EHS 655 Lecture 7 Exposure modeling categorical analysis
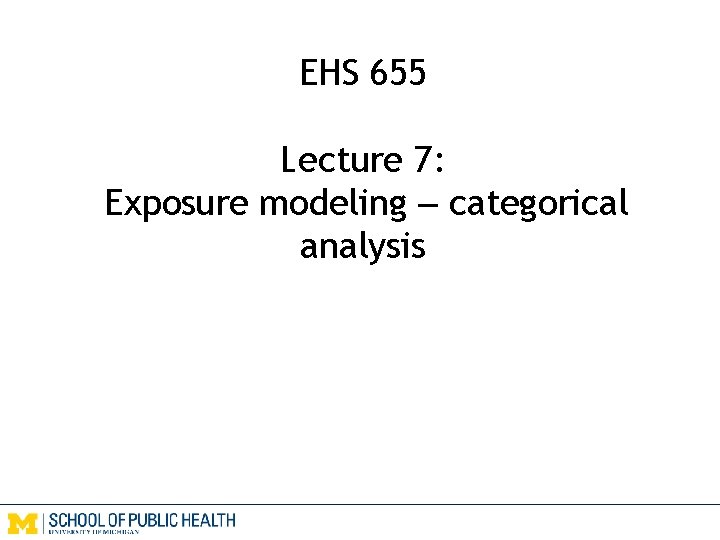
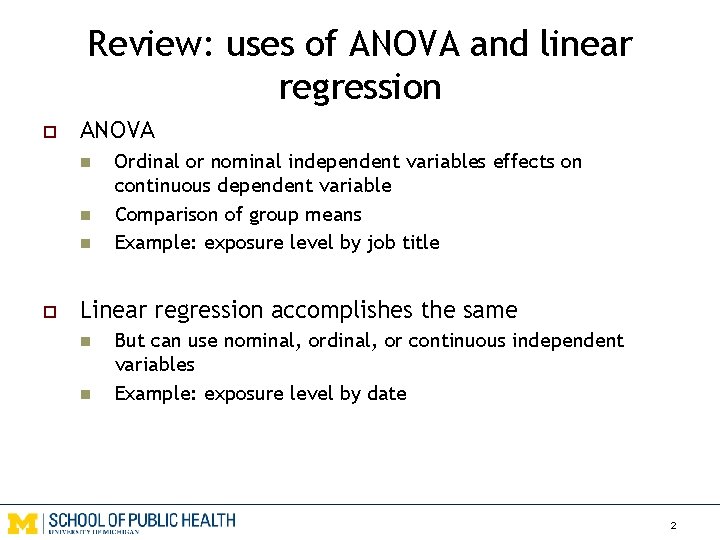
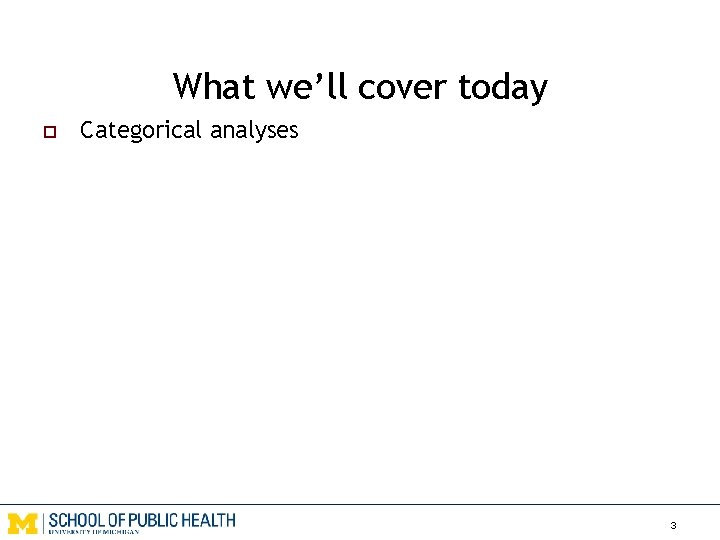
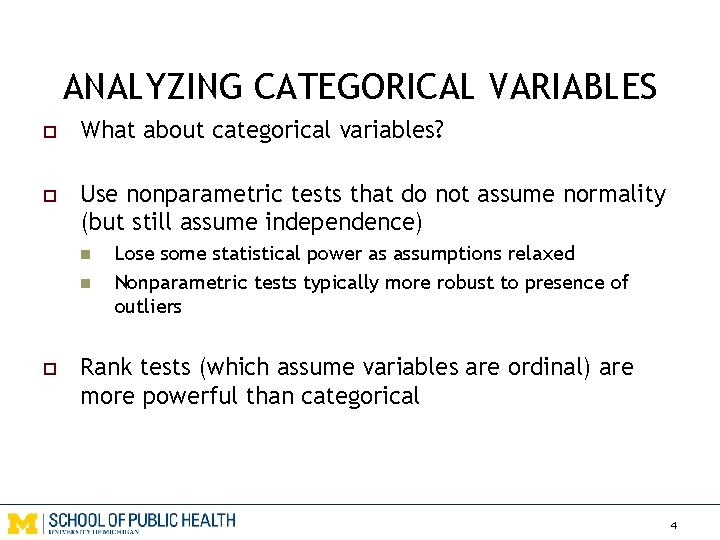
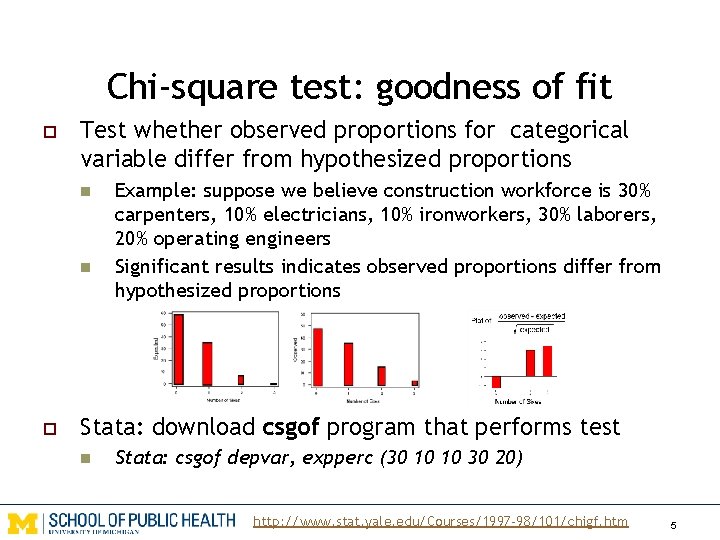
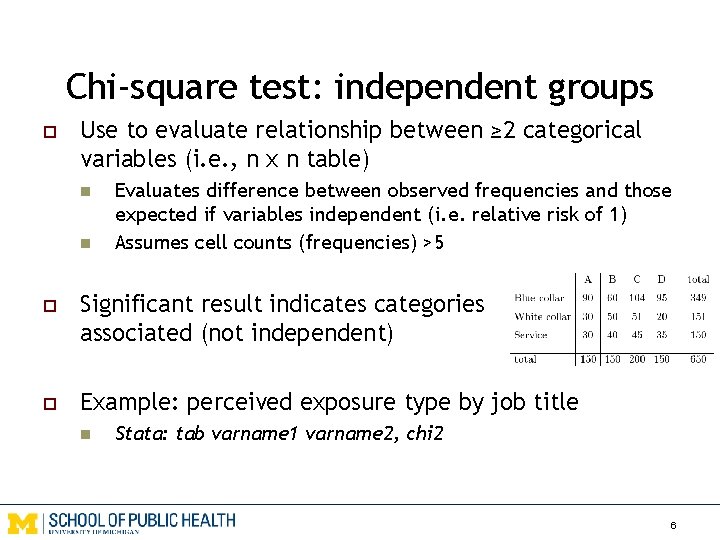
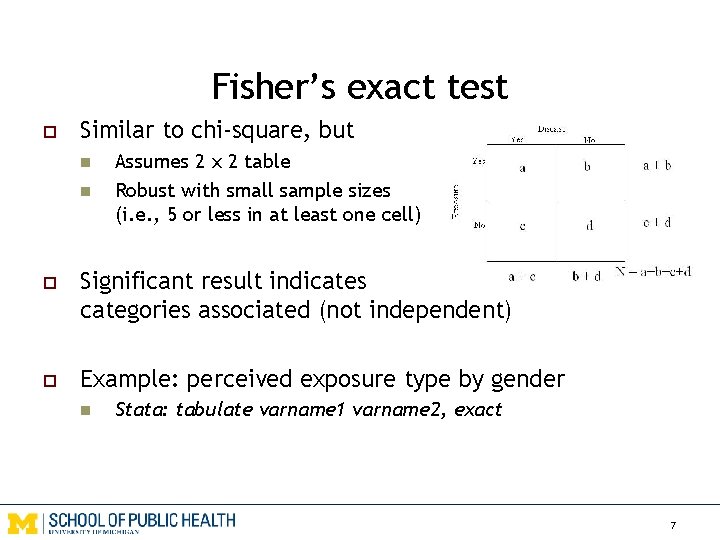
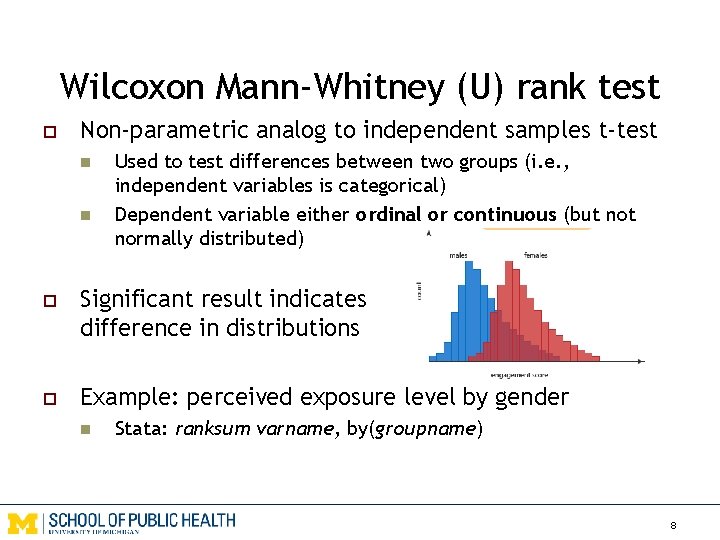
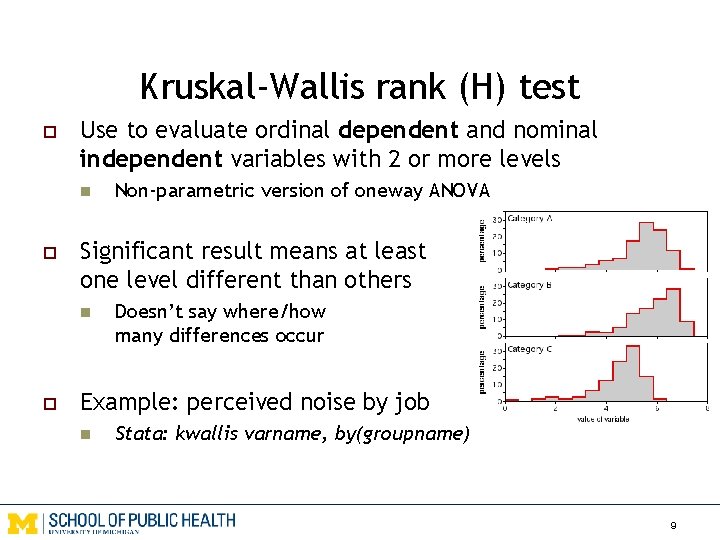
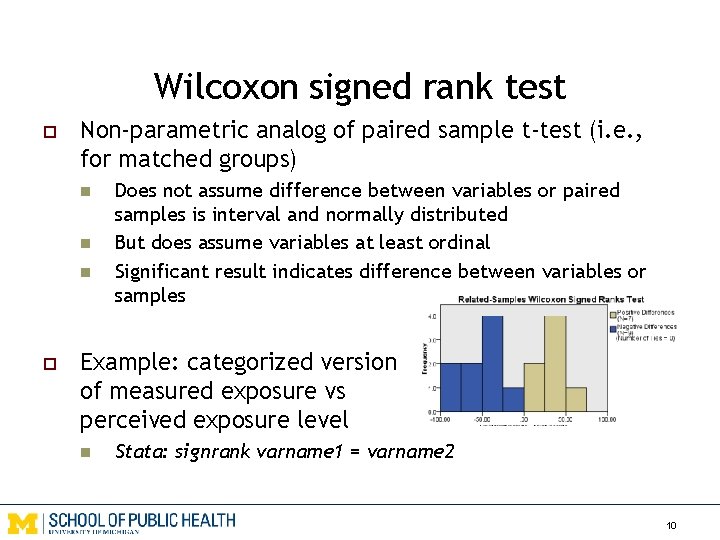
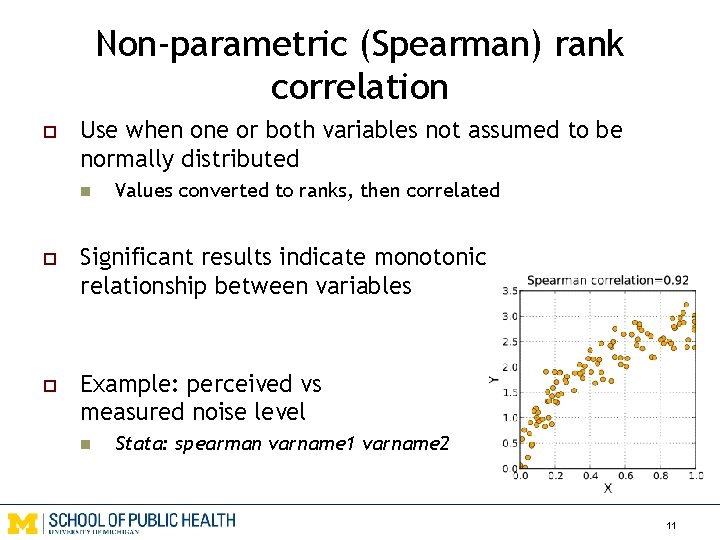
- Slides: 11
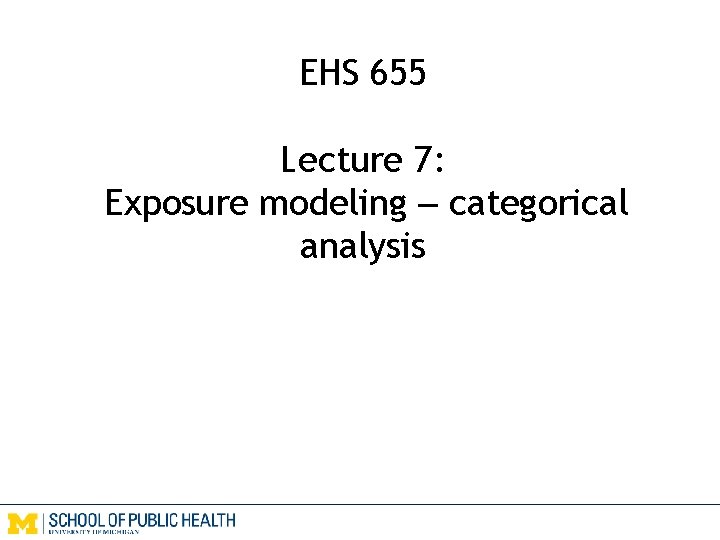
EHS 655 Lecture 7: Exposure modeling – categorical analysis
