Anan Garg Johana Rodriguez Thore Koch Vineela Datla
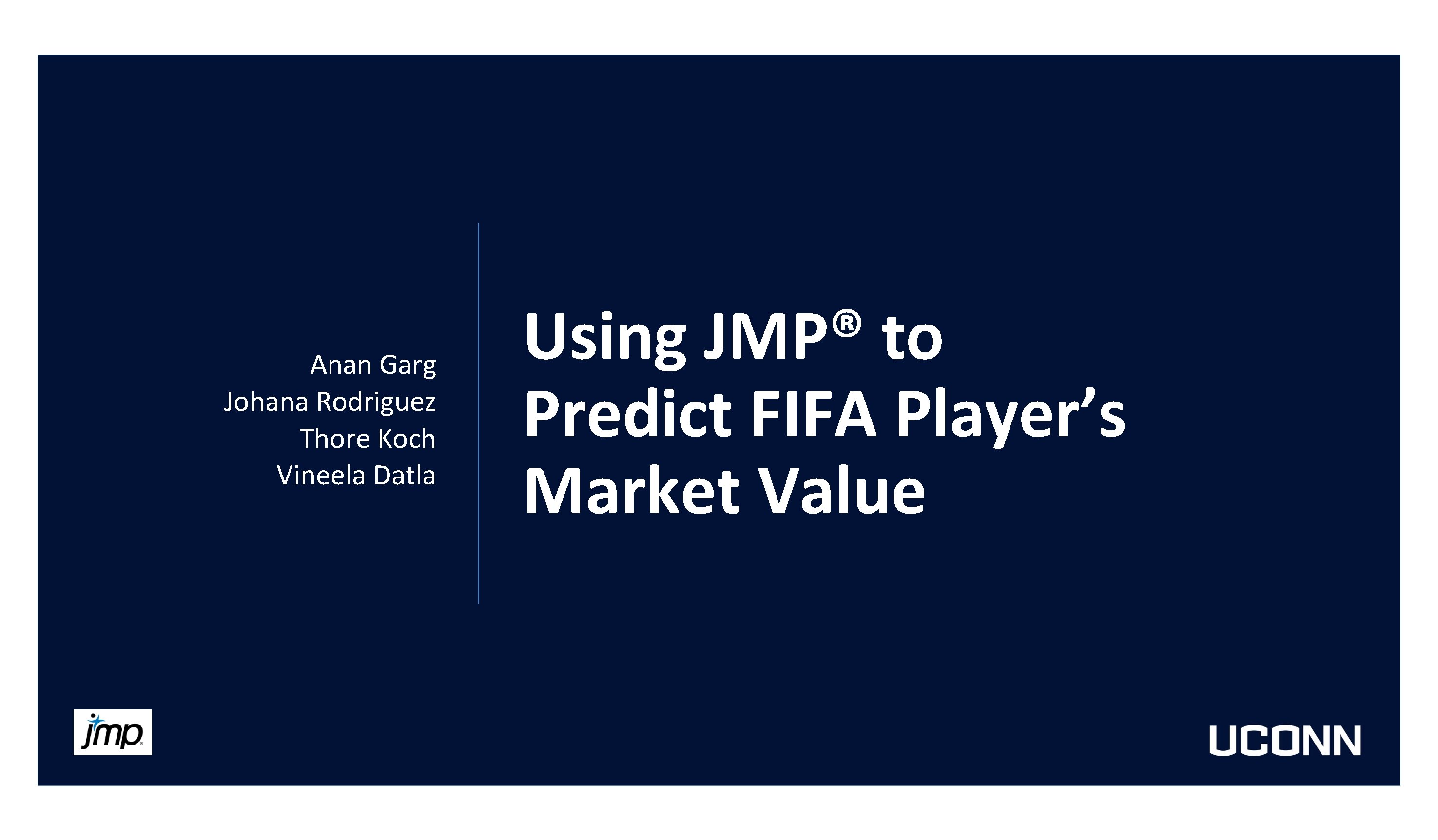
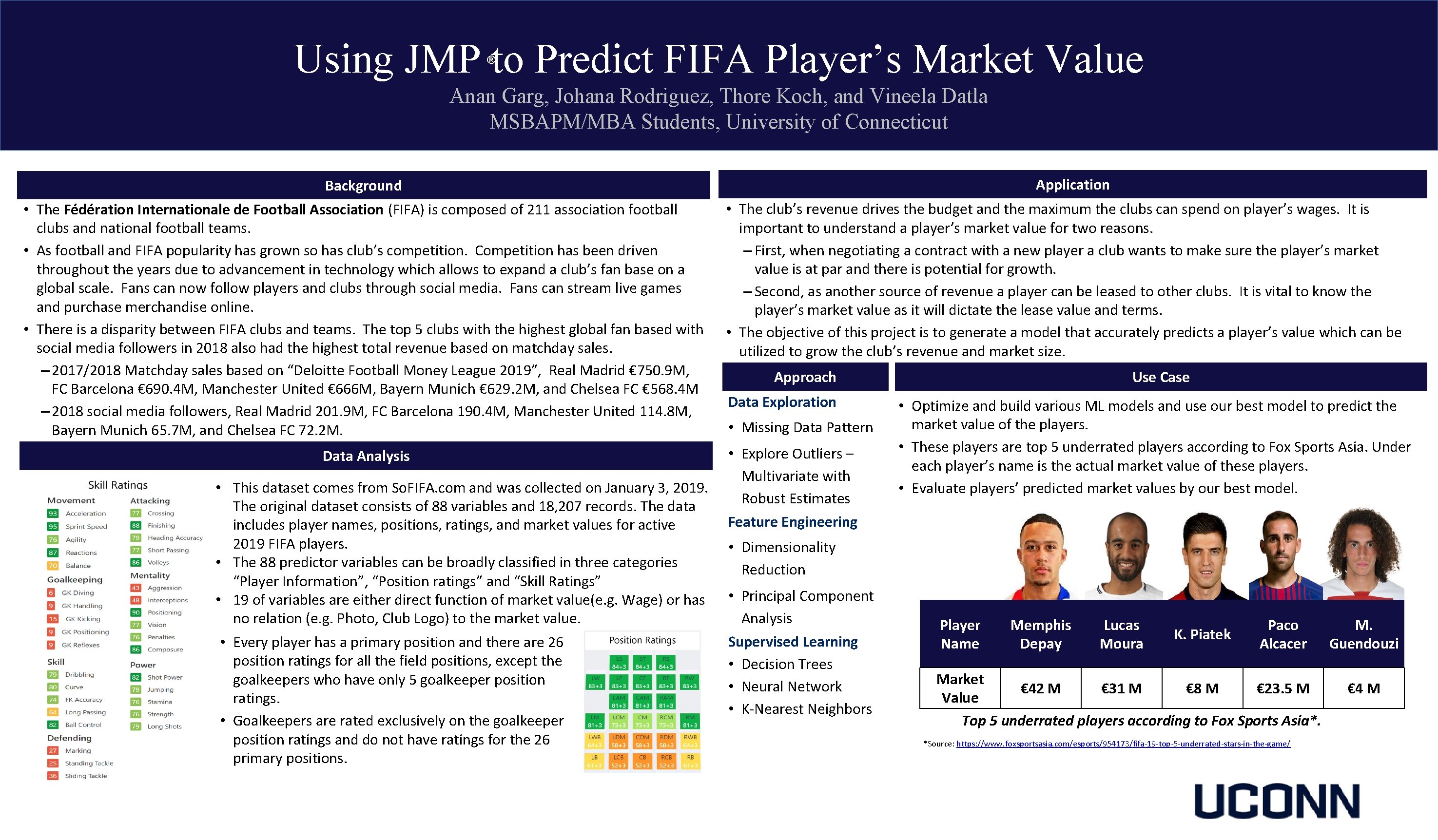
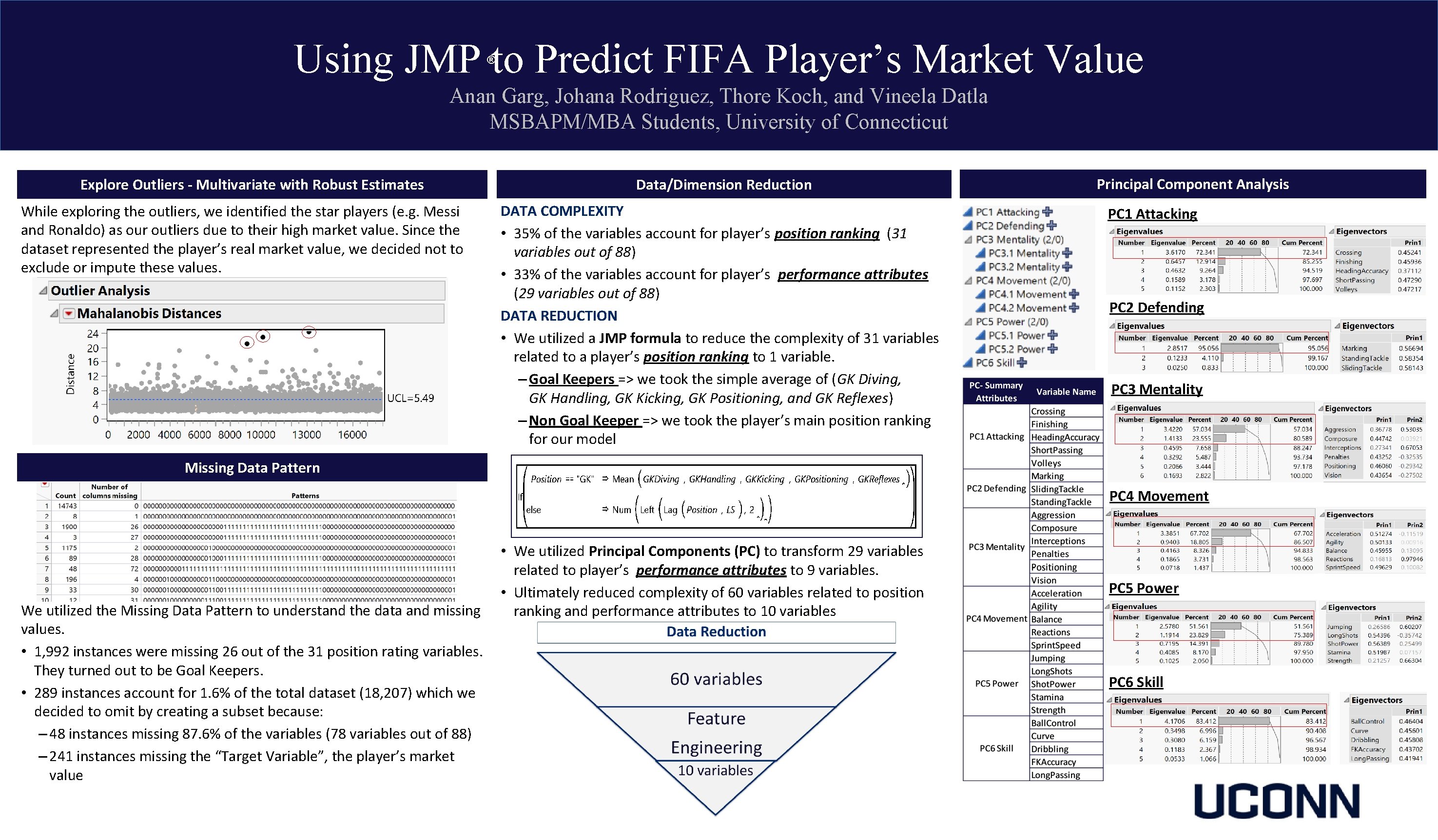
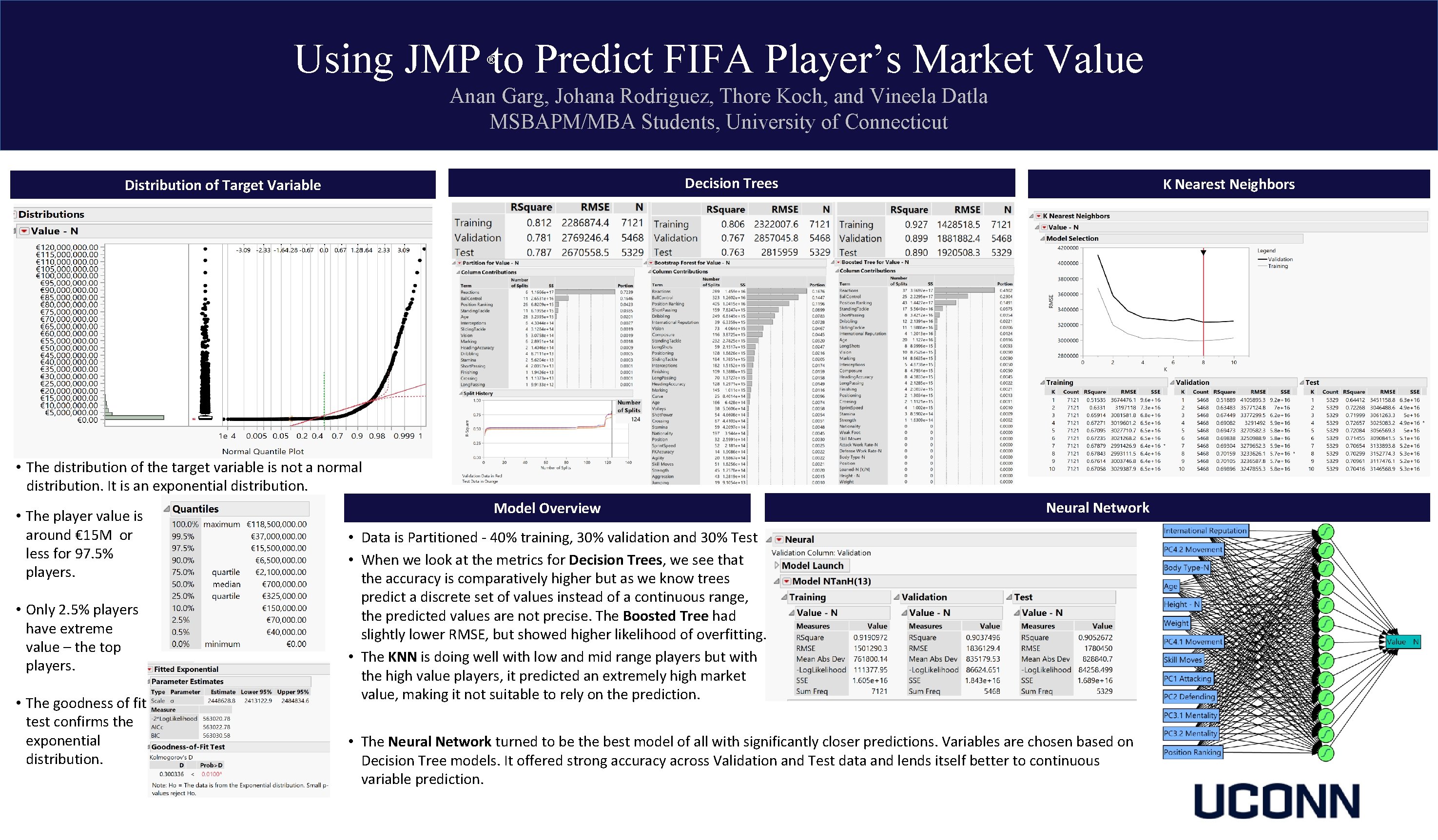
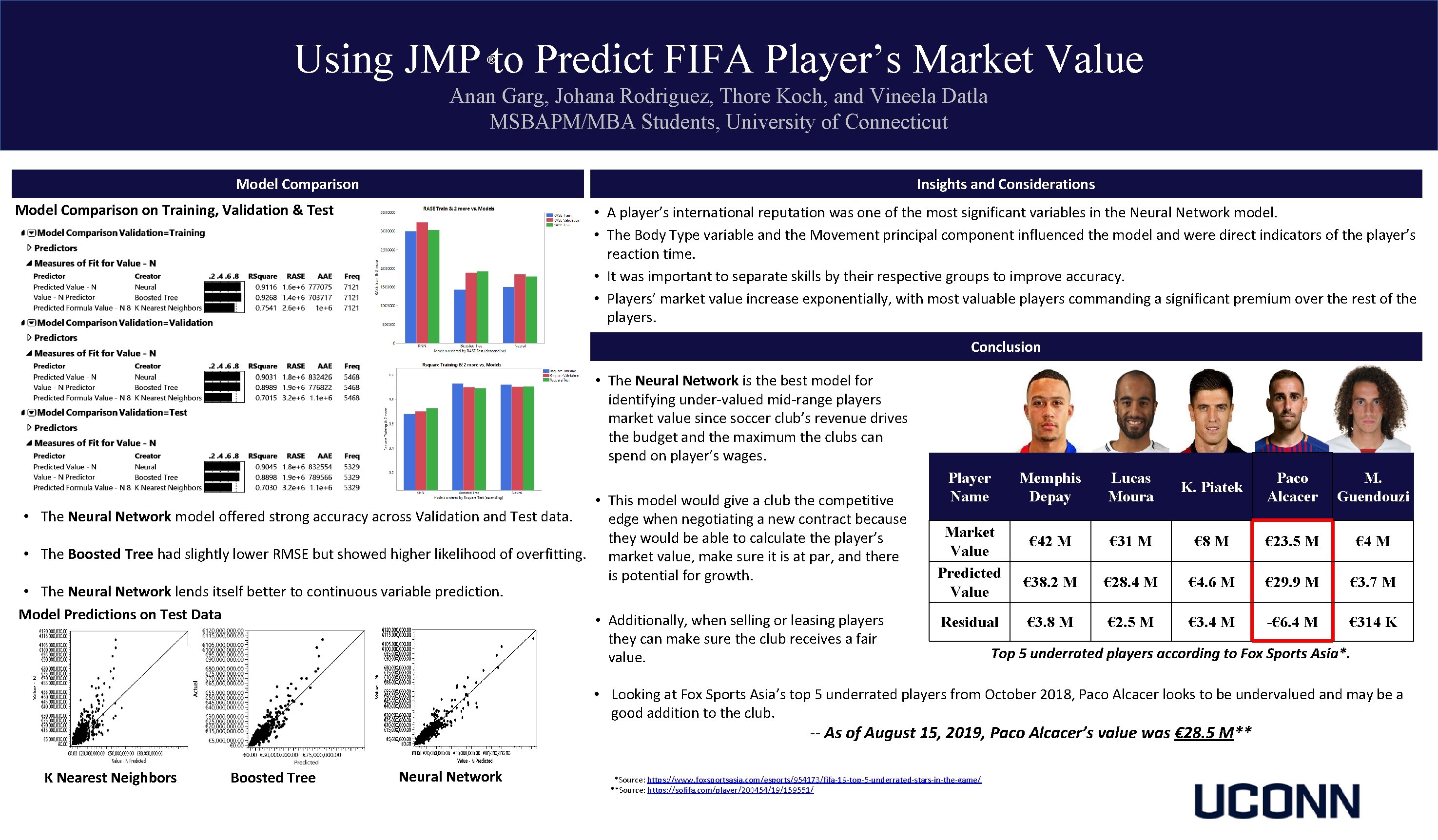
- Slides: 5
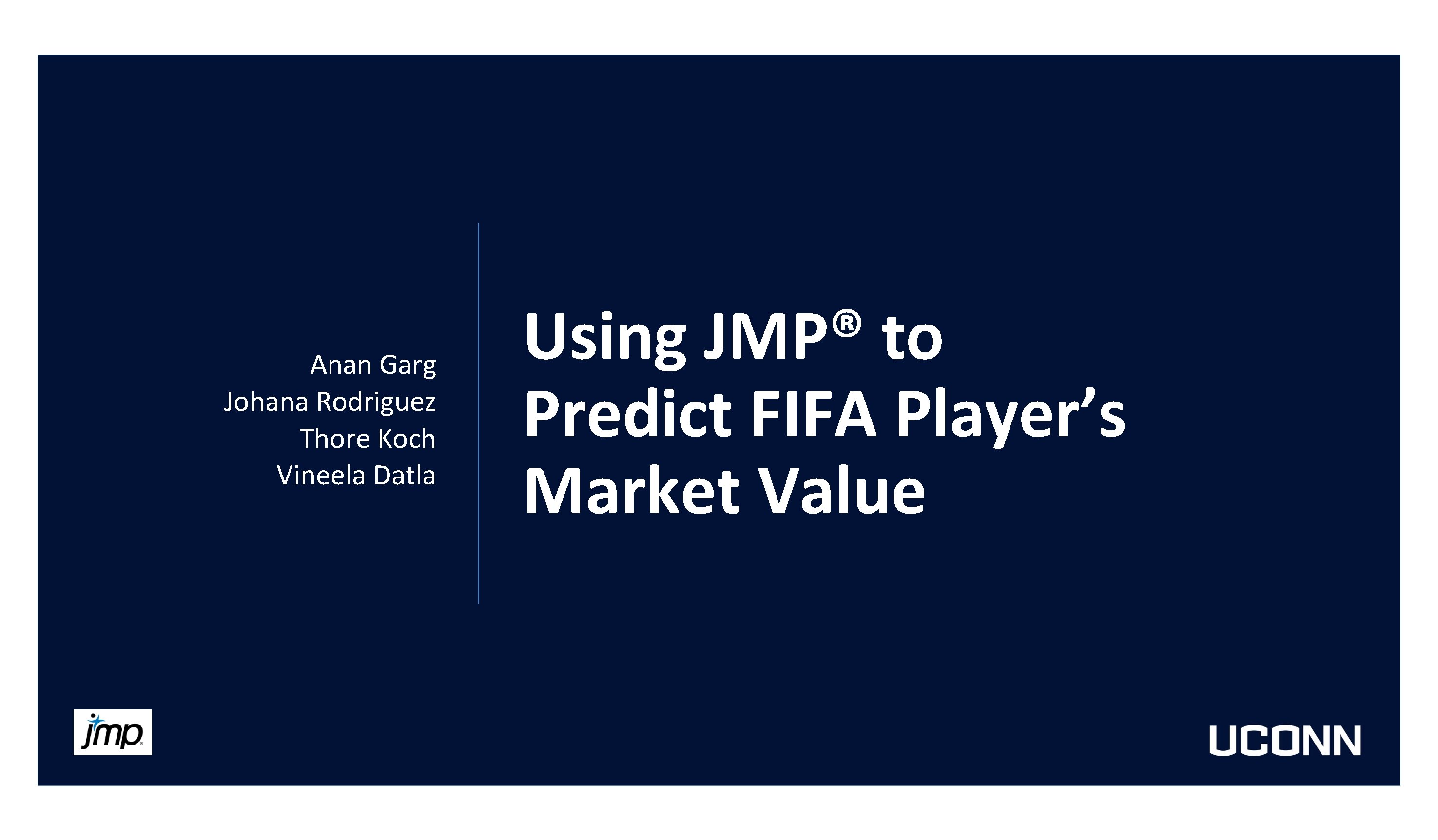
Anan Garg Johana Rodriguez Thore Koch Vineela Datla Using JMP® to Predict FIFA Player’s Market Value
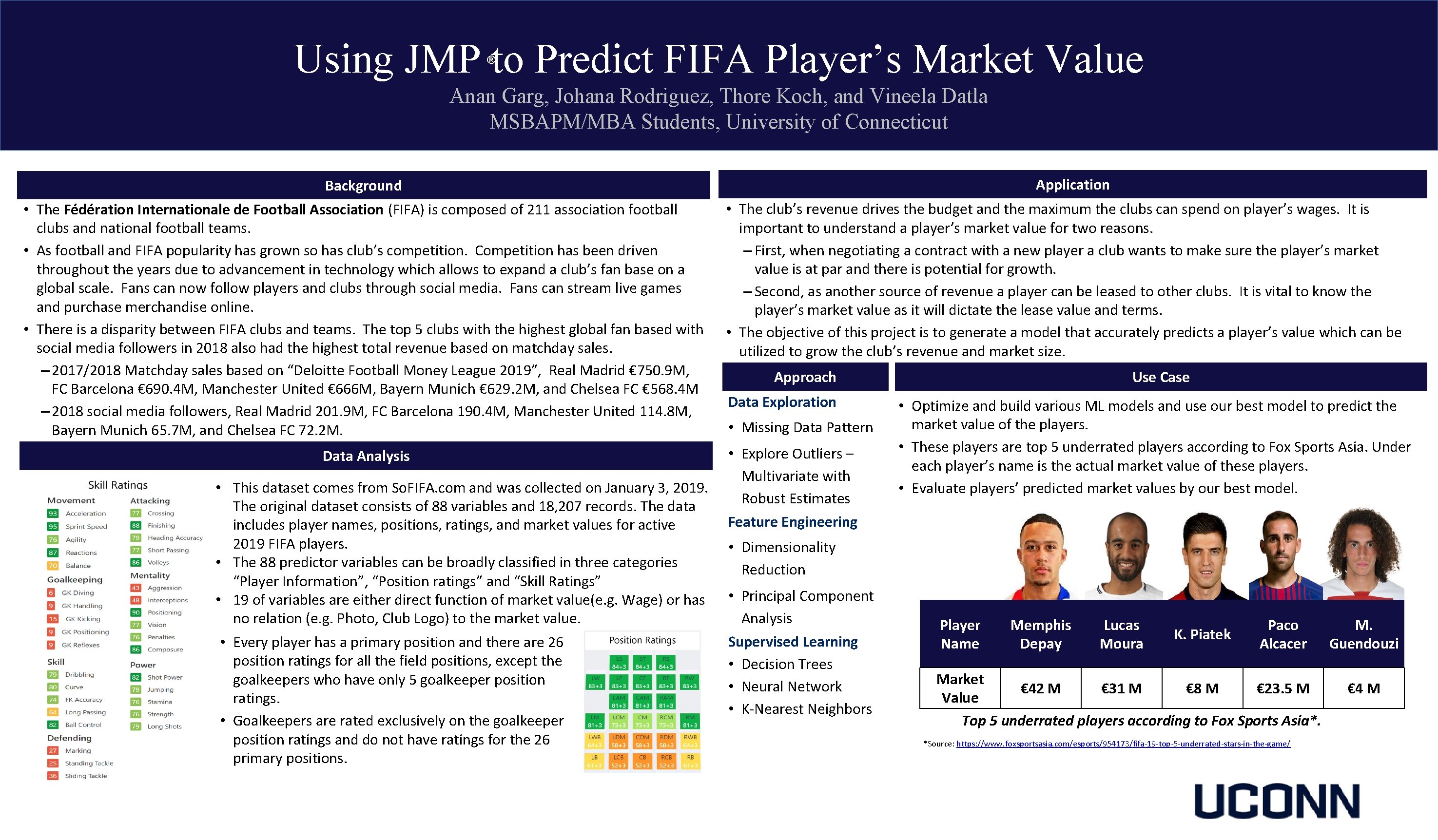
Using JMP to Predict FIFA Player’s Market Value ® Anan Garg, Johana Rodriguez, Thore Koch, and Vineela Datla MSBAPM/MBA Students, University of Connecticut Application Background • The Fédération Internationale de Football Association (FIFA) is composed of 211 association football clubs and national football teams. • As football and FIFA popularity has grown so has club’s competition. Competition has been driven throughout the years due to advancement in technology which allows to expand a club’s fan base on a global scale. Fans can now follow players and clubs through social media. Fans