Prosody in RecognitionUnderstanding JH 1162022 1 Prosody in
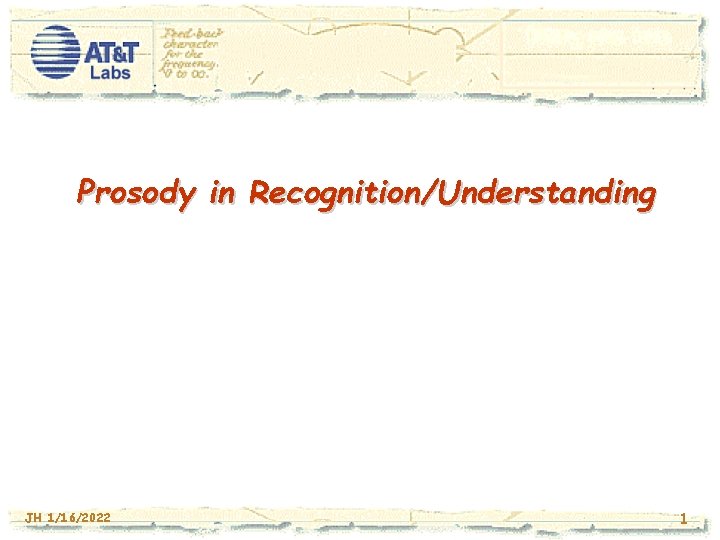
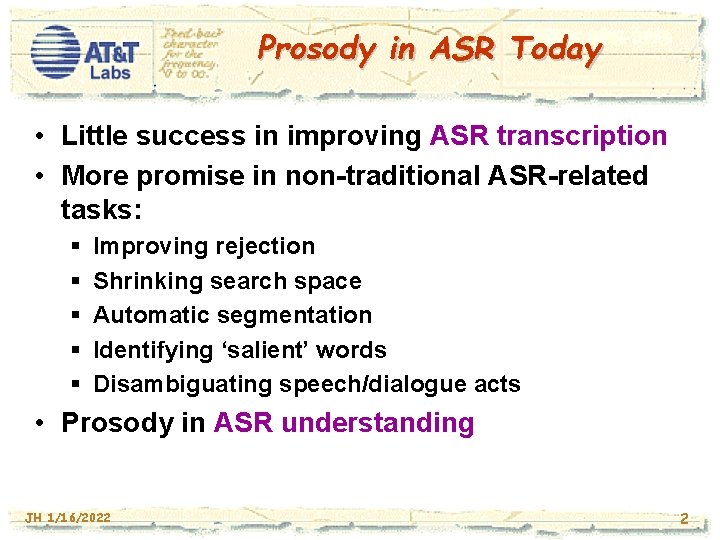
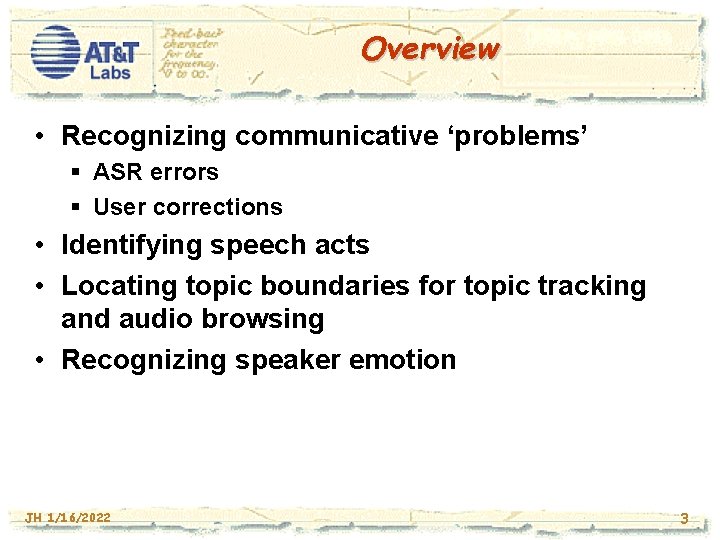
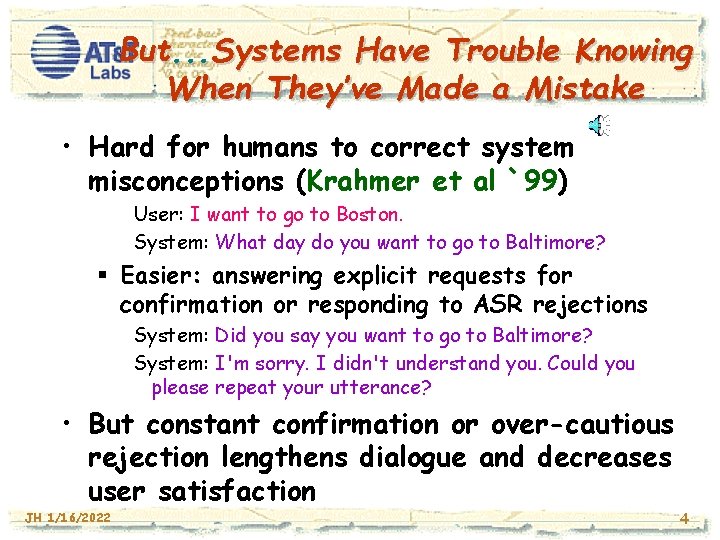
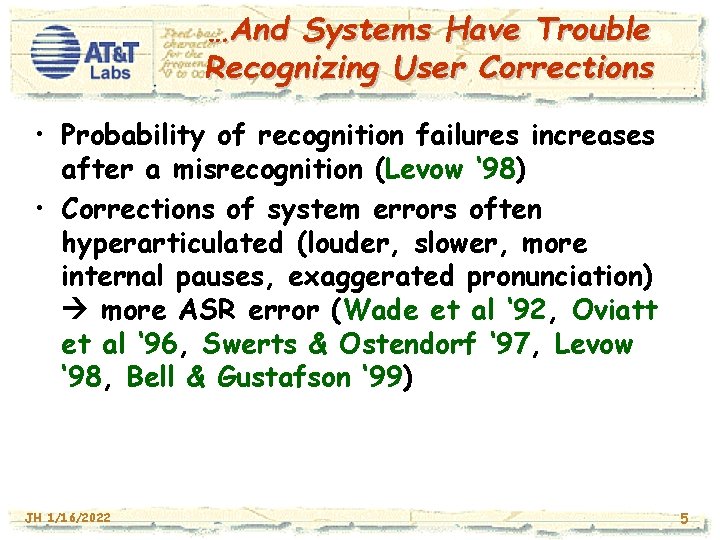
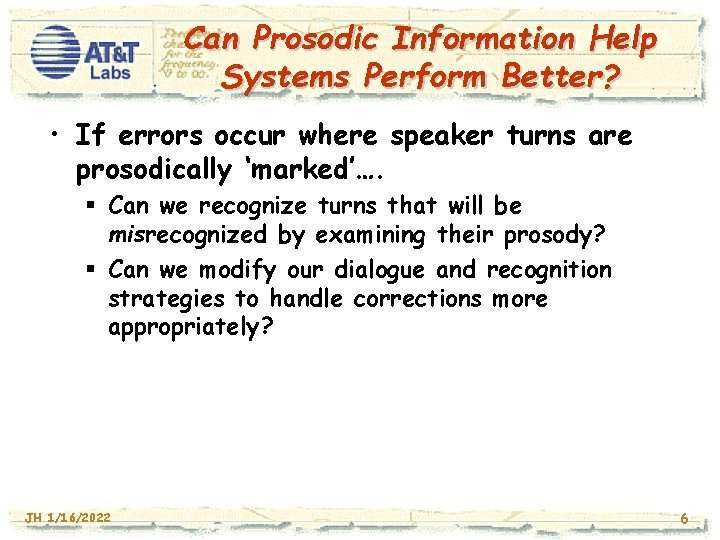
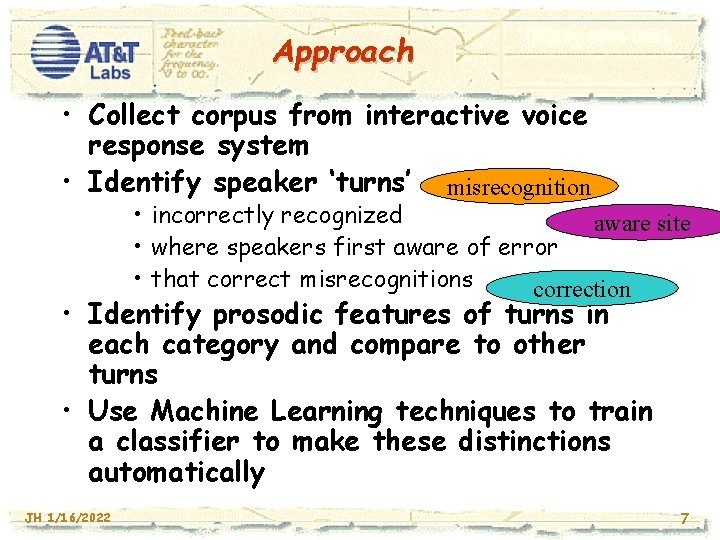
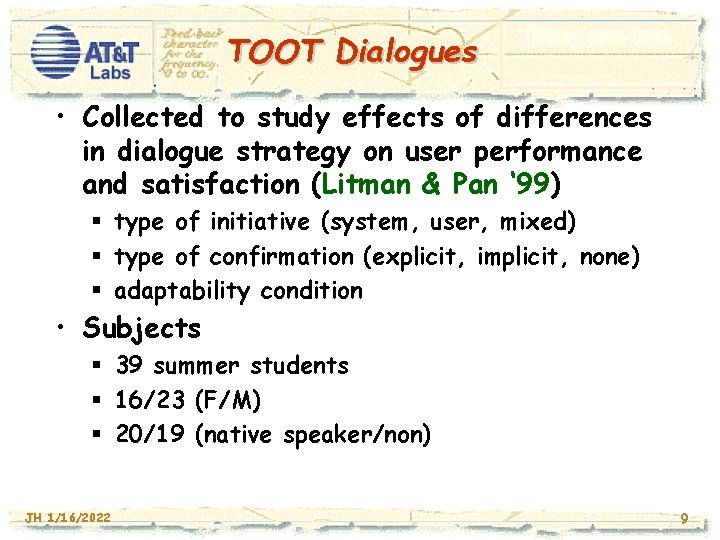
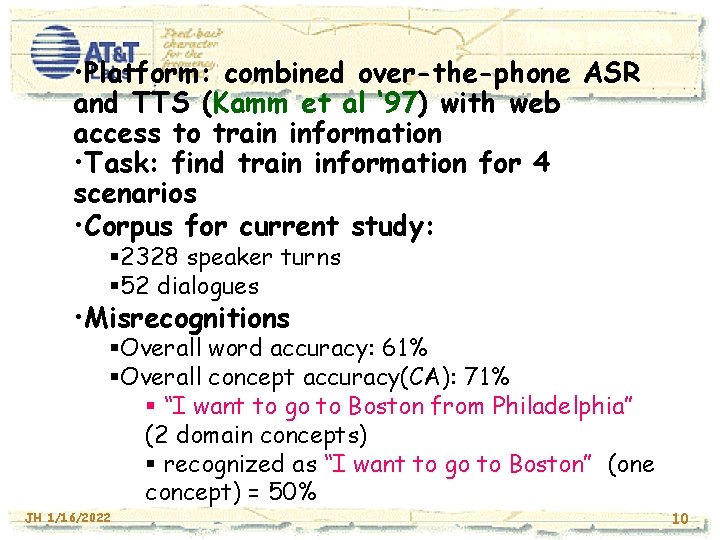
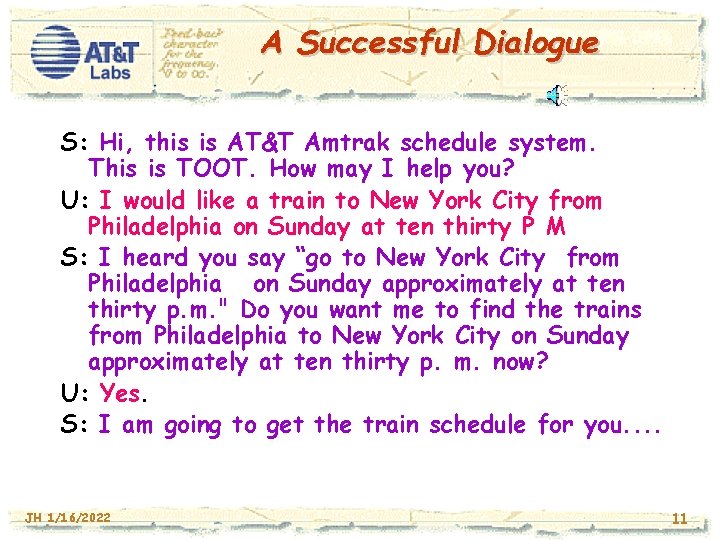
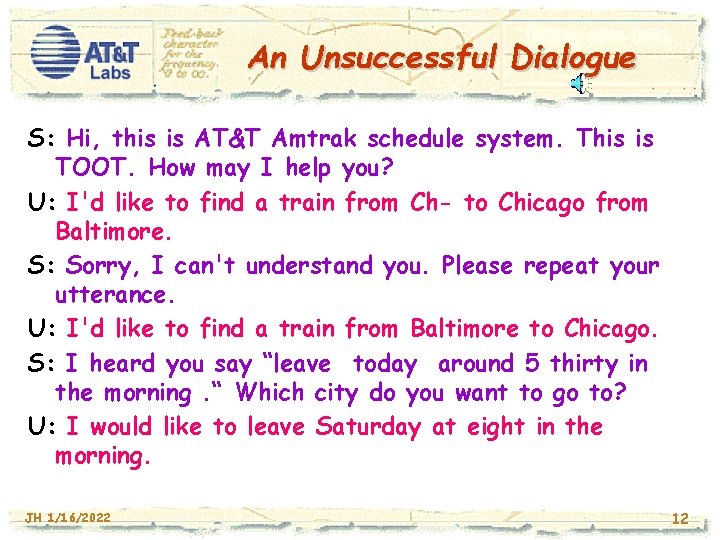
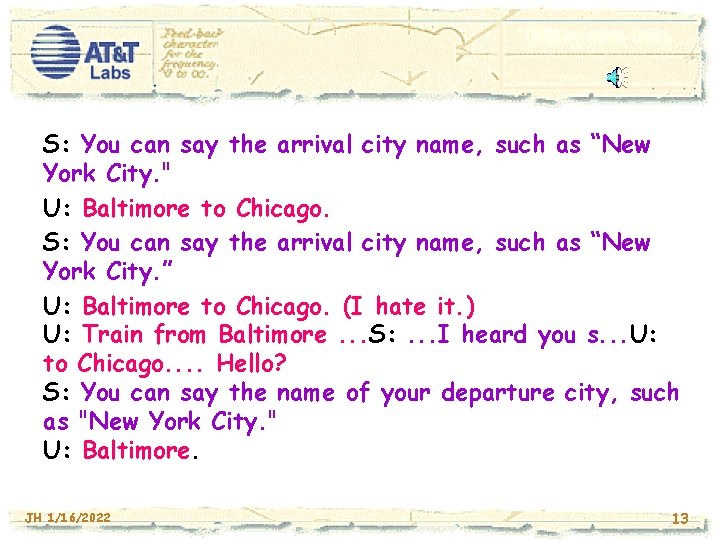
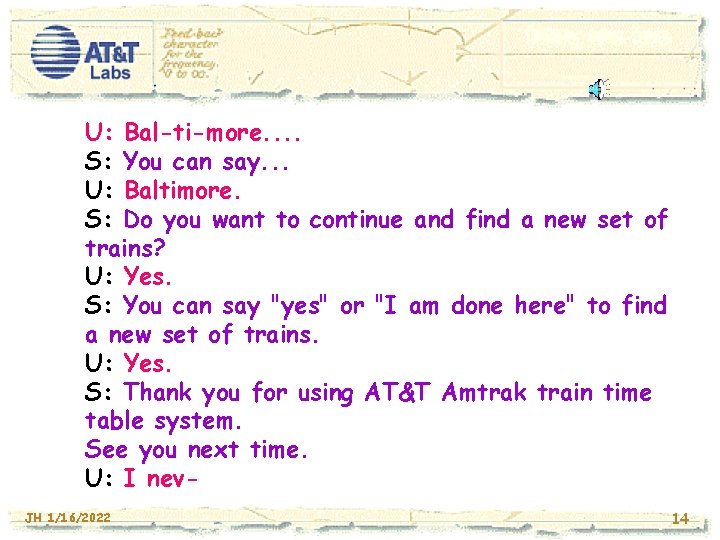
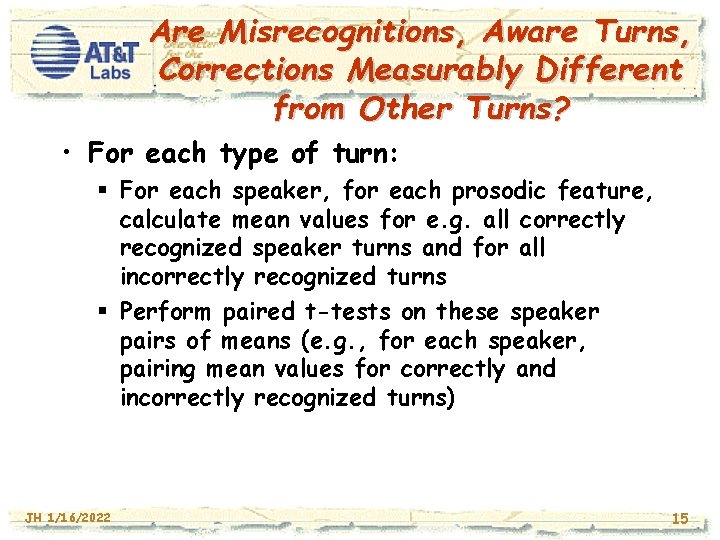
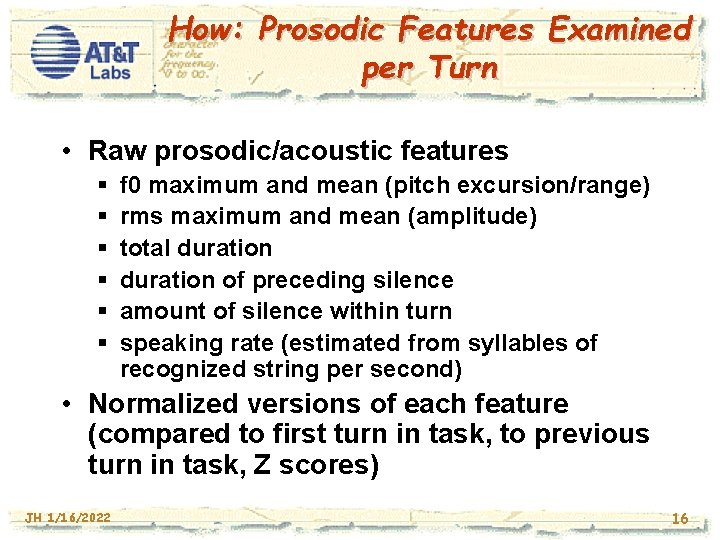
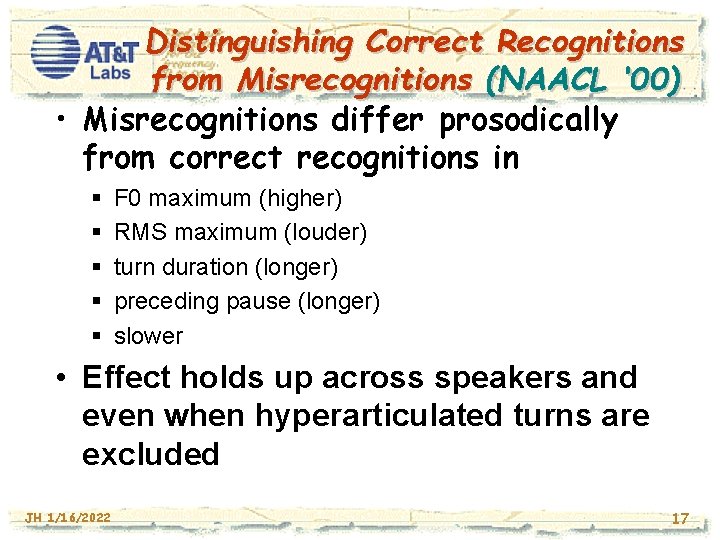
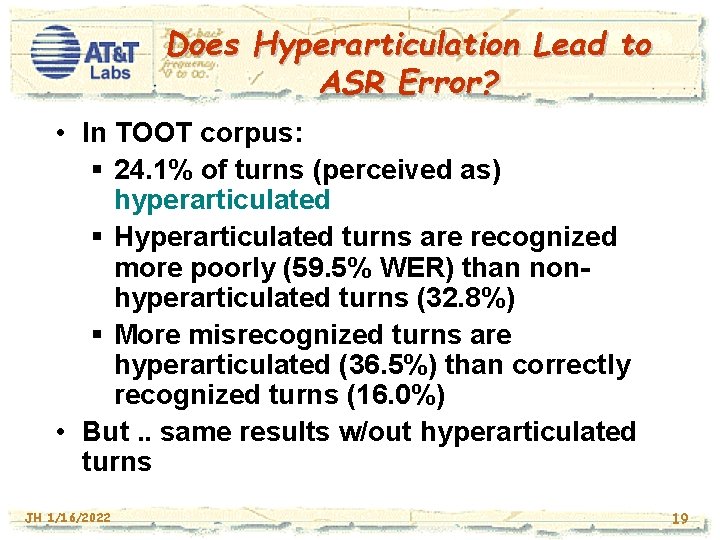
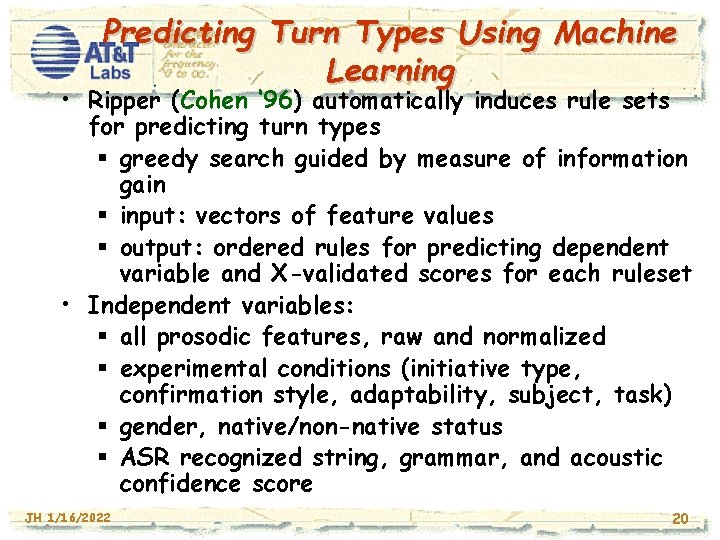
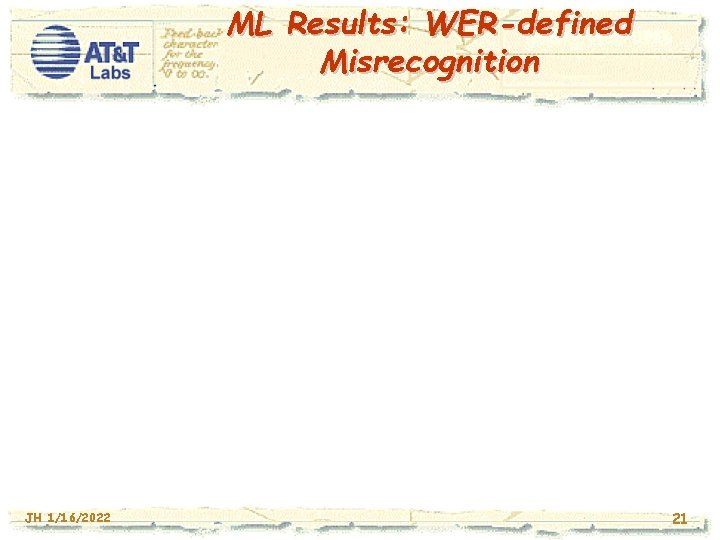
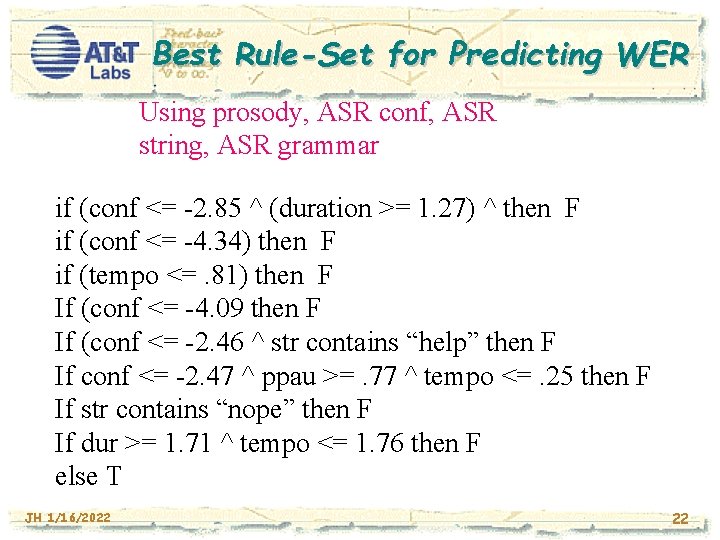
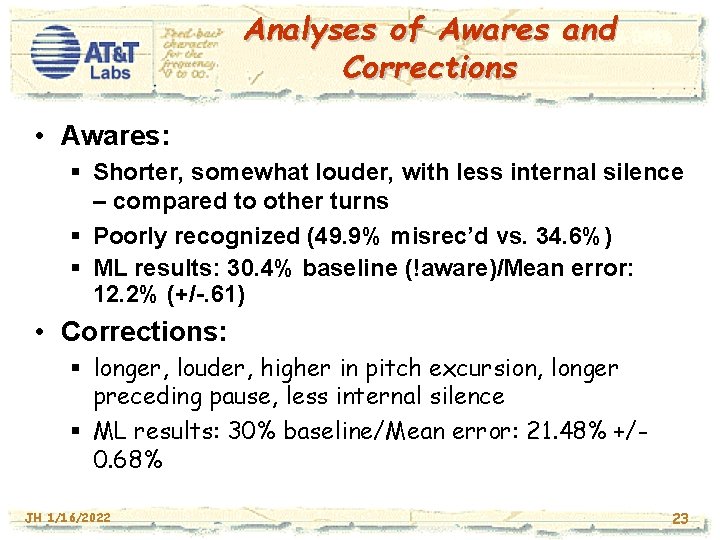
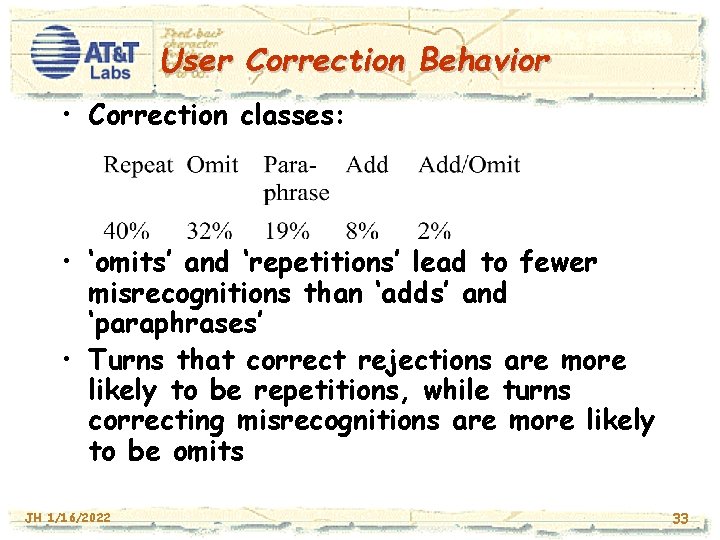
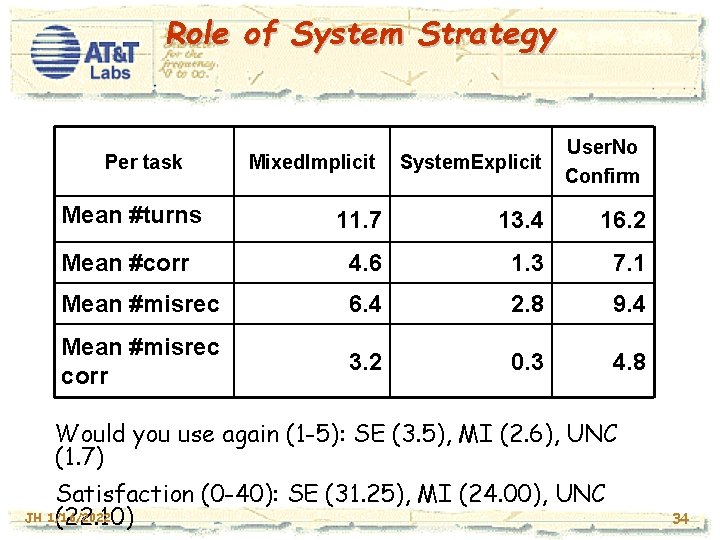
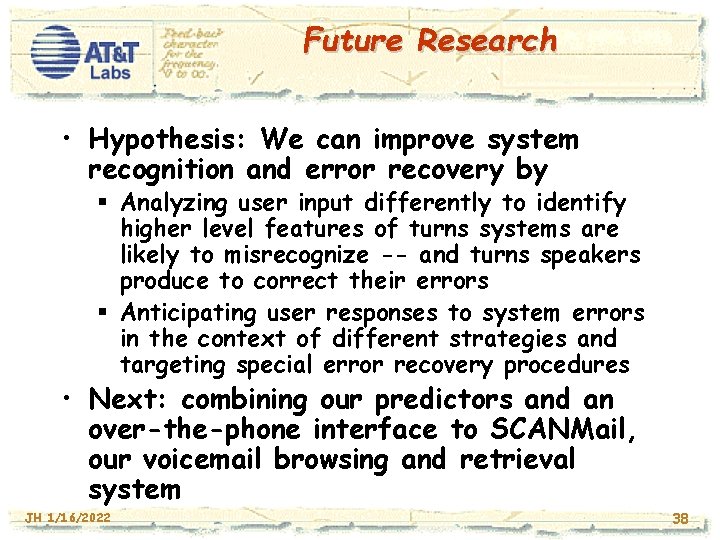
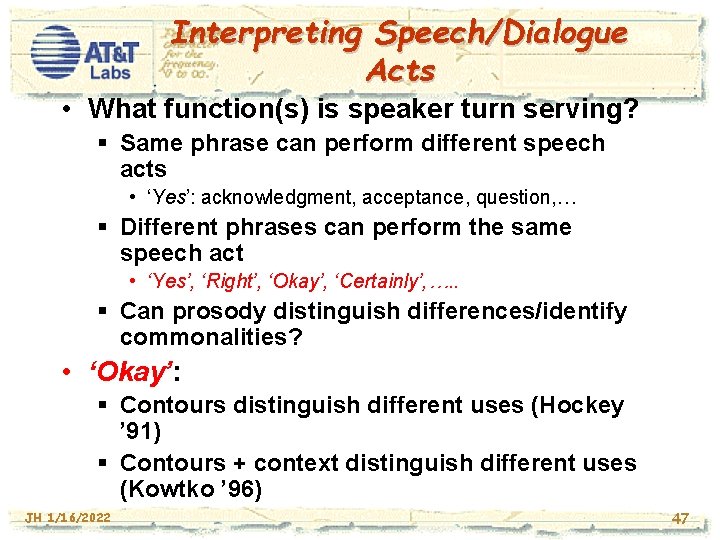
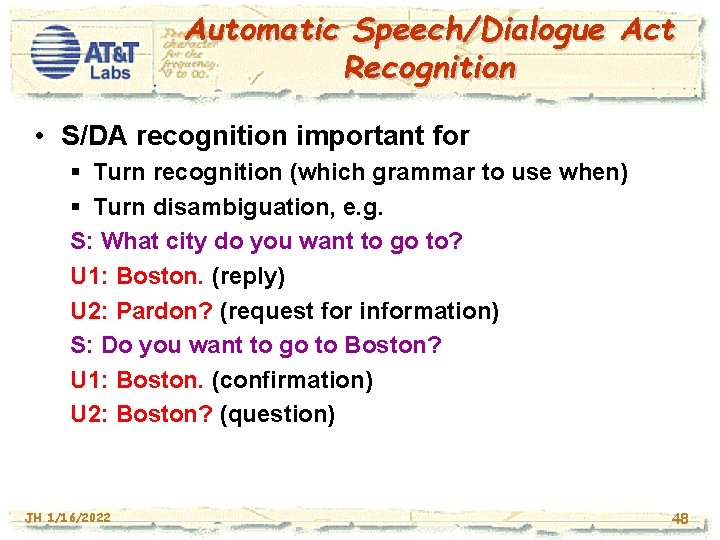
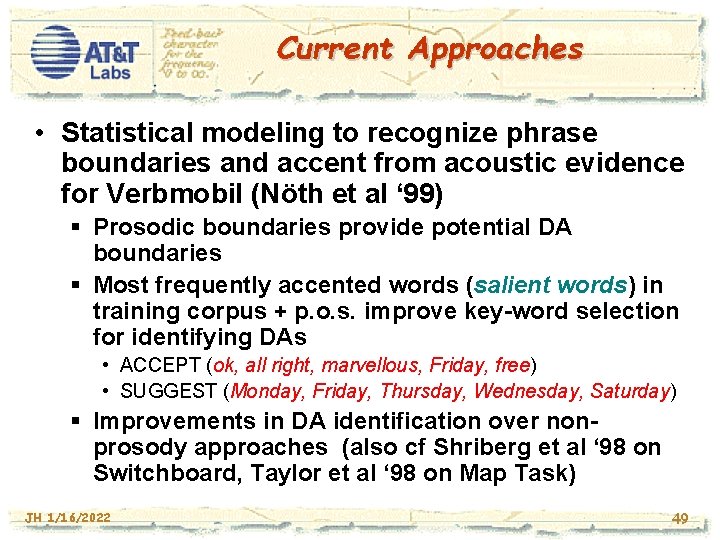
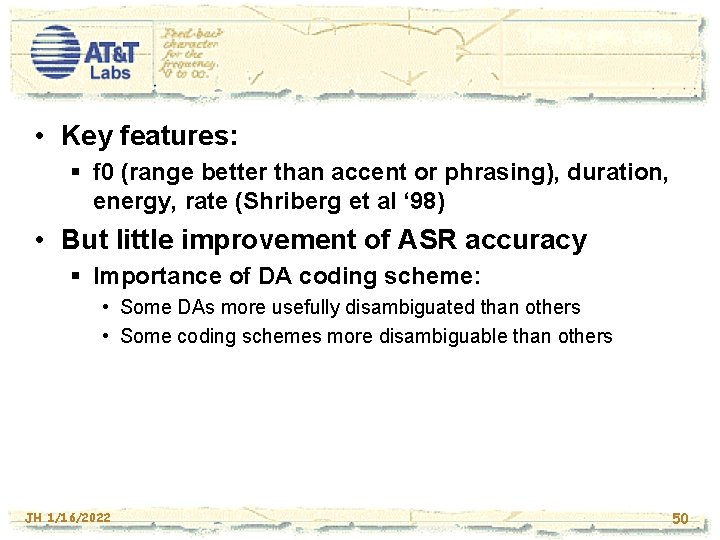