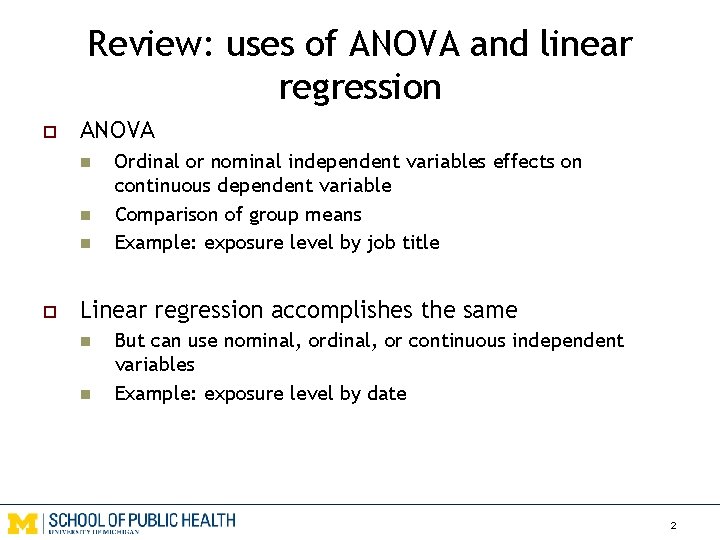
Review: uses of ANOVA and linear regression o ANOVA n n n o Ordinal or nominal independent variables effects on continuous dependent variable Comparison of group means Example: exposure level by job title Linear regression accomplishes the same n n But can use nominal, ordinal, or continuous independent variables Example: exposure level by date 2
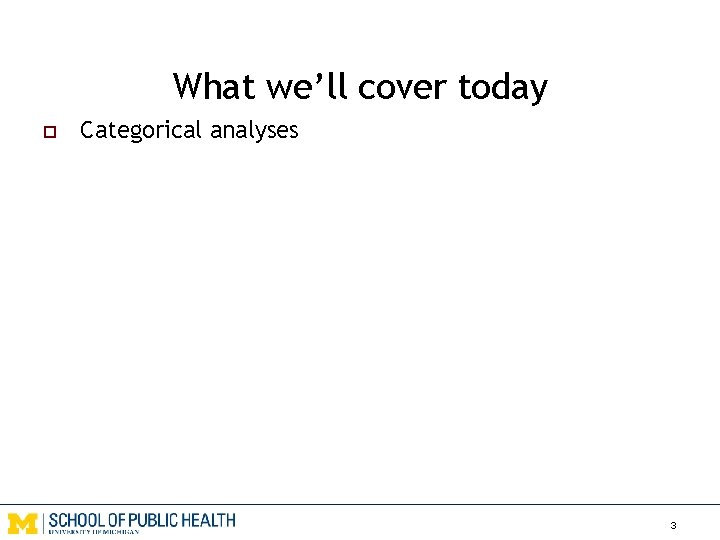
What we’ll cover today o Categorical analyses 3
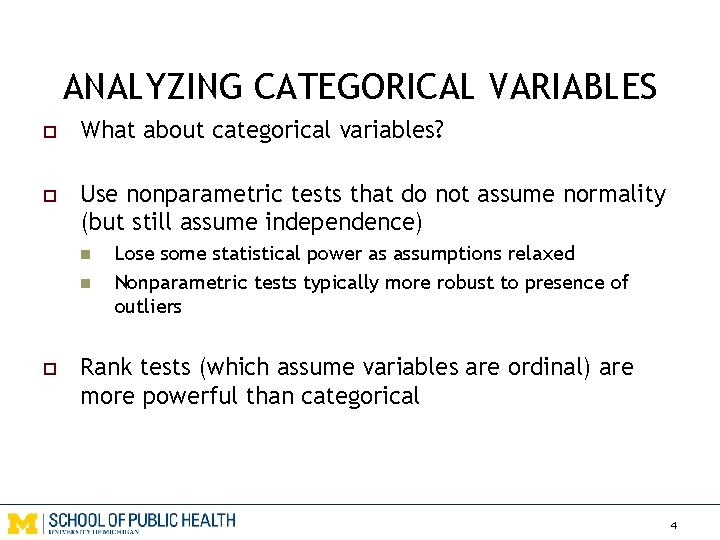
ANALYZING CATEGORICAL VARIABLES o What about categorical variables? o Use nonparametric tests that