The Many Faces of Learning Analytics Adaptive Learning
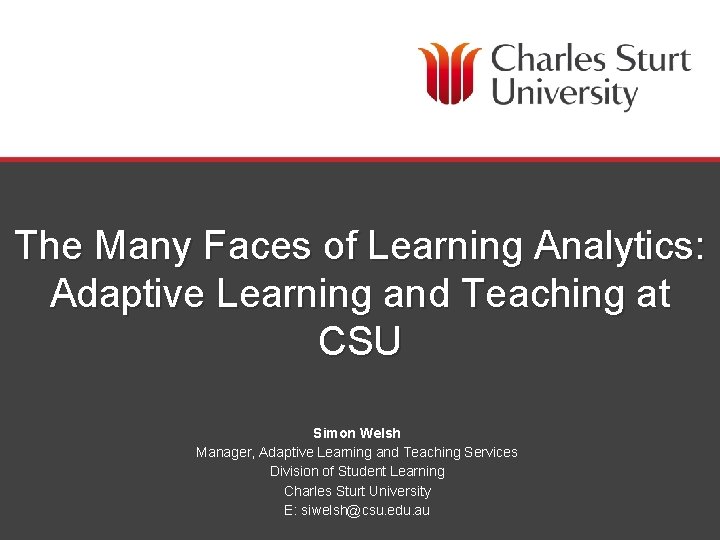
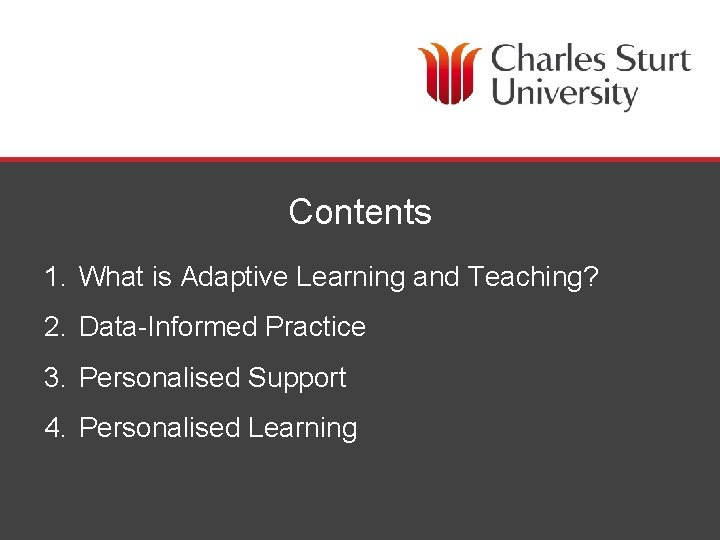
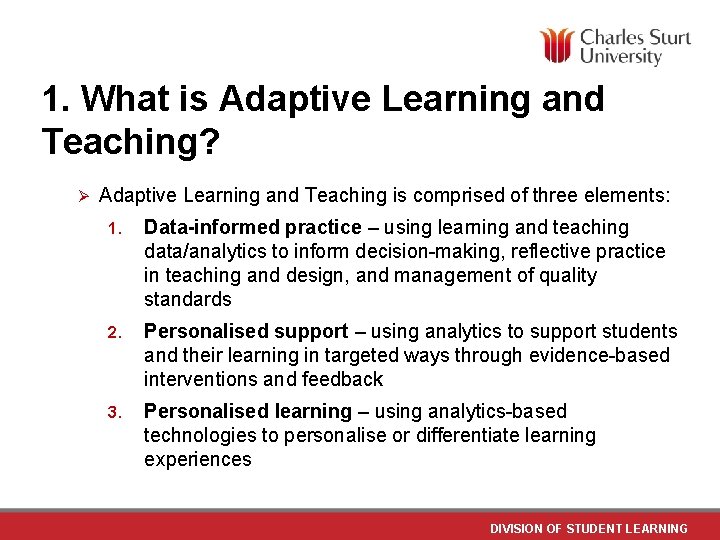
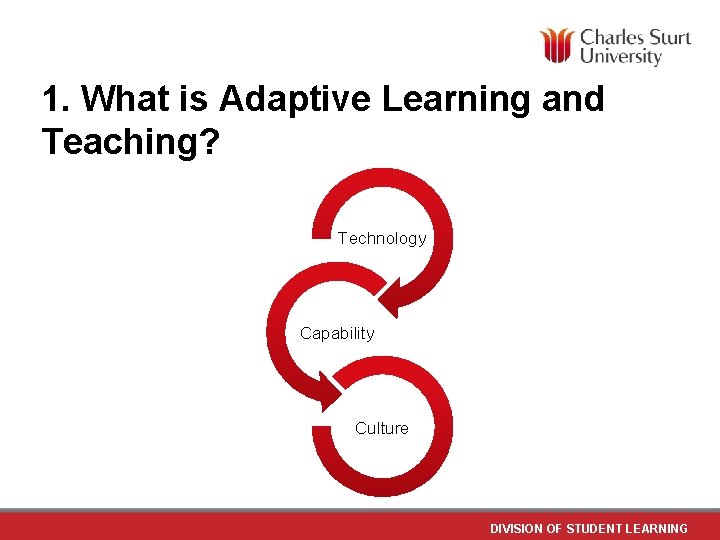
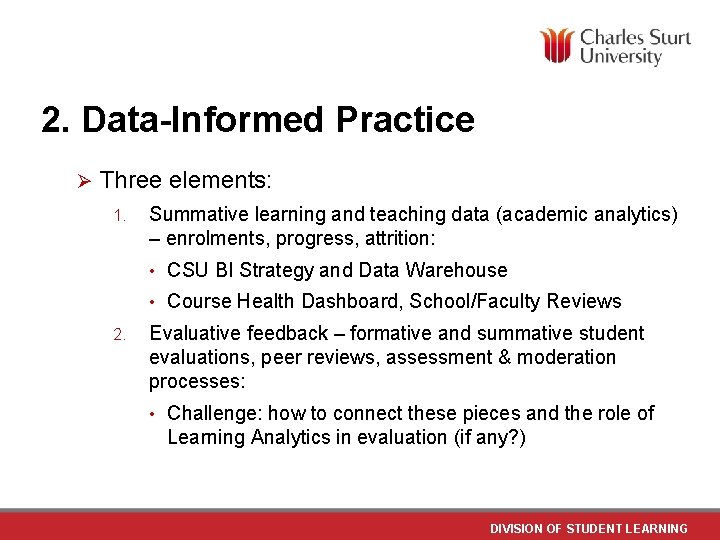
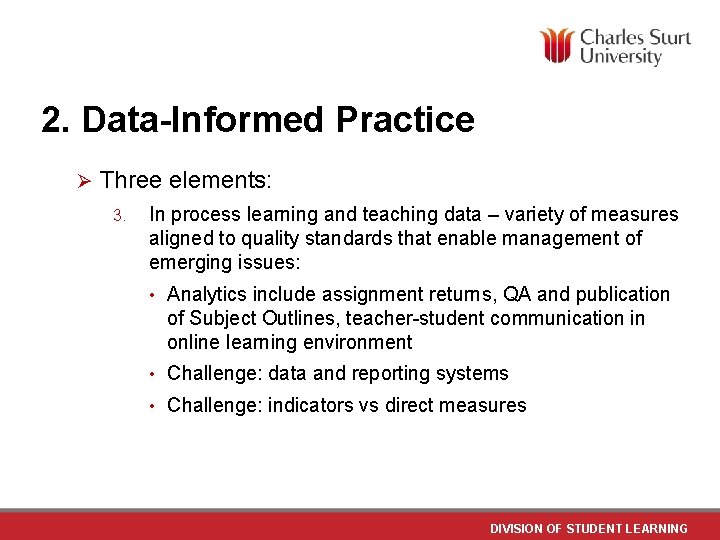
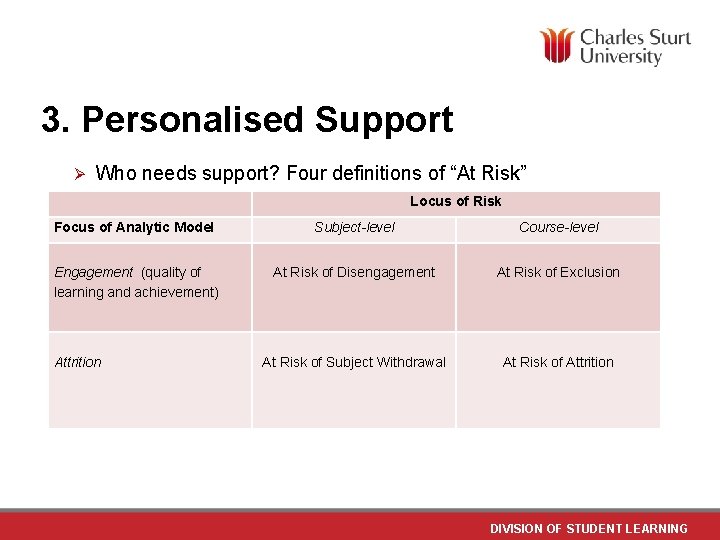
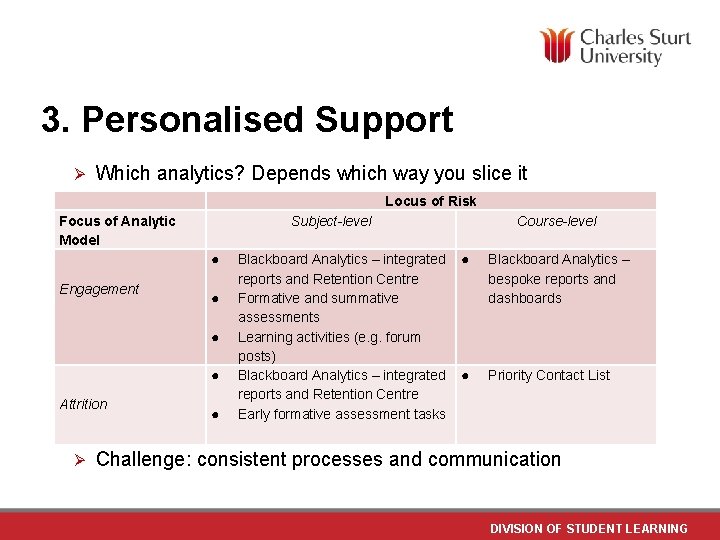
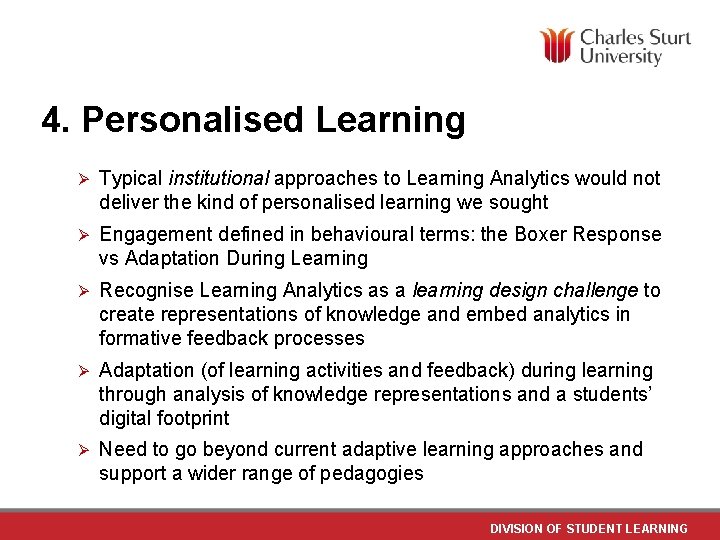
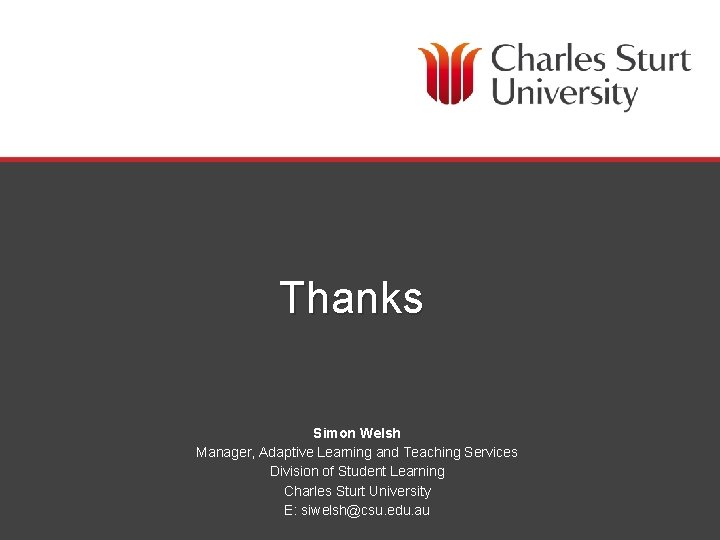
- Slides: 10
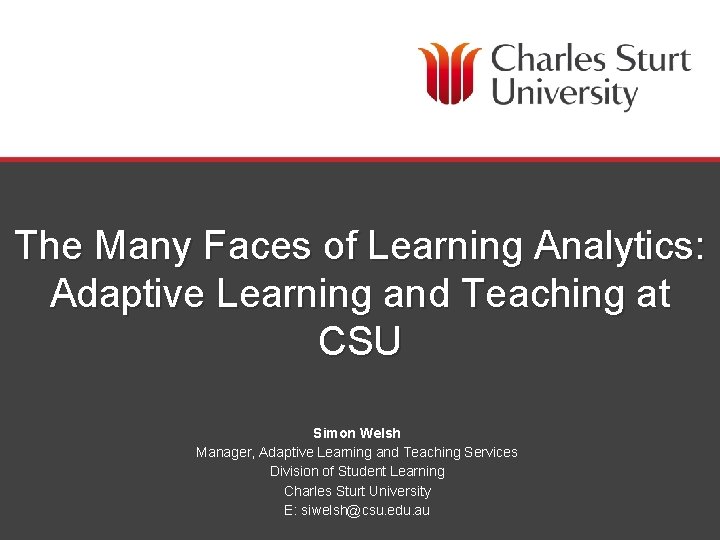
The Many Faces of Learning Analytics: Adaptive Learning and Teaching at CSU Simon Welsh Manager, Adaptive Learning and Teaching Services Division of Student Learning Charles Sturt University E: siwelsh@csu. edu. au DIVISION OF STUDENT LEARNING
