SU3 T Matsumoto M Kitazawa Y Kohno ar
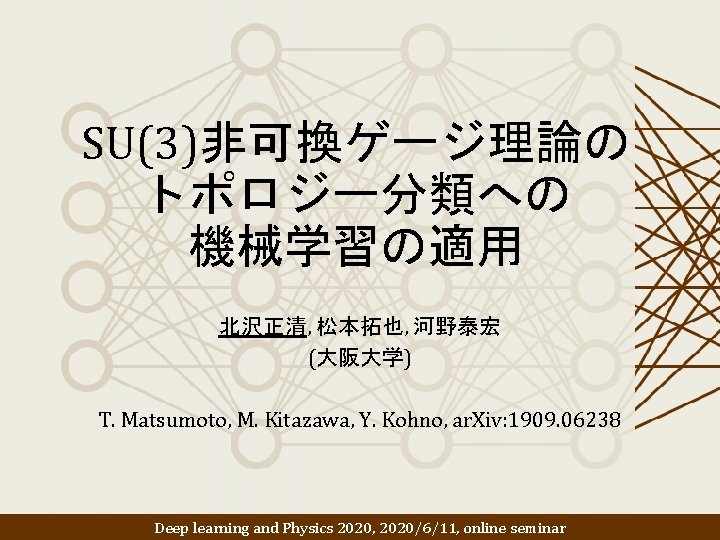
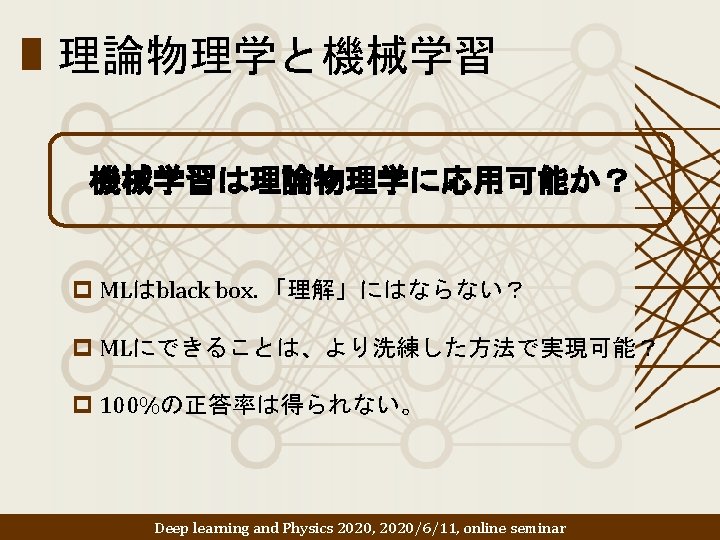
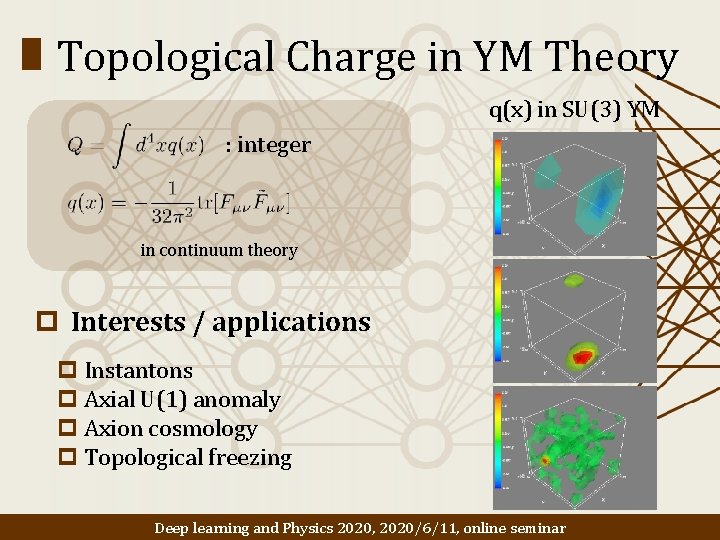
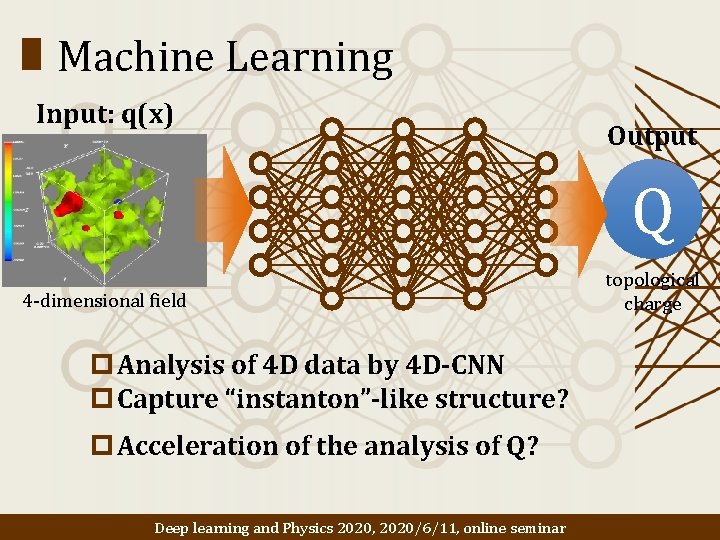
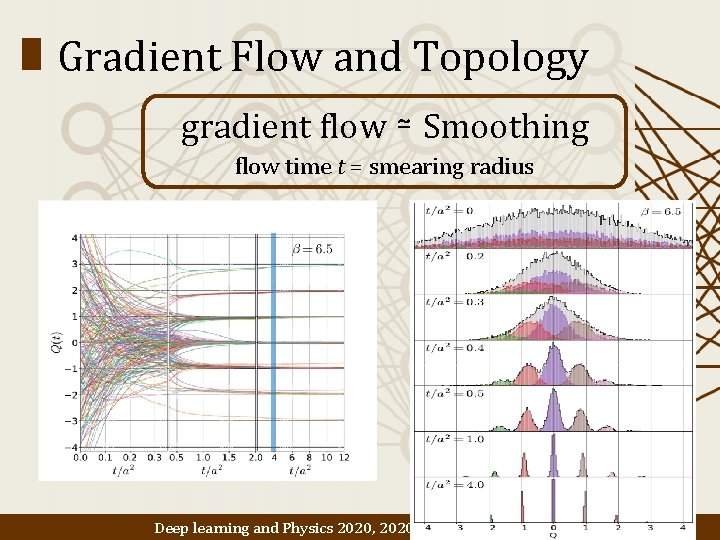
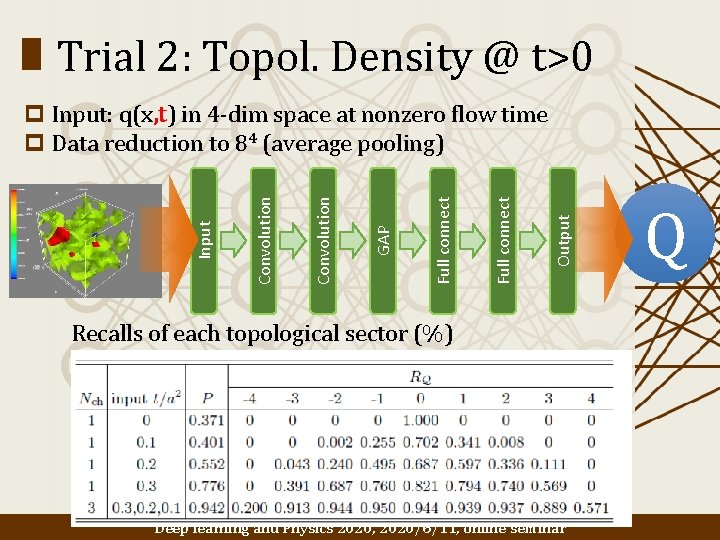
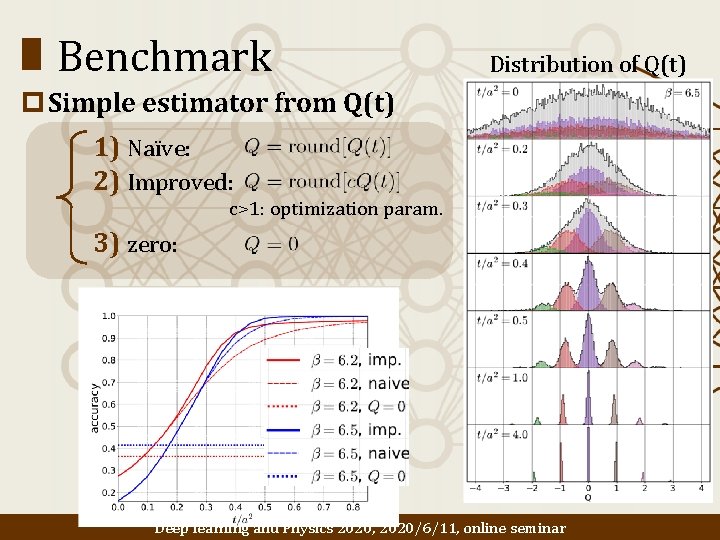
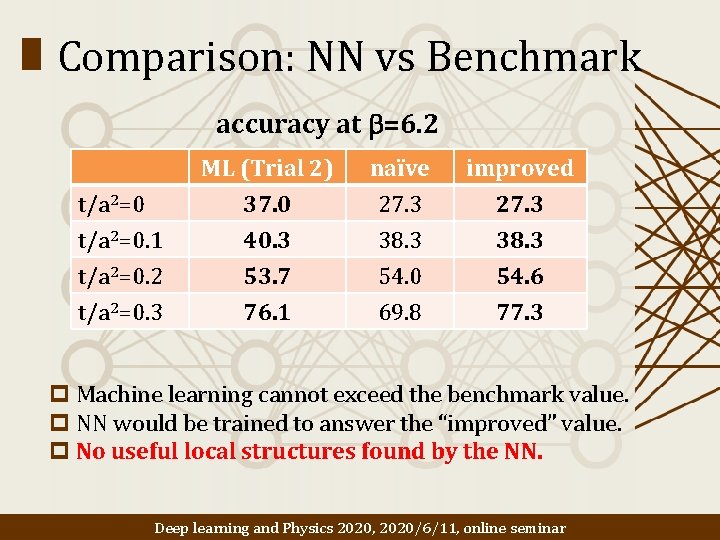
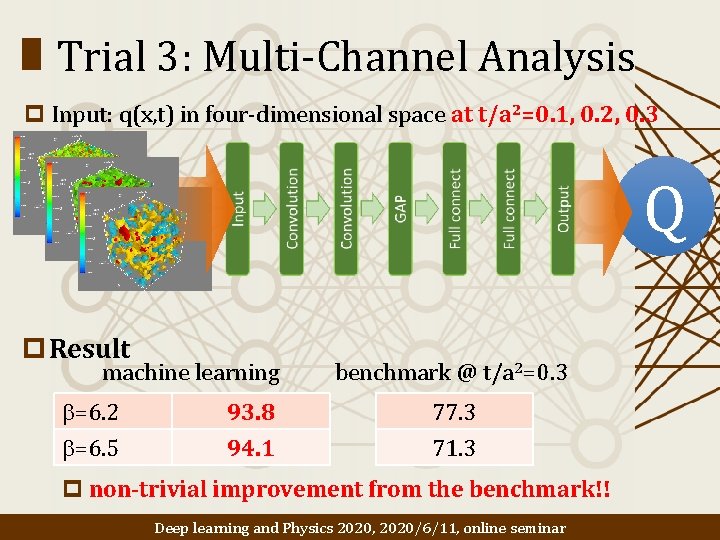
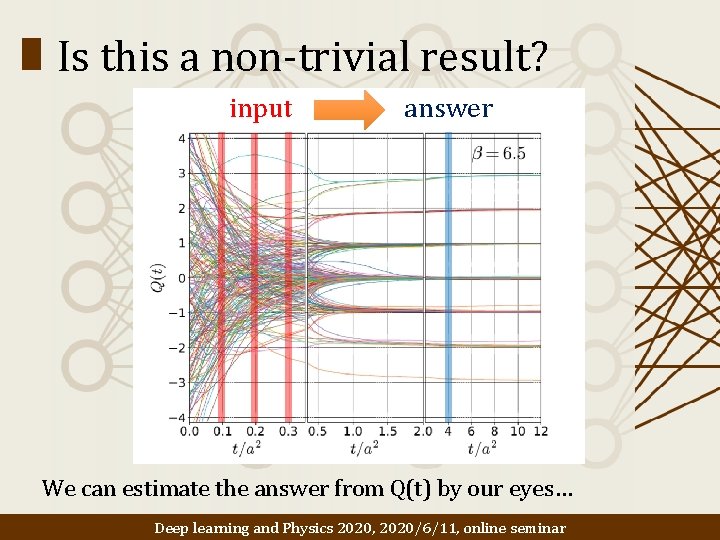
![Trial 4: Feed Q(t) [0 -dim] Q(0. 3) p Result b=6. 2 b=6. 5 Trial 4: Feed Q(t) [0 -dim] Q(0. 3) p Result b=6. 2 b=6. 5](https://slidetodoc.com/presentation_image_h2/2ea070bcea682d789b2cfaa5e83d98d7/image-11.jpg)
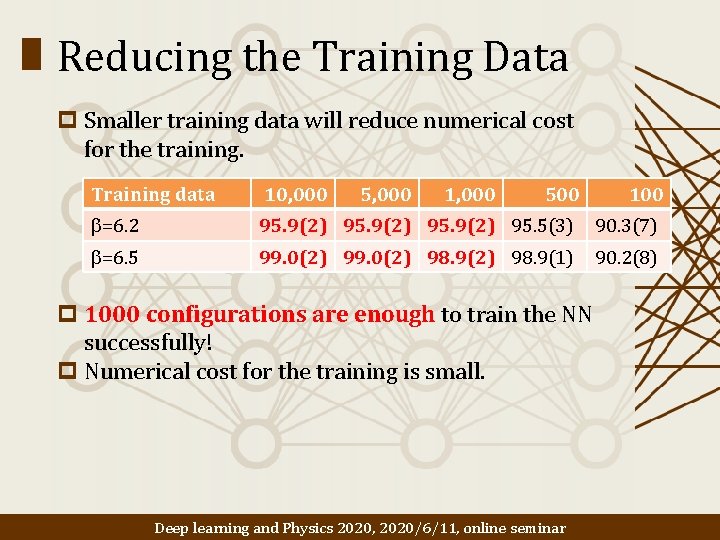
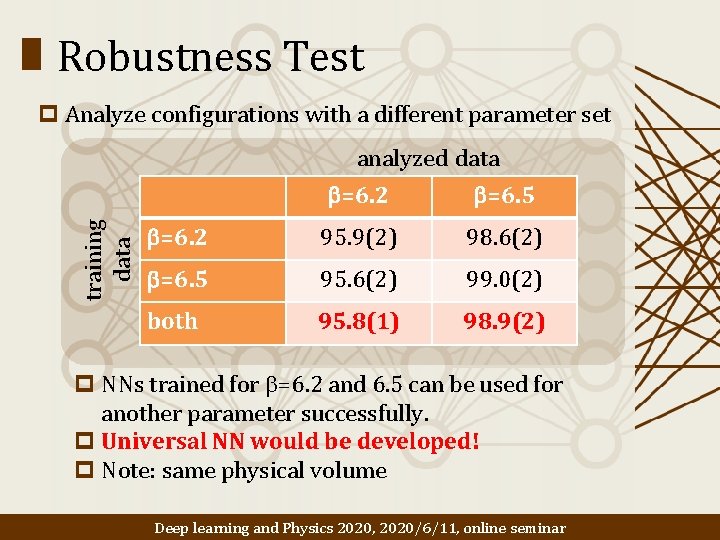
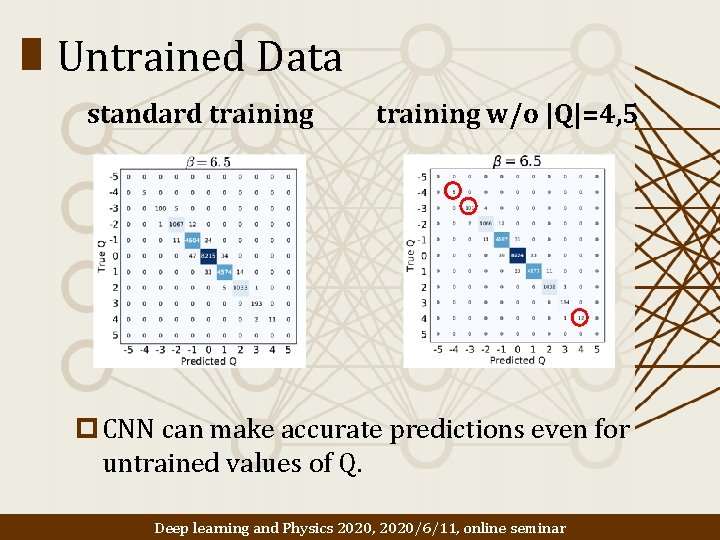
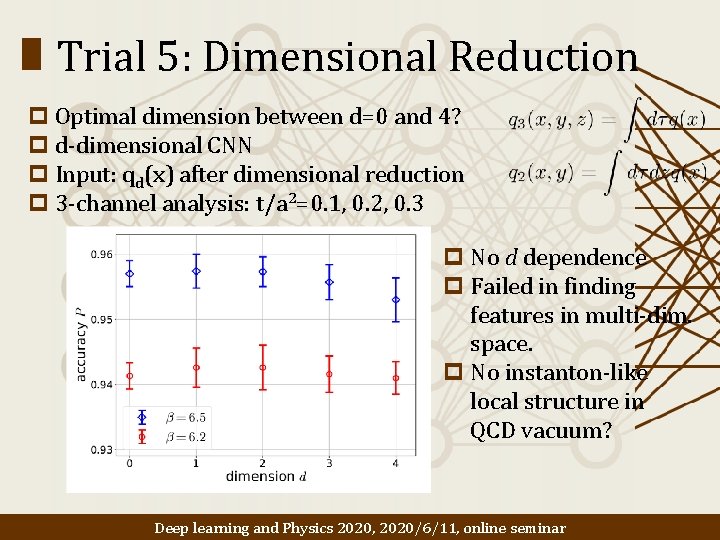
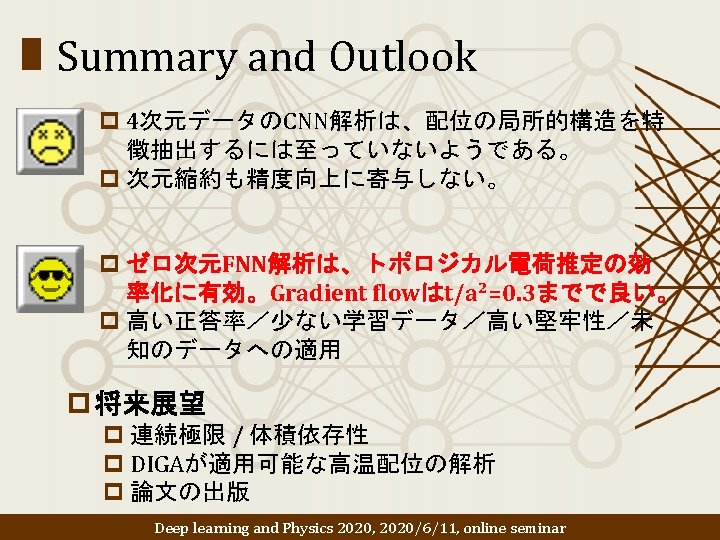
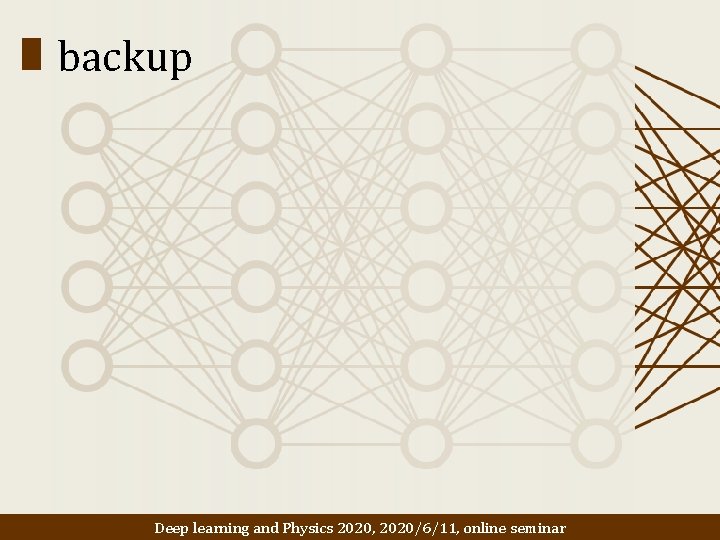
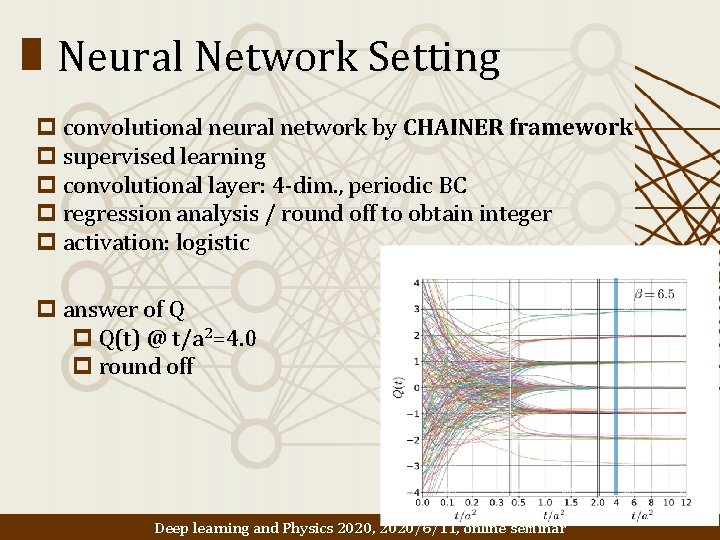
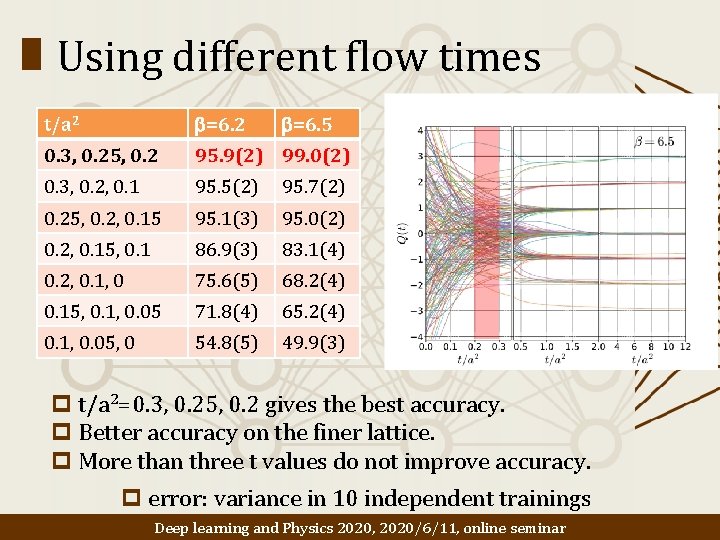
