Growth performance of different chicken strains in ACGG
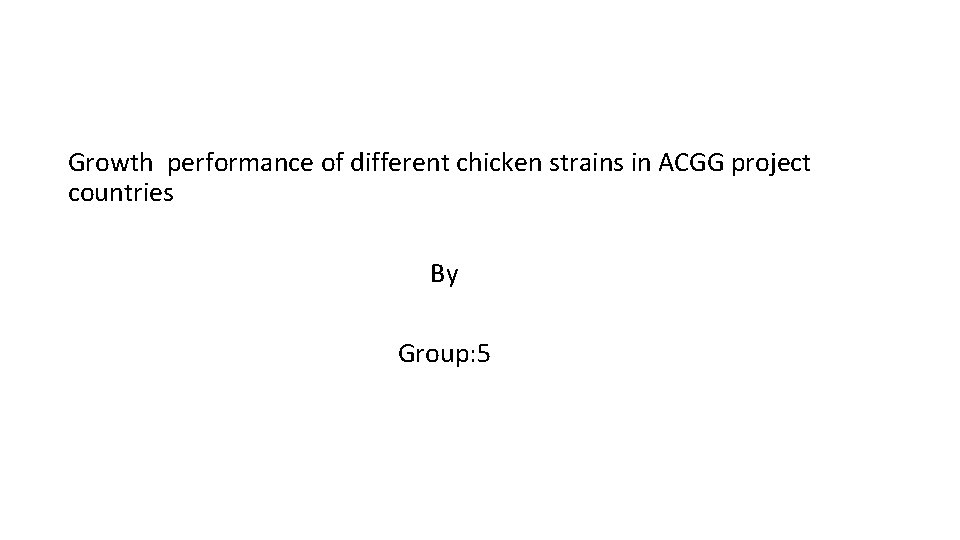
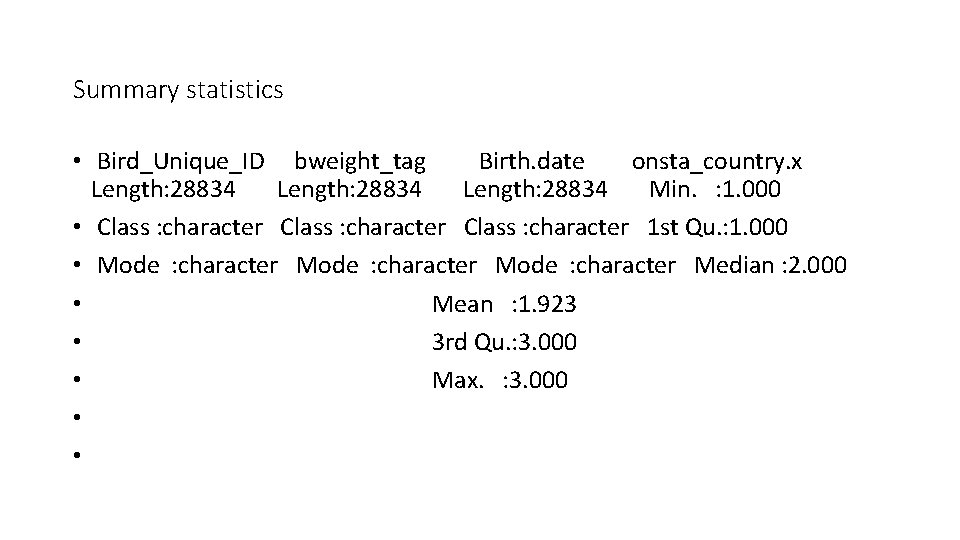
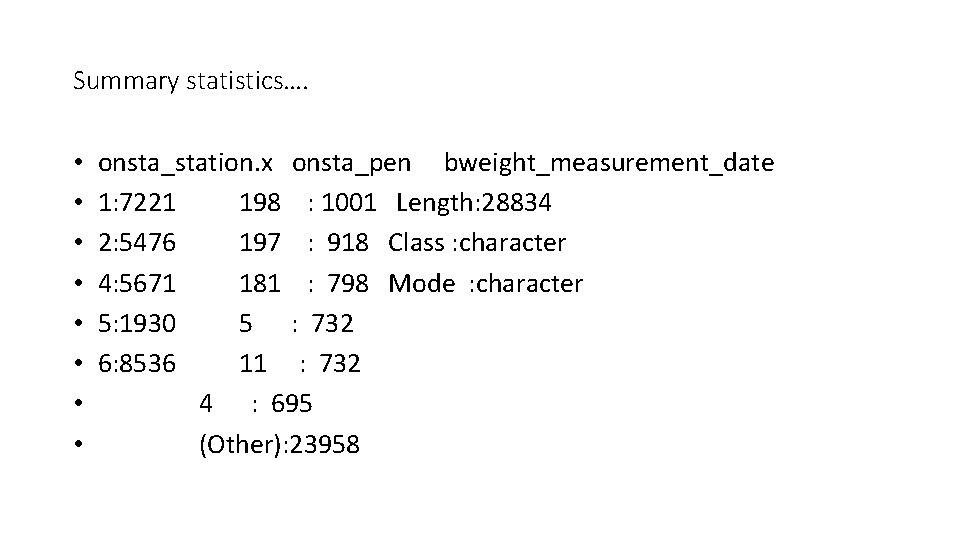
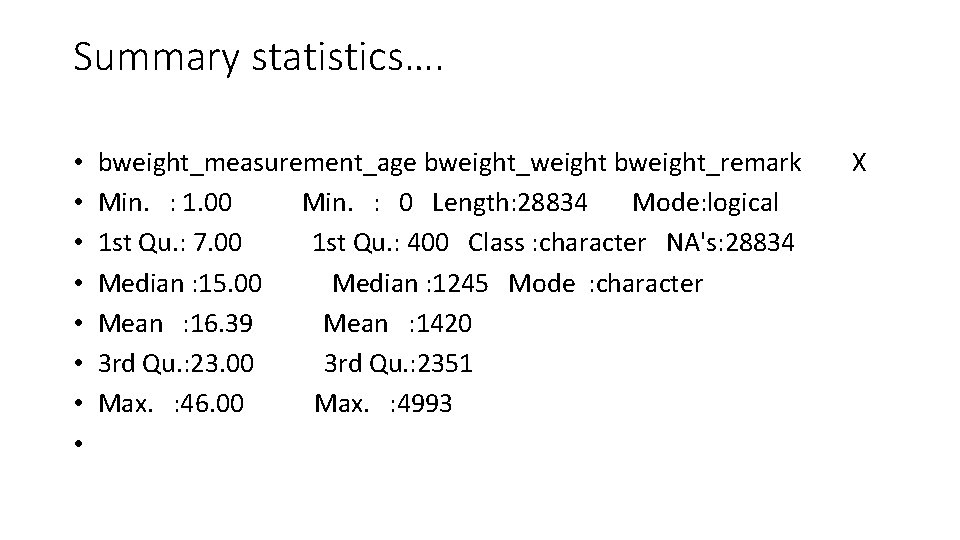
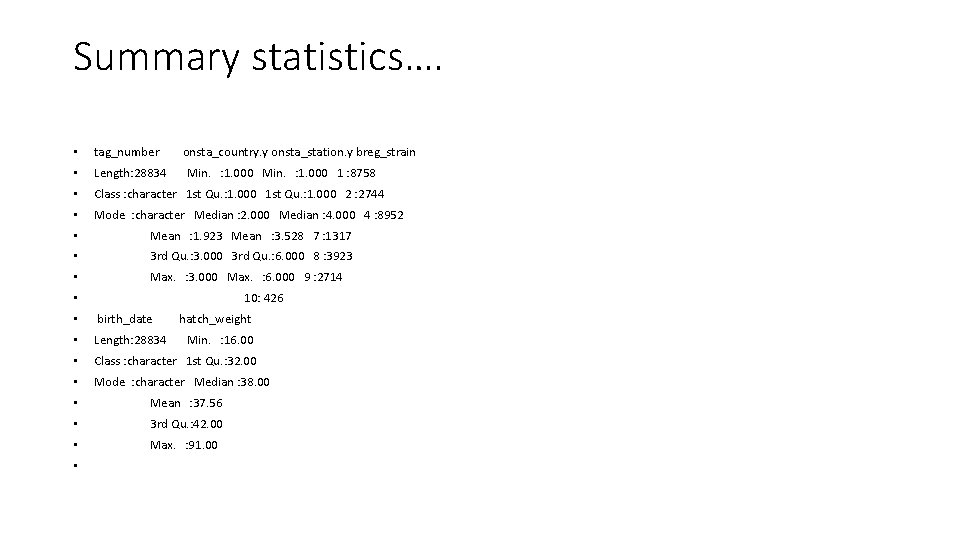
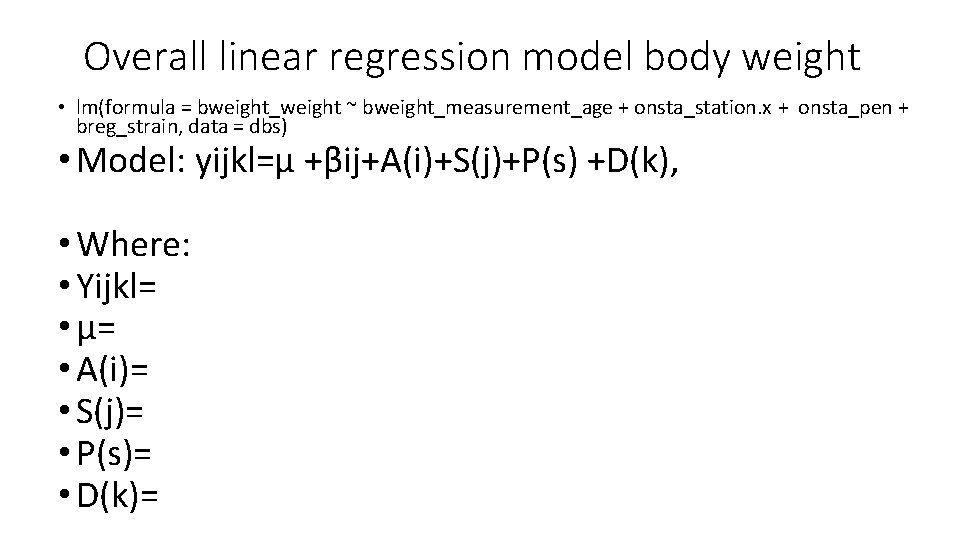
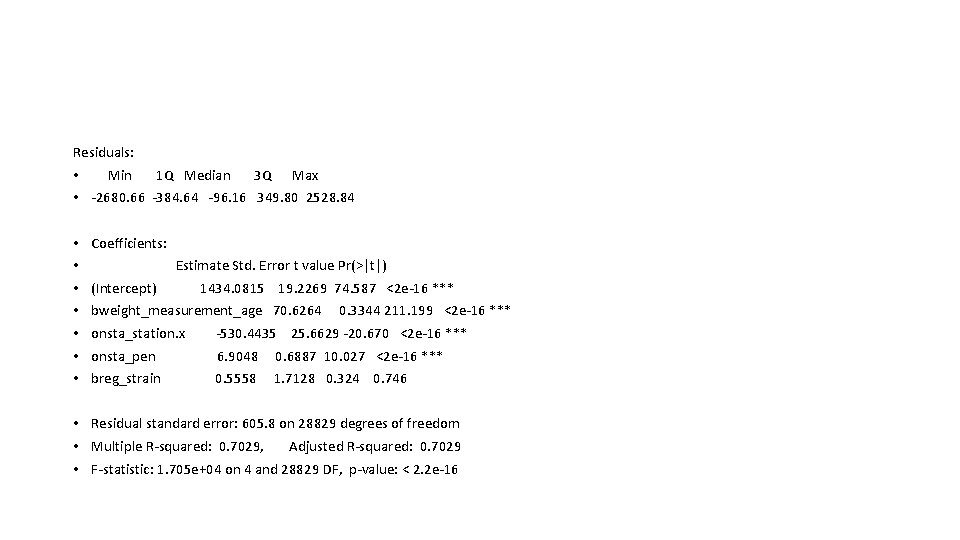
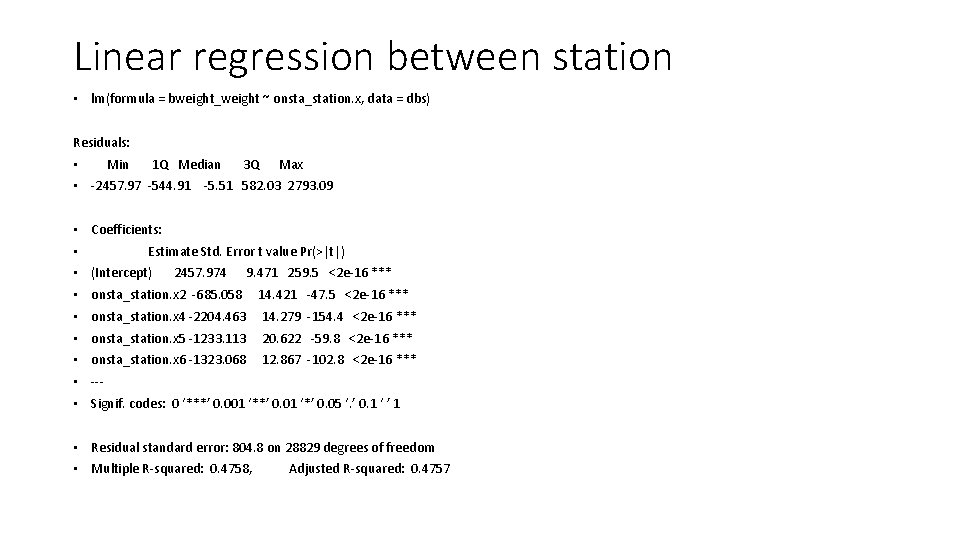
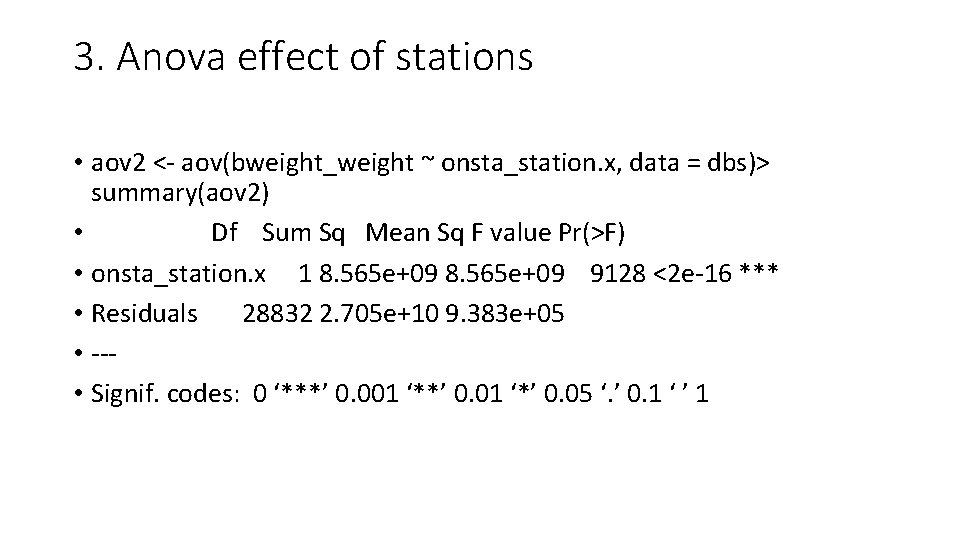
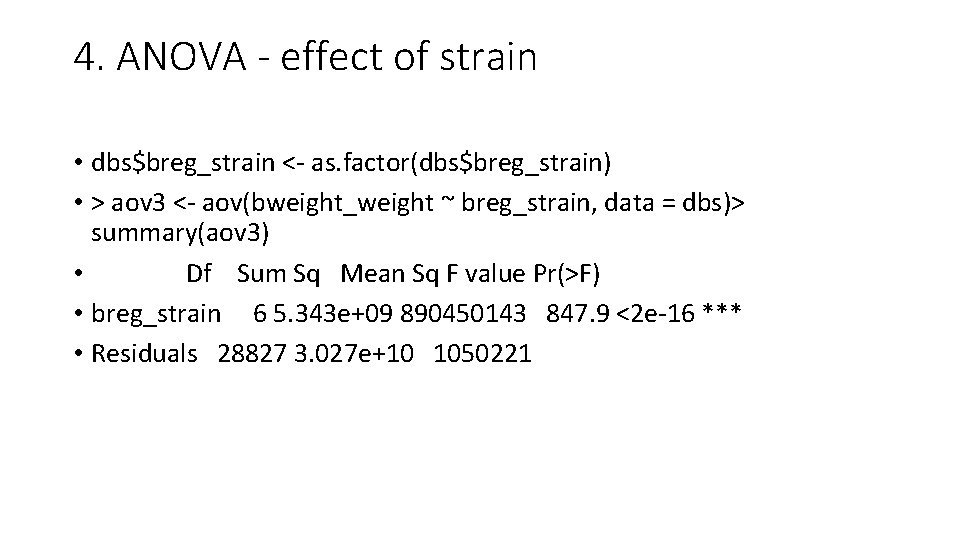
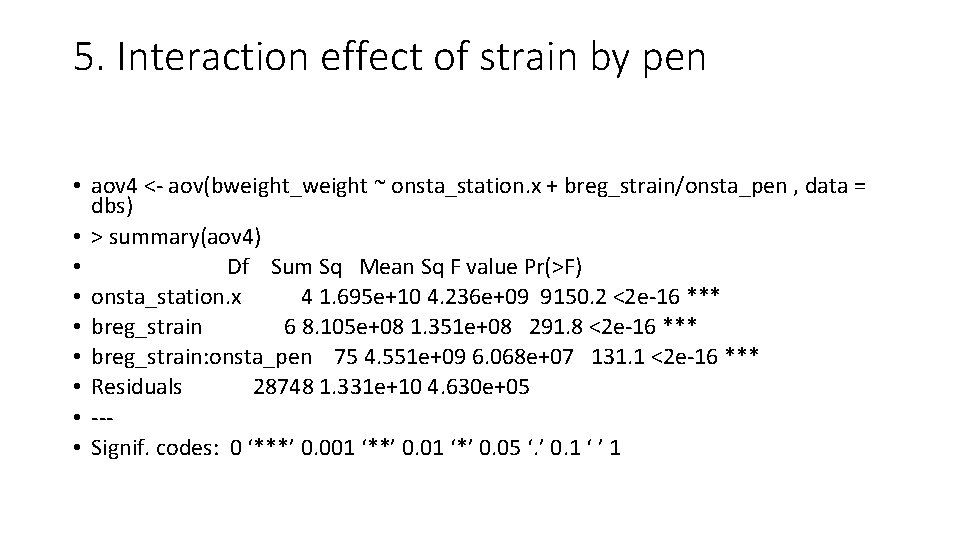
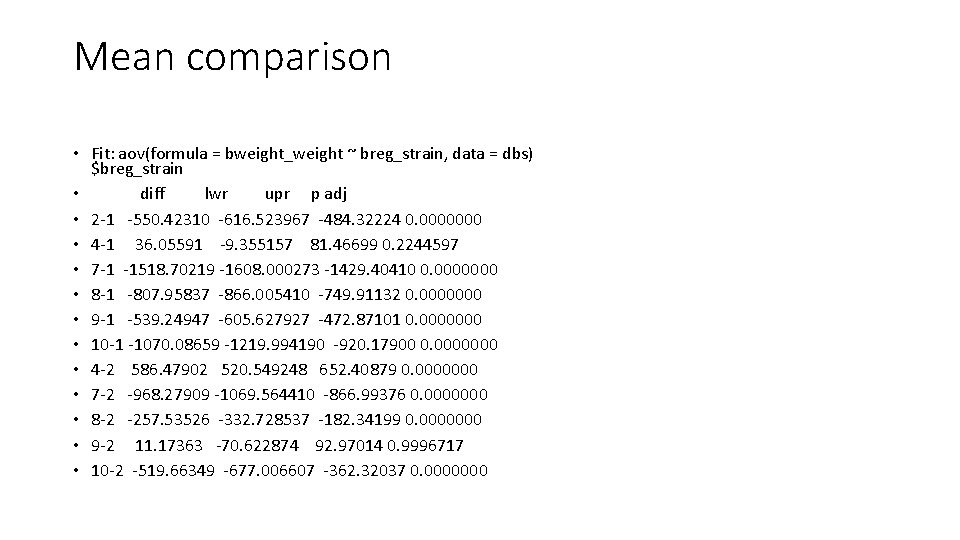
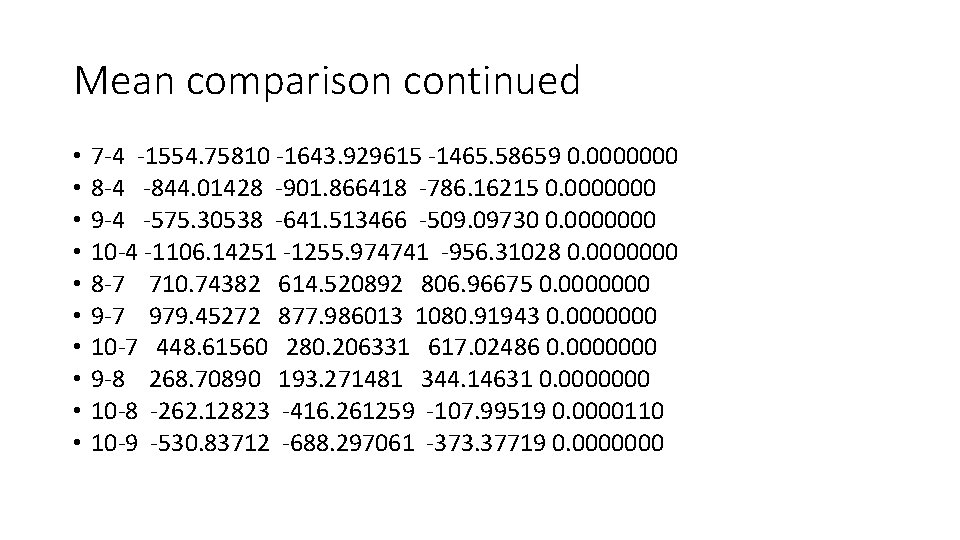
- Slides: 13
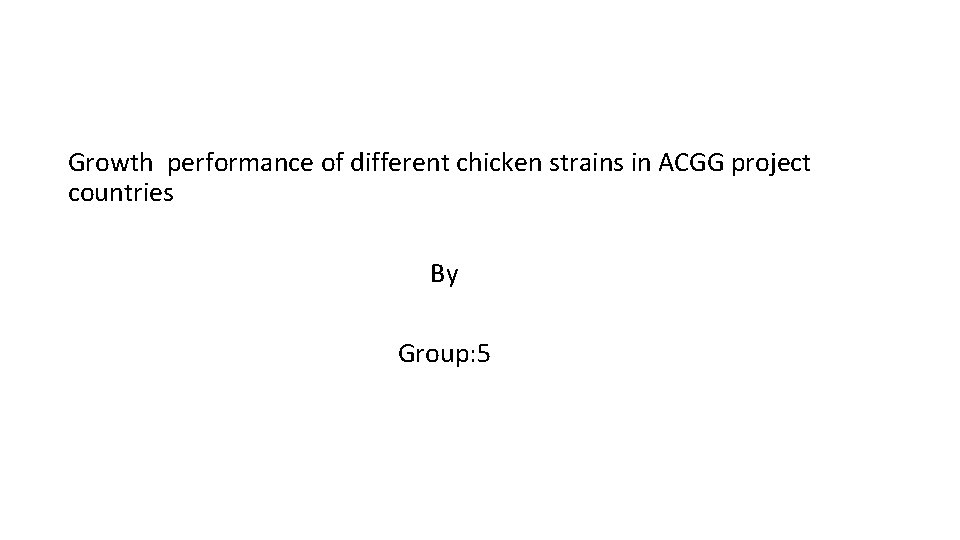
Growth performance of different chicken strains in ACGG project countries By Group: 5
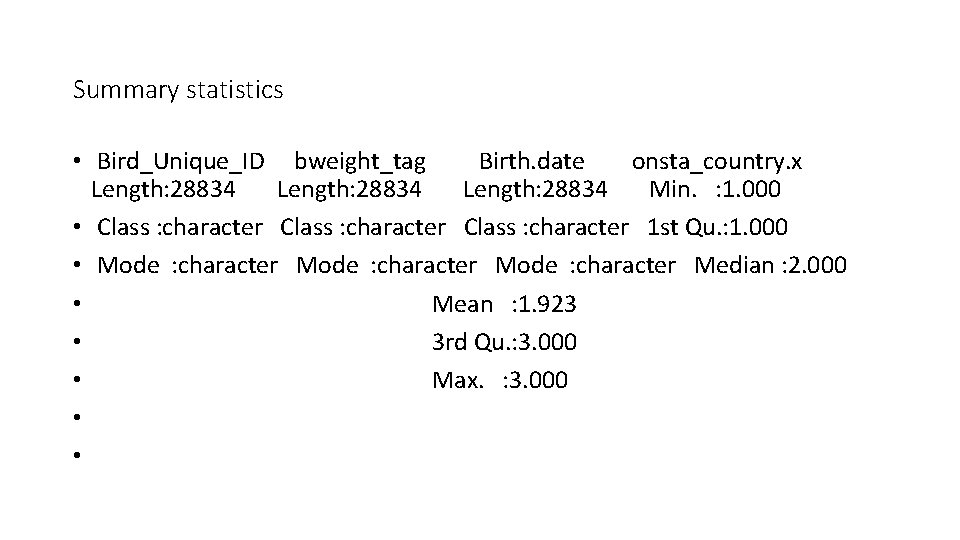