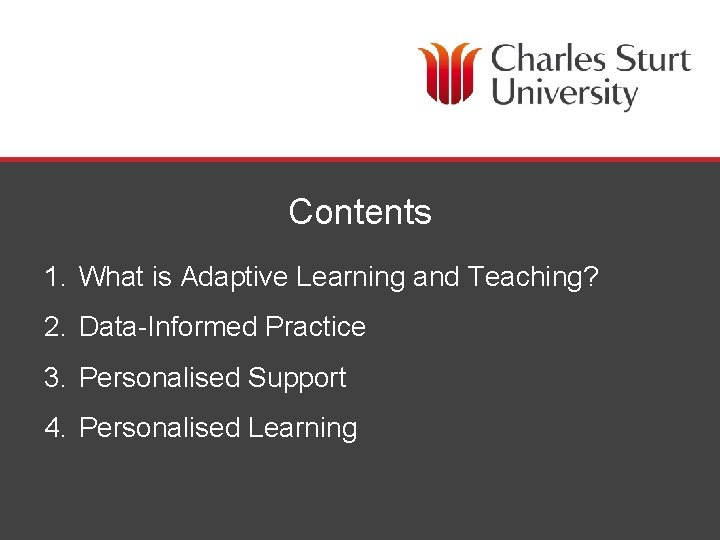
Contents 1. What is Adaptive Learning and Teaching? 2. Data-Informed Practice 3. Personalised Support 4. Personalised Learning DIVISION OF STUDENT LEARNING
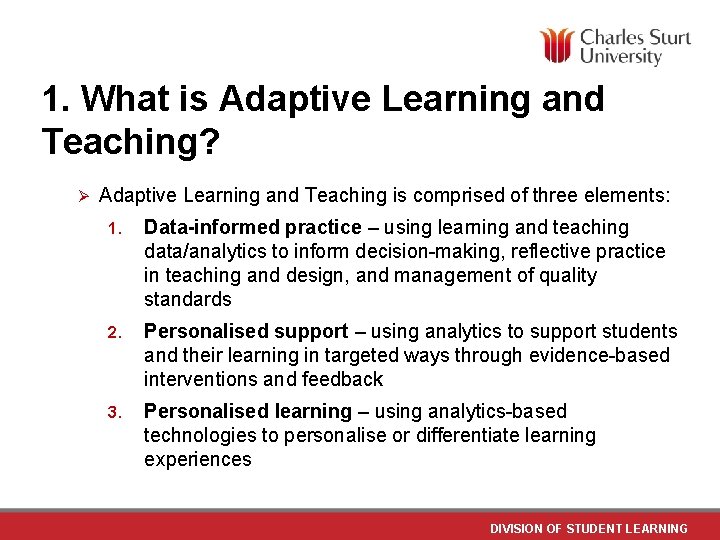
1. What is Adaptive Learning and Teaching? Ø Adaptive Learning and Teaching is comprised of three elements: 1. Data-informed practice – using learning and teaching data/analytics to inform decision-making, reflective practice in teaching and design, and management of quality standards 2. Personalised support – using analytics to support students and their learning in targeted ways through evidence-based