can stream live games and purchase merchandise online. • There is a disparity between FIFA clubs and teams. The top 5 clubs with the highest global fan based with social media followers in 2018 also had the highest total revenue based on matchday sales. – 2017/2018 Matchday sales based on “Deloitte Football Money League 2019”, Real Madrid € 750. 9 M, FC Barcelona € 690. 4 M, Manchester United € 666 M, Bayern Munich € 629. 2 M, and Chelsea FC € 568. 4 M – 2018 social media followers, Real Madrid 201. 9 M, FC Barcelona 190. 4 M, Manchester United 114. 8 M, Bayern Munich 65. 7 M, and Chelsea FC 72. 2 M. Data Analysis • This dataset comes from So. FIFA. com and was collected on January 3, 2019. The original dataset consists of 88 variables and 18, 207 records. The data includes player names, positions, ratings, and market values for active 2019 FIFA players. • The 88 predictor variables can be broadly classified in three categories “Player Information”, “Position ratings” and “Skill Ratings” • 19 of variables are either direct function of market value(e. g. Wage) or has no relation (e. g. Photo, Club Logo) to the market value. • Every player has a primary position and there are 26 position ratings for all the field positions, except the goalkeepers who have only 5 goalkeeper position ratings. • Goalkeepers are rated exclusively on the goalkeeper position ratings and do not have ratings for the 26 primary positions. • The club’s revenue drives the budget and the maximum the clubs can spend on player’s wages. It is important to understand a player’s market value for two reasons. – First, when negotiating a contract with a new player a club wants to make sure the player’s market value is at par and there is potential for growth. – Second, as another source of revenue a player can be leased to other clubs. It is vital to know the player’s market value as it will dictate the lease value and terms. • The objective of this project is to generate a model that accurately predicts a player’s value which can be utilized to grow the club’s revenue and market