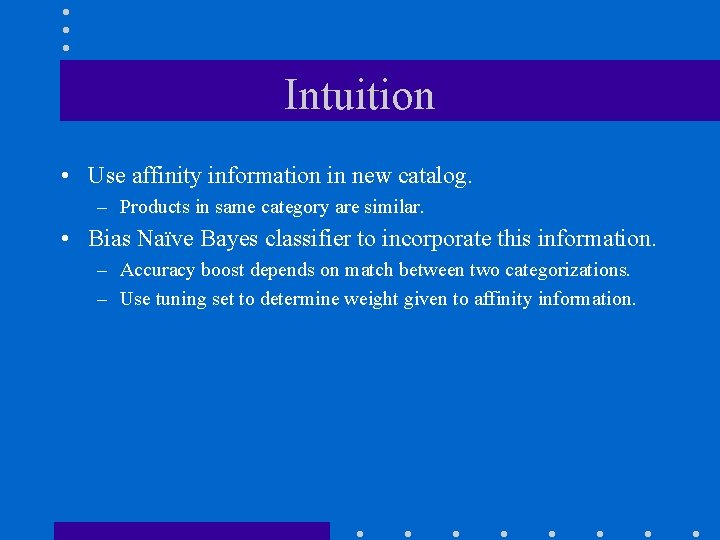
Intuition • Use affinity information in new catalog. – Products in same category are similar. • Bias Naïve Bayes classifier to incorporate this information. – Accuracy boost depends on match between two categorizations. – Use tuning set to determine weight given to affinity information.
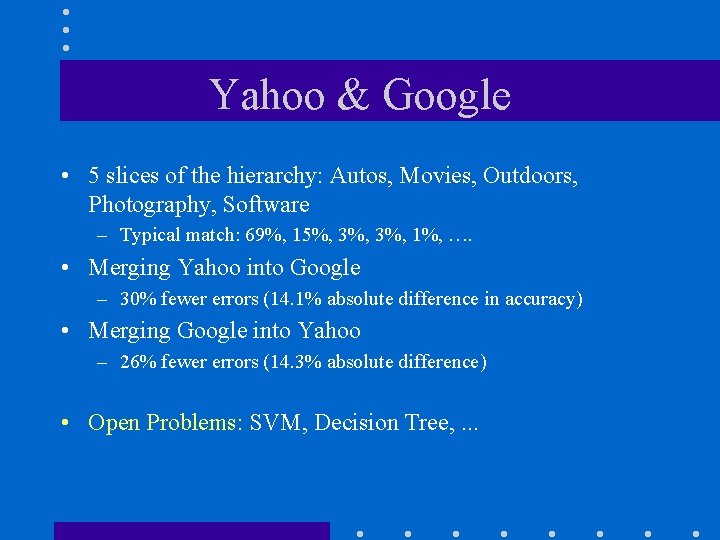
Yahoo & Google • 5 slices of the hierarchy: Autos, Movies, Outdoors, Photography, Software – Typical match: 69%, 15%, 3%, 1%, …. • Merging Yahoo into Google – 30% fewer errors (14. 1% absolute difference in accuracy) • Merging Google into Yahoo – 26% fewer errors (14. 3% absolute difference) • Open Problems: SVM, Decision Tree, . . .
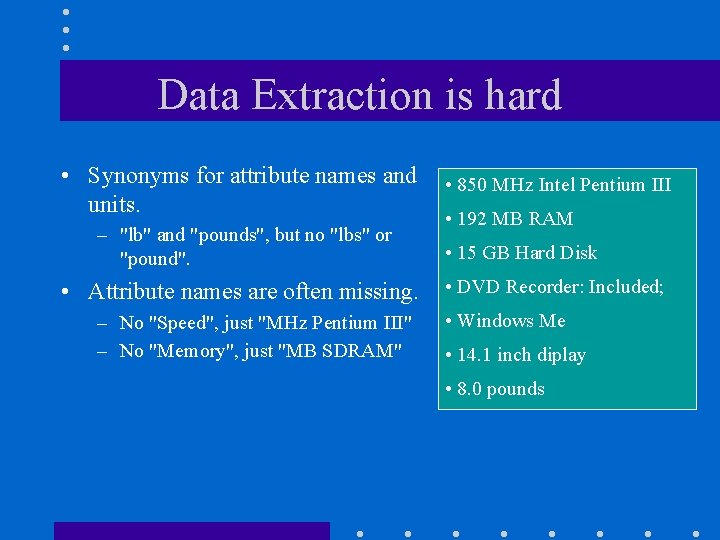