Summary statistics • Bird_Unique_ID bweight_tag Birth. date onsta_country. x Length: 28834 Min. : 1. 000 • Class : character 1 st Qu. : 1. 000 • Mode : character Median : 2. 000 • Mean : 1. 923 • 3 rd Qu. : 3. 000 • Max. : 3. 000 • •
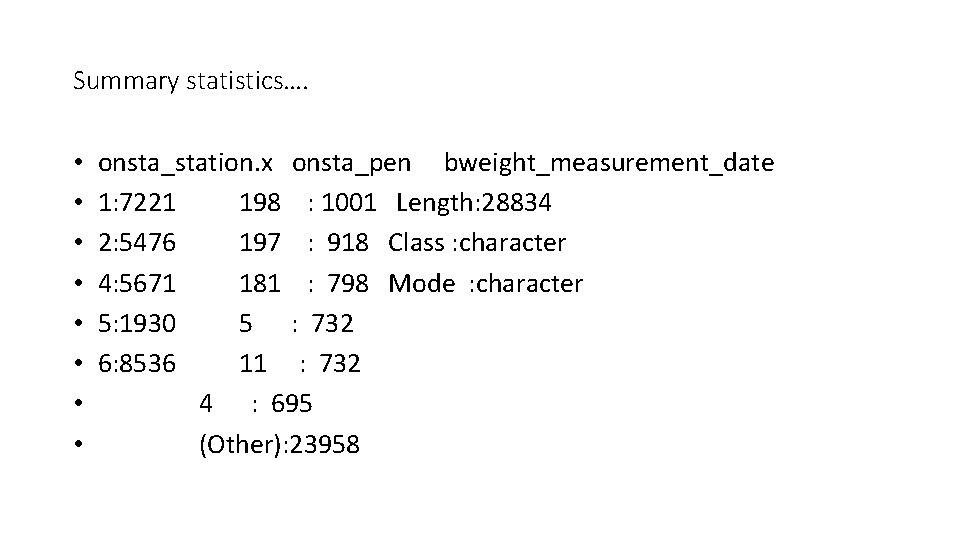
Summary statistics…. • • onsta_station. x onsta_pen bweight_measurement_date 1: 7221 198 : 1001 Length: 28834 2: 5476 197 : 918 Class : character 4: 5671 181 : 798 Mode : character 5: 1930 5 : 732 6: 8536 11 : 732 4 : 695 (Other): 23958
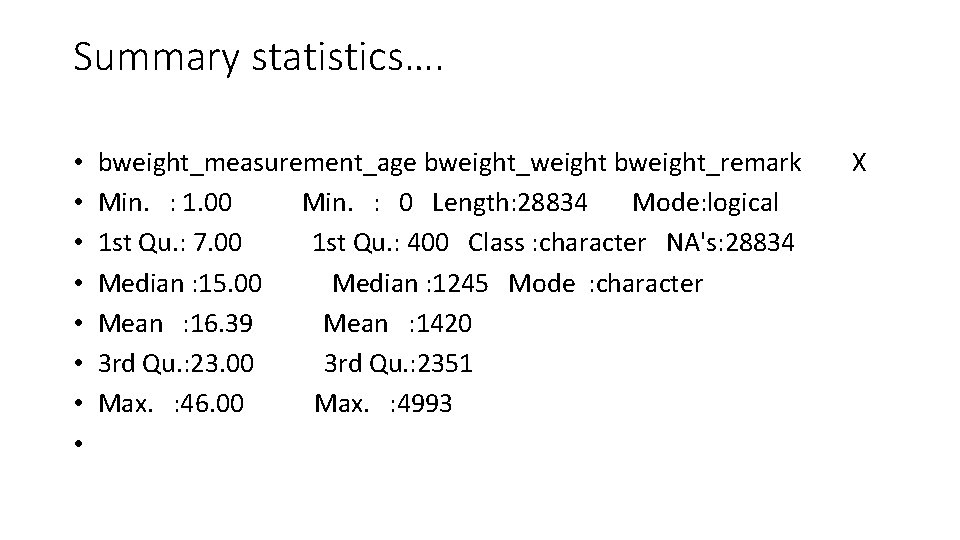
Summary statistics…. • • bweight_measurement_age bweight_weight bweight_remark Min. : 1. 00 Min. : 0 Length: 28834 Mode: logical 1 st Qu. : 7. 00 1 st Qu. : 400 Class : character NA's: 28834 Median : 15. 00 Median : 1245 Mode : character Mean : 16. 39 Mean : 1420 3 rd Qu. : 23. 00 3 rd Qu. : 2351 Max. : 46. 00 Max. : 4993 X
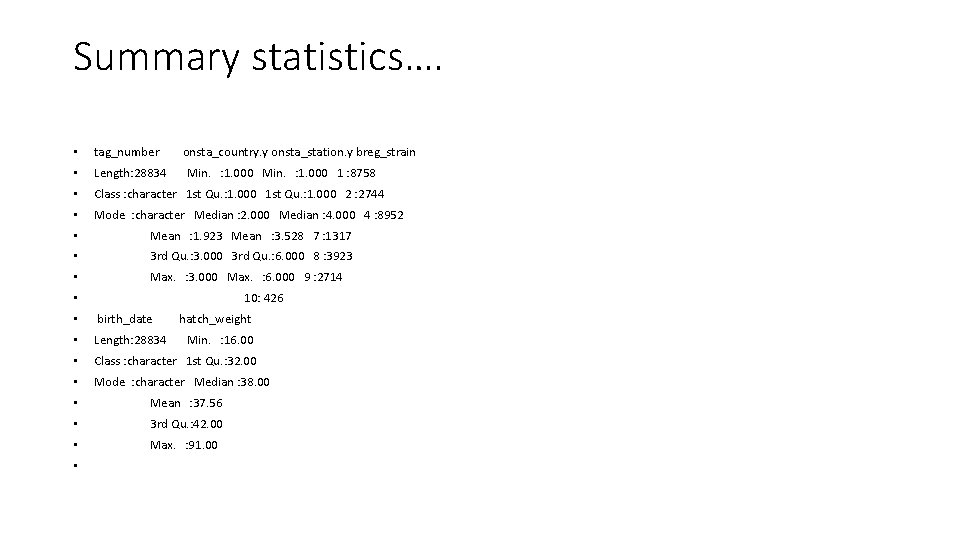