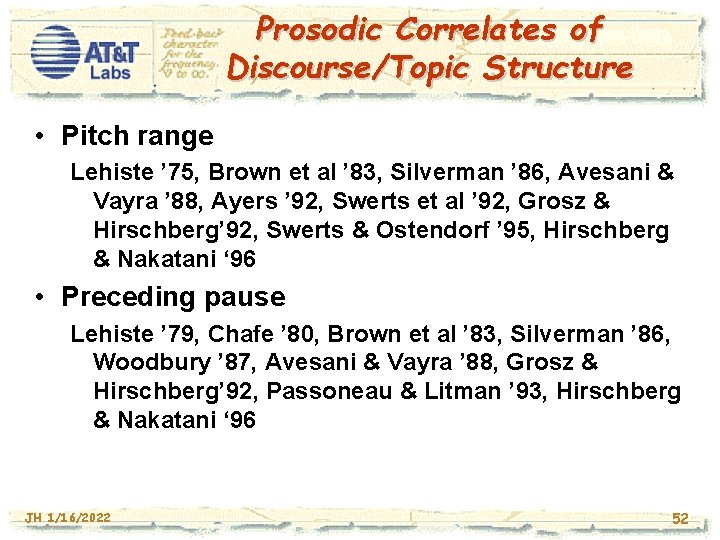
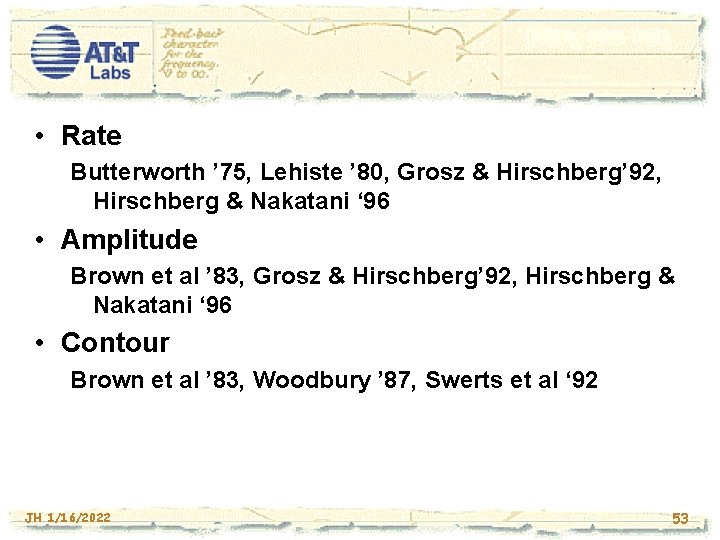
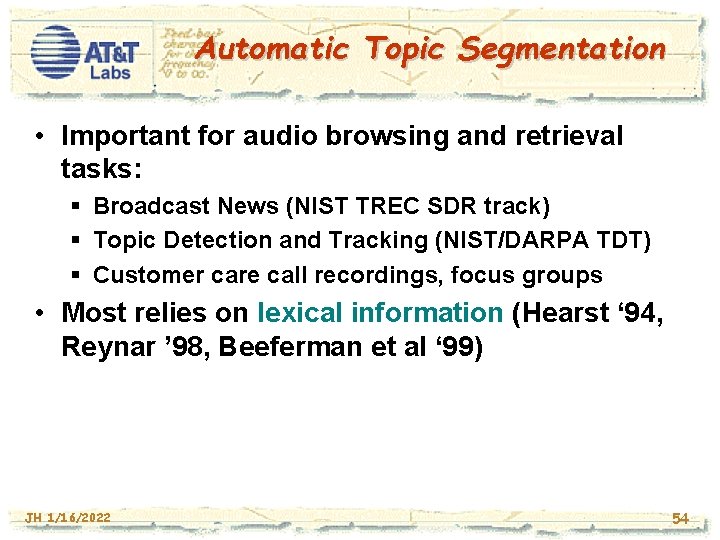
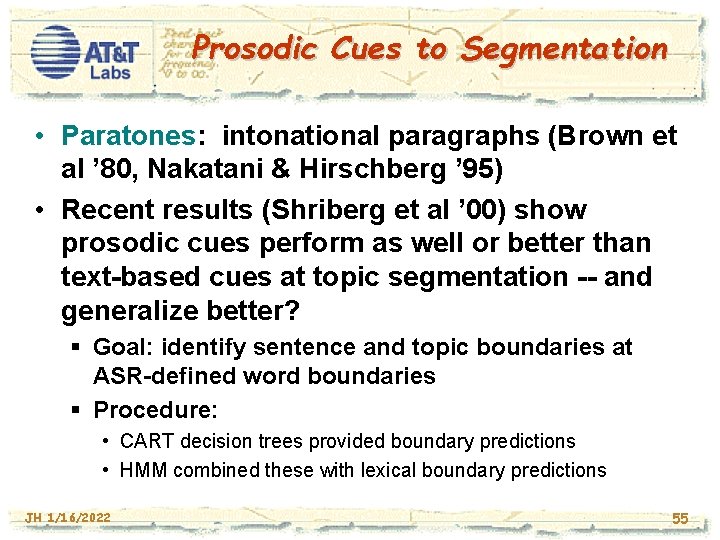
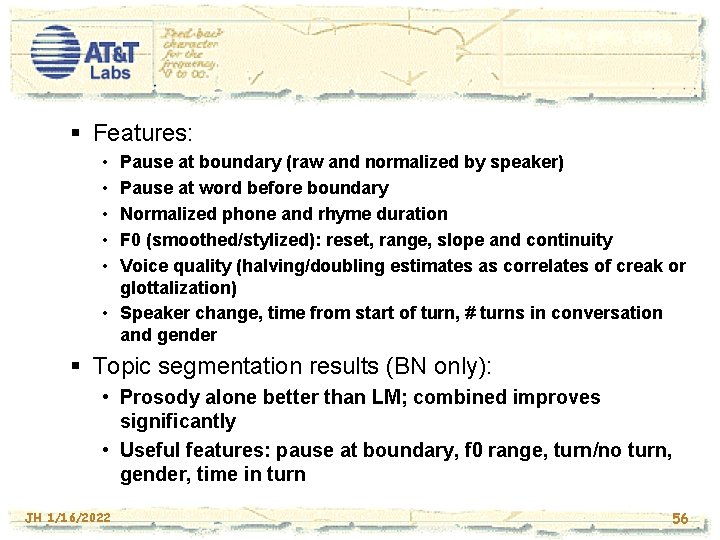
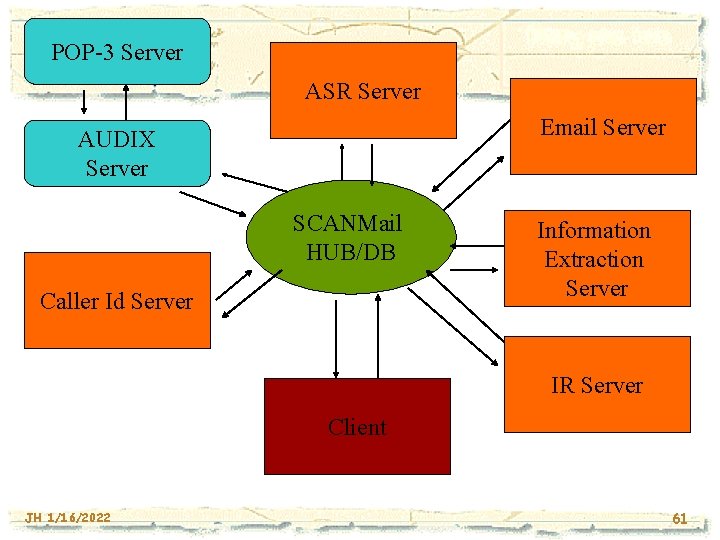
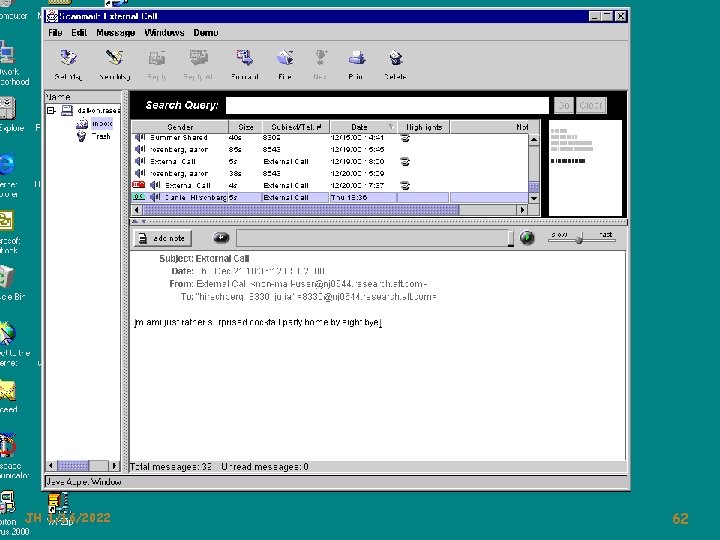
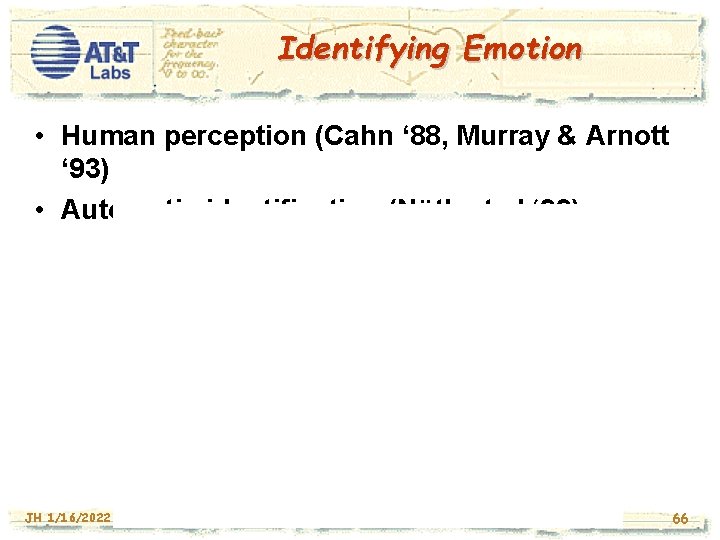
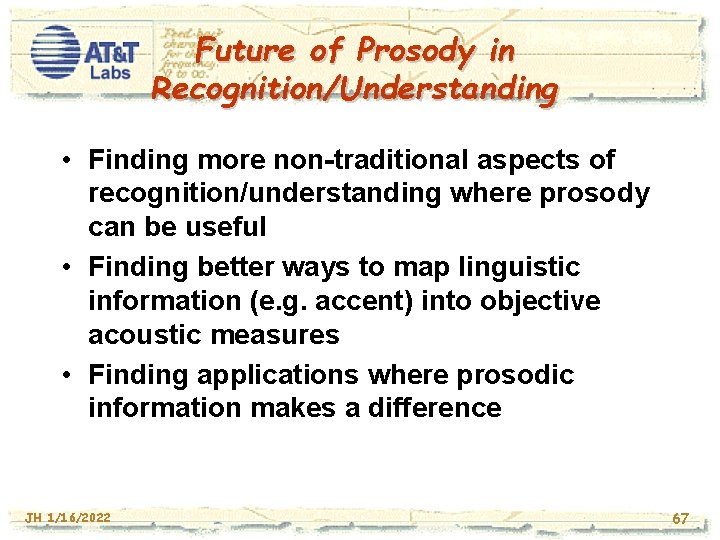
- Slides: 37
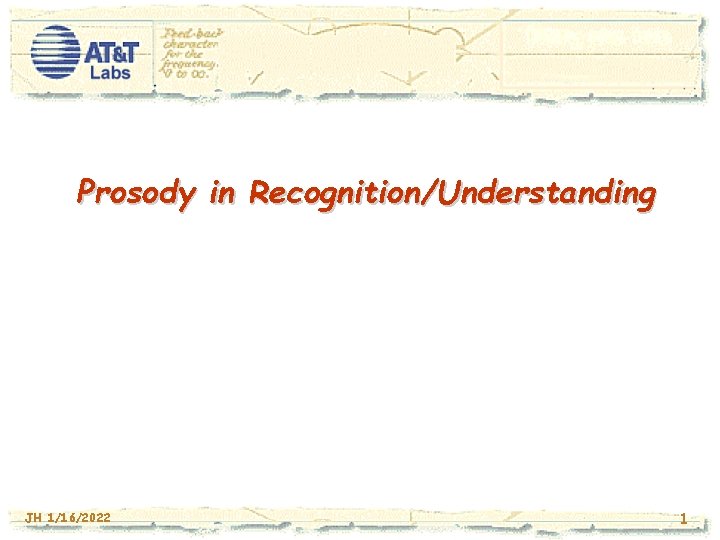
Prosody in Recognition/Understanding JH 1/16/2022 1
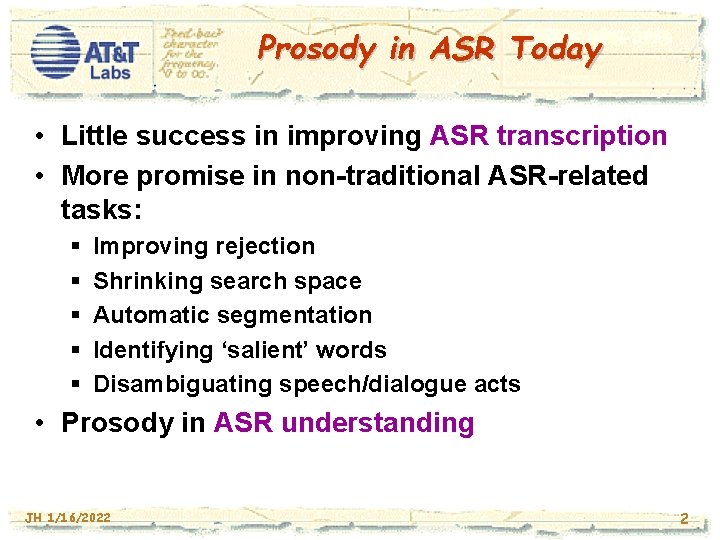
Prosody in ASR Today • Little success in improving ASR transcription • More promise in non-traditional ASR-related tasks: § § § Improving rejection Shrinking search space Automatic segmentation Identifying ‘salient’ words Disambiguating speech/dialogue acts • Prosody in ASR understanding JH 1/16/2022 2
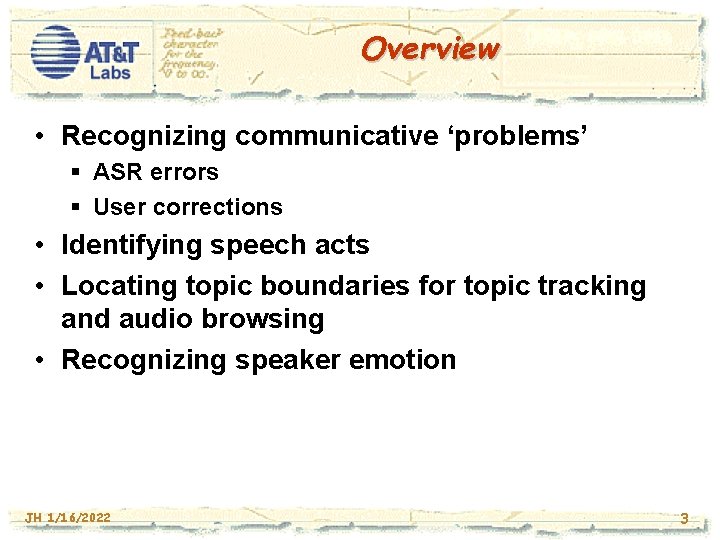
Overview • Recognizing communicative ‘problems’ § ASR errors § User corrections • Identifying speech acts • Locating topic boundaries for topic tracking and audio browsing • Recognizing speaker emotion JH 1/16/2022 3
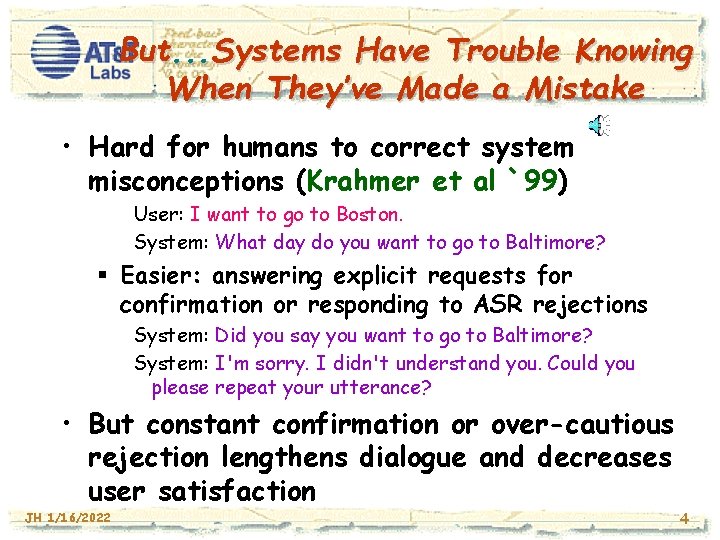