Data Extraction is hard • Synonyms for attribute names and units. – "lb" and "pounds", but no "lbs" or "pound". • Attribute names are often missing. – No "Speed", just "MHz Pentium III" – No "Memory", just "MB SDRAM" • 850 MHz Intel Pentium III • 192 MB RAM • 15 GB Hard Disk • DVD Recorder: Included; • Windows Me • 14. 1 inch diplay • 8. 0 pounds
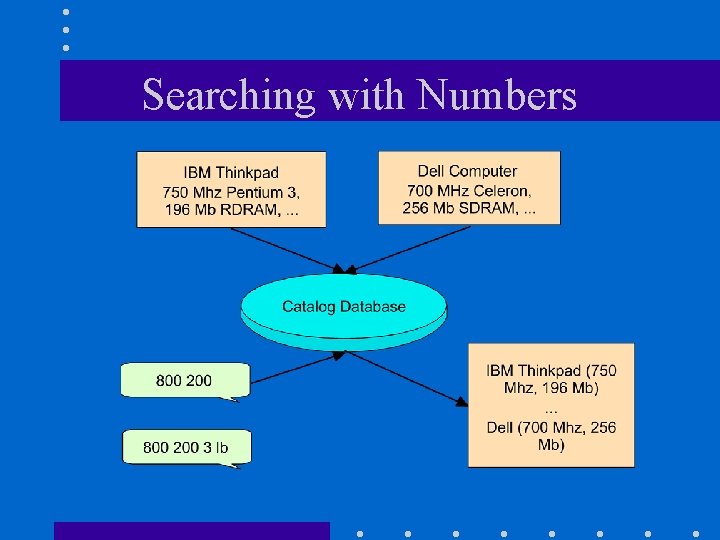
Searching with Numbers
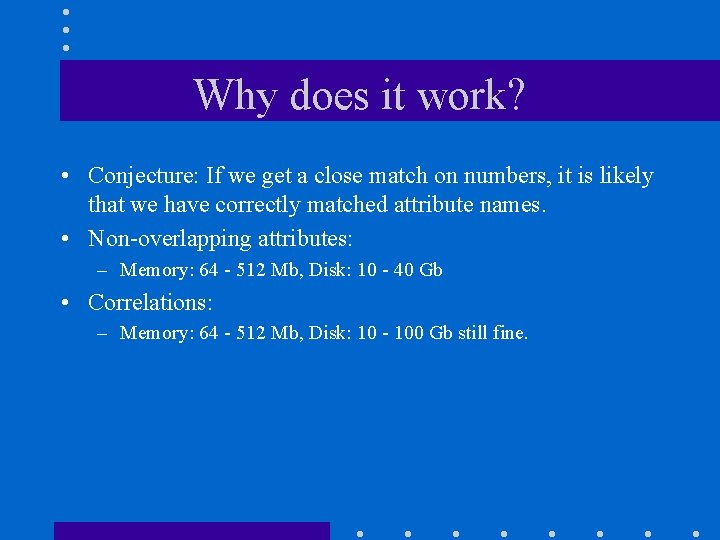
Why does it work? • Conjecture: If we get a close match on numbers, it is likely that we have correctly matched attribute names. • Non-overlapping attributes: – Memory: 64 - 512 Mb, Disk: 10 - 40 Gb • Correlations: – Memory: 64 - 512 Mb, Disk: 10 - 100 Gb still fine.
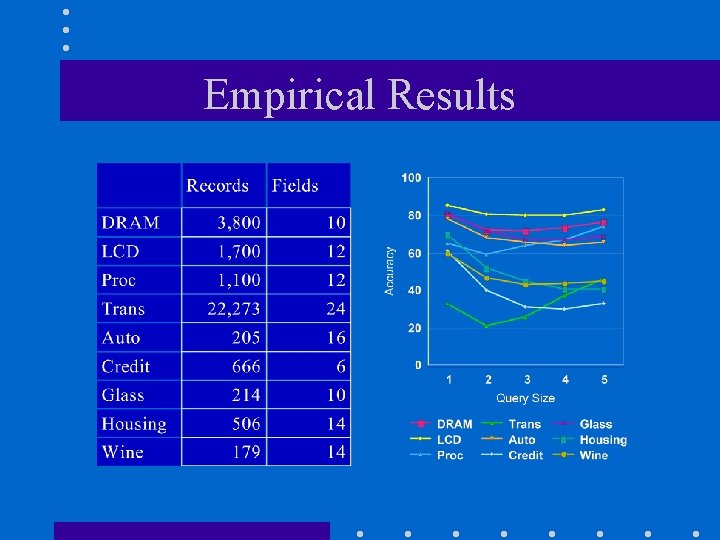
Empirical Results
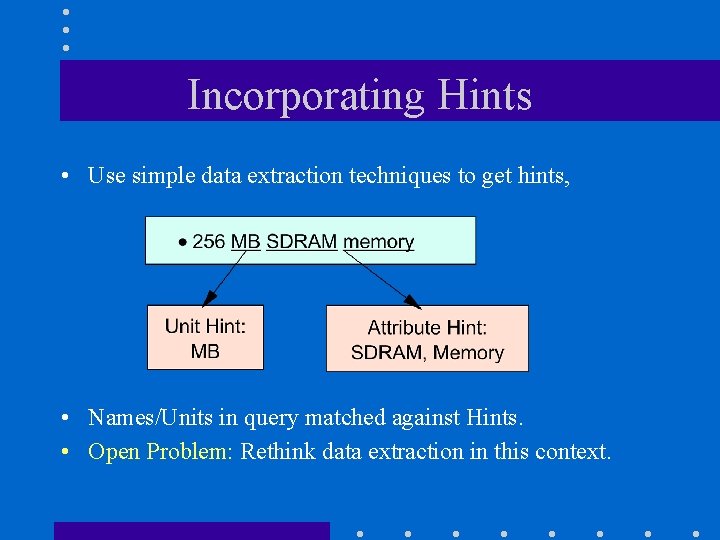
Incorporating Hints • Use simple data extraction techniques to get hints, • Names/Units in query matched against Hints. • Open Problem: Rethink data extraction in this context.