do not assume normality (but still assume independence) n n o Lose some statistical power as assumptions relaxed Nonparametric tests typically more robust to presence of outliers Rank tests (which assume variables are ordinal) are more powerful than categorical 4
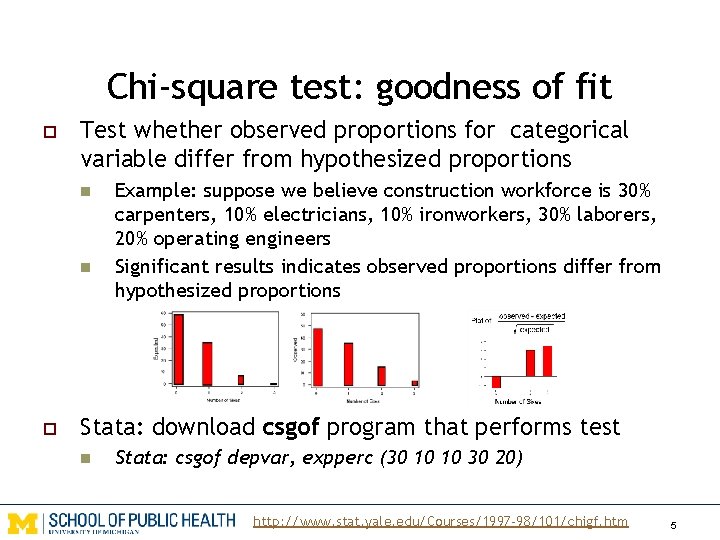
Chi-square test: goodness of fit o Test whether observed proportions for categorical variable differ from hypothesized proportions n n o Example: suppose we believe construction workforce is 30% carpenters, 10% electricians, 10% ironworkers, 30% laborers, 20% operating engineers Significant results indicates