Summary statistics…. • tag_number onsta_country. y onsta_station. y breg_strain • Length: 28834 • Class : character 1 st Qu. : 1. 000 2 : 2744 • Mode : character Median : 2. 000 Median : 4. 000 4 : 8952 • Mean : 1. 923 Mean : 3. 528 7 : 1317 • 3 rd Qu. : 3. 000 3 rd Qu. : 6. 000 8 : 3923 • Max. : 3. 000 Max. : 6. 000 9 : 2714 Min. : 1. 000 1 : 8758 10: 426 • • birth_date hatch_weight • Length: 28834 • Class : character 1 st Qu. : 32. 00 • Mode : character Median : 38. 00 Min. : 16. 00 • Mean : 37. 56 • 3 rd Qu. : 42. 00 • Max. : 91. 00 •
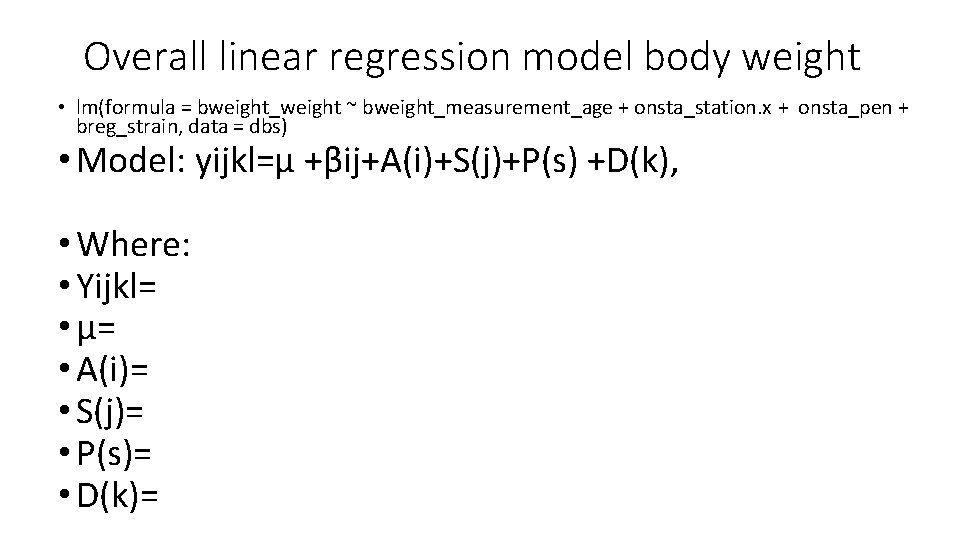
Overall linear regression model body weight • lm(formula = bweight_weight ~ bweight_measurement_age + onsta_station. x + onsta_pen + breg_strain, data = dbs) • Model: yijkl=µ +βij+A(i)+S(j)+P(s) +D(k), • Where: • Yijkl= • µ= • A(i)= • S(j)= • P(s)= • D(k)=
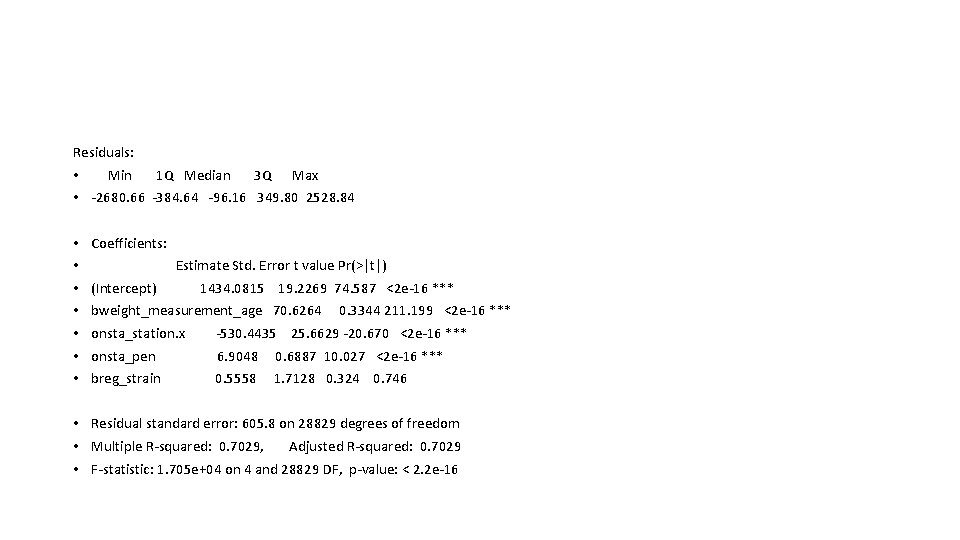