But. . . Systems Have Trouble Knowing When They’ve Made a Mistake • Hard for humans to correct system misconceptions (Krahmer et al `99) User: I want to go to Boston. System: What day do you want to go to Baltimore? § Easier: answering explicit requests for confirmation or responding to ASR rejections System: Did you say you want to go to Baltimore? System: I'm sorry. I didn't understand you. Could you please repeat your utterance? • But constant confirmation or over-cautious rejection lengthens dialogue and decreases user satisfaction JH 1/16/2022 4
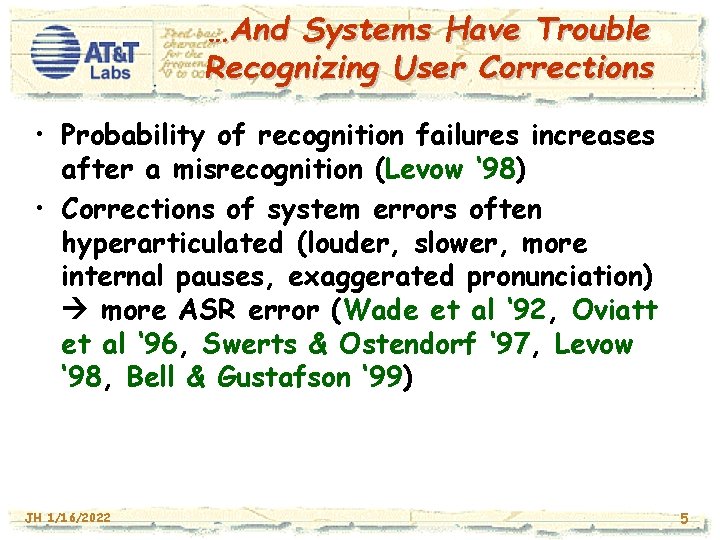
…And Systems Have Trouble Recognizing User Corrections • Probability of recognition failures increases after a misrecognition (Levow ‘ 98) • Corrections of system errors often hyperarticulated (louder, slower, more internal pauses, exaggerated pronunciation) more ASR error (Wade et al ‘ 92, Oviatt et al ‘ 96, Swerts & Ostendorf ‘ 97, Levow ‘ 98, Bell & Gustafson ‘ 99) JH 1/16/2022 5
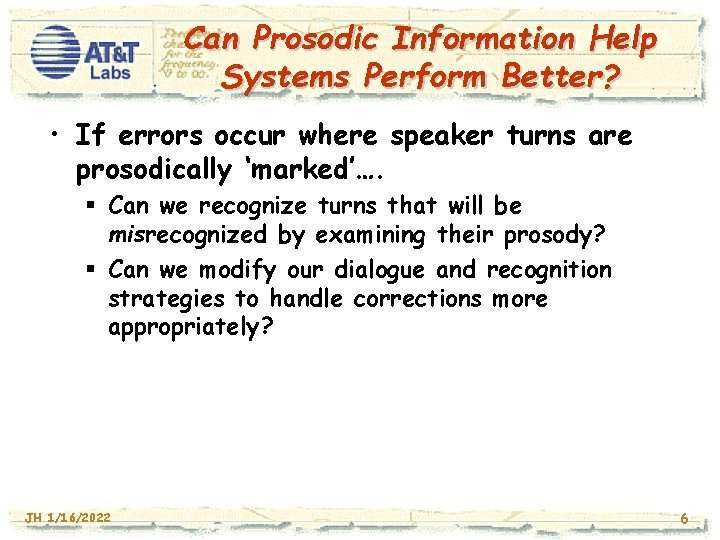
Can Prosodic Information Help Systems Perform Better? • If errors occur where speaker turns are prosodically ‘marked’…. § Can we recognize turns that will be misrecognized by examining their prosody? § Can we modify our dialogue and recognition strategies to handle corrections more appropriately? JH 1/16/2022 6