observed proportions differ from hypothesized proportions Stata: download csgof program that performs test n Stata: csgof depvar, expperc (30 10 10 30 20) http: //www. stat. yale. edu/Courses/1997 -98/101/chigf. htm 5
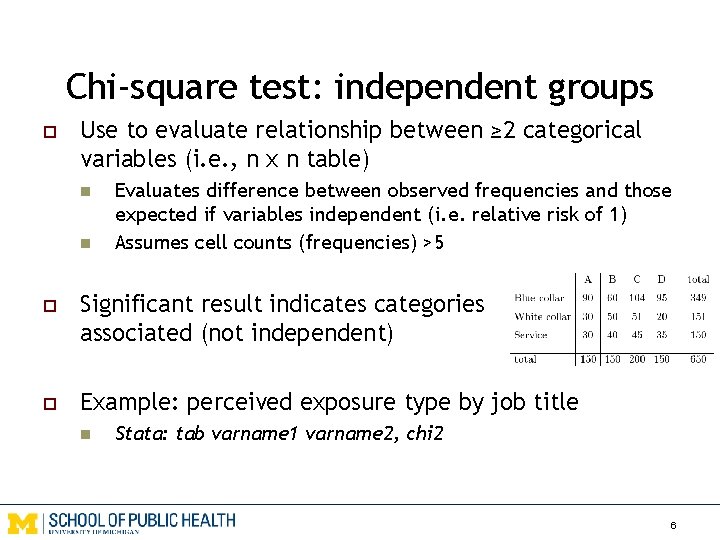
Chi-square test: independent groups o Use to evaluate relationship between ≥ 2 categorical variables (i. e. , n x n table) n n Evaluates difference between observed frequencies and those expected if variables independent (i. e. relative risk of 1) Assumes cell counts (frequencies) >5 o Significant result indicates categories associated (not independent) o Example: perceived exposure type by job title n Stata: tab varname 1 varname 2, chi 2 6
