1 Modeling Diagnostics of A Furnace System SFR
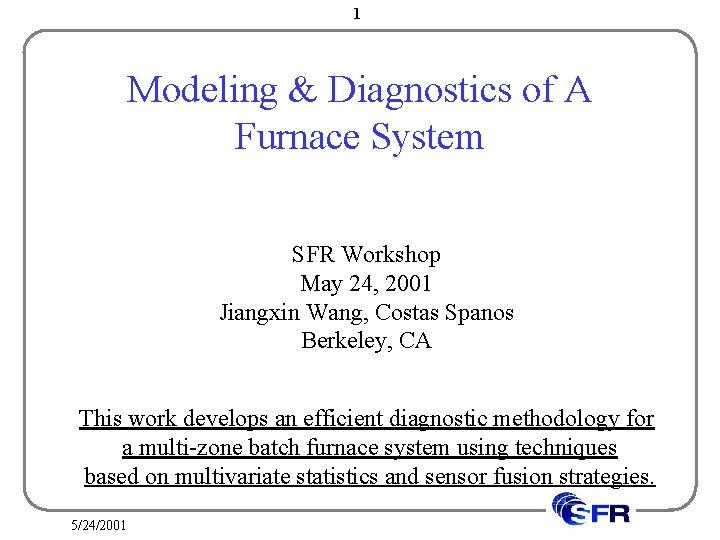
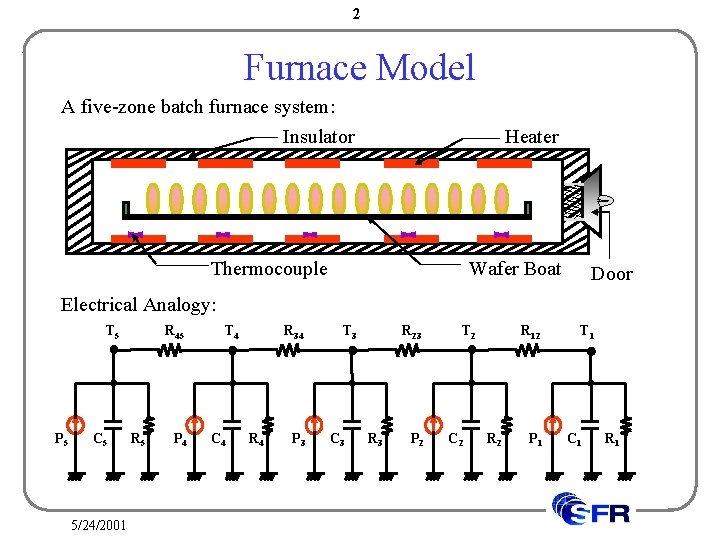
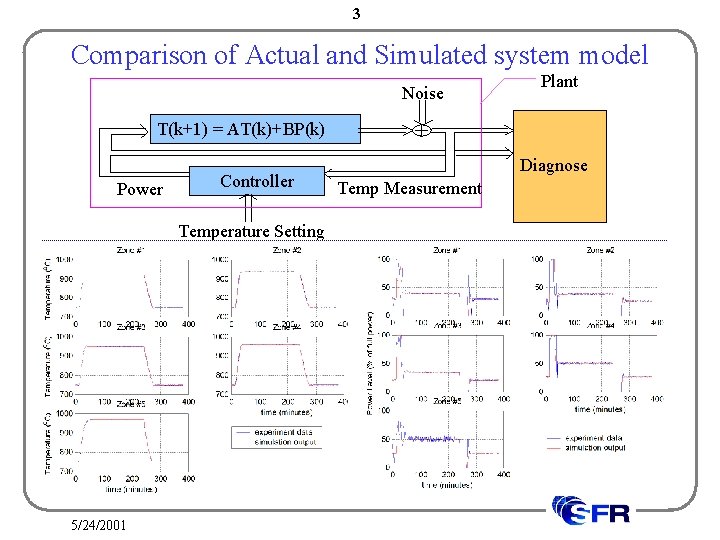
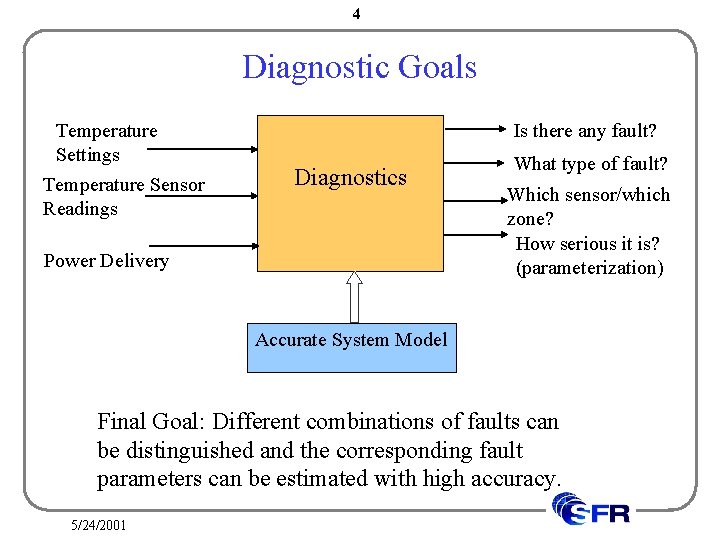
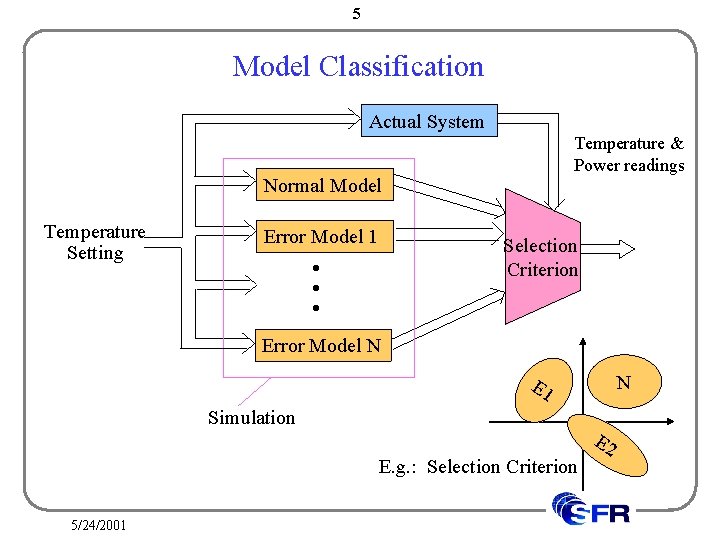
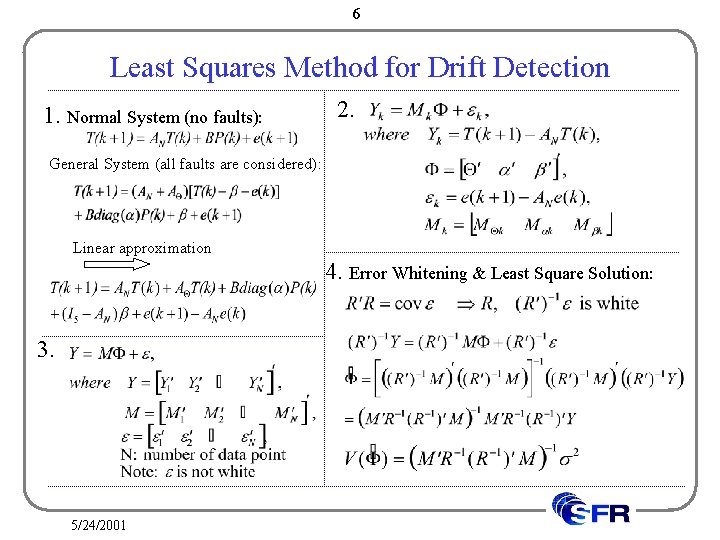
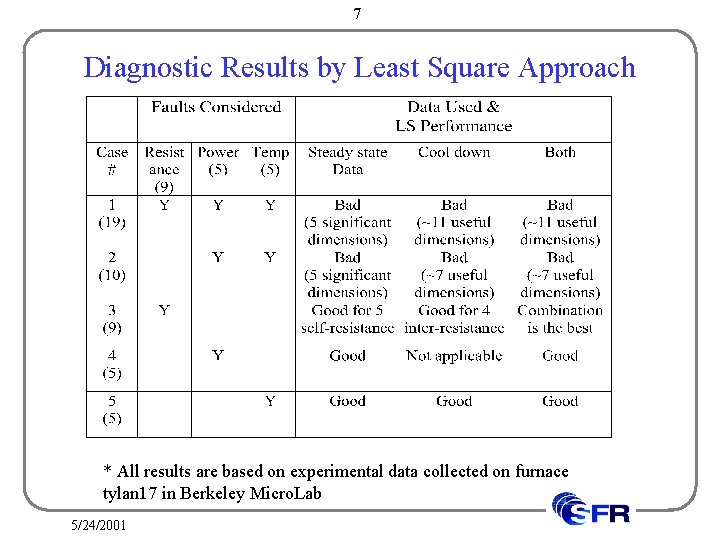
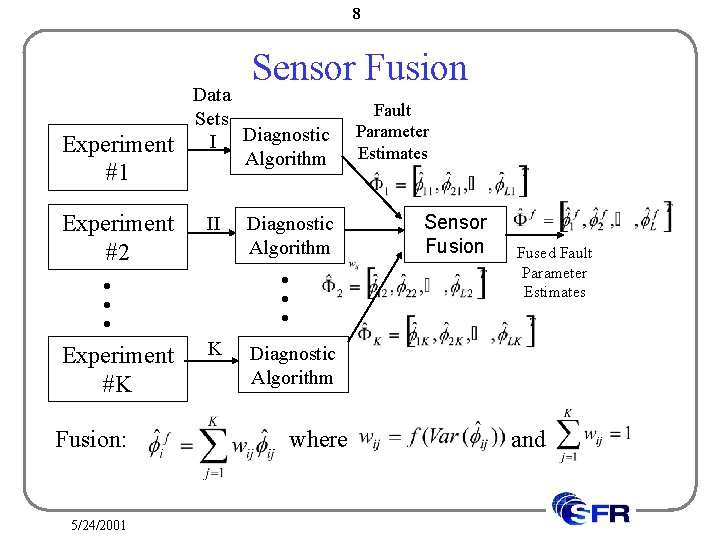
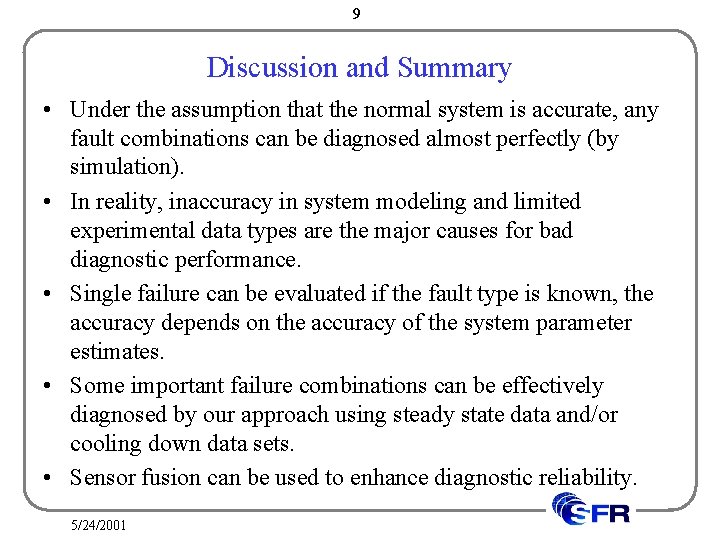
- Slides: 9
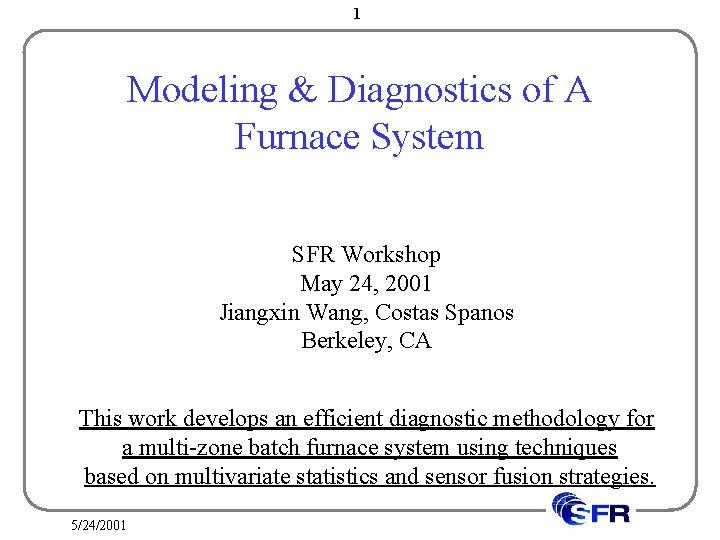