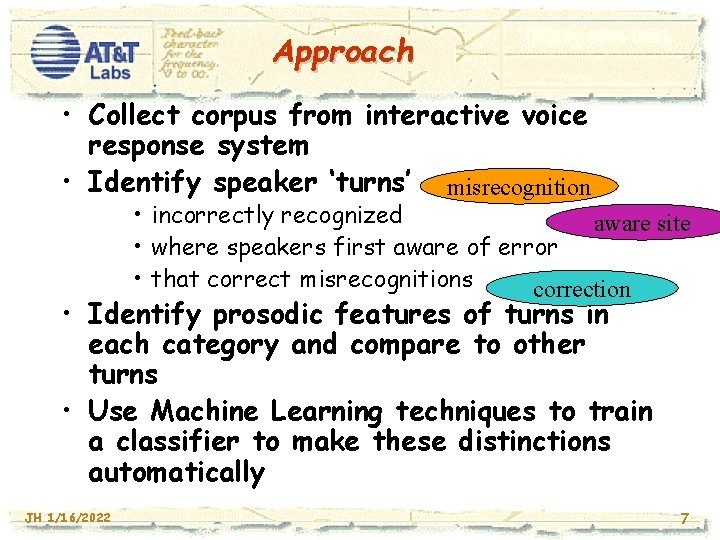
Approach • Collect corpus from interactive voice response system • Identify speaker ‘turns’ misrecognition • incorrectly recognized aware site • where speakers first aware of error • that correct misrecognitions correction • Identify prosodic features of turns in each category and compare to other turns • Use Machine Learning techniques to train a classifier to make these distinctions automatically JH 1/16/2022 7
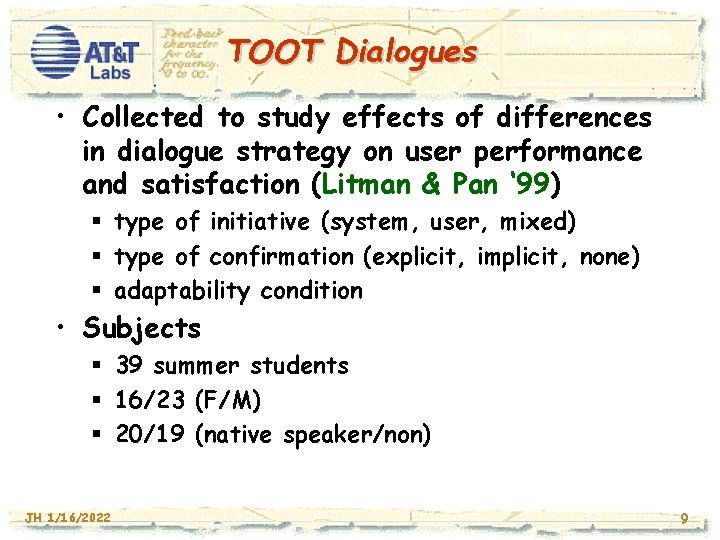
TOOT Dialogues • Collected to study effects of differences in dialogue strategy on user performance and satisfaction (Litman & Pan ‘ 99) § type of initiative (system, user, mixed) § type of confirmation (explicit, implicit, none) § adaptability condition • Subjects § 39 summer students § 16/23 (F/M) § 20/19 (native speaker/non) JH 1/16/2022 9
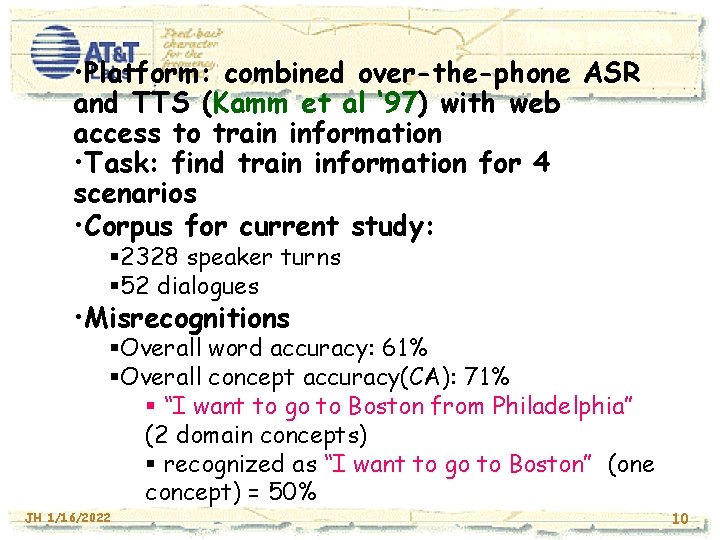
• Platform: combined over-the-phone ASR and TTS (Kamm et al ‘ 97) with web access to train information • Task: find train information for 4 scenarios • Corpus for current study: § 2328 speaker turns § 52 dialogues • Misrecognitions §Overall word accuracy: 61% §Overall concept accuracy(CA): 71% § “I want to go to Boston from Philadelphia” (2 domain concepts) § recognized as “I want to go to Boston” (one concept) = 50% JH 1/16/2022 10
