RESEARCH TEAM Darla K Munroe 1 PI Tao
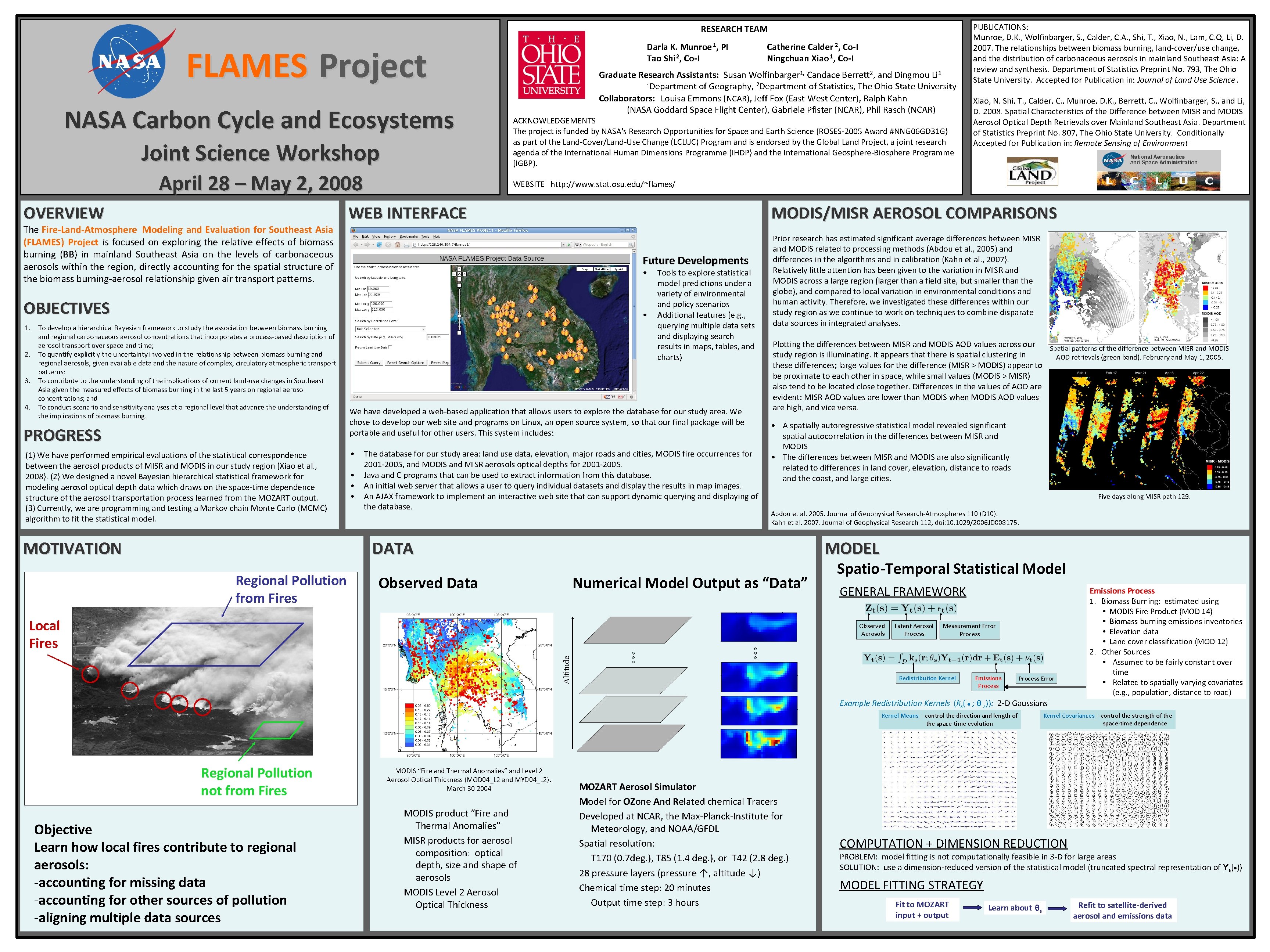
- Slides: 1
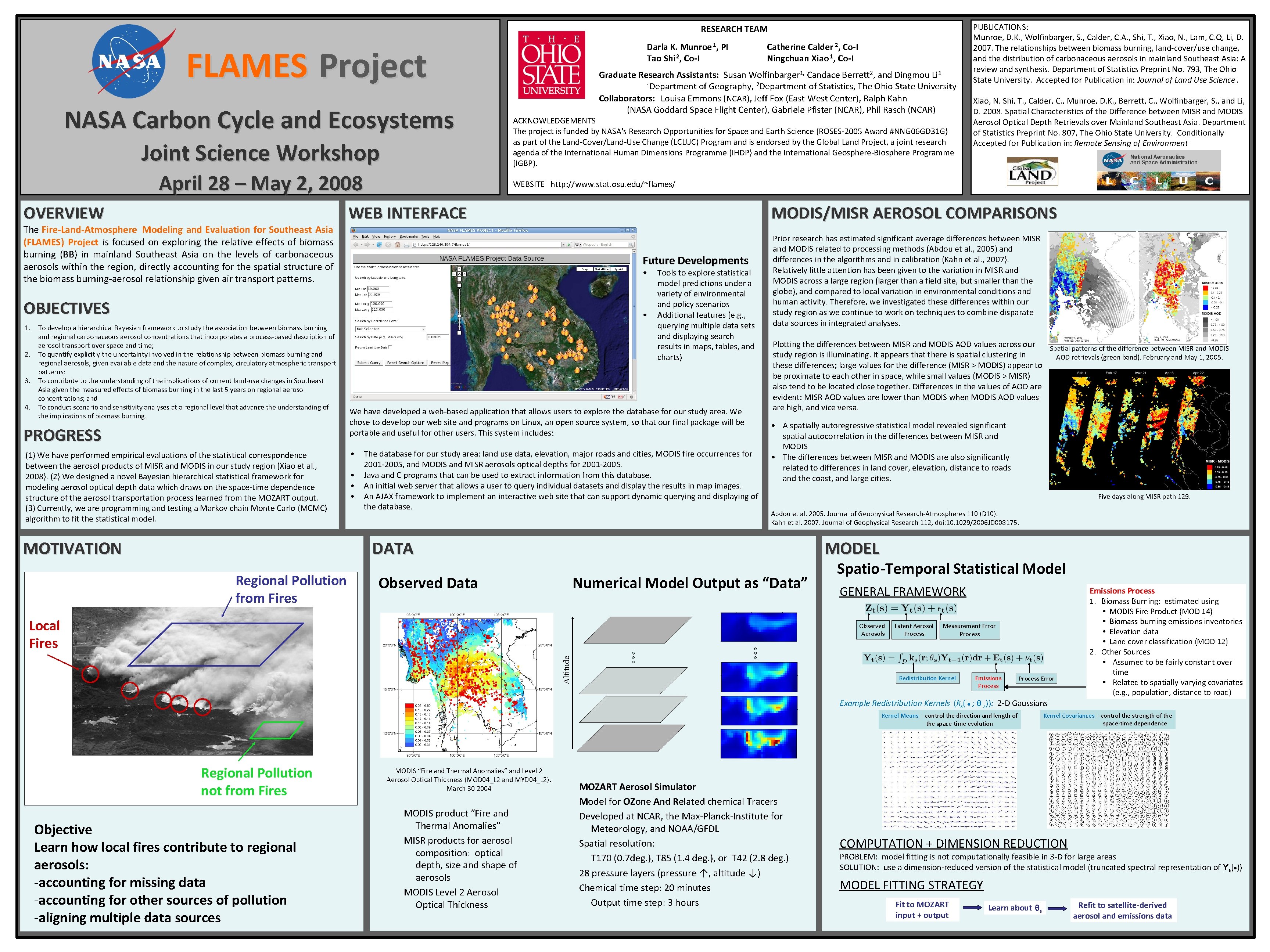
RESEARCH TEAM Darla K. Munroe 1, PI Tao Shi 2, Co-I FLAMES Project NASA Carbon Cycle and Ecosystems Joint Science Workshop April 28 – May 2, 2008 OVERVIEW The Fire-Land-Atmosphere Modeling and Evaluation for Southeast Asia (FLAMES) Project is focused on exploring the relative effects of biomass burning (BB) in mainland Southeast Asia on the levels of carbonaceous aerosols within the region, directly accounting for the spatial structure of the biomass burning-aerosol relationship given air transport patterns. Graduate Research Assistants: Susan Wolfinbarger 1, Candace Berrett 2, and Dingmou Li 1 1 Department of Geography, 2 Department of Statistics, The Ohio State University Collaborators: Louisa Emmons (NCAR), Jeff Fox (East-West Center), Ralph Kahn (NASA Goddard Space Flight Center), Gabriele Pfister (NCAR), Phil Rasch (NCAR) ACKNOWLEDGEMENTS The project is funded by NASA's Research Opportunities for Space and Earth Science (ROSES-2005 Award #NNG 06 GD 31 G) as part of the Land-Cover/Land-Use Change (LCLUC) Program and is endorsed by the Global Land Project, a joint research agenda of the International Human Dimensions Programme (IHDP) and the International Geosphere-Biosphere Programme (IGBP). WEB INTERFACE MODIS/MISR AEROSOL COMPARISONS Future Developments • 2. 3. 4. • To develop a hierarchical Bayesian framework to study the association between biomass burning and regional carbonaceous aerosol concentrations that incorporates a process-based description of aerosol transport over space and time; To quantify explicitly the uncertainty involved in the relationship between biomass burning and regional aerosols, given available data and the nature of complex, circulatory atmospheric transport patterns; To contribute to the understanding of the implications of current land-use changes in Southeast Asia given the measured effects of biomass burning in the last 5 years on regional aerosol