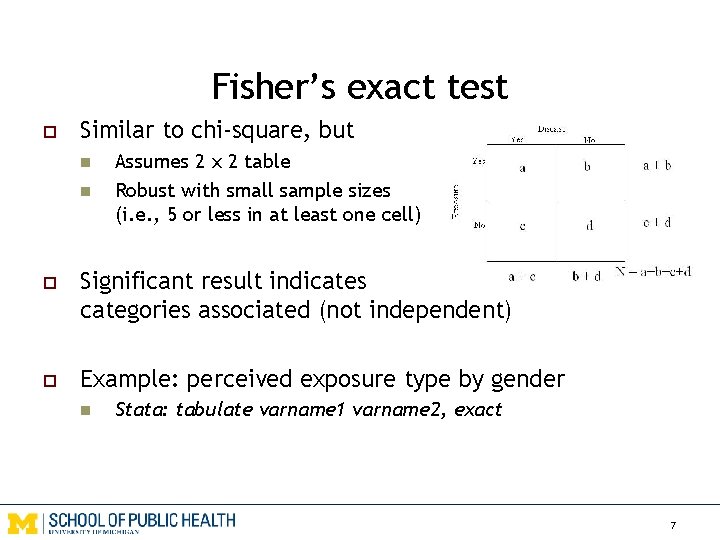
Fisher’s exact test o Similar to chi-square, but n n Assumes 2 x 2 table Robust with small sample sizes (i. e. , 5 or less in at least one cell) o Significant result indicates categories associated (not independent) o Example: perceived exposure type by gender n Stata: tabulate varname 1 varname 2, exact 7
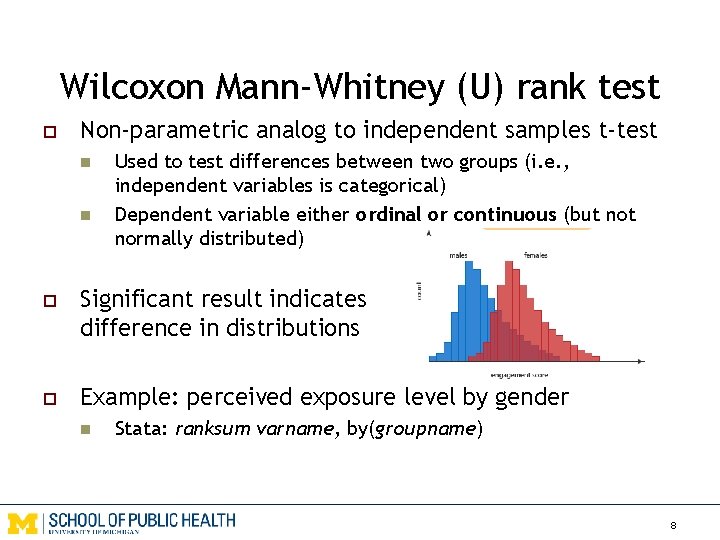
Wilcoxon Mann-Whitney (U) rank test o Non-parametric analog to independent samples t-test n n Used to test differences between two groups (i. e. , independent variables is categorical) Dependent variable either ordinal or continuous (but normally distributed) o Significant result indicates difference in distributions o Example: perceived exposure level by gender n Stata: ranksum varname, by(groupname) 8
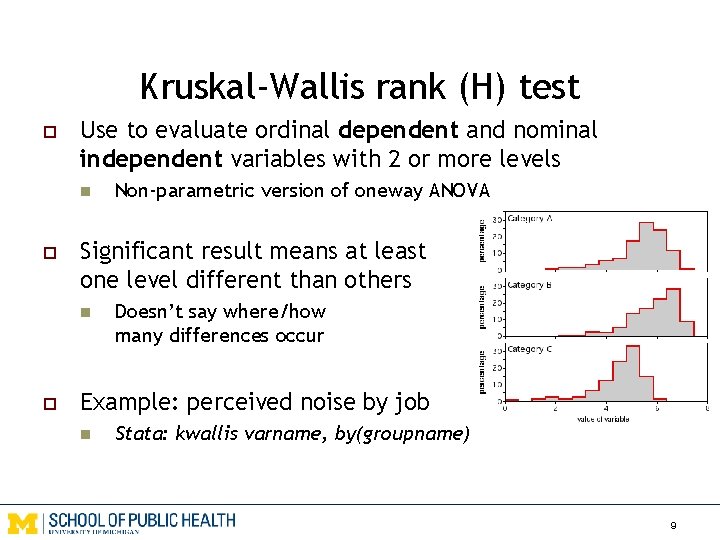
Kruskal-Wallis rank (H) test o Use to evaluate ordinal dependent and nominal independent variables with 2 or more levels n o Significant result means at least one level different than others n o Non-parametric version of oneway ANOVA Doesn’t say where/how many differences occur Example: perceived noise by job n Stata: kwallis varname, by(groupname) 9