1 Modeling & Diagnostics of A Furnace System SFR Workshop May 24, 2001 Jiangxin Wang, Costas Spanos Berkeley, CA This work develops an efficient diagnostic methodology for a multi-zone batch furnace system using techniques based on multivariate statistics and sensor fusion strategies. 5/24/2001
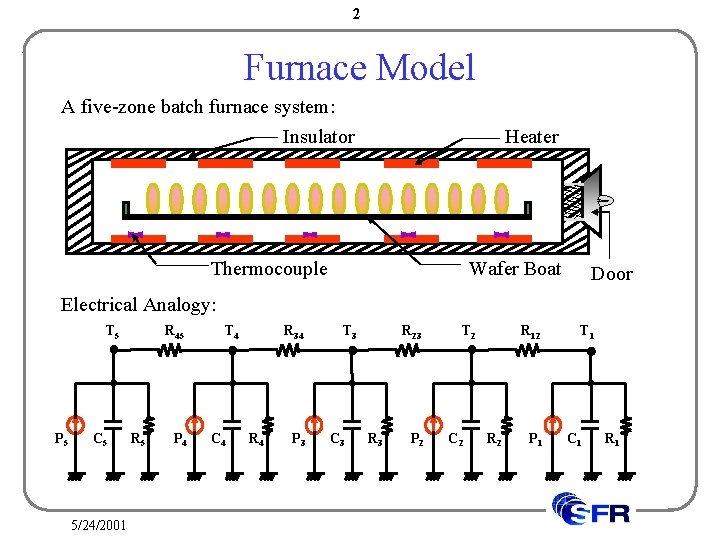
2 Furnace Model A five-zone batch furnace system: Insulator Heater Thermocouple Wafer Boat Door Electrical Analogy: T 5 P 5 C 5 5/24/2001 R 45 R 5 P 4 T 4 C 4 R 34 R 4 P 3 T 3 C 3 R 23 R 3 P 2 T 2 C 2 R 12 R 2 P 1 T 1 C 1 R 1
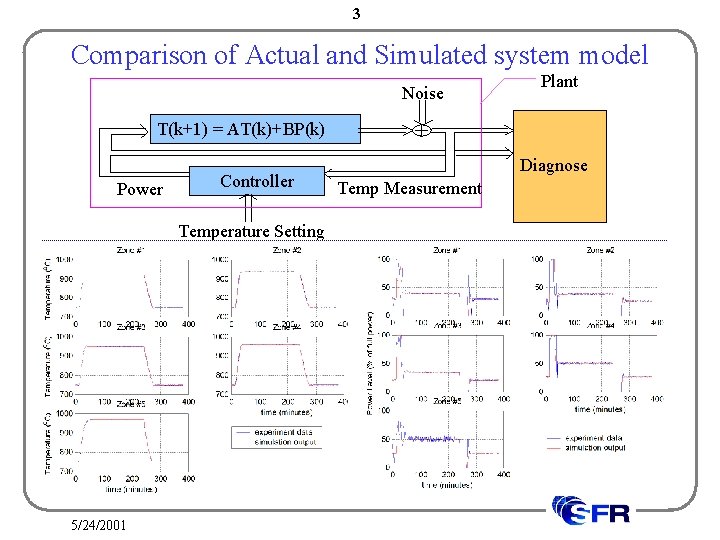
