Modeling and Simulation of a LongWave Infrared Polarimetric
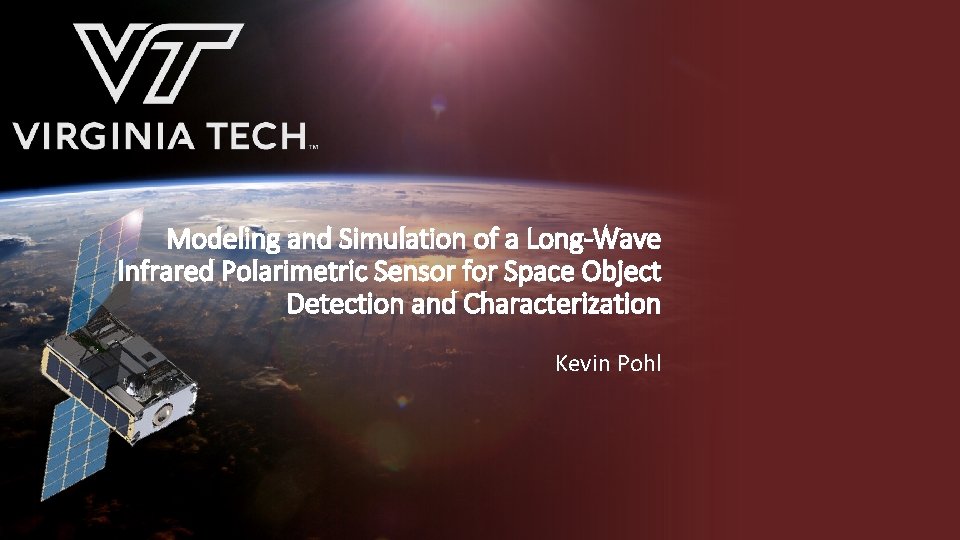
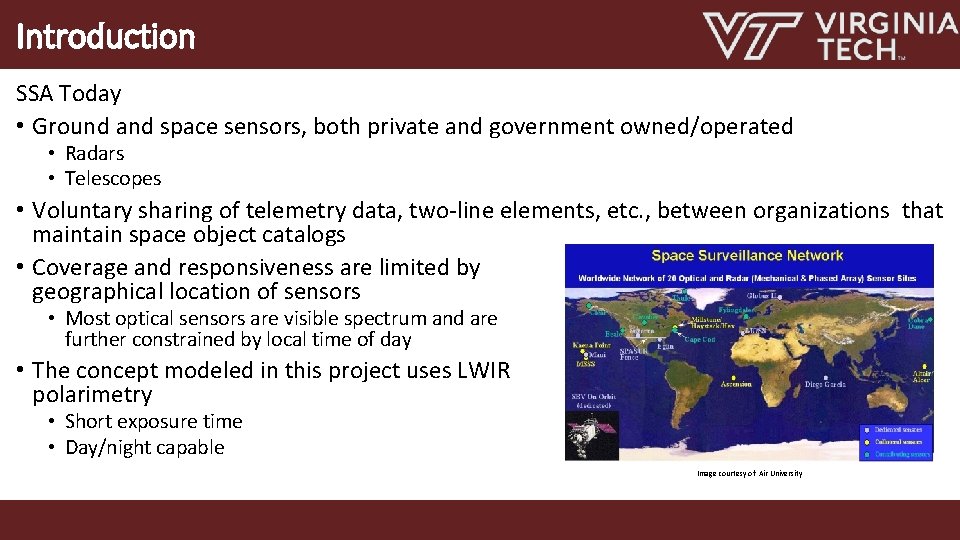
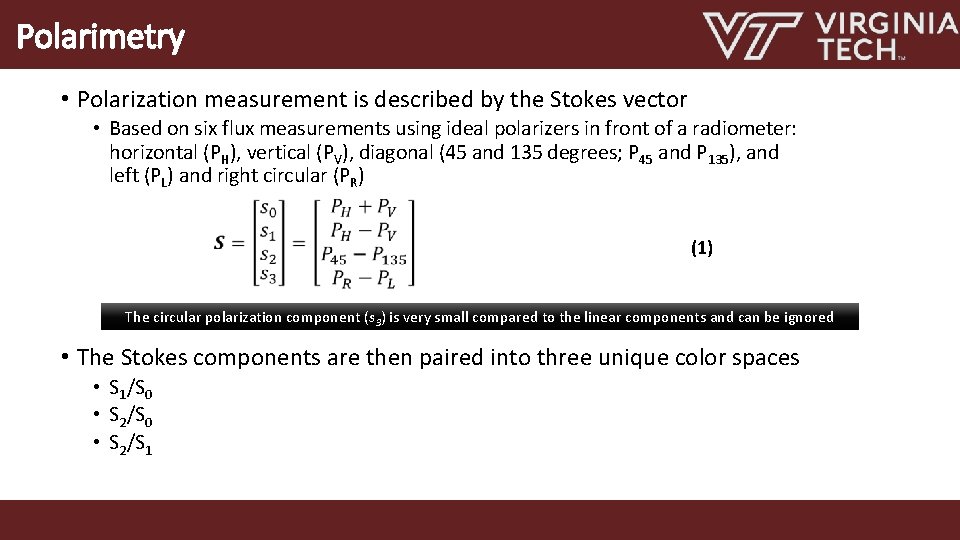
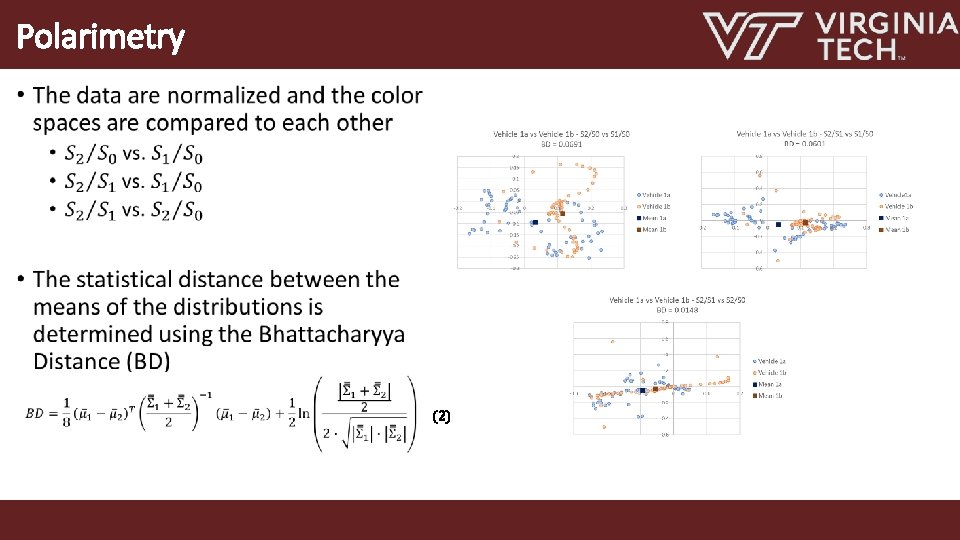
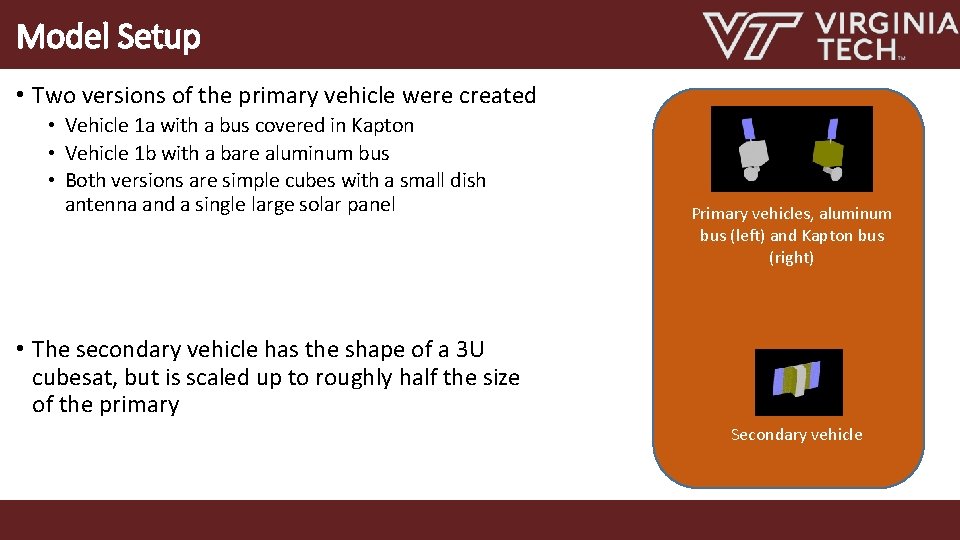
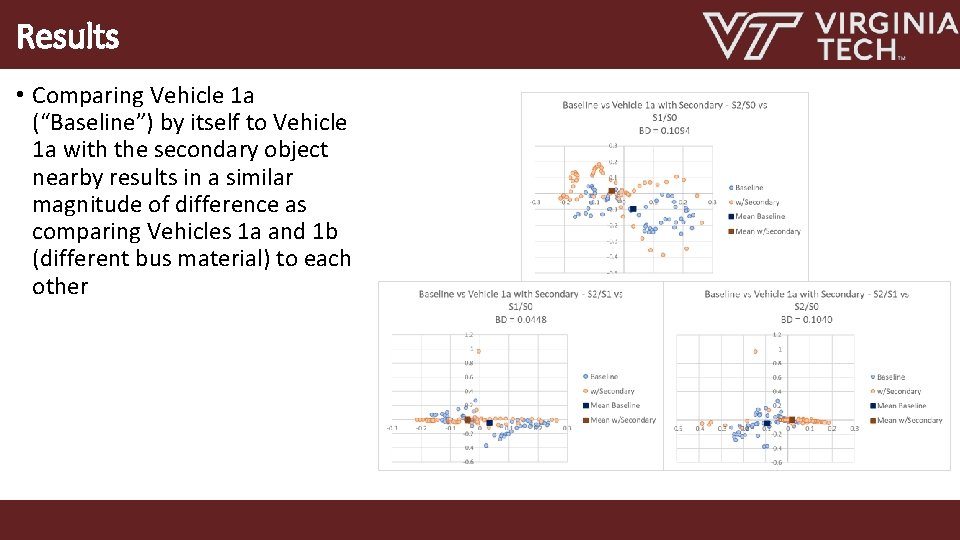
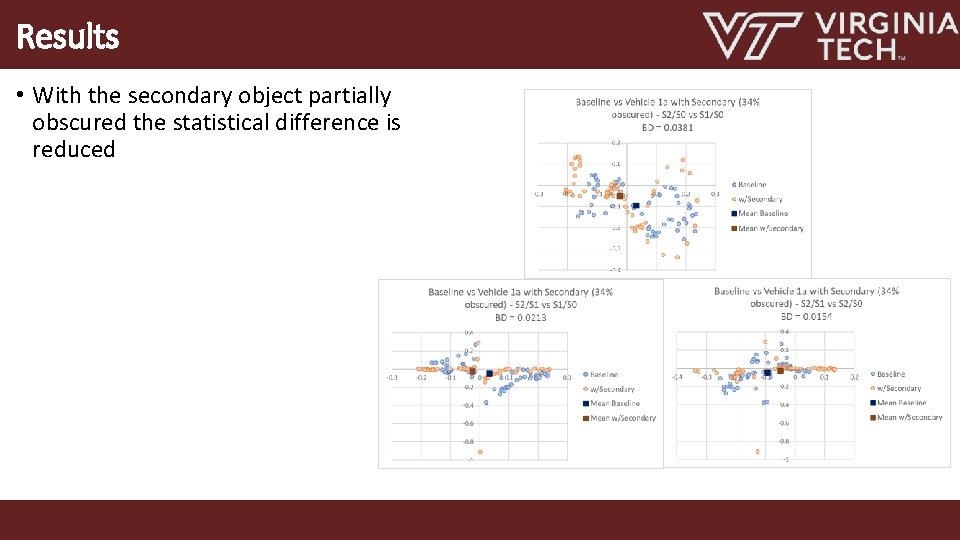
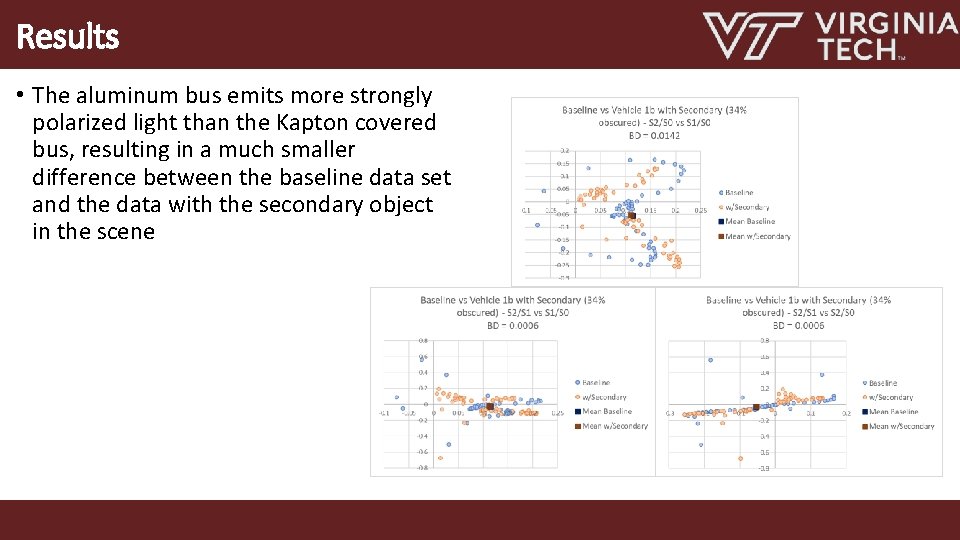
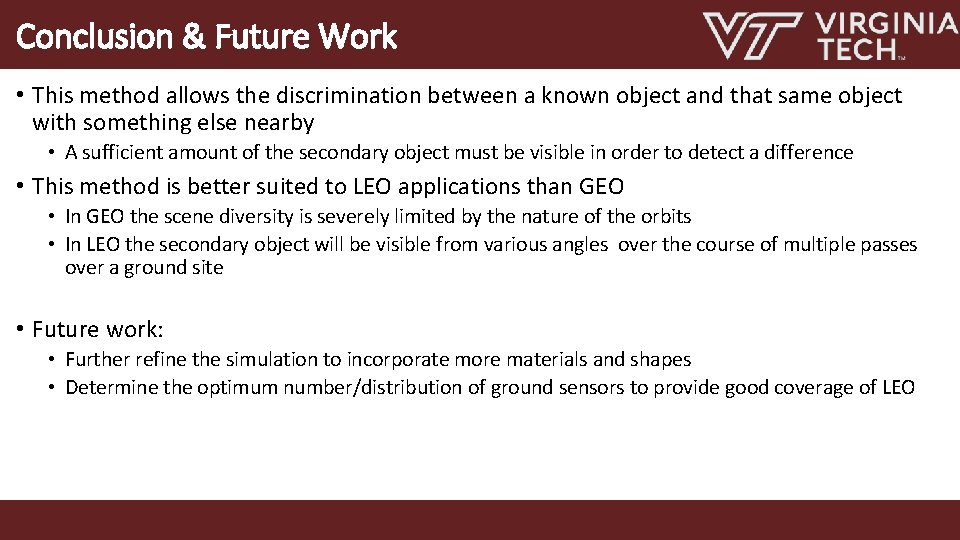
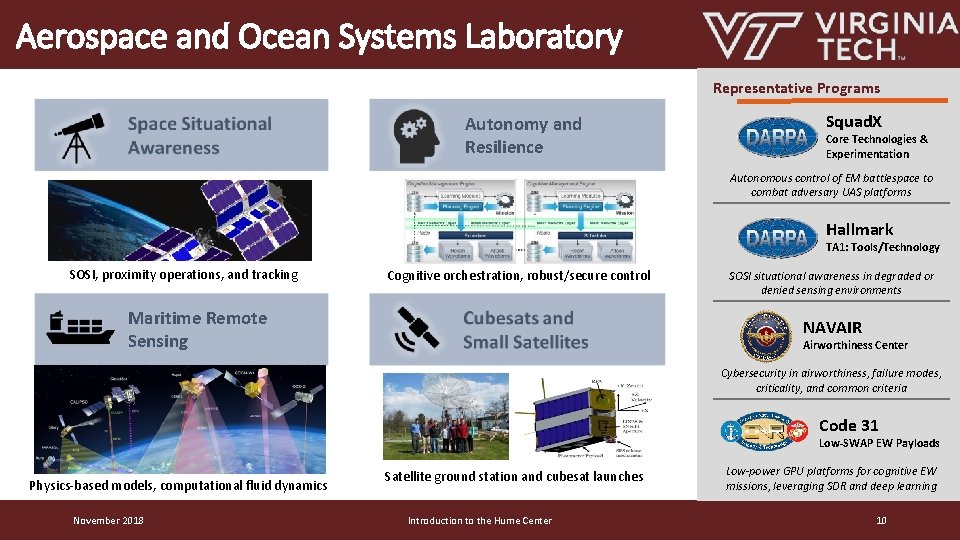
- Slides: 10
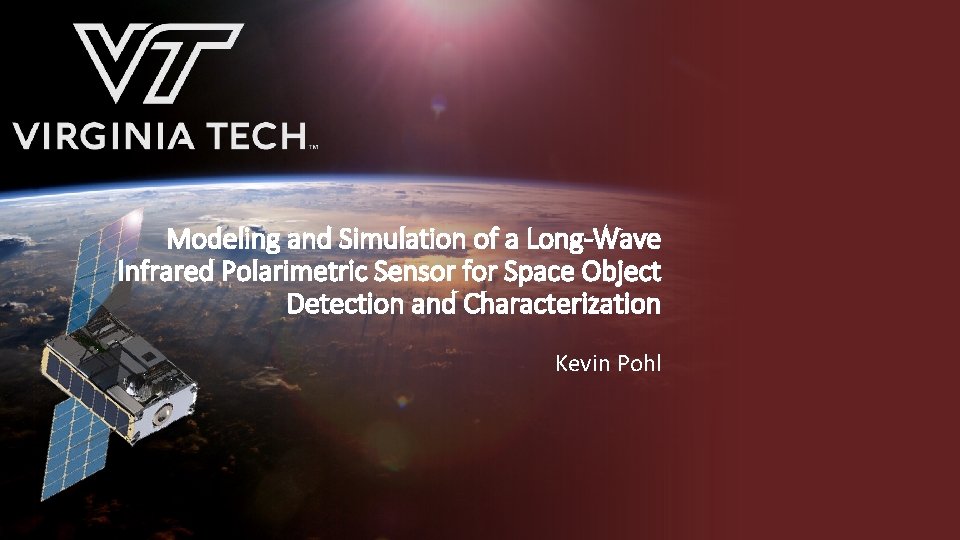
Modeling and Simulation of a Long-Wave Infrared Polarimetric Sensor for Space Object Detection and Characterization Kevin Pohl