size. Approach Data Exploration • Missing Data Pattern • Explore Outliers – Multivariate with Robust Estimates Feature Engineering Use Case • Optimize and build various ML models and use our best model to predict the market value of the players. • These players are top 5 underrated players according to Fox Sports Asia. Under each player’s name is the actual market value of these players. • Evaluate players’ predicted market values by our best model. • Dimensionality Reduction • Principal Component Analysis Supervised Learning • Decision Trees • Neural Network • K-Nearest Neighbors Player Name Memphis Depay Lucas Moura K. Piatek Paco Alcacer Market € 42 M € 31 M € 8 M € 23. 5 M Value Top 5 underrated players according to Fox Sports Asia*. *Source: https: //www. foxsportsasia. com/esports/954173/fifa-19 -top-5 -underrated-stars-in-the-game/ M. Guendouzi € 4 M
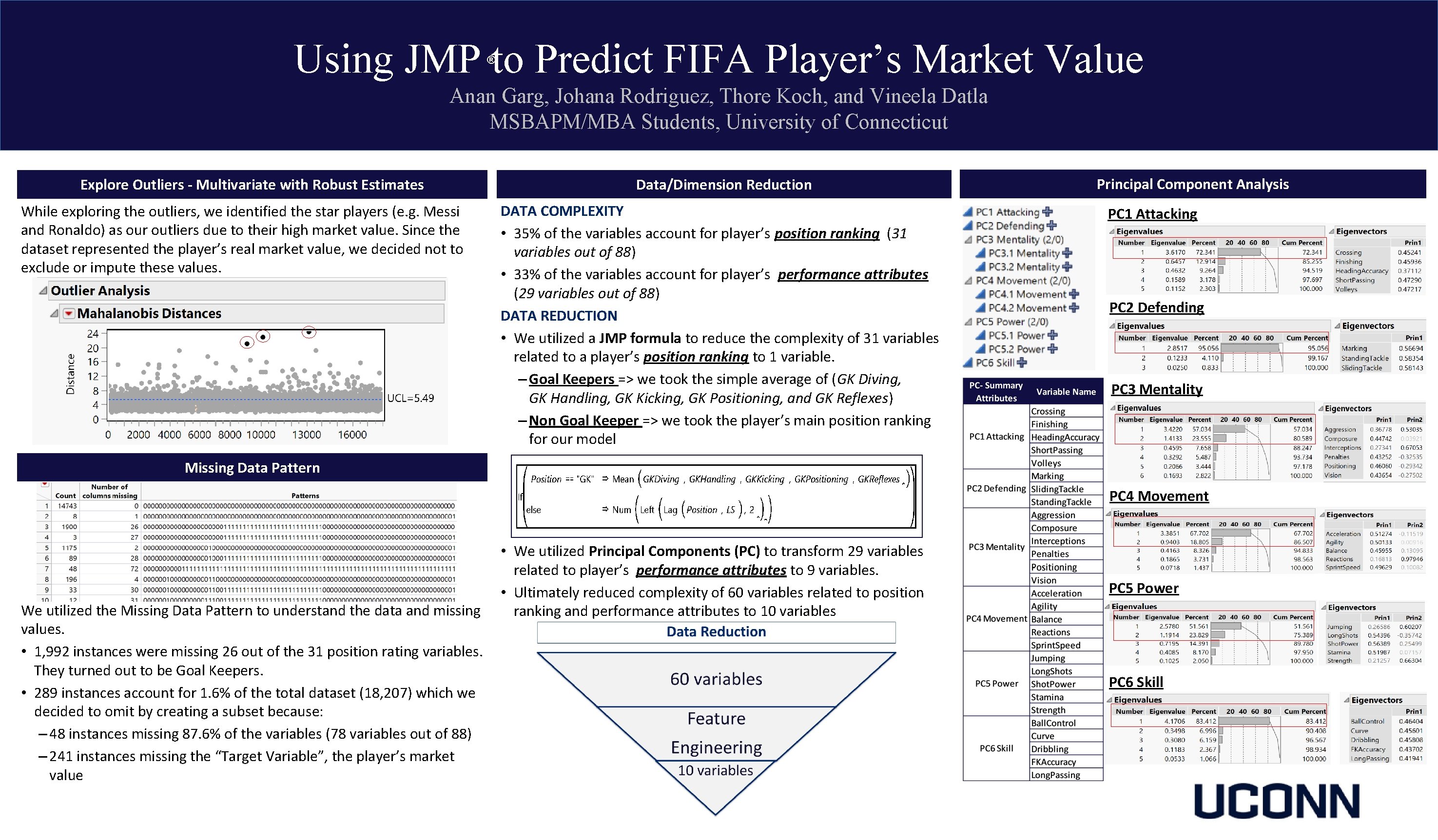