Residuals: • Min 1 Q Median 3 Q Max • -2680. 66 -384. 64 -96. 16 349. 80 2528. 84 • • Coefficients: Estimate Std. Error t value Pr(>|t|) (Intercept) 1434. 0815 19. 2269 74. 587 <2 e-16 *** bweight_measurement_age 70. 6264 0. 3344 211. 199 <2 e-16 *** onsta_station. x -530. 4435 25. 6629 -20. 670 <2 e-16 *** onsta_pen 6. 9048 0. 6887 10. 027 <2 e-16 *** breg_strain 0. 5558 1. 7128 0. 324 0. 746 • Residual standard error: 605. 8 on 28829 degrees of freedom • Multiple R-squared: 0. 7029, Adjusted R-squared: 0. 7029 • F-statistic: 1. 705 e+04 on 4 and 28829 DF, p-value: < 2. 2 e-16
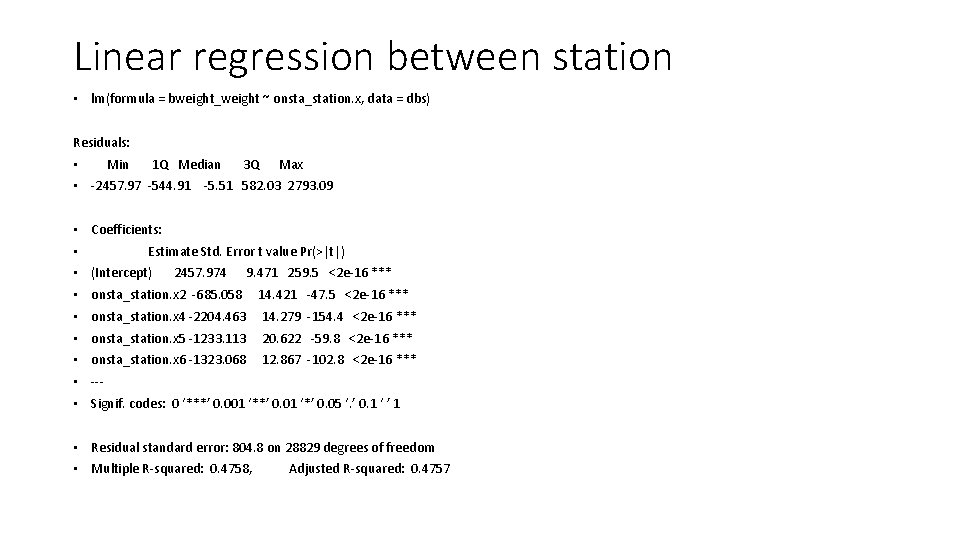
Linear regression between station • lm(formula = bweight_weight ~ onsta_station. x, data = dbs) Residuals: • Min 1 Q Median 3 Q Max • -2457. 97 -544. 91 -5. 51 582. 03 2793. 09 • Coefficients: • Estimate Std. Error t value Pr(>|t|) • (Intercept) 2457. 974 9. 471 259. 