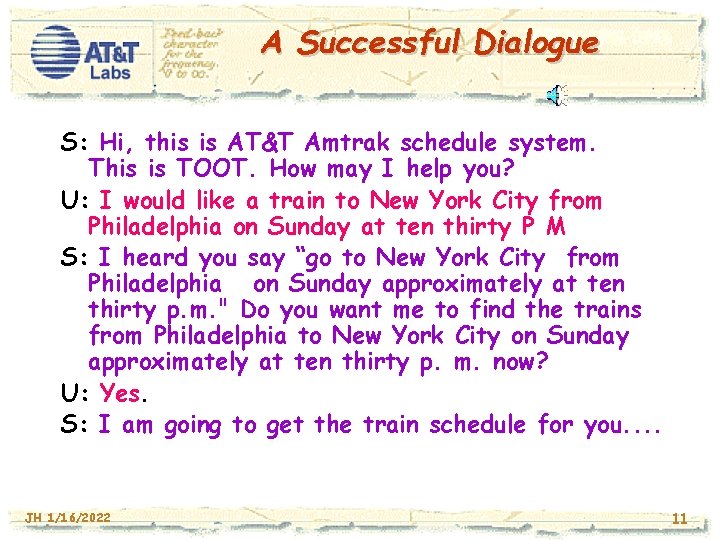
A Successful Dialogue S: Hi, this is AT&T Amtrak schedule system. This is TOOT. How may I help you? U: I would like a train to New York City from Philadelphia on Sunday at ten thirty P M S: I heard you say “go to New York City from Philadelphia on Sunday approximately at ten thirty p. m. " Do you want me to find the trains from Philadelphia to New York City on Sunday approximately at ten thirty p. m. now? U: Yes. S: I am going to get the train schedule for you. . JH 1/16/2022 11
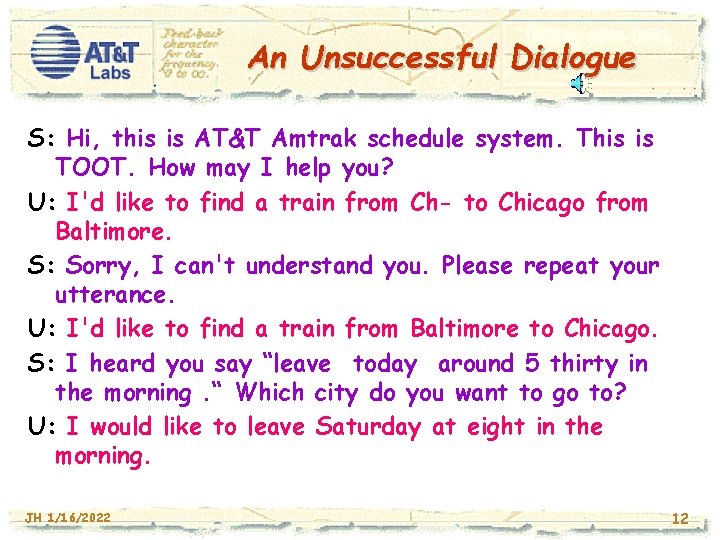
An Unsuccessful Dialogue S: Hi, this is AT&T Amtrak schedule system. This is TOOT. How may I help you? U: I'd like to find a train from Ch- to Chicago from Baltimore. S: Sorry, I can't understand you. Please repeat your utterance. U: I'd like to find a train from Baltimore to Chicago. S: I heard you say “leave today around 5 thirty in the morning. “ Which city do you want to go to? U: I would like to leave Saturday at eight in the morning. JH 1/16/2022 12
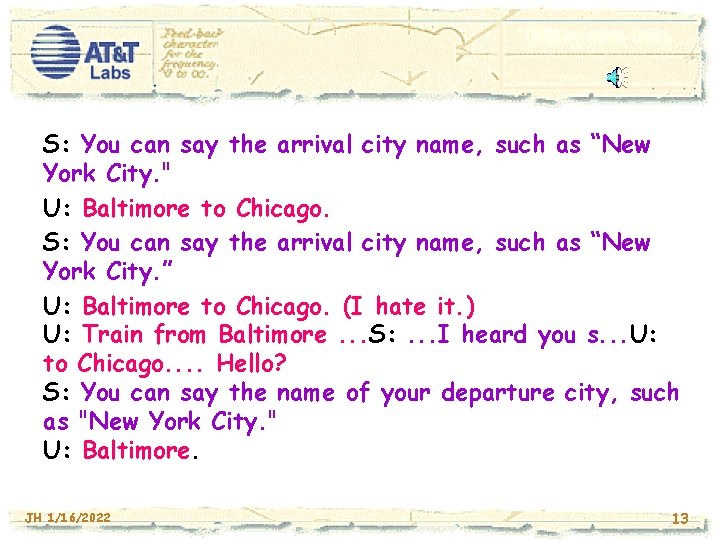