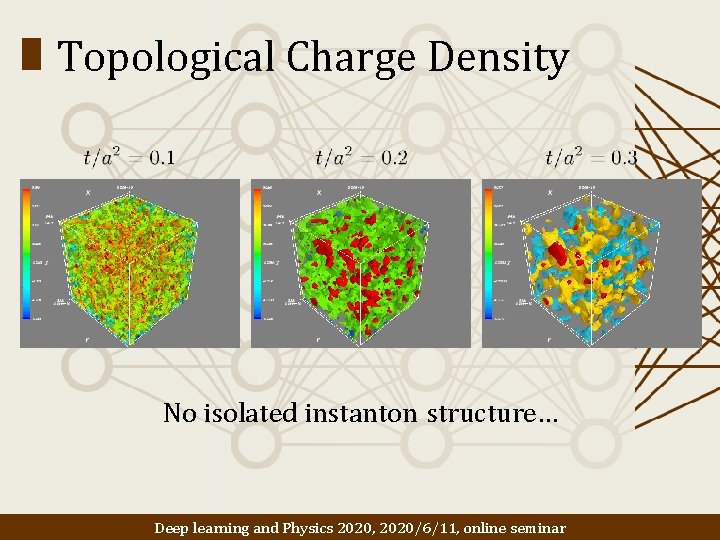
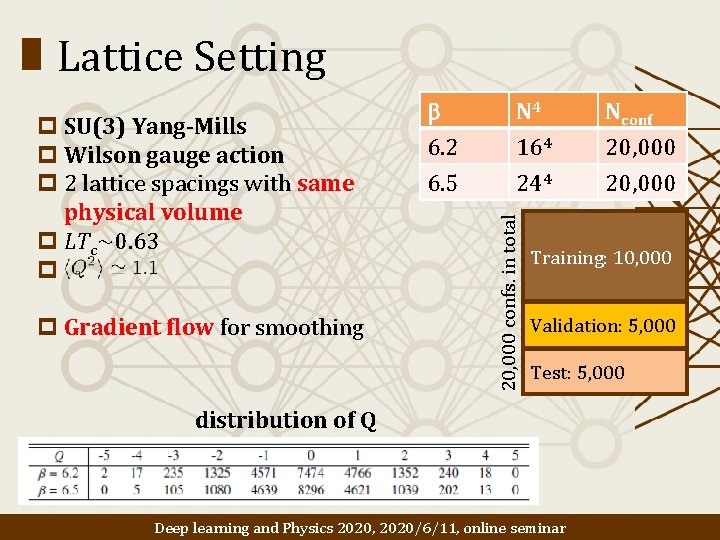
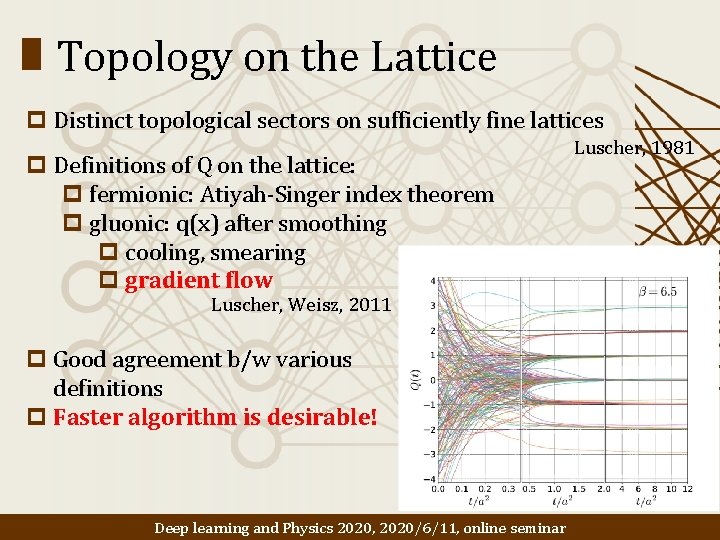
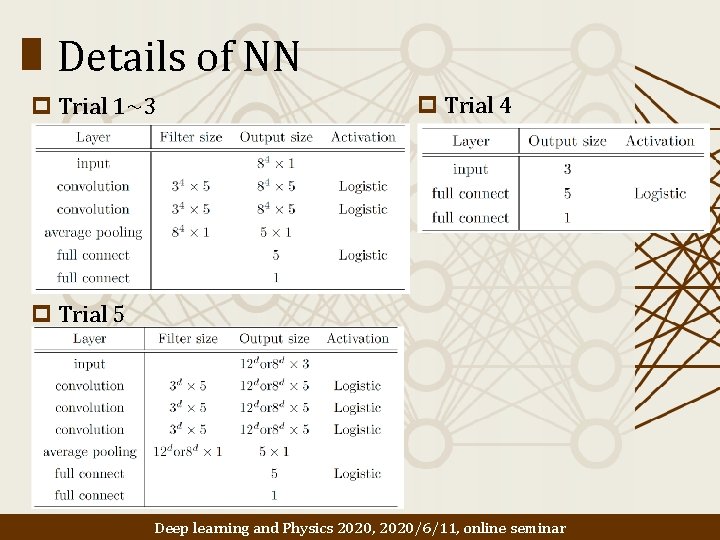
- Slides: 23
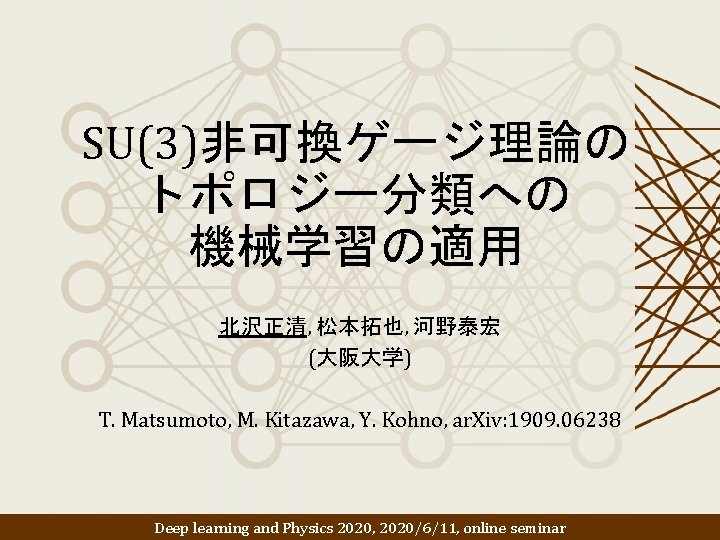
SU(3)非可換ゲージ理論の トポロジー分類への 機械学習の適用 北沢正清, 松本拓也, 河野泰宏 (大阪大学) T. Matsumoto, M. Kitazawa, Y. Kohno, ar. Xiv: 1909. 06238 Deep learning and Physics 2020, 2020/6/11, online seminar
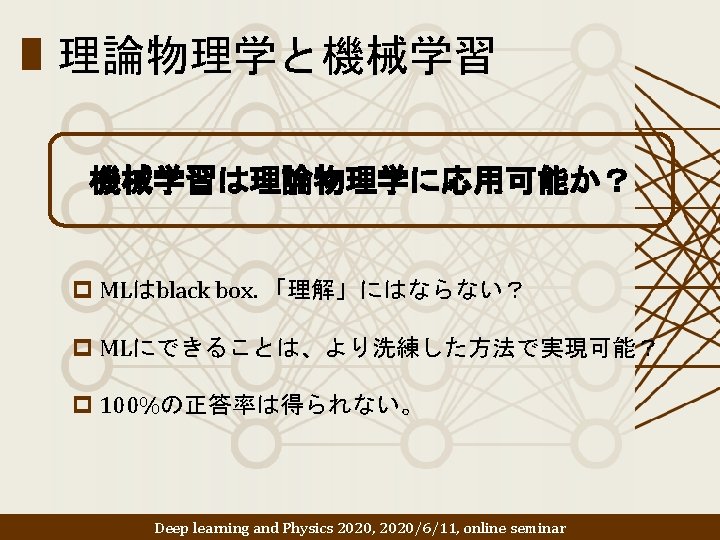
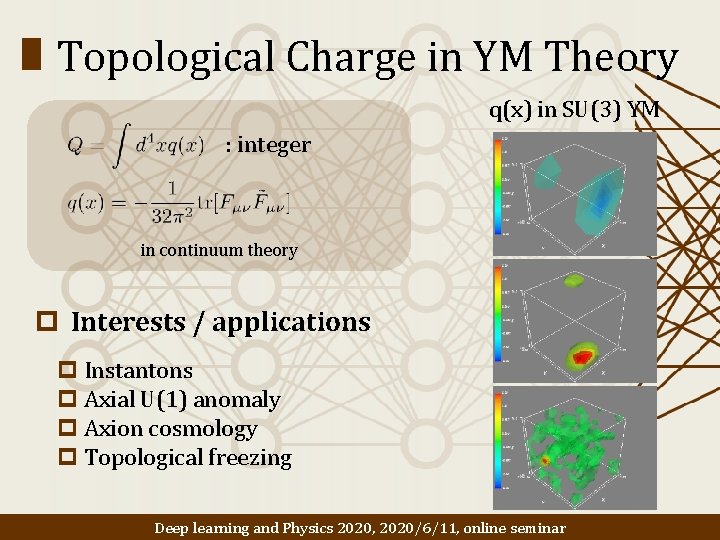
Topological Charge in YM Theory q(x) in SU(3) YM : integer in continuum theory p Interests / applications p Instantons p Axial U(1) anomaly p Axion cosmology p Topological freezing Deep learning and Physics 2020, 2020/6/11, online seminar
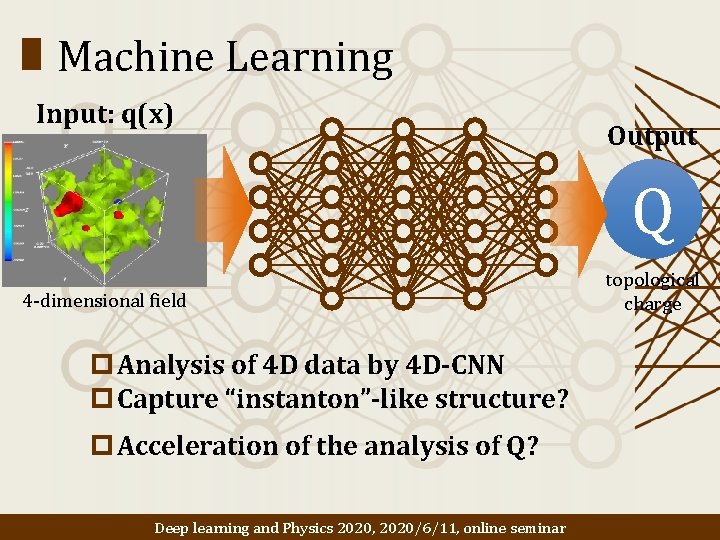
Machine Learning Input: q(x) Output Q 4 -dimensional field p Analysis of 4 D data by 4 D-CNN p Capture “instanton”-like structure? p Acceleration of the analysis of Q? Deep learning and Physics 2020, 2020/6/11, online seminar topological charge