interventions and feedback 3. Personalised learning – using analytics-based technologies to personalise or differentiate learning experiences DIVISION OF STUDENT LEARNING
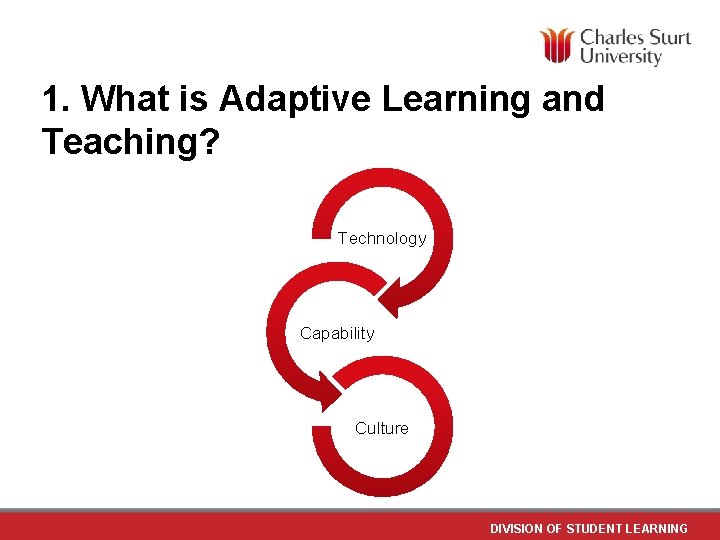
1. What is Adaptive Learning and Teaching? Technology Capability Culture DIVISION OF STUDENT LEARNING
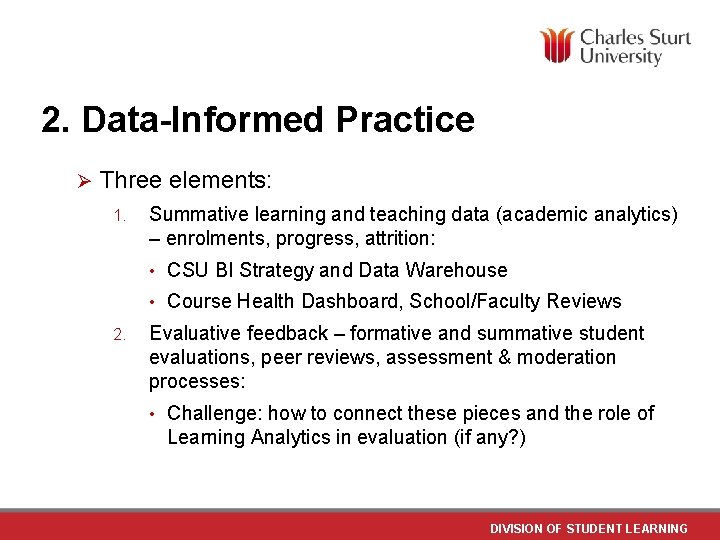
2. Data-Informed Practice Ø Three elements: 1. 2. Summative learning and teaching data (academic analytics) – enrolments, progress, attrition: • CSU BI Strategy and Data Warehouse • Course Health Dashboard, School/Faculty Reviews Evaluative feedback – formative and summative student evaluations, peer reviews, assessment & moderation