3 Comparison of Actual and Simulated system model Noise T(k+1) = AT(k)+BP(k) Power Controller Temperature Setting 5/24/2001 Plant + Diagnose Temp Measurement
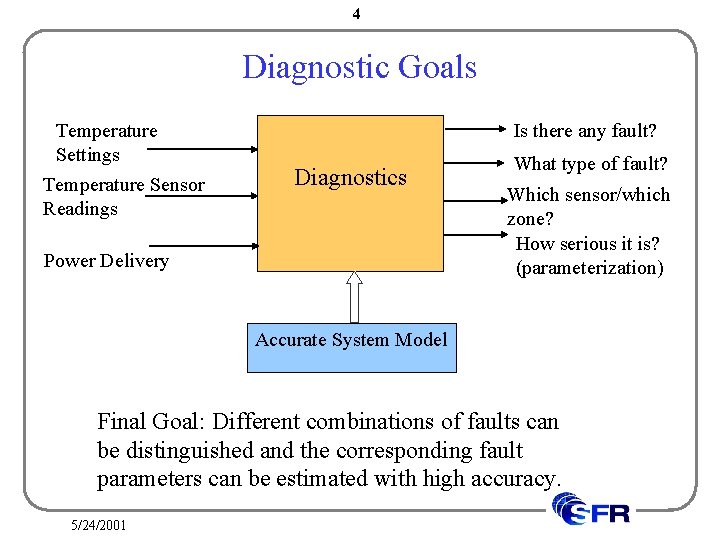
4 Diagnostic Goals Temperature Settings Temperature Sensor Readings Is there any fault? Diagnostics Power Delivery What type of fault? Which sensor/which zone? How serious it is? (parameterization) Accurate System Model Final Goal: Different combinations of faults can be distinguished and the corresponding fault parameters can be estimated with high accuracy. 5/24/2001