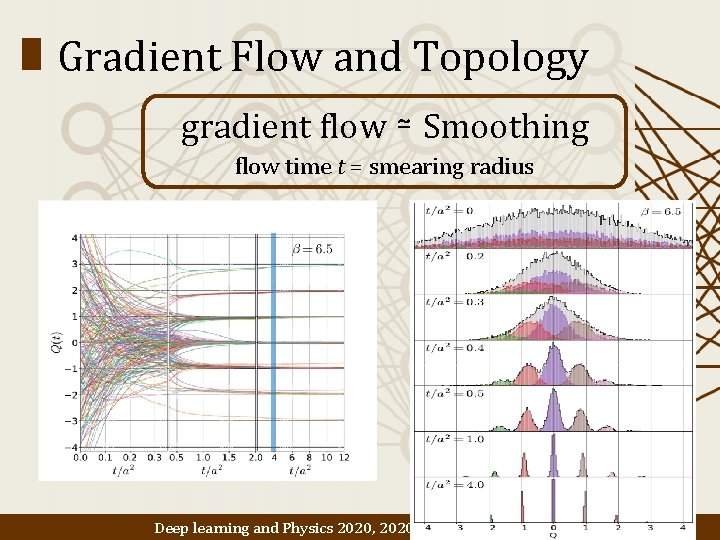
Gradient Flow and Topology gradient flow ≃ Smoothing flow time t = smearing radius Deep learning and Physics 2020, 2020/6/11, online seminar
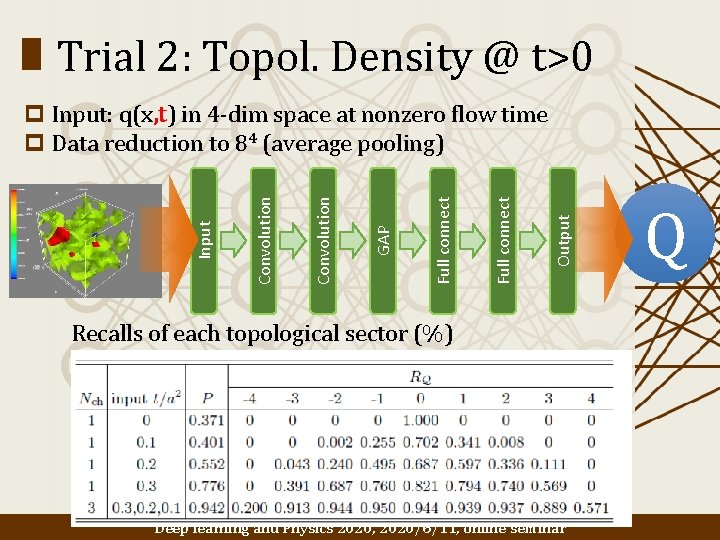
Trial 2: Topol. Density @ t>0 Output Full connect GAP Convolution Input p Input: q(x, t) in 4 -dim space at nonzero flow time p Data reduction to 84 (average pooling) Recalls of each topological sector (%) Deep learning and Physics 2020, 2020/6/11, online seminar Q
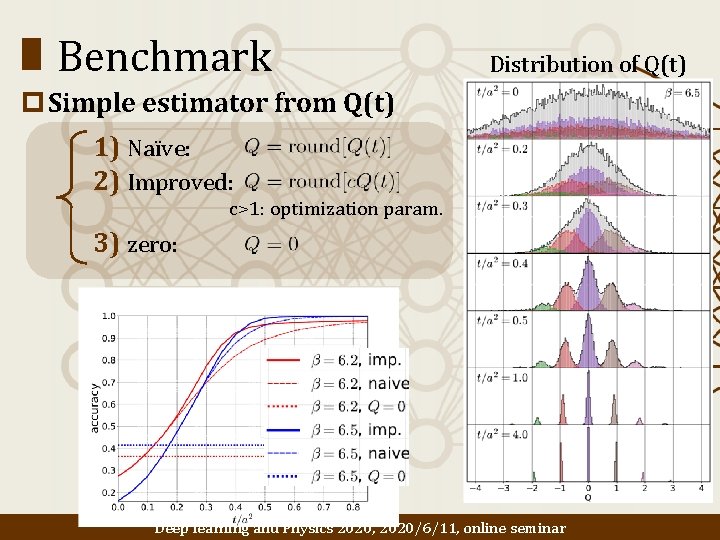
Benchmark Distribution of Q(t) p Simple estimator from Q(t) 1) Naïve: 2) Improved: c>1: optimization param. 3) zero: Deep learning and Physics 2020, 2020/6/11, online seminar
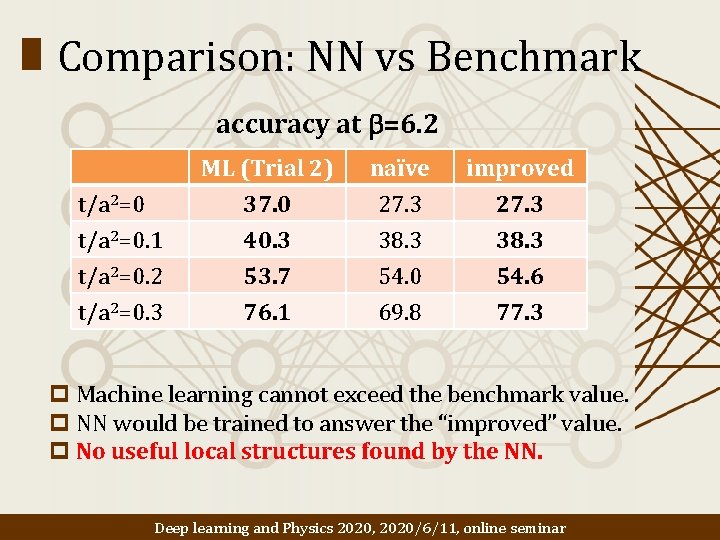
Comparison: NN vs Benchmark accuracy at b=6. 2 t/a 2=0. 1 t/a 2=0. 2 ML (Trial 2) 37. 0 40. 3 53. 7 naïve 27. 3 38. 3 54. 0 improved 27. 3 38. 3 54. 6 t/a 2=0. 3 76. 1 69. 8 77. 