concentrations; and To conduct scenario and sensitivity analyses at a regional level that advance the understanding of the implications of biomass burning. PROGRESS (1) We have performed empirical evaluations of the statistical correspondence between the aerosol products of MISR and MODIS in our study region (Xiao et al. , 2008). (2) We designed a novel Bayesian hierarchical statistical framework for modeling aerosol optical depth data which draws on the space-time dependence structure of the aerosol transportation process learned from the MOZART output. (3) Currently, we are programming and testing a Markov chain Monte Carlo (MCMC) algorithm to fit the statistical model. MOTIVATION Tools to explore statistical model predictions under a variety of environmental and policy scenarios Additional features (e. g. , querying multiple data sets and displaying search results in maps, tables, and charts) We have developed a web-based application that allows users to explore the database for our study area. We chose to develop our web site and programs on Linux, an open source system, so that our final package will be portable and useful for other users. This system includes: • • The database for our study area: land use data, elevation, major roads and cities, MODIS fire occurrences for 2001 -2005, and MODIS and MISR aerosols optical depths for 2001 -2005. Java and C programs that can be used to extract information from this database. An initial web server that allows a user to query individual datasets and display the results in map images. An AJAX framework to implement an interactive web site that can support dynamic querying and displaying of the database. Prior research has estimated significant average differences between MISR and MODIS related to processing methods (Abdou et al. , 2005) and differences in the algorithms and in calibration (Kahn et al. , 2007). Relatively little attention has been given to the variation in MISR and MODIS across a large region (larger than a field site, but smaller than the globe), and compared to local