Concretizing fixed and random effects Some folks for
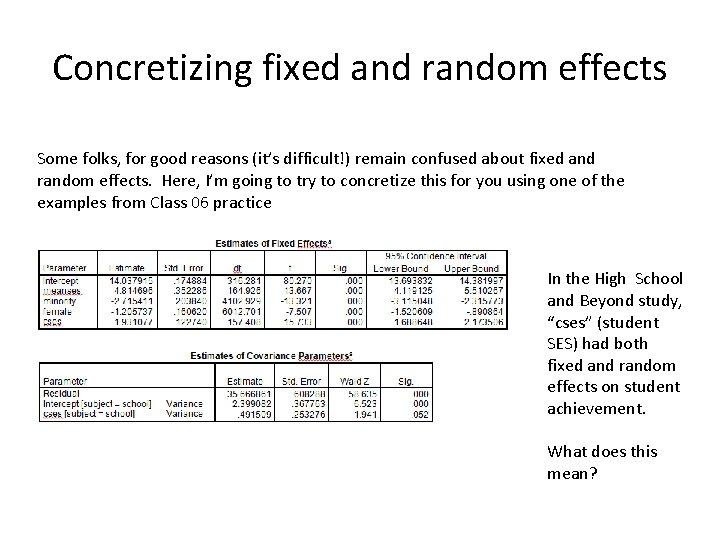
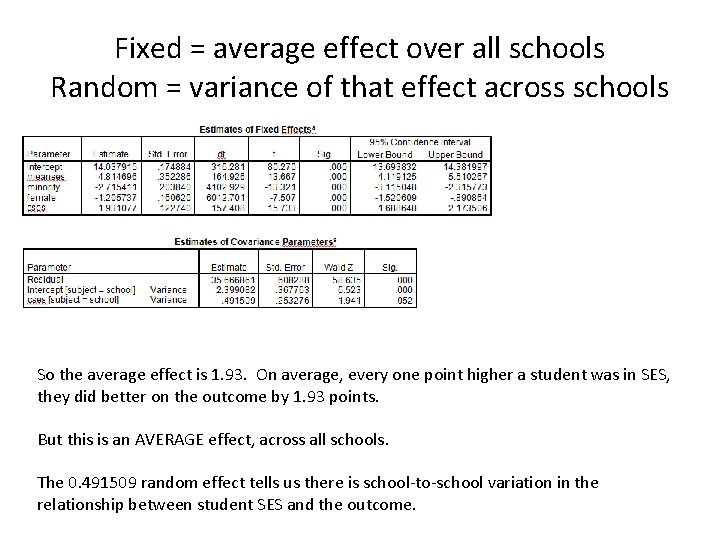
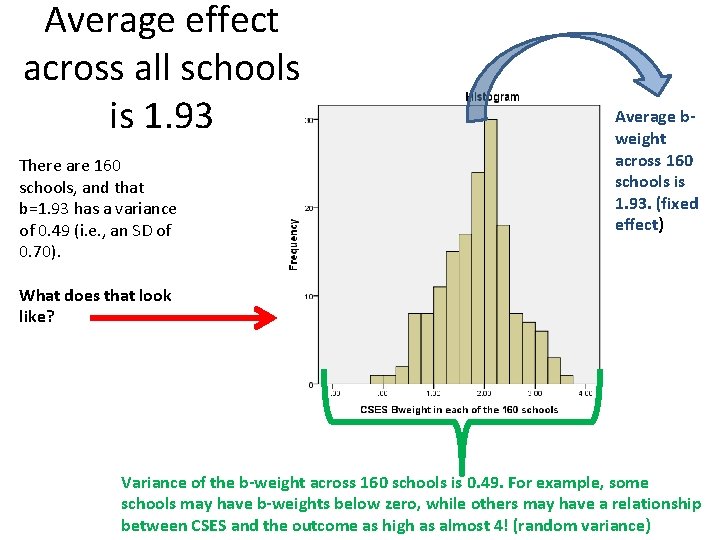
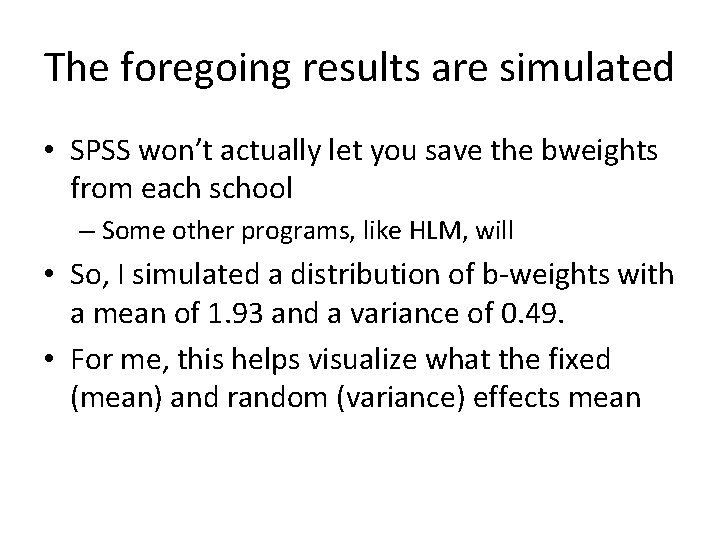
- Slides: 4
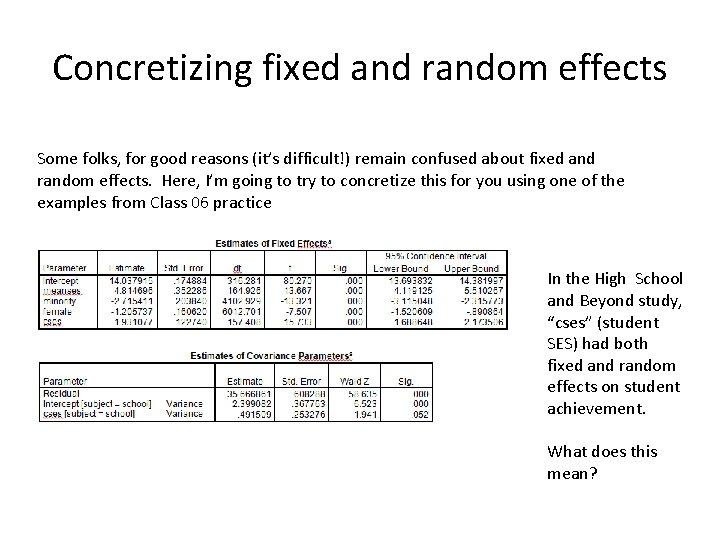
Concretizing fixed and random effects Some folks, for good reasons (it’s difficult!) remain confused about fixed and random effects. Here, I’m going to try to concretize this for you using one of the examples from Class 06 practice In the High School and Beyond