3 p Machine learning cannot exceed the benchmark value. p NN would be trained to answer the “improved” value. p No useful local structures found by the NN. Deep learning and Physics 2020, 2020/6/11, online seminar
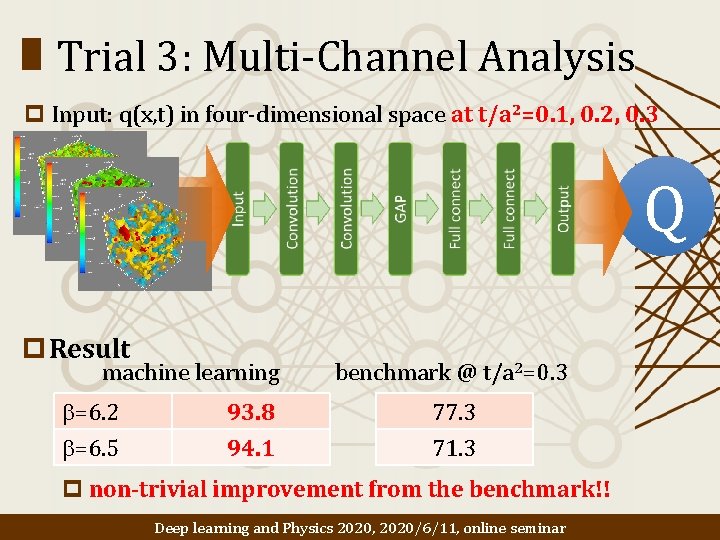
Trial 3: Multi-Channel Analysis p Input: q(x, t) in four-dimensional space at t/a 2=0. 1, 0. 2, 0. 3 Q p Result machine learning b=6. 2 b=6. 5 93. 8 94. 1 benchmark @ t/a 2=0. 3 77. 3 71. 3 p non-trivial improvement from the benchmark!! Deep learning and Physics 2020, 2020/6/11, online seminar
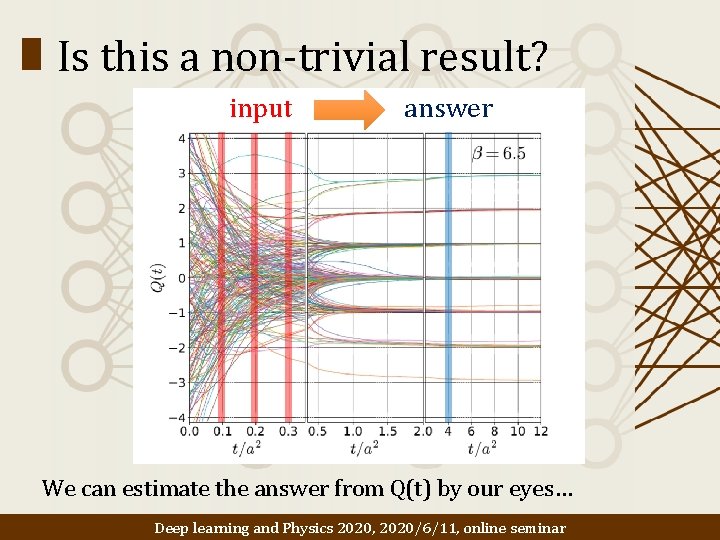
Is this a non-trivial result? input answer We can estimate the answer from Q(t) by our eyes… Deep learning and Physics 2020, 2020/6/11, online seminar
![Trial 4 Feed Qt 0 dim Q0 3 p Result b6 2 b6 5 Trial 4: Feed Q(t) [0 -dim] Q(0. 3) p Result b=6. 2 b=6. 5](https://slidetodoc.com/presentation_image_h2/2ea070bcea682d789b2cfaa5e83d98d7/image-11.jpg)