Using JMP to Predict FIFA Player’s Market Value ® Anan Garg, Johana Rodriguez, Thore Koch, and Vineela Datla MSBAPM/MBA Students, University of Connecticut Explore Outliers - Multivariate with Robust Estimates While exploring the outliers, we identified the star players (e. g. Messi and Ronaldo) as our outliers due to their high market value. Since the dataset represented the player’s real market value, we decided not to exclude or impute these values. Data/Dimension Reduction DATA COMPLEXITY • 35% of the variables account for player’s position ranking (31 variables out of 88) • 33% of the variables account for player’s performance attributes (29 variables out of 88) DATA REDUCTION • We utilized a JMP formula to reduce the complexity of 31 variables related to a player’s position ranking to 1 variable. – Goal Keepers => we took the simple average of (GK Diving, GK Handling, GK Kicking, GK Positioning, and GK Reflexes) – Non Goal Keeper => we took the player’s main position ranking for our model Principal Component Analysis PC 1 Attacking PC 2 Defending PC 3 Mentality Missing Data Pattern PC 4 Movement We utilized the Missing Data Pattern to understand the data and missing values. • 1, 992 instances were missing 26 out of the 31 position rating variables. They turned out to be Goal Keepers. • 289 instances account for 1. 6% of the total dataset (18, 207) which we decided to omit by creating a subset because: – 48 instances missing 87. 6% of the variables (78 variables out of 88) – 241 instances missing the “Target Variable”, the player’s market value • We utilized Principal Components (PC) to transform 29 variables related to player’s performance attributes to 9 variables. • Ultimately reduced complexity of 60 variables related to position ranking and performance attributes to 10 variables PC 5 Power PC 6 Skill
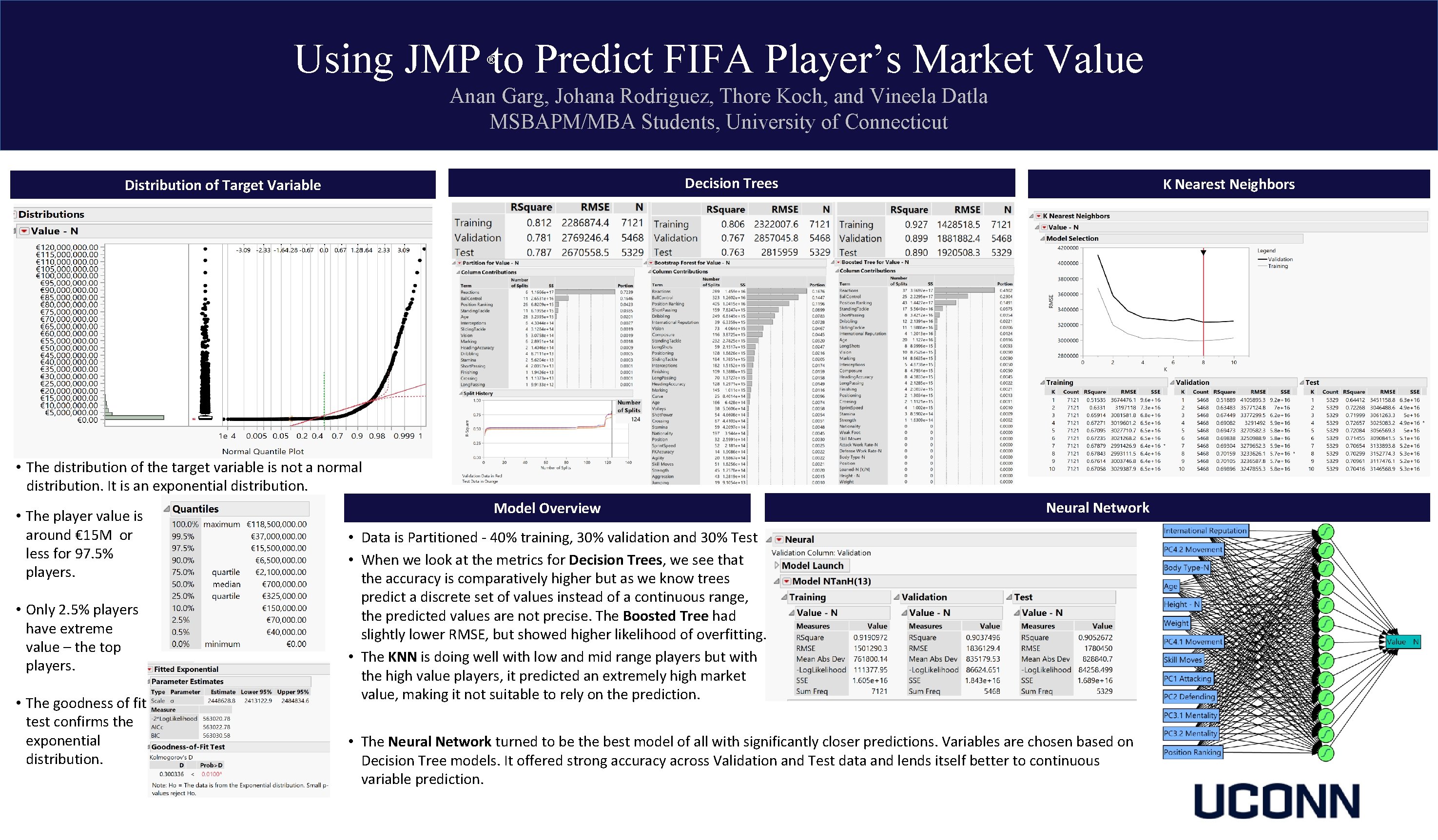
Using JMP to Predict FIFA Player’s Market Value ® Anan Garg, Johana Rodriguez, Thore Koch, and Vineela Datla MSBAPM/MBA Students, University of Connecticut Decision Trees Distribution of Target Variable K Nearest Neighbors • The distribution of the target variable is not a normal distribution. It is an exponential distribution. • The player value is around € 15 M or less for 97. 5% players. • Only 2. 5% players have extreme value – the top players. • The goodness of fit test confirms the exponential distribution. Model Overview Neural Network • Data is Partitioned - 40% training, 30% validation and 30% Test • When we look at the metrics for Decision Trees, we see that the accuracy is comparatively higher but as we know trees predict a discrete set of values instead of a continuous range, the predicted values are not precise. The Boosted Tree had slightly lower RMSE, but showed higher likelihood of overfitting. • The KNN is doing well with low and mid range players but with the high value players, it predicted an extremely high market value, making it not suitable to rely on the prediction. • The Neural Network turned to be the best model of all with significantly closer predictions. Variables are chosen based on Decision Tree models. It offered strong accuracy across Validation and Test data and lends itself better to continuous variable prediction.