variation in environmental conditions and human activity. Therefore, we investigated these differences within our study region as we continue to work on techniques to combine disparate data sources in integrated analyses. Plotting the differences between MISR and MODIS AOD values across our Spatial patterns of the difference between MISR and MODIS study region is illuminating. It appears that there is spatial clustering in AOD retrievals (green band). February and May 1, 2005. these differences; large values for the difference (MISR > MODIS) appear to be proximate to each other in space, while small values (MODIS > MISR) also tend to be located close together. Differences in the values of AOD are evident: MISR AOD values are lower than MODIS when MODIS AOD values are high, and vice versa. • A spatially autoregressive statistical model revealed significant spatial autocorrelation in the differences between MISR and MODIS • The differences between MISR and MODIS are also significantly related to differences in land cover, elevation, distance to roads and the coast, and large cities. Five days along MISR path 129. Abdou et al. 2005. Journal of Geophysical Research-Atmospheres 110 (D 10). Kahn et al. 2007. Journal of Geophysical Research 112, doi: 10. 1029/2006 JD 008175. DATA Regional Pollution from Fires Xiao, N. Shi, T. , Calder, C. , Munroe, D. K. , Berrett, C. , Wolfinbarger, S. , and Li, D. 2008. Spatial Characteristics of the Difference between MISR and MODIS Aerosol Optical Depth Retrievals over Mainland Southeast Asia. Department of Statistics Preprint No. 807, The Ohio State University. Conditionally Accepted for Publication in: Remote Sensing of Environment WEBSITE http: //www. stat. osu. edu/~flames/ OBJECTIVES 1. Catherine Calder 2, Co-I Ningchuan Xiao 1, Co-I PUBLICATIONS: Munroe, D. K. , Wolfinbarger, S. , Calder, C. A. , Shi, T. , Xiao, N. , Lam, C. Q, Li, D. 2007. The relationships between biomass burning, land-cover/use change, and the distribution of carbonaceous aerosols in mainland Southeast Asia: A review