Trial 4: Feed Q(t) [0 -dim] Q(0. 3) p Result b=6. 2 b=6. 5 Q(t) 94. 1 95. 7 tuning input t 95. 9 99. 0 output Q input Output Q(0. 2) Full connect (5) Q(0. 1) Input (3) p Input: Q(t) at t/a 2=0. 1, 0. 2, 0. 3 Trial 3 (4 dim) benchmark 93. 8 94. 1 p Good accuracy is obtained only from Q(t) Deep learning and Physics 2020, 2020/6/11, online seminar 77. 3 71. 3
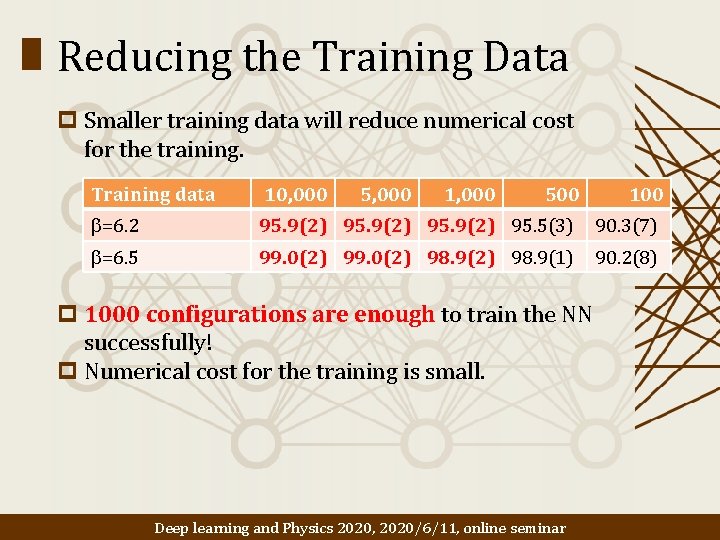
Reducing the Training Data p Smaller training data will reduce numerical cost for the training. Training data 10, 000 5, 000 1, 000 500 100 b=6. 2 95. 9(2) 95. 5(3) 90. 3(7) b=6. 5 99. 0(2) 98. 9(2) 98. 9(1) 90. 2(8) p 1000 configurations are enough to train the NN successfully! p Numerical cost for the training is small. Deep learning and Physics 2020, 2020/6/11, online seminar
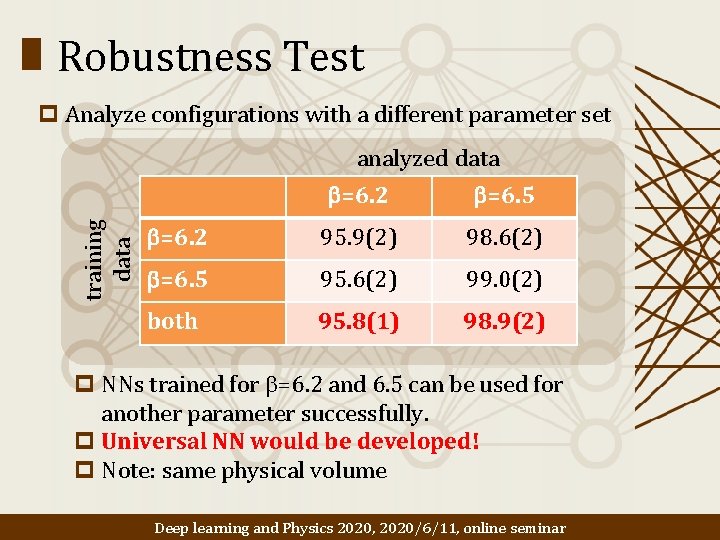
Robustness Test p Analyze configurations with a different parameter set training data analyzed data b=6. 2 b=6. 5 b=6. 2 95. 9(2) 98. 6(2) b=6. 5 95. 6(2) 99. 0(2) both 95. 8(1) 98. 9(2) p NNs trained for b=6. 2 and 6. 5 can be used for another parameter successfully. p Universal NN would be developed! p Note: same physical volume Deep learning and Physics 2020, 2020/6/11, online seminar