S: You can say the arrival city name, such as “New York City. " U: Baltimore to Chicago. S: You can say the arrival city name, such as “New York City. ” U: Baltimore to Chicago. (I hate it. ) U: Train from Baltimore. . . S: . . . I heard you s. . . U: to Chicago. . Hello? S: You can say the name of your departure city, such as "New York City. " U: Baltimore. JH 1/16/2022 13
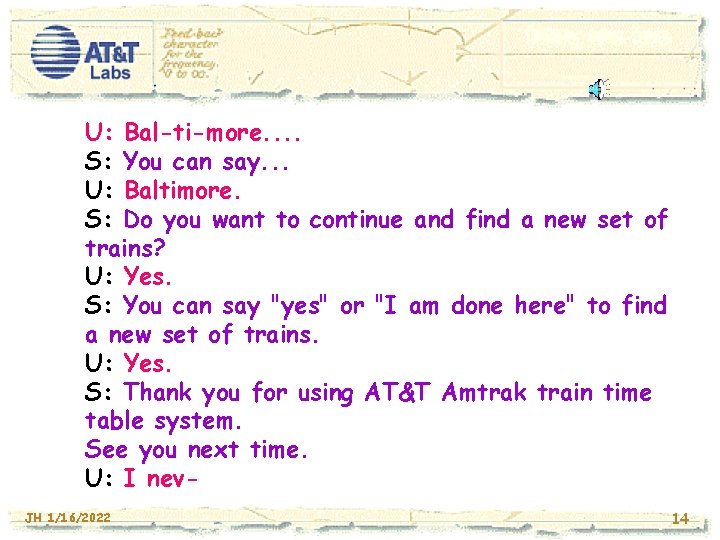
U: Bal-ti-more. . S: You can say. . . U: Baltimore. S: Do you want to continue and find a new set of trains? U: Yes. S: You can say "yes" or "I am done here" to find a new set of trains. U: Yes. S: Thank you for using AT&T Amtrak train time table system. See you next time. U: I nev. JH 1/16/2022 14
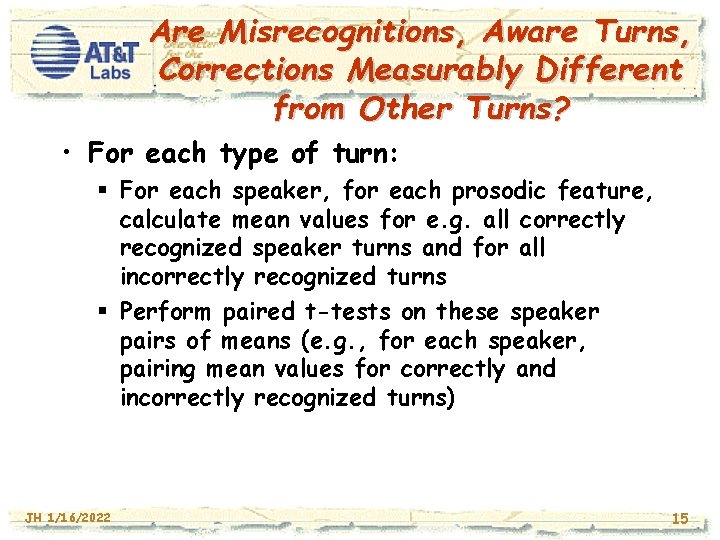
Are Misrecognitions, Aware Turns, Corrections Measurably Different from Other Turns? • For each type of turn: § For each speaker, for each prosodic feature, calculate mean values for e. g. all correctly recognized speaker turns and for all incorrectly recognized turns § Perform paired t-tests on these speaker pairs of means (e. g. , for each speaker, pairing mean values for correctly and incorrectly recognized turns) JH 1/16/2022 15