processes: • Challenge: how to connect these pieces and the role of Learning Analytics in evaluation (if any? ) DIVISION OF STUDENT LEARNING
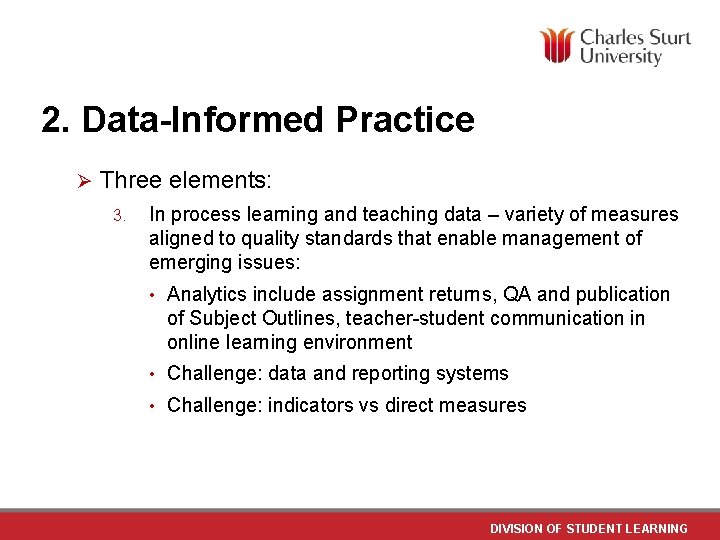
2. Data-Informed Practice Ø Three elements: 3. In process learning and teaching data – variety of measures aligned to quality standards that enable management of emerging issues: • Analytics include assignment returns, QA and publication of Subject Outlines, teacher-student communication in online learning environment • Challenge: data and reporting systems • Challenge: indicators vs direct measures DIVISION OF STUDENT LEARNING
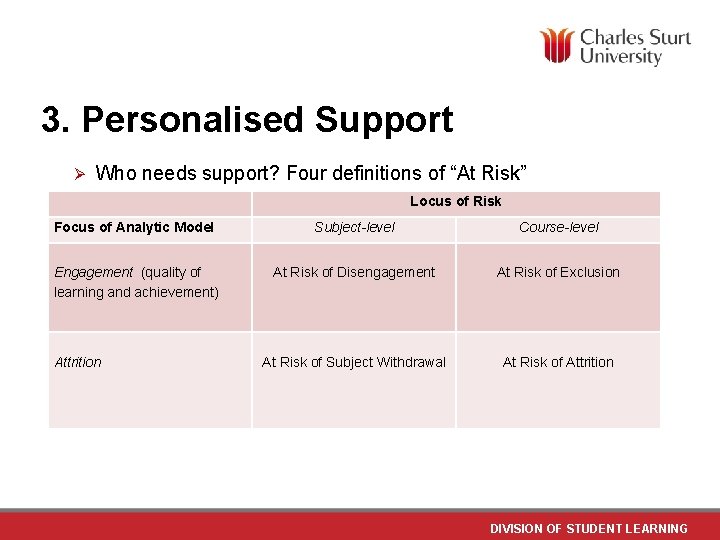