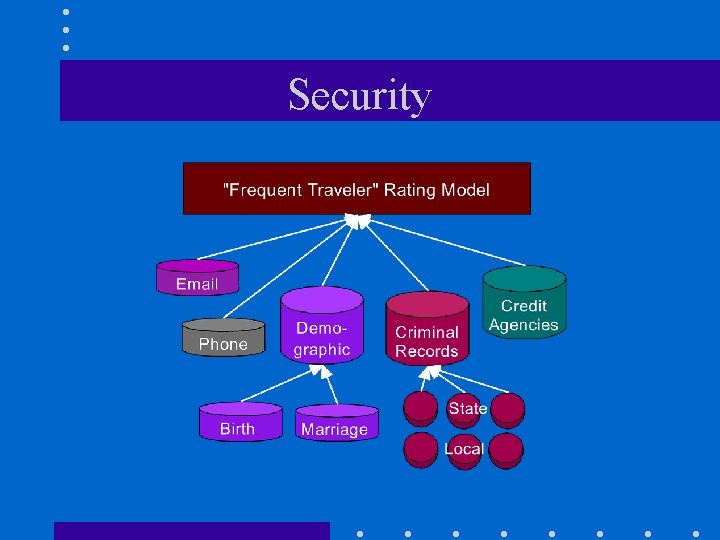
Security
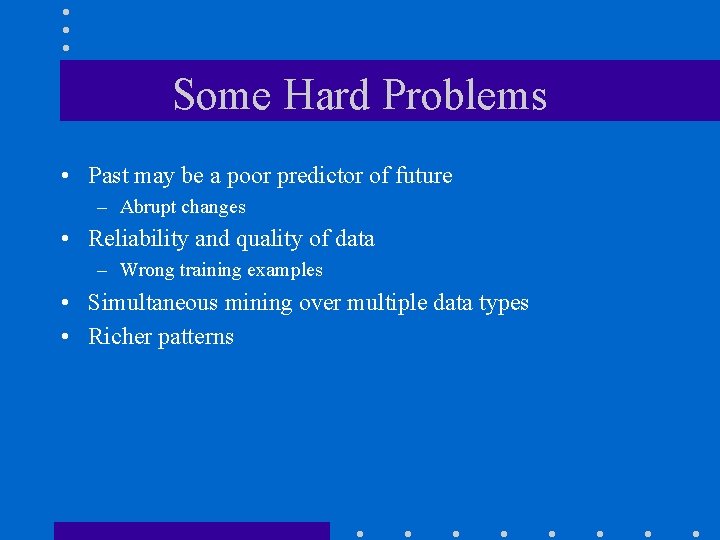
Some Hard Problems • Past may be a poor predictor of future – Abrupt changes • Reliability and quality of data – Wrong training examples • Simultaneous mining over multiple data types • Richer patterns
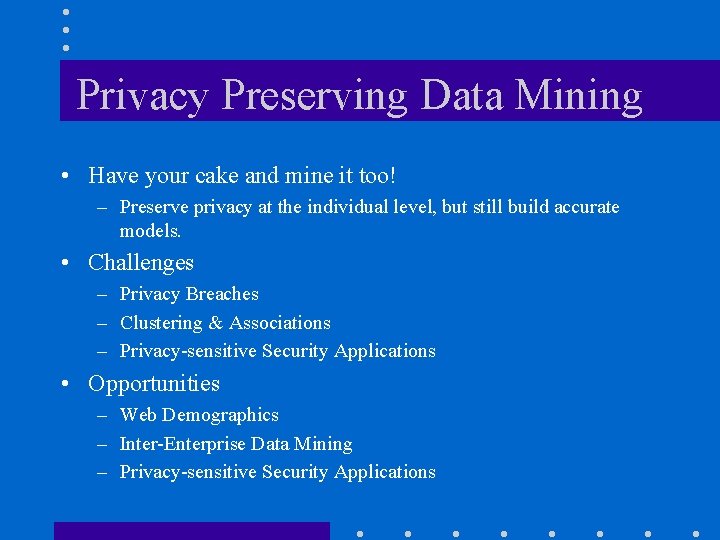
Privacy Preserving Data Mining • Have your cake and mine it too! – Preserve privacy at the individual level, but still build accurate models. • Challenges – Privacy Breaches – Clustering & Associations – Privacy-sensitive Security Applications • Opportunities – Web Demographics – Inter-Enterprise Data Mining – Privacy-sensitive Security Applications