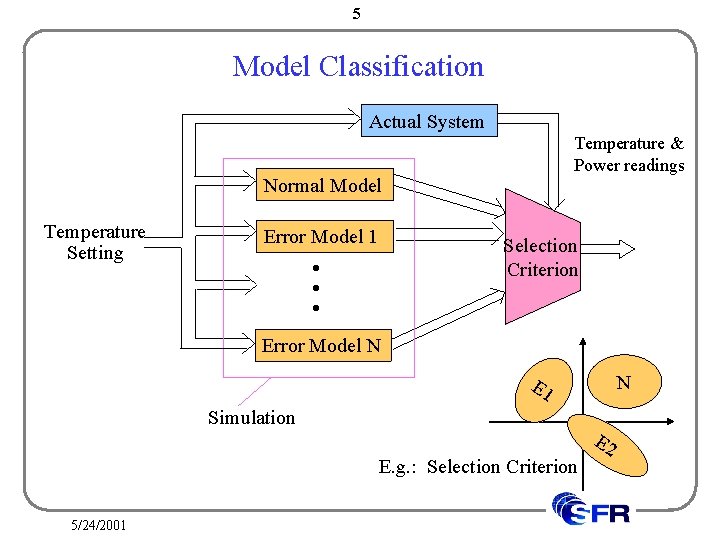
5 Model Classification Actual System Temperature & Power readings Normal Model Temperature Setting Error Model 1 Selection Criterion • • • Error Model N N E 1 Simulation E. g. : Selection Criterion 5/24/2001 E 2
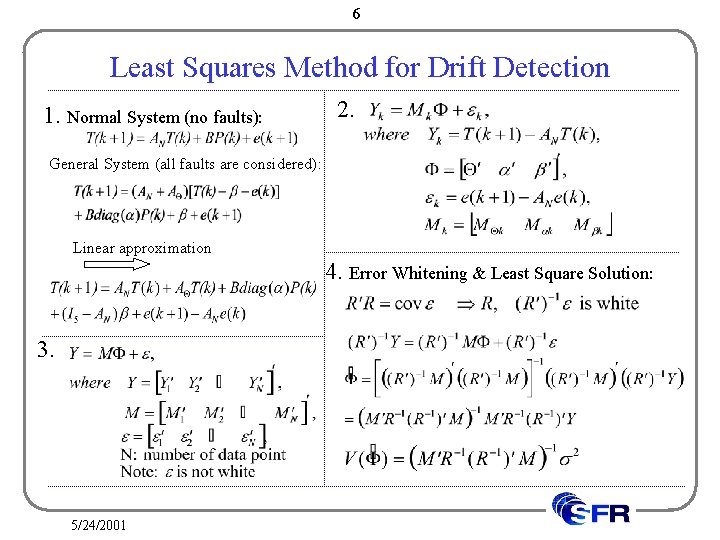
6 Least Squares Method for Drift Detection 1. Normal System (no faults): 2. General System (all faults are considered): Linear approximation 4. Error Whitening & Least Square Solution: 3. 5/24/2001
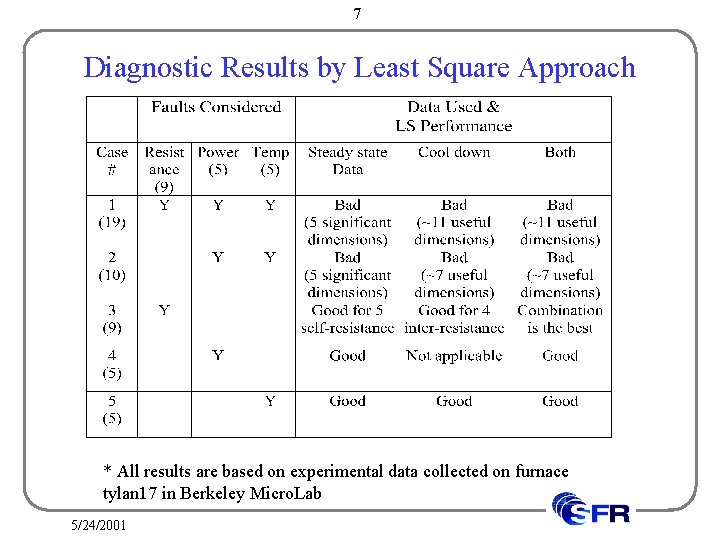