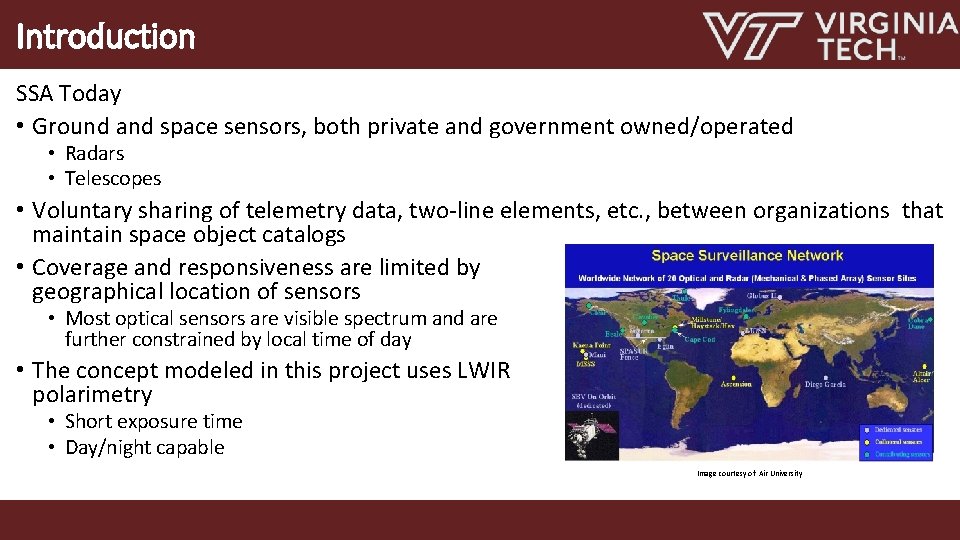
Introduction SSA Today • Ground and space sensors, both private and government owned/operated • Radars • Telescopes • Voluntary sharing of telemetry data, two-line elements, etc. , between organizations that maintain space object catalogs • Coverage and responsiveness are limited by geographical location of sensors • Most optical sensors are visible spectrum and are further constrained by local time of day • The concept modeled in this project uses LWIR polarimetry • Short exposure time • Day/night capable Image courtesy of Air University