study, “cses” (student SES) had both fixed and random effects on student achievement. What does this mean?
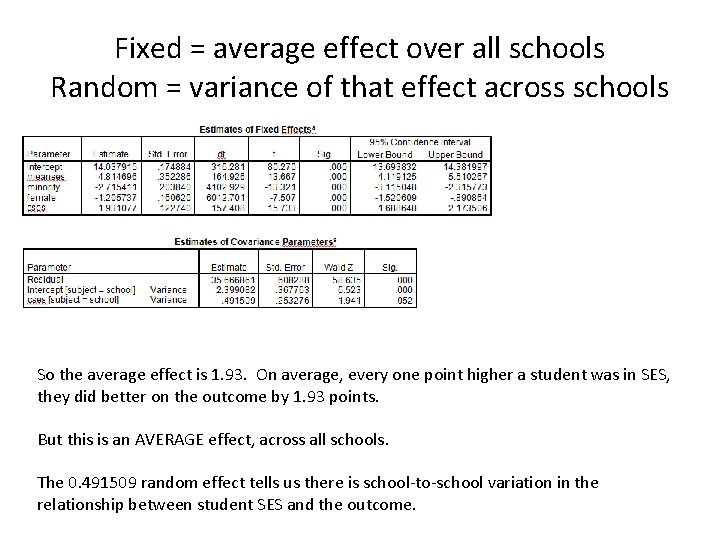
Fixed = average effect over all schools Random = variance of that effect across schools So the average effect is 1. 93. On average, every one point higher a student was in SES, they did better on the outcome by 1. 93 points. But this is an AVERAGE effect, across all schools. The 0. 491509 random effect tells us there is school-to-school variation in the relationship between student SES and the outcome.