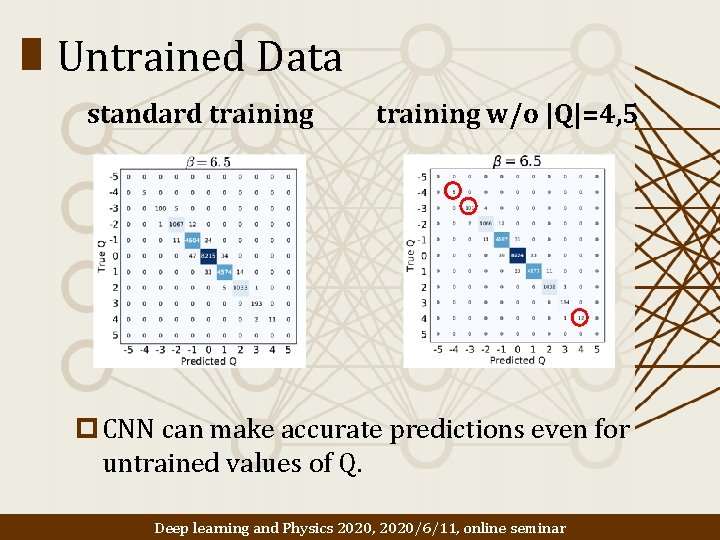
Untrained Data standard training w/o |Q|=4, 5 p CNN can make accurate predictions even for untrained values of Q. Deep learning and Physics 2020, 2020/6/11, online seminar
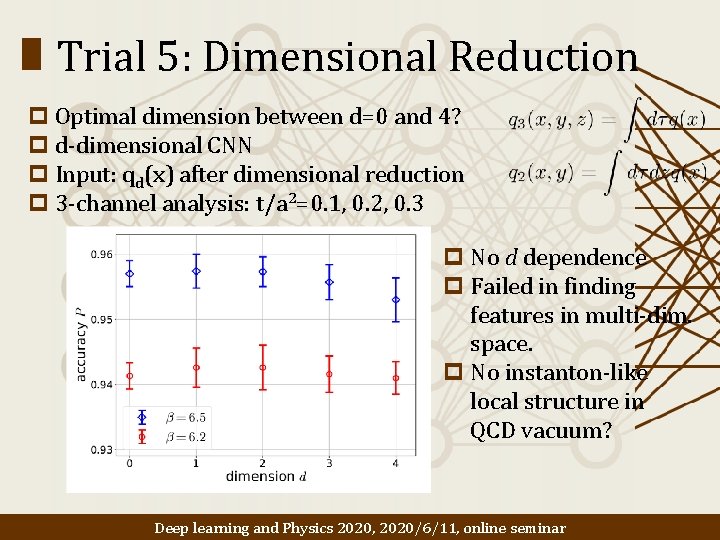
Trial 5: Dimensional Reduction p Optimal dimension between d=0 and 4? p d-dimensional CNN p Input: qd(x) after dimensional reduction p 3 -channel analysis: t/a 2=0. 1, 0. 2, 0. 3 p No d dependence p Failed in finding features in multi-dim. space. p No instanton-like local structure in QCD vacuum? Deep learning and Physics 2020, 2020/6/11, online seminar
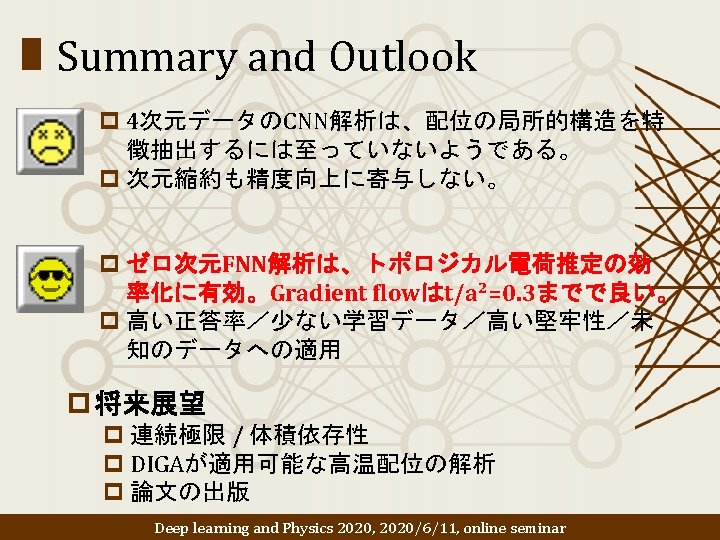
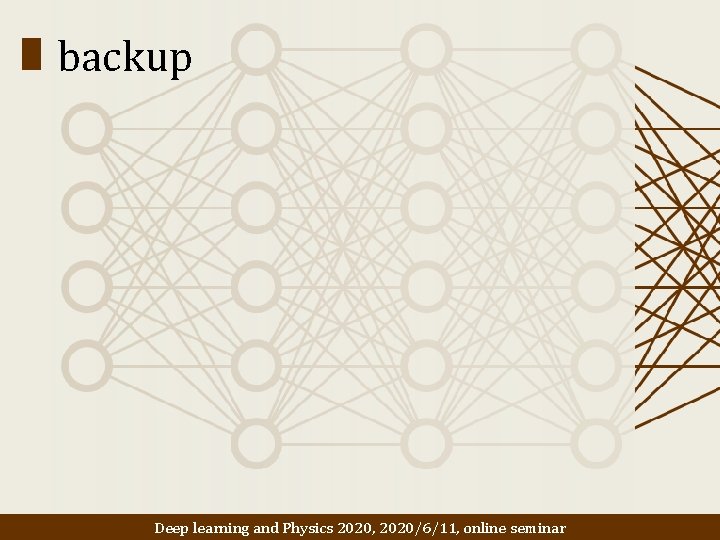
backup Deep learning and Physics 2020, 2020/6/11, online seminar
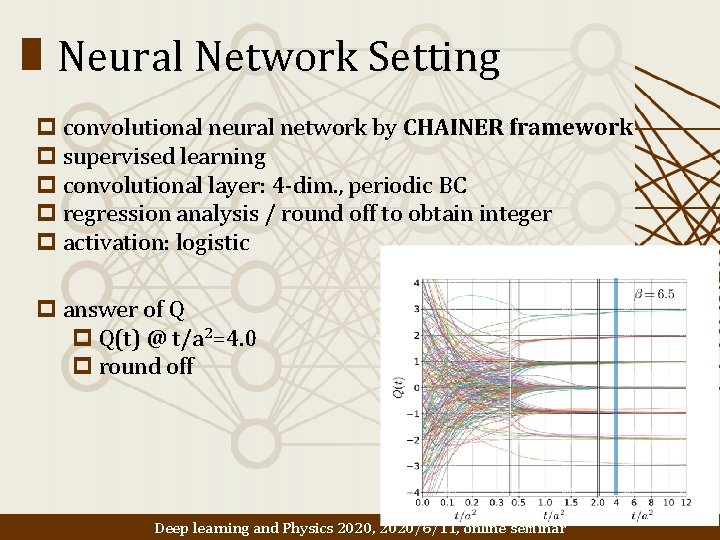