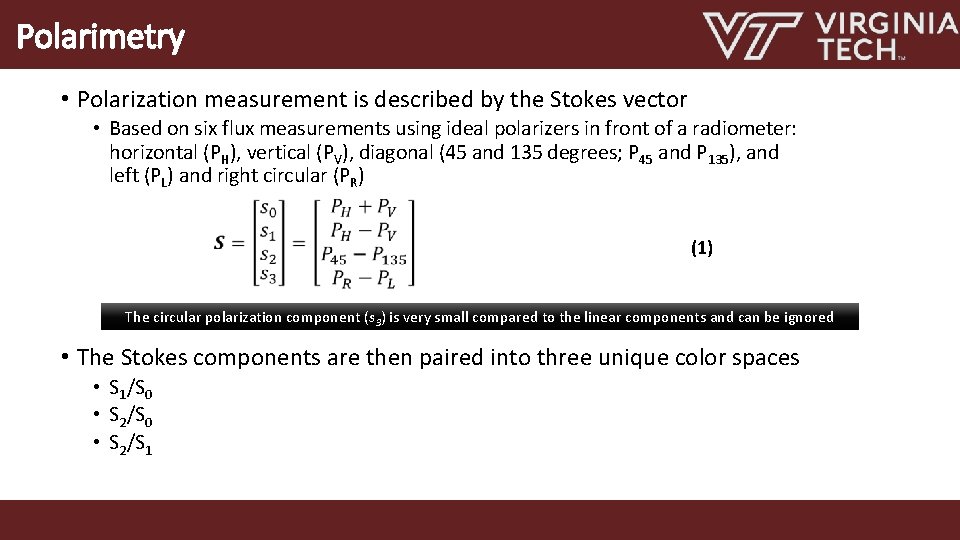
Polarimetry • Polarization measurement is described by the Stokes vector • Based on six flux measurements using ideal polarizers in front of a radiometer: horizontal (PH), vertical (PV), diagonal (45 and 135 degrees; P 45 and P 135), and left (PL) and right circular (PR) (1) The circular polarization component (s 3) is very small compared to the linear components and can be ignored • The Stokes components are then paired into three unique color spaces • S 1/S 0 • S 2/S 0 • S 2/S 1
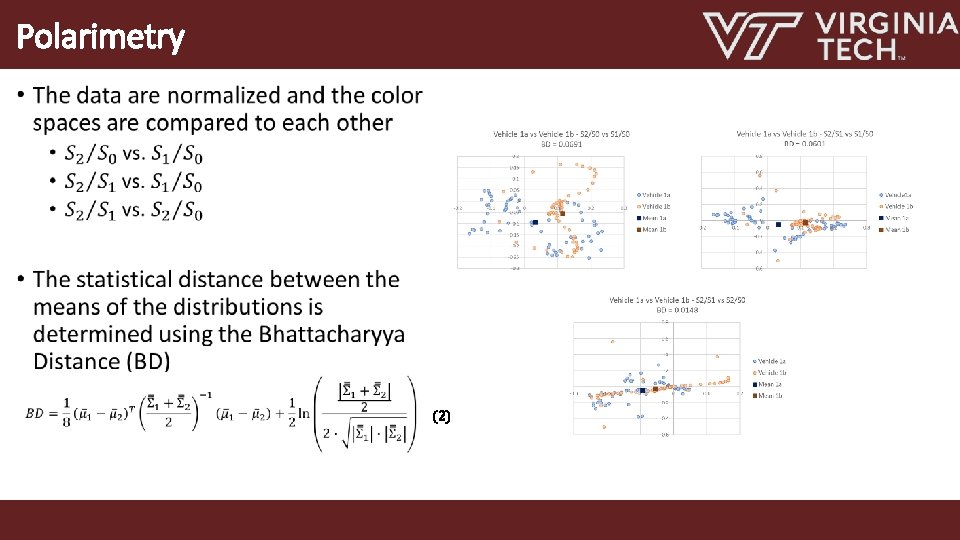