5 <2 e-16 *** • onsta_station. x 2 -685. 058 14. 421 -47. 5 <2 e-16 *** • onsta_station. x 4 -2204. 463 14. 279 -154. 4 <2 e-16 *** • onsta_station. x 5 -1233. 113 20. 622 -59. 8 <2 e-16 *** • onsta_station. x 6 -1323. 068 12. 867 -102. 8 <2 e-16 *** • -- • Signif. codes: 0 ‘***’ 0. 001 ‘**’ 0. 01 ‘*’ 0. 05 ‘. ’ 0. 1 ‘ ’ 1 • Residual standard error: 804. 8 on 28829 degrees of freedom • Multiple R-squared: 0. 4758, Adjusted R-squared: 0. 4757
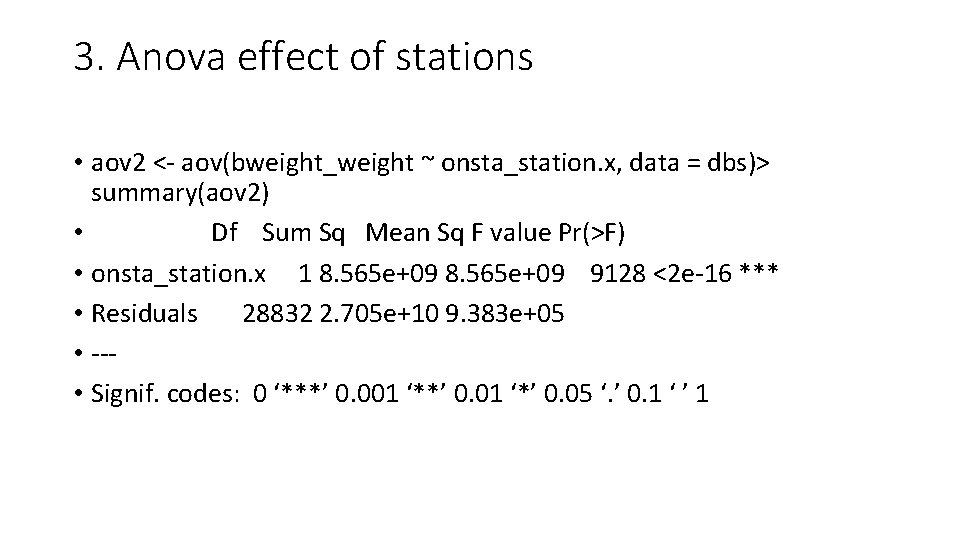
3. Anova effect of stations • aov 2 <- aov(bweight_weight ~ onsta_station. x, data = dbs)> summary(aov 2) • Df Sum Sq Mean Sq F value Pr(>F) • onsta_station. x 1 8. 565 e+09 9128 <2 e-16 *** • Residuals 28832 2. 705 e+10 9. 383 e+05 • -- • Signif. codes: 0 ‘***’ 0. 001 ‘**’ 0. 01 ‘*’ 0. 05 ‘. ’ 0. 1 ‘ ’ 1
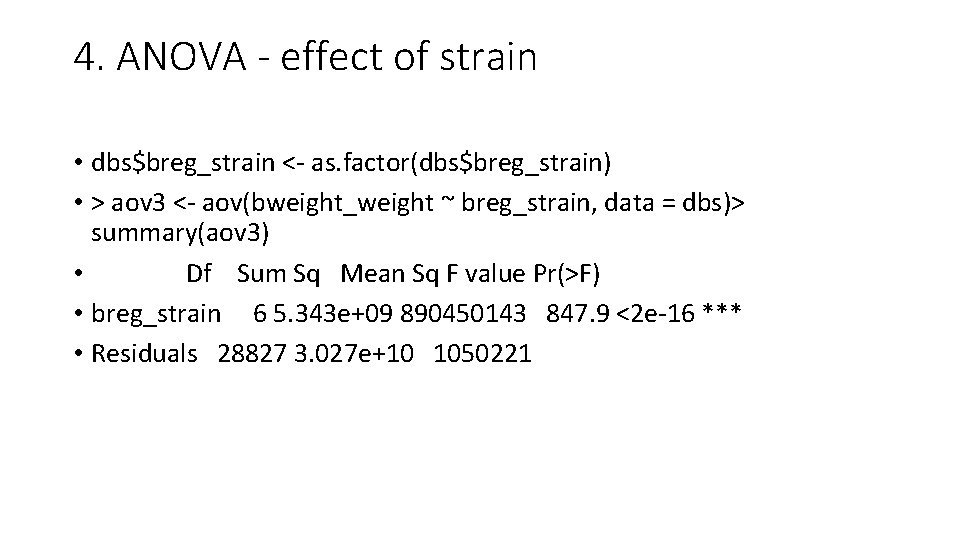