3. Personalised Support Ø Who needs support? Four definitions of “At Risk” Locus of Risk Focus of Analytic Model Subject-level Course-level Engagement (quality of learning and achievement) At Risk of Disengagement At Risk of Exclusion At Risk of Subject Withdrawal At Risk of Attrition DIVISION OF STUDENT LEARNING
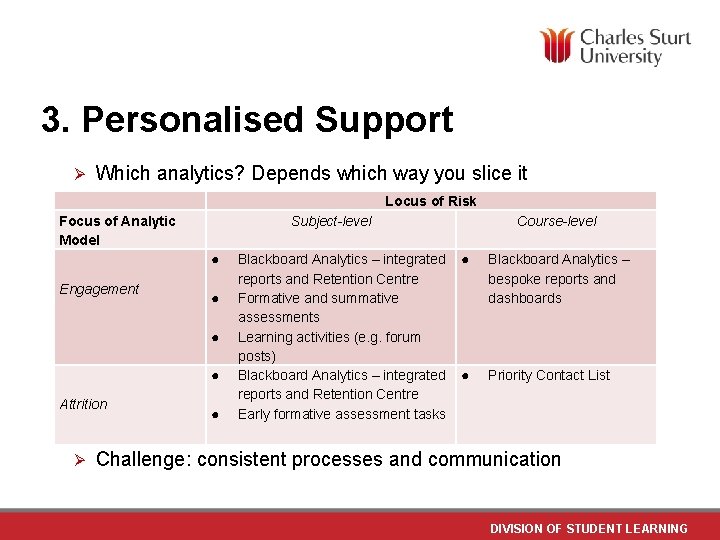
3. Personalised Support Ø Which analytics? Depends which way you slice it Locus of Risk Focus of Analytic Model Subject-level ● Engagement ● ● ● Attrition Ø ● Blackboard Analytics – integrated reports and Retention Centre Formative and summative assessments Learning activities (e. g. forum posts) Blackboard Analytics – integrated reports and Retention Centre Early formative assessment tasks