Polarimetry • (2)
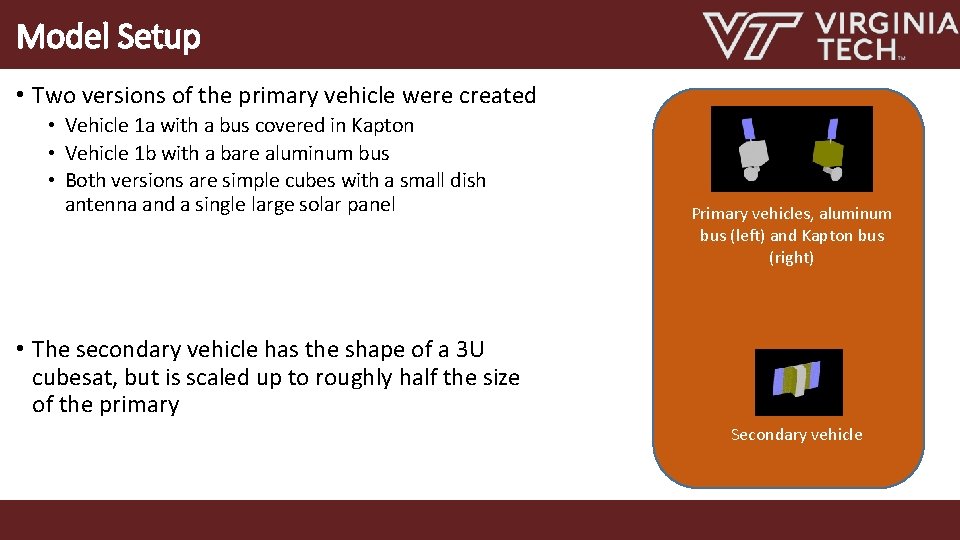
Model Setup • Two versions of the primary vehicle were created • Vehicle 1 a with a bus covered in Kapton • Vehicle 1 b with a bare aluminum bus • Both versions are simple cubes with a small dish antenna and a single large solar panel Primary vehicles, aluminum bus (left) and Kapton bus (right) • The secondary vehicle has the shape of a 3 U cubesat, but is scaled up to roughly half the size of the primary Secondary vehicle
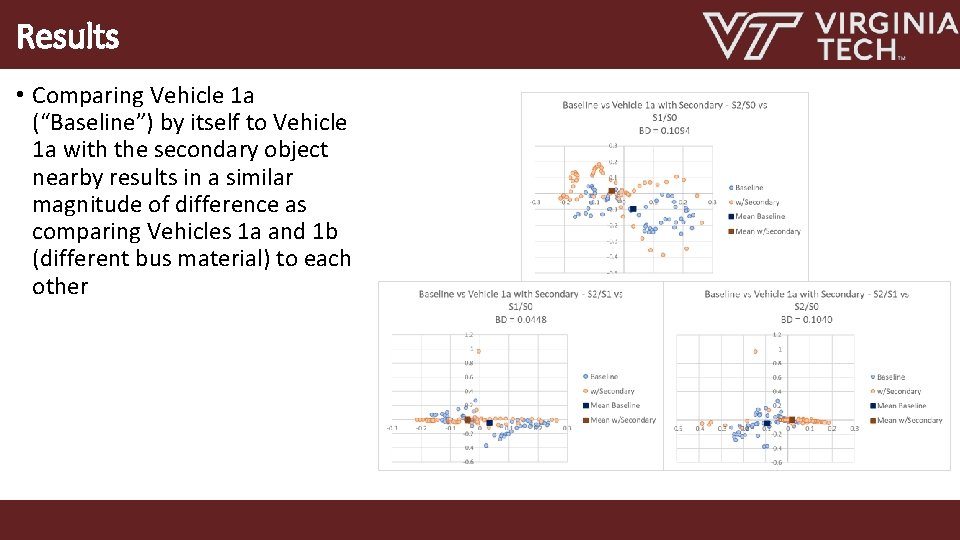