Neural Network Setting p convolutional neural network by CHAINER framework p supervised learning p convolutional layer: 4 -dim. , periodic BC p regression analysis / round off to obtain integer p activation: logistic p answer of Q p Q(t) @ t/a 2=4. 0 p round off Deep learning and Physics 2020, 2020/6/11, online seminar
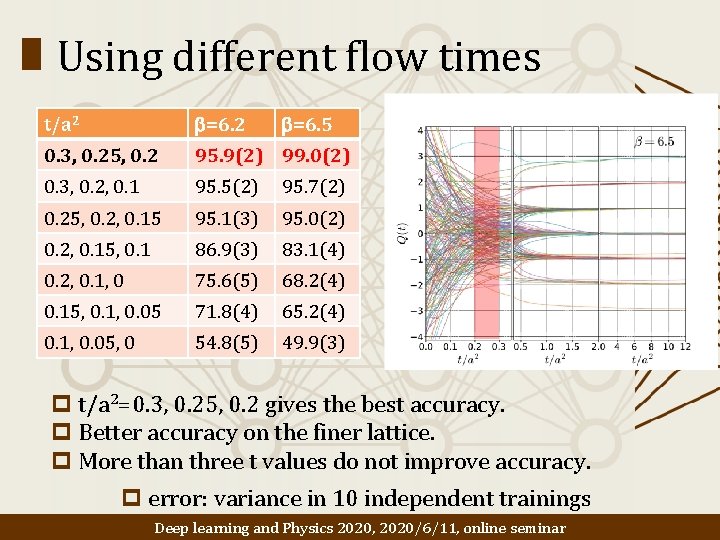
Using different flow times t/a 2 b=6. 5 0. 3, 0. 25, 0. 2 95. 9(2) 99. 0(2) 0. 3, 0. 2, 0. 1 95. 5(2) 95. 7(2) 0. 25, 0. 2, 0. 15 95. 1(3) 95. 0(2) 0. 2, 0. 15, 0. 1 86. 9(3) 83. 1(4) 0. 2, 0. 1, 0 75. 6(5) 68. 2(4) 0. 15, 0. 1, 0. 05 71. 8(4) 65. 2(4) 0. 1, 0. 05, 0 54. 8(5) 49. 9(3) p t/a 2=0. 3, 0. 25, 0. 2 gives the best accuracy. p Better accuracy on the finer lattice. p More than three t values do not improve accuracy. p error: variance in 10 independent trainings Deep learning and Physics 2020, 2020/6/11, online seminar
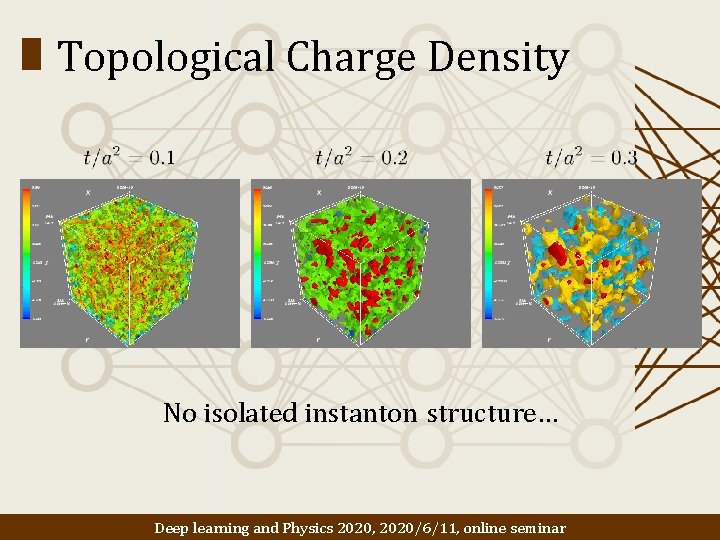
Topological Charge Density No isolated instanton structure… Deep learning and Physics 2020, 2020/6/11, online seminar