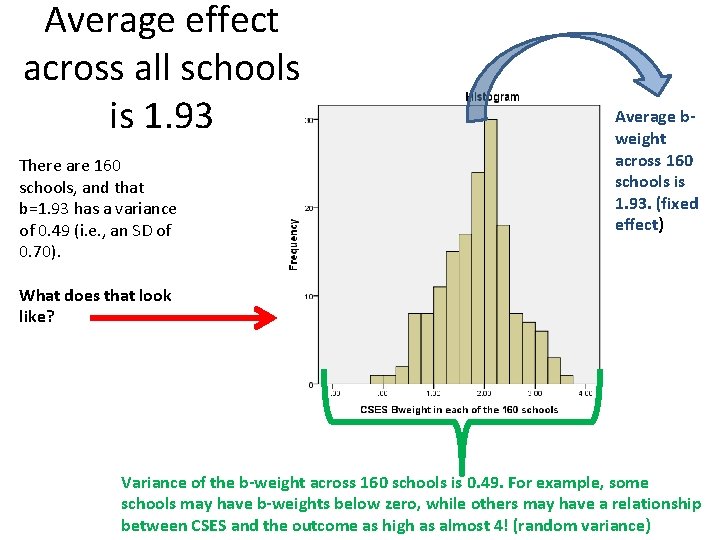
Average effect across all schools is 1. 93 There are 160 schools, and that b=1. 93 has a variance of 0. 49 (i. e. , an SD of 0. 70). Average bweight across 160 schools is 1. 93. (fixed effect) What does that look like? Variance of the b-weight across 160 schools is 0. 49. For example, some schools may have b-weights below zero, while others may have a relationship between CSES and the outcome as high as almost 4! (random variance)
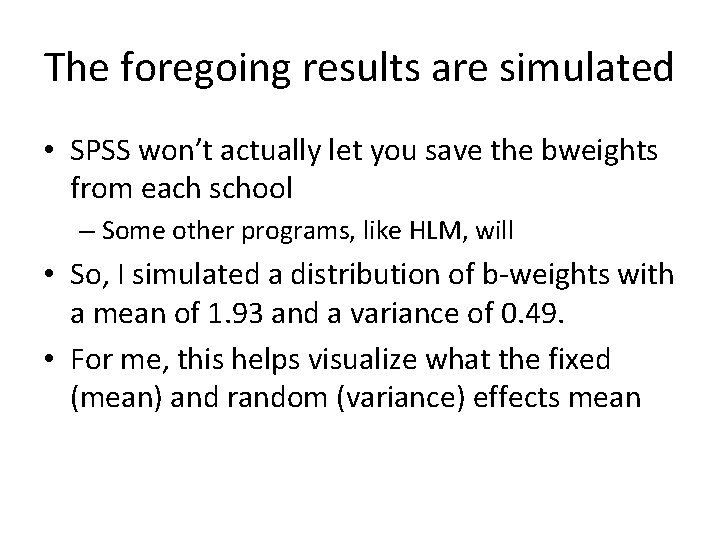
The foregoing results are simulated • SPSS won’t actually let you save the bweights from each school – Some other programs, like HLM, will • So, I simulated a distribution of b-weights with a mean of 1. 93 and a variance of 0. 49. • For me, this helps visualize what the fixed (mean) and random (variance) effects mean