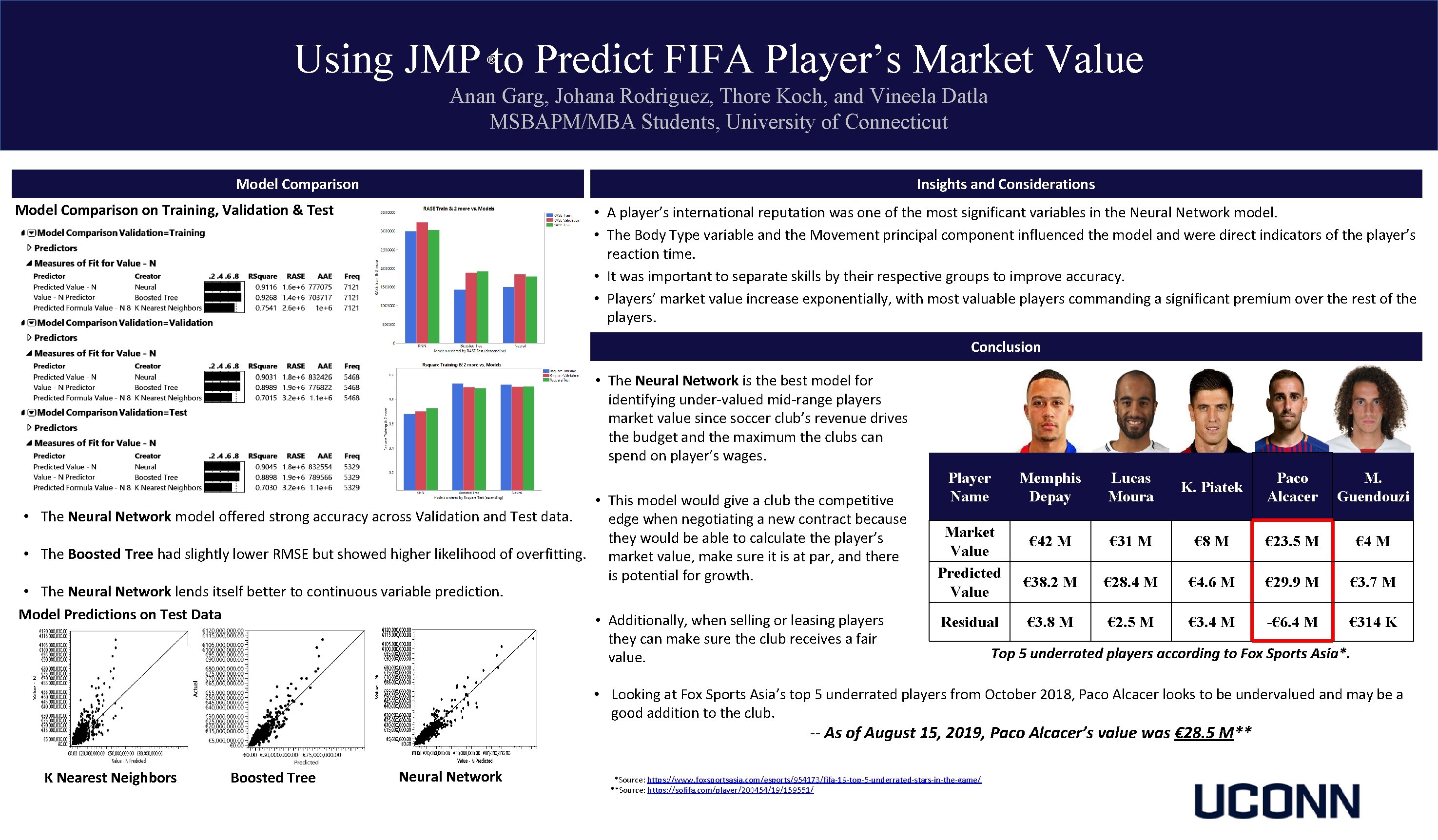
Using JMP to Predict FIFA Player’s Market Value ® Anan Garg, Johana Rodriguez, Thore Koch, and Vineela Datla MSBAPM/MBA Students, University of Connecticut Model Comparison Insights and Considerations Model Comparison on Training, Validation & Test • A player’s international reputation was one of the most significant variables in the Neural Network model. • The Body Type variable and the Movement principal component influenced the model and were direct indicators of the player’s reaction time. • It was important to separate skills by their respective groups to improve accuracy. • Players’ market value increase exponentially, with most valuable players commanding a significant premium over the rest of the players. Conclusion • The Neural Network is the best model for identifying under-valued mid-range players market value since soccer club’s revenue drives the budget and the maximum the clubs can spend on player’s wages. • This model would give a club the competitive • The Neural Network model offered strong accuracy across Validation and Test data. edge when negotiating a new contract because they would be able to calculate the player’s • The Boosted Tree had slightly lower RMSE but showed higher likelihood of overfitting. market value, make sure it is at par, and there is potential for growth. • The Neural Network lends itself better to continuous variable prediction. Model Predictions on Test Data • Additionally, when selling or leasing players they can make sure the club receives a fair value. Player Name Market Value Predicted Value Residual Memphis Depay Lucas Moura K. Piatek Paco Alcacer M. Guendouzi € 42 M € 31 M € 8 M € 23. 5 M € 4 M € 38. 2 M € 28. 4 M € 4. 6 M € 29. 9 M € 3. 7 M € 3. 8 M € 2. 5 M € 3. 4 M -€ 6. 4 M € 314 K Top 5 underrated players according to Fox Sports Asia*. • Looking at Fox Sports Asia’s top 5 underrated players from October 2018, Paco Alcacer looks to be undervalued and may be a good addition to the club. -- As of August 15, 2019, Paco Alcacer’s value was € 28. 5 M** K Nearest Neighbors Boosted Tree Neural Network *Source: https: //www. foxsportsasia. com/esports/954173/fifa-19 -top-5 -underrated-stars-in-the-game/ **Source: https: //sofifa. com/player/200454/19/159551/