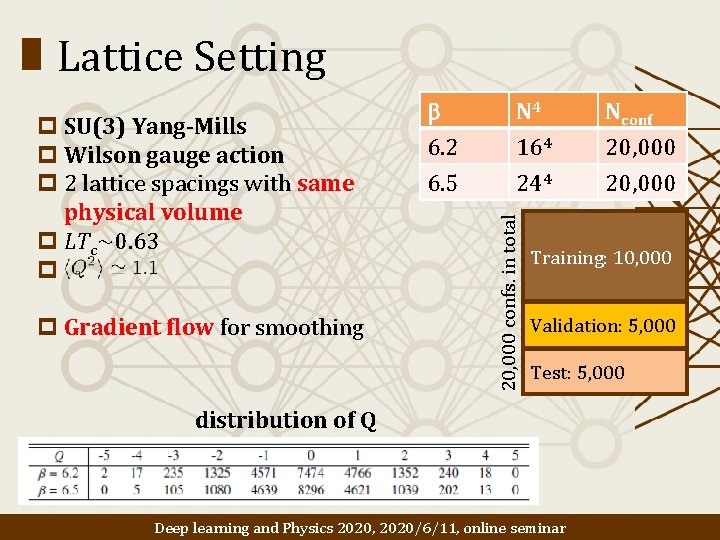
Lattice Setting p Gradient flow for smoothing N 4 164 244 20, 000 confs. in total p SU(3) Yang-Mills p Wilson gauge action p 2 lattice spacings with same physical volume p LTc~0. 63 p b 6. 2 6. 5 Nconf 20, 000 Training: 10, 000 Validation: 5, 000 Test: 5, 000 distribution of Q Deep learning and Physics 2020, 2020/6/11, online seminar
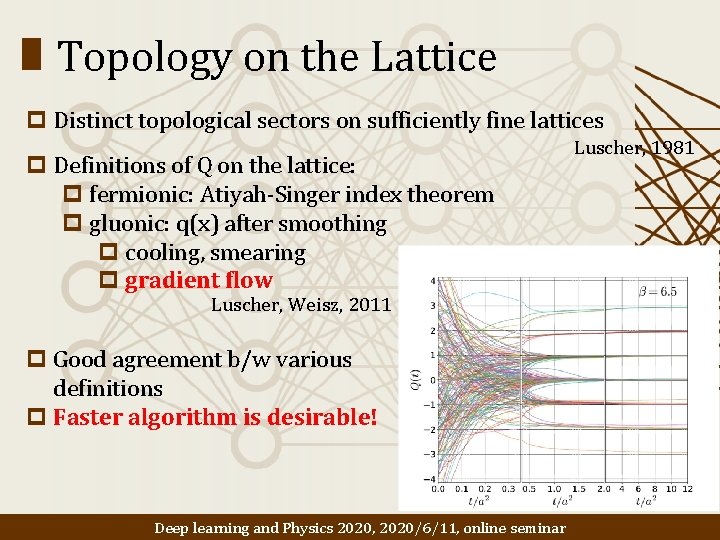
Topology on the Lattice p Distinct topological sectors on sufficiently fine lattices p Definitions of Q on the lattice: p fermionic: Atiyah-Singer index theorem p gluonic: q(x) after smoothing p cooling, smearing p gradient flow Luscher, Weisz, 2011 p Good agreement b/w various definitions p Faster algorithm is desirable! Deep learning and Physics 2020, 2020/6/11, online seminar Luscher, 1981
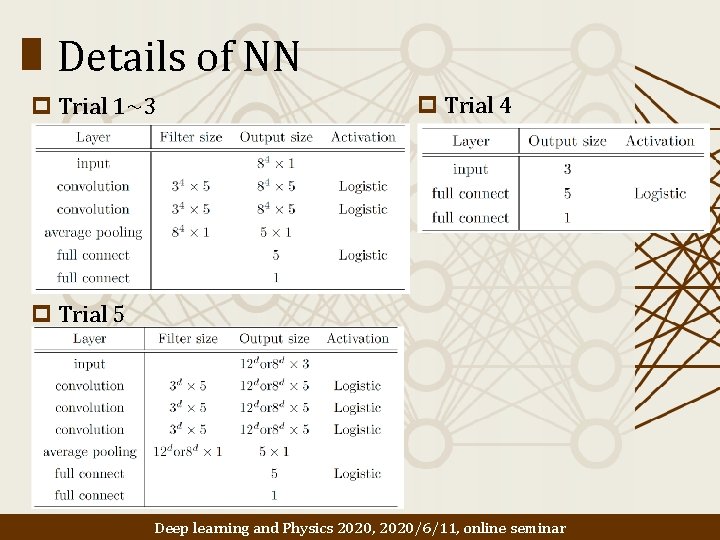
Details of NN p Trial 1~3 p Trial 4 p Trial 5 Deep learning and Physics 2020, 2020/6/11, online seminar