4. ANOVA - effect of strain • dbs$breg_strain <- as. factor(dbs$breg_strain) • > aov 3 <- aov(bweight_weight ~ breg_strain, data = dbs)> summary(aov 3) • Df Sum Sq Mean Sq F value Pr(>F) • breg_strain 6 5. 343 e+09 890450143 847. 9 <2 e-16 *** • Residuals 28827 3. 027 e+10 1050221
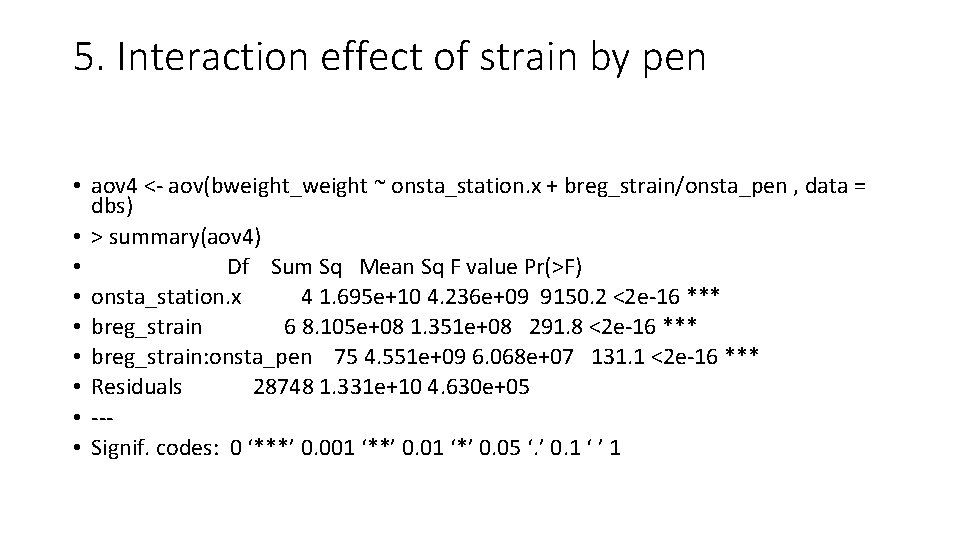
5. Interaction effect of strain by pen • aov 4 <- aov(bweight_weight ~ onsta_station. x + breg_strain/onsta_pen , data = dbs) • > summary(aov 4) • Df Sum Sq Mean Sq F value Pr(>F) • onsta_station. x 4 1. 695 e+10 4. 236 e+09 9150. 2 <2 e-16 *** • breg_strain 6 8. 105 e+08 1. 351 e+08 291. 8 <2 e-16 *** • breg_strain: onsta_pen 75 4. 551 e+09 6. 068 e+07 131. 1 <2 e-16 *** • Residuals 28748 1. 331 e+10 4. 630 e+05 • -- • Signif. codes: 0 ‘***’ 0. 001 ‘**’ 0. 01 ‘*’ 0. 05 ‘. ’ 0. 1 ‘ ’ 1
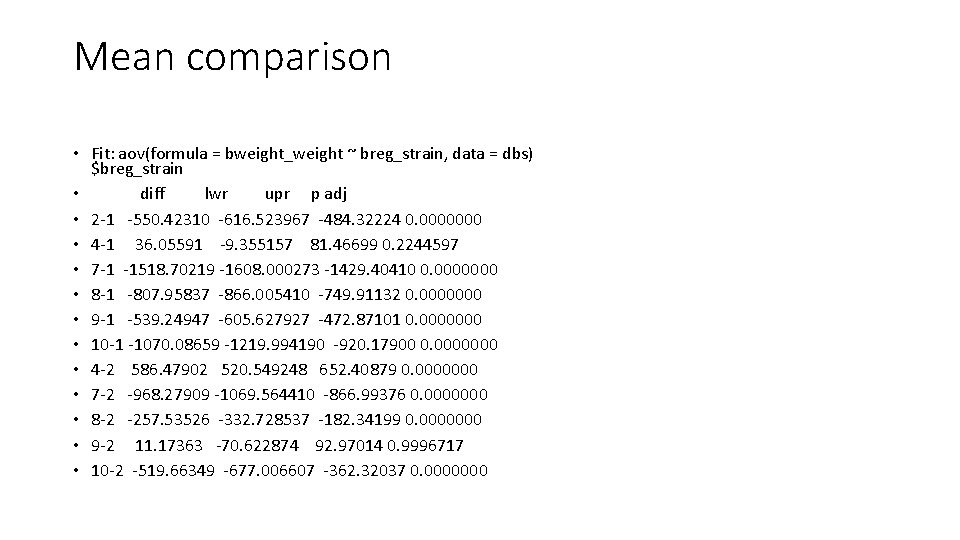