Course-level ● Blackboard Analytics – bespoke reports and dashboards ● Priority Contact List Challenge: consistent processes and communication DIVISION OF STUDENT LEARNING
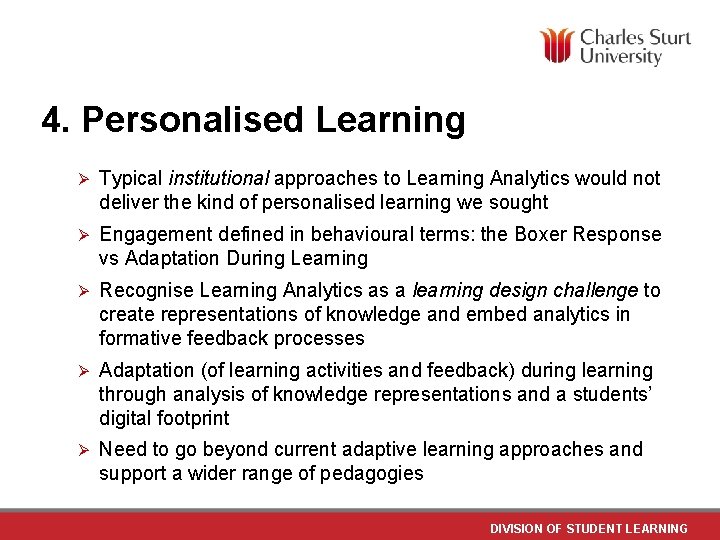
4. Personalised Learning Ø Typical institutional approaches to Learning Analytics would not deliver the kind of personalised learning we sought Ø Engagement defined in behavioural terms: the Boxer Response vs Adaptation During Learning Ø Recognise Learning Analytics as a learning design challenge to create representations of knowledge and embed analytics in formative feedback processes Ø Adaptation (of learning activities and feedback) during learning through analysis of knowledge