7 Diagnostic Results by Least Square Approach * All results are based on experimental data collected on furnace tylan 17 in Berkeley Micro. Lab 5/24/2001
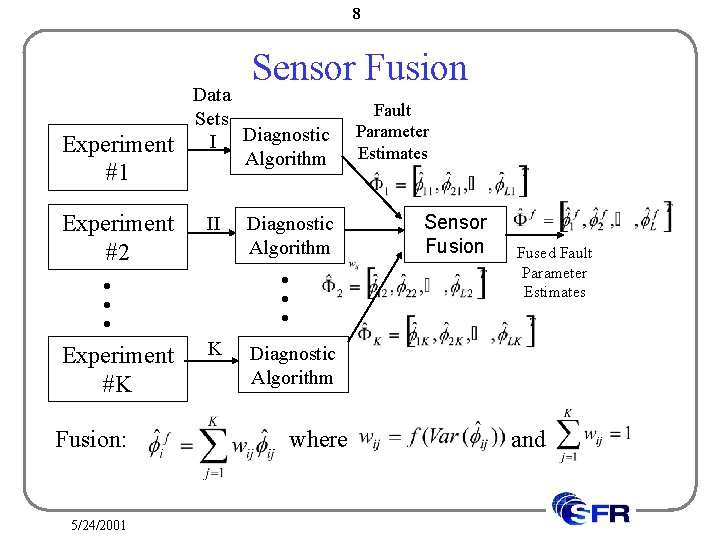
8 Sensor Fusion Experiment #1 Experiment #2 Data Sets I Diagnostic Algorithm II Experiment #K Fusion: 5/24/2001 Diagnostic Algorithm K Fault Parameter Estimates Sensor Fusion Fused Fault Parameter Estimates Diagnostic Algorithm where and
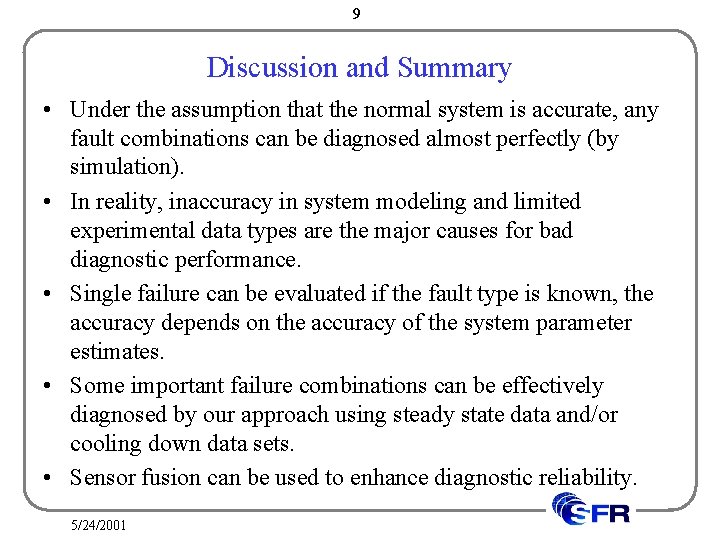
9 Discussion and Summary • Under the assumption that the normal system is accurate, any fault combinations can be diagnosed almost perfectly (by simulation). • In reality, inaccuracy in system modeling and limited experimental data types are the major causes for bad diagnostic performance. • Single failure can be evaluated if the fault type is known, the accuracy depends on the accuracy of the system parameter estimates. • Some important failure combinations can be effectively diagnosed by our approach using steady state data and/or cooling down data sets. • Sensor fusion can be used to enhance diagnostic reliability. 5/24/2001