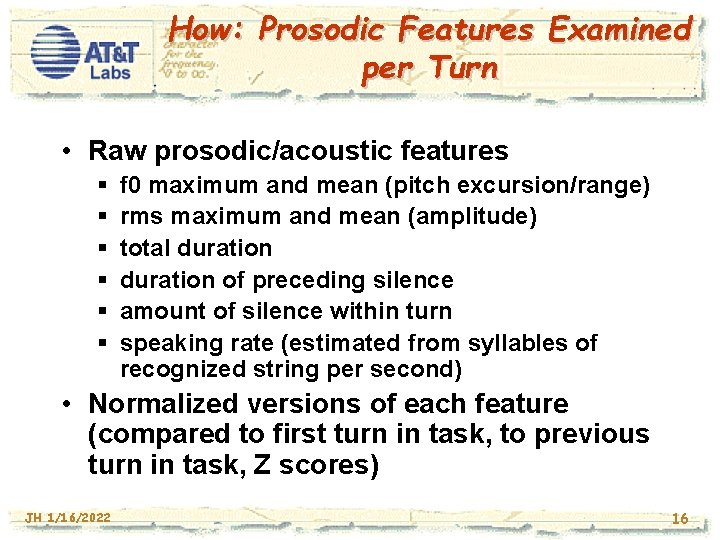
How: Prosodic Features Examined per Turn • Raw prosodic/acoustic features § § § f 0 maximum and mean (pitch excursion/range) rms maximum and mean (amplitude) total duration of preceding silence amount of silence within turn speaking rate (estimated from syllables of recognized string per second) • Normalized versions of each feature (compared to first turn in task, to previous turn in task, Z scores) JH 1/16/2022 16
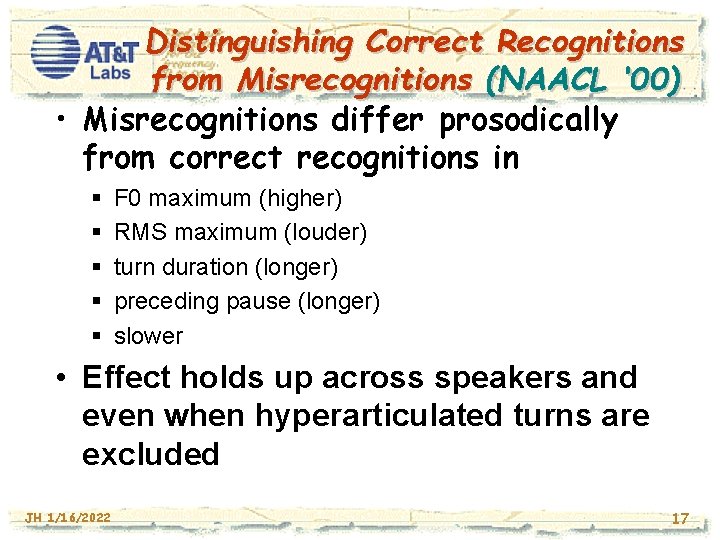
Distinguishing Correct Recognitions from Misrecognitions (NAACL ‘ 00) • Misrecognitions differ prosodically from correct recognitions in § § § F 0 maximum (higher) RMS maximum (louder) turn duration (longer) preceding pause (longer) slower • Effect holds up across speakers and even when hyperarticulated turns are excluded JH 1/16/2022 17
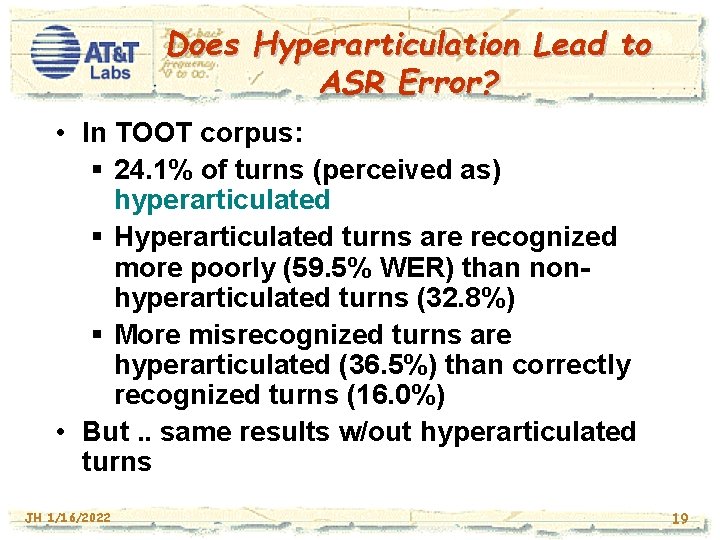
Does Hyperarticulation Lead to ASR Error? • In TOOT corpus: § 24. 1% of turns (perceived as) hyperarticulated § Hyperarticulated turns are recognized more poorly (59. 5% WER) than nonhyperarticulated turns (32. 8%) § More misrecognized turns are hyperarticulated (36. 5%) than correctly recognized turns (16. 0%) • But. . same results w/out hyperarticulated turns JH 1/16/2022 19