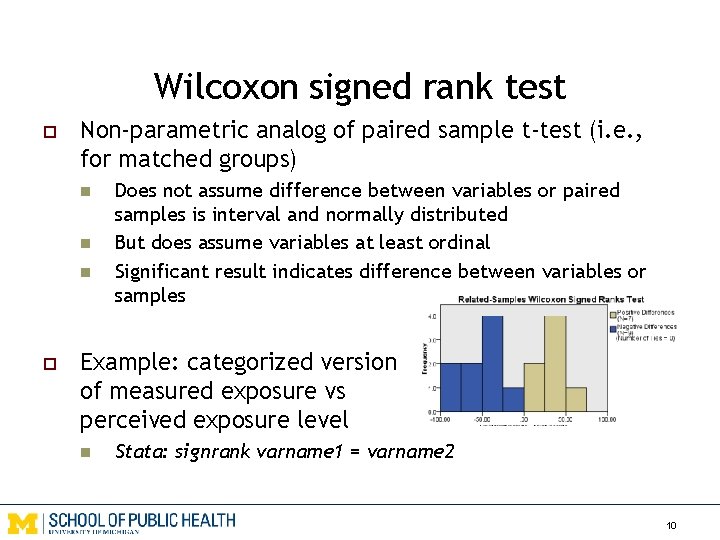
Wilcoxon signed rank test o Non-parametric analog of paired sample t-test (i. e. , for matched groups) n n n o Does not assume difference between variables or paired samples is interval and normally distributed But does assume variables at least ordinal Significant result indicates difference between variables or samples Example: categorized version of measured exposure vs perceived exposure level n Stata: signrank varname 1 = varname 2 10
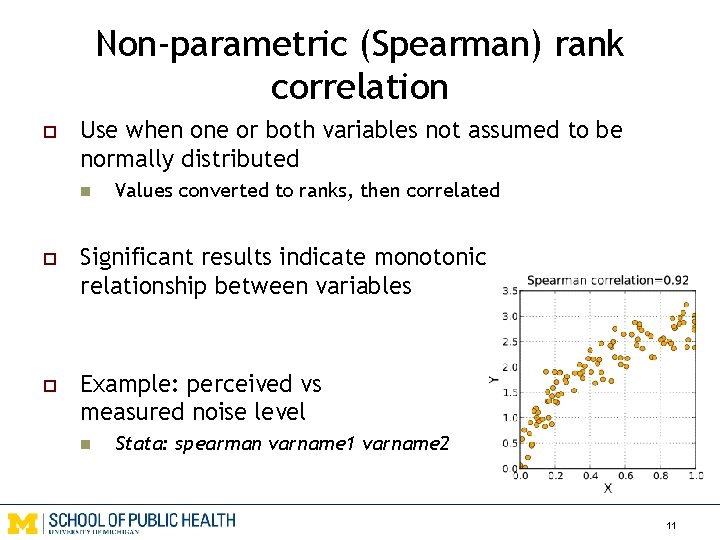
Non-parametric (Spearman) rank correlation o Use when one or both variables not assumed to be normally distributed n Values converted to ranks, then correlated o Significant results indicate monotonic relationship between variables o Example: perceived vs measured noise level n Stata: spearman varname 1 varname 2 11
Managing economic exposure and translation exposure
Eksposur translasi adalah
What is economic exposure
Managing economic exposure and translation exposure
Marc 655
Ba655
Norsafe mako 655
Helen erickson nursing theory
Relational vs dimensional data modeling
01:640:244 lecture notes - lecture 15: plat, idah, farad
Categorical logic examples in nursing
Ehs mit