Mean comparison • Fit: aov(formula = bweight_weight ~ breg_strain, data = dbs) $breg_strain • diff lwr upr p adj • 2 -1 -550. 42310 -616. 523967 -484. 32224 0. 0000000 • 4 -1 36. 05591 -9. 355157 81. 46699 0. 2244597 • 7 -1 -1518. 70219 -1608. 000273 -1429. 40410 0. 0000000 • 8 -1 -807. 95837 -866. 005410 -749. 91132 0. 0000000 • 9 -1 -539. 24947 -605. 627927 -472. 87101 0. 0000000 • 10 -1 -1070. 08659 -1219. 994190 -920. 17900 0. 0000000 • 4 -2 586. 47902 520. 549248 652. 40879 0. 0000000 • 7 -2 -968. 27909 -1069. 564410 -866. 99376 0. 0000000 • 8 -2 -257. 53526 -332. 728537 -182. 34199 0. 0000000 • 9 -2 11. 17363 -70. 622874 92. 97014 0. 9996717 • 10 -2 -519. 66349 -677. 006607 -362. 32037 0. 0000000
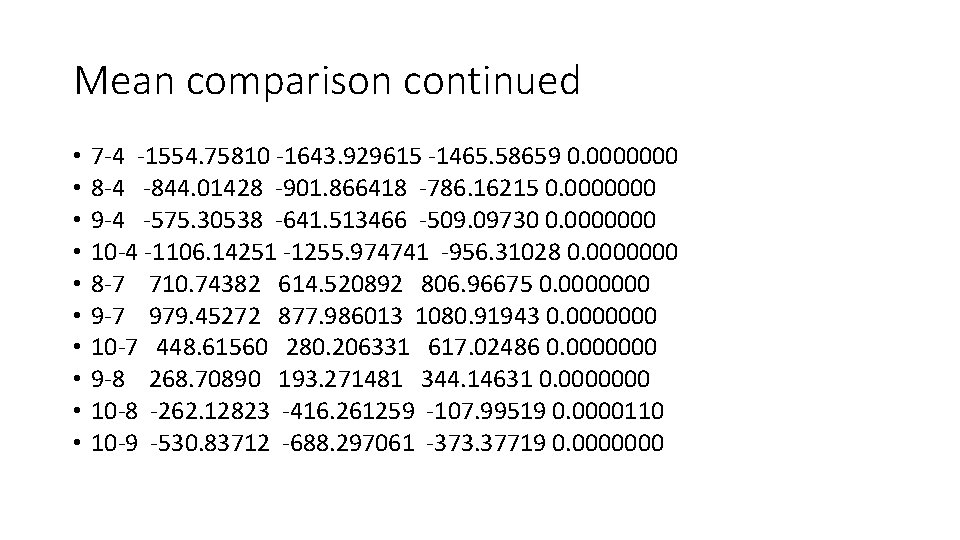
Mean comparison continued • • • 7 -4 -1554. 75810 -1643. 929615 -1465. 58659 0. 0000000 8 -4 -844. 01428 -901. 866418 -786. 16215 0. 0000000 9 -4 -575. 30538 -641. 513466 -509. 09730 0. 0000000 10 -4 -1106. 14251 -1255. 974741 -956. 31028 0. 0000000 8 -7 710. 74382 614. 520892 806. 96675 0. 0000000 9 -7 979. 45272 877. 986013 1080. 91943 0. 0000000 10 -7 448. 61560 280. 206331 617. 02486 0. 0000000 9 -8 268. 70890 193. 271481 344. 14631 0. 0000000 10 -8 -262. 12823 -416. 261259 -107. 99519 0. 0000110 10 -9 -530. 83712 -688. 297061 -373. 37719 0. 0000000
What are the greatest strains on walter and ruth's marriage
Sprains and strains
Ssy in machine design
Define growth analysis
Ground tissue
Step growth polymerization vs chain growth
Primary growth and secondary growth in plants
Primary growth and secondary growth in plants
Geometric growth graph
Neoclassical growth theory vs. endogenous growth theory
Organic growth vs inorganic growth
Stages of developmetn
Plant growth with different liquids
Principles of human growth and development