Results • Comparing Vehicle 1 a (“Baseline”) by itself to Vehicle 1 a with the secondary object nearby results in a similar magnitude of difference as comparing Vehicles 1 a and 1 b (different bus material) to each other
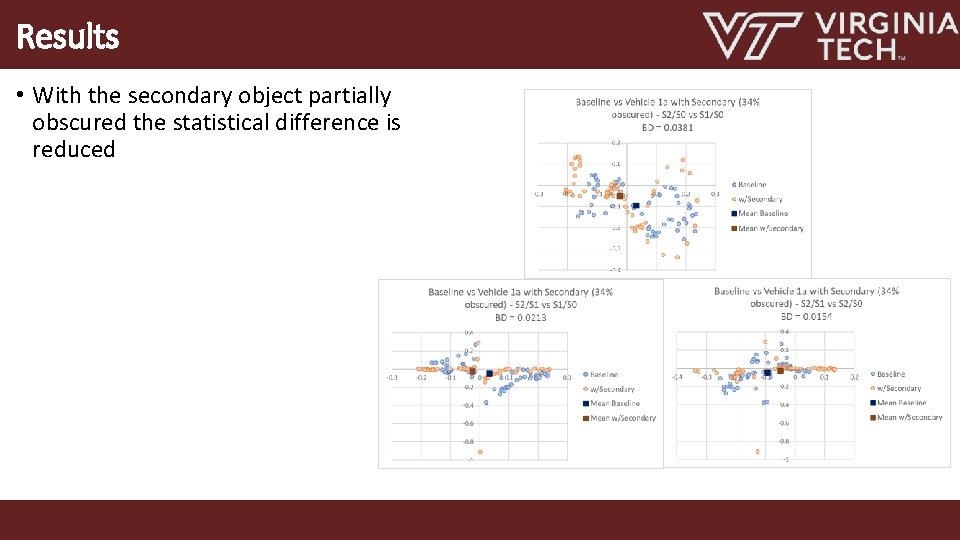
Results • With the secondary object partially obscured the statistical difference is reduced
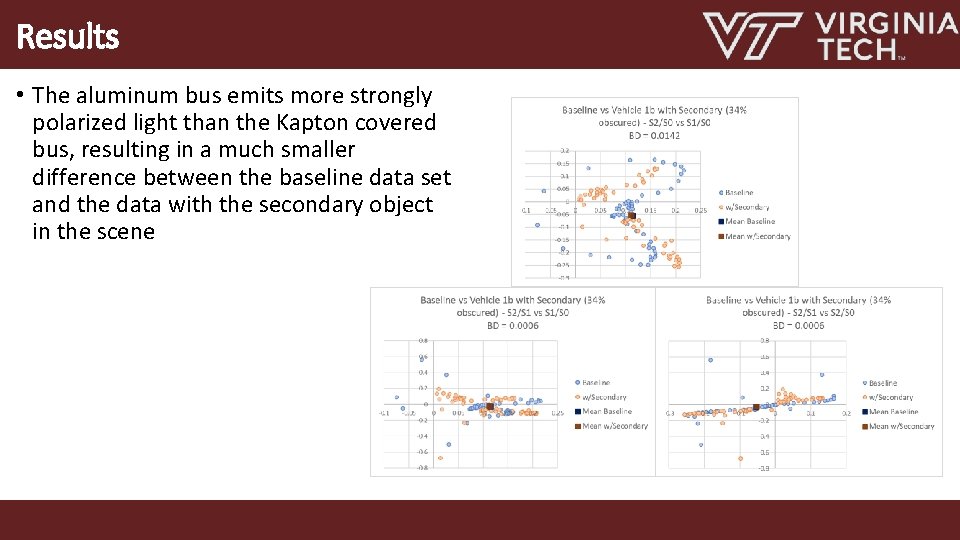
Results • The aluminum bus emits more strongly polarized light than the Kapton covered bus, resulting in a much smaller difference between the baseline data set and the data with the secondary object in the scene
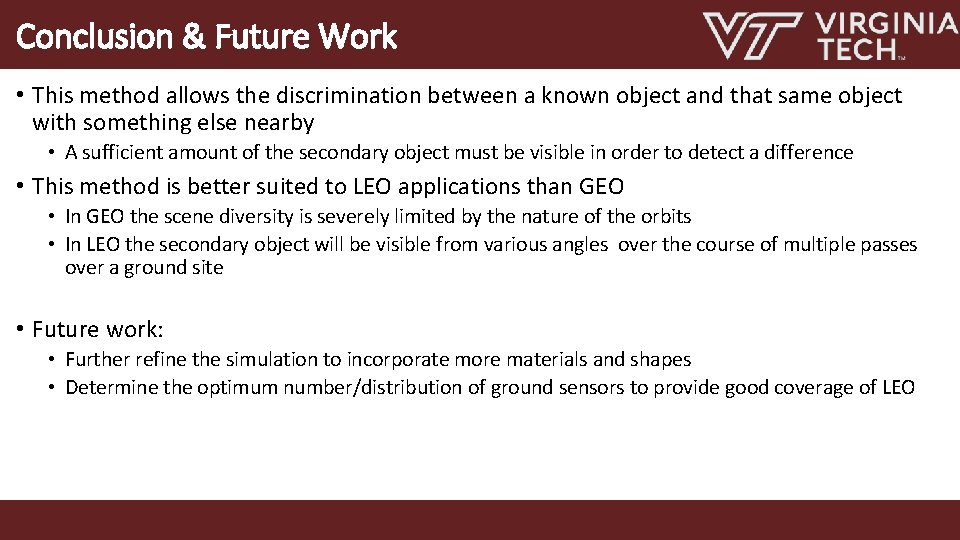