representations and a students’ digital footprint Ø Need to go beyond current adaptive learning approaches and support a wider range of pedagogies DIVISION OF STUDENT LEARNING
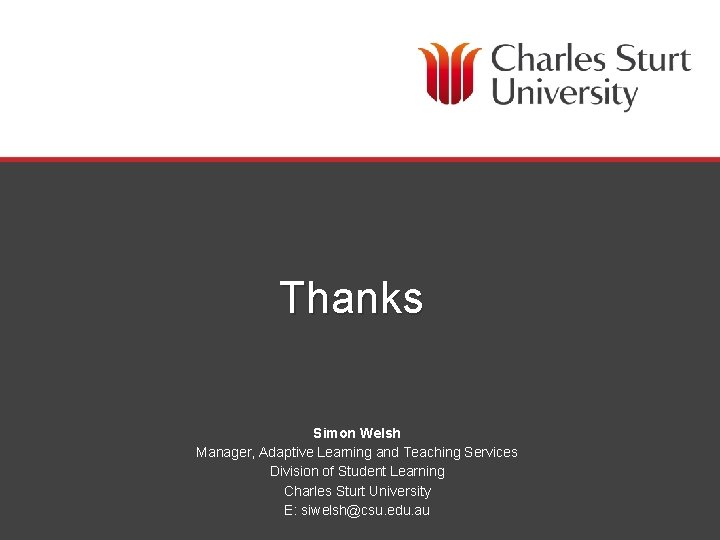
Thanks Simon Welsh Manager, Adaptive Learning and Teaching Services Division of Student Learning Charles Sturt University E: siwelsh@csu. edu. au DIVISION OF STUDENT LEARNING
Adaptive suite vs host analytics
6 faces, 12 edges and 8 vertices
Teramond
How many edges does a rectangular pyramid have
Many faces mask
Many faces of autism
Cylinder has how many faces edges and vertices
Many faces do you see
How many faces do you see in this picture
Sinthia cousineau
Once upon a time okara