and synthesis. Department of Statistics Preprint No. 793, The Ohio State University. Accepted for Publication in: Journal of Land Use Science. MODEL Observed Data Numerical Model Output as “Data” Local Fires Spatio-Temporal Statistical Model GENERAL FRAMEWORK Altitude Observed Aerosols Latent Aerosol Process Measurement Error Process Redistribution Kernel Emissions Process Error Example Redistribution Kernels (ks( ; s)): 2 -D Gaussians Kernel Means - control the direction and length of the space-time evolution Regional Pollution not from Fires Objective Learn how local fires contribute to regional aerosols: -accounting for missing data -accounting for other sources of pollution -aligning multiple data sources MODIS “Fire and Thermal Anomalies” and Level 2 Aerosol Optical Thickness (MOD 04_L 2 and MYD 04_L 2), March 30 2004 MODIS product “Fire and Thermal Anomalies” MISR products for aerosol composition: optical depth, size and shape of aerosols MODIS Level 2 Aerosol Optical Thickness MOZART Aerosol Simulator Model for OZone And Related chemical Tracers Developed at NCAR, the Max-Planck-Institute for Meteorology, and NOAA/GFDL Spatial resolution: T 170 (0. 7 deg. ), T 85 (1. 4 deg. ), or T 42 (2. 8 deg. ) 28 pressure layers (pressure ↑, altitude ↓) Chemical time step: 20 minutes Output time step: 3 hours Emissions Process 1. Biomass Burning: estimated using • MODIS Fire Product (MOD 14) • Biomass burning emissions inventories • Elevation data • Land cover classification (MOD 12) 2. Other Sources • Assumed to be fairly constant over time • Related to spatially-varying covariates (e. g. , population, distance to road) Kernel Covariances - control the strength of the space-time dependence COMPUTATION + DIMENSION REDUCTION PROBLEM: model fitting is not computationally feasible in 3 -D for large areas SOLUTION: use a dimension-reduced version of the statistical model (truncated spectral representation of Yt( )) MODEL FITTING STRATEGY Fit to MOZART input + output Learn about s Refit to satellite-derived aerosol and emissions data