Conclusion & Future Work • This method allows the discrimination between a known object and that same object with something else nearby • A sufficient amount of the secondary object must be visible in order to detect a difference • This method is better suited to LEO applications than GEO • In GEO the scene diversity is severely limited by the nature of the orbits • In LEO the secondary object will be visible from various angles over the course of multiple passes over a ground site • Future work: • Further refine the simulation to incorporate more materials and shapes • Determine the optimum number/distribution of ground sensors to provide good coverage of LEO
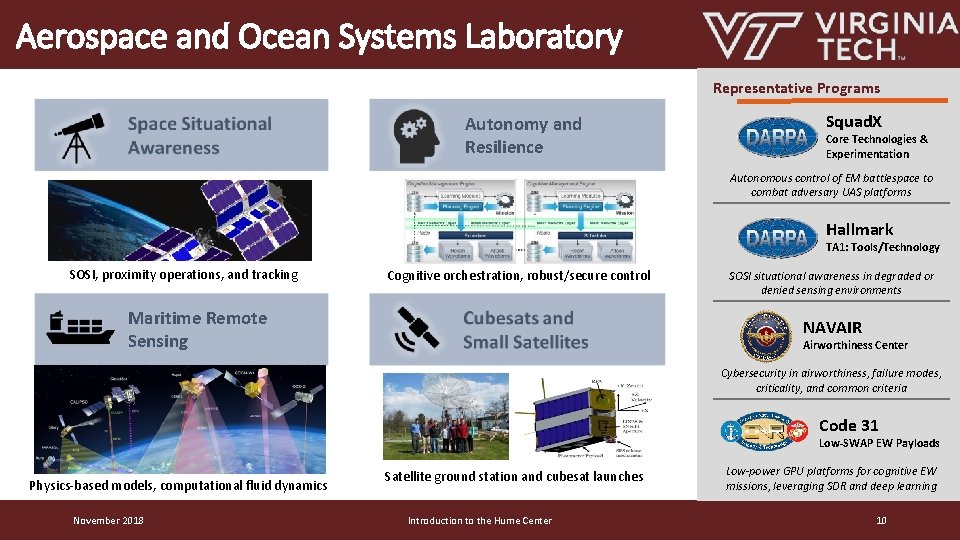
Aerospace and Ocean Systems Laboratory Representative Programs Autonomy and Resilience Squad. X Core Technologies & Experimentation Autonomous control of EM battlespace to combat adversary UAS platforms Hallmark TA 1: Tools/Technology SOSI, proximity operations, and tracking Cognitive orchestration, robust/secure control Maritime Remote Sensing SOSI situational awareness in degraded or denied sensing environments NAVAIR Airworthiness Center Cybersecurity in airworthiness, failure modes, criticality, and common criteria Code 31 Low-SWAP EW Payloads Physics-based models, computational fluid dynamics November 2018 Satellite ground station and cubesat launches Introduction to the Hume Center Low-power GPU platforms for cognitive EW missions, leveraging SDR and deep learning 10
Outgoing longwave radiation
Olr earth
Modeling and role modeling theory
Simulation kelton
Pharmaceutical simulation and modeling
Introduction to modeling and simulation
Biomedical modeling and simulation
Simulasi itu apa
Modeling and simulation
Relational vs dimensional data modeling
Basic simulation modeling