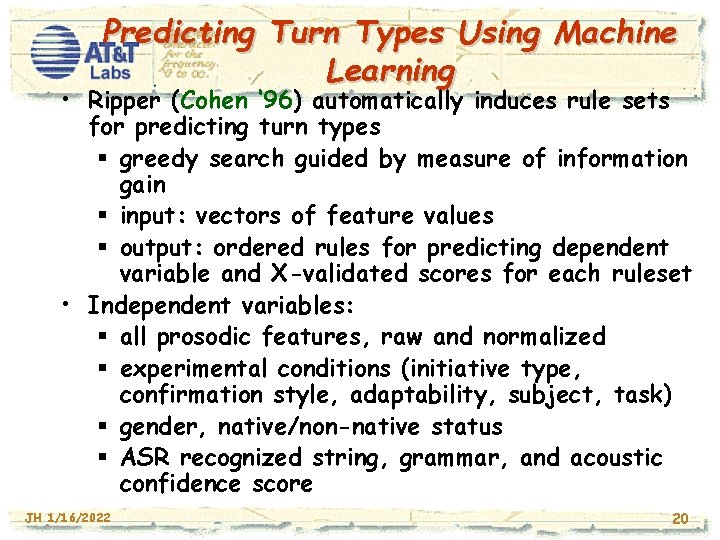
Predicting Turn Types Using Machine Learning • Ripper (Cohen ‘ 96) automatically induces rule sets for predicting turn types § greedy search guided by measure of information gain § input: vectors of feature values § output: ordered rules for predicting dependent variable and X-validated scores for each ruleset • Independent variables: § all prosodic features, raw and normalized § experimental conditions (initiative type, confirmation style, adaptability, subject, task) § gender, native/non-native status § ASR recognized string, grammar, and acoustic confidence score JH 1/16/2022 20
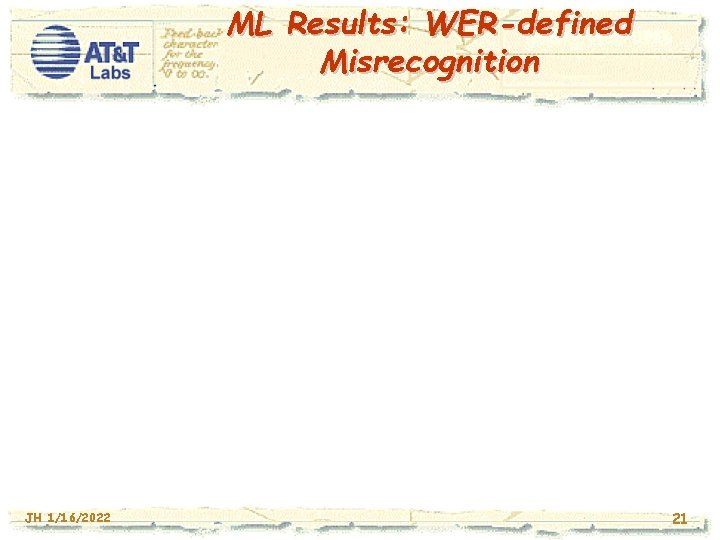
ML Results: WER-defined Misrecognition JH 1/16/2022 21
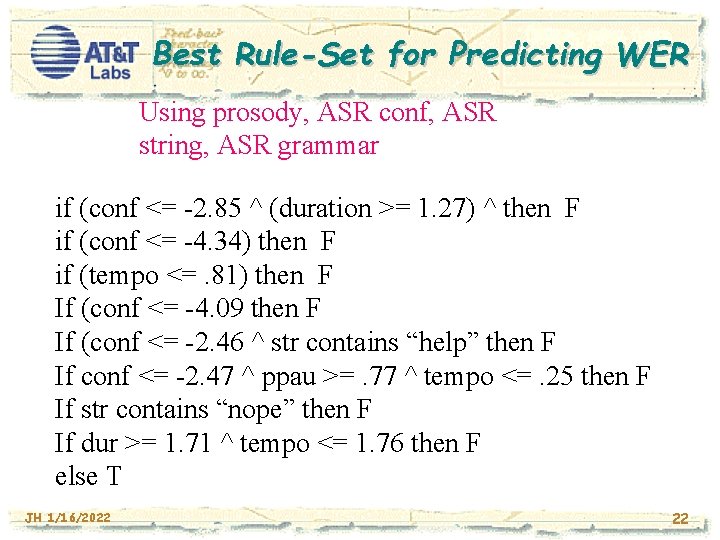
Best Rule-Set for Predicting WER Using prosody, ASR conf, ASR string, ASR grammar if (conf <= -2. 85 ^ (duration >= 1. 27) ^ then F if (conf <= -4. 34) then F if (tempo <=. 81) then F If (conf <= -4. 09 then F If (conf <= -2. 46 ^ str contains “help” then F If conf <= -2. 47 ^ ppau >=. 77 ^ tempo <=. 25 then F If str contains “nope” then F If dur >= 1. 71 ^ tempo <= 1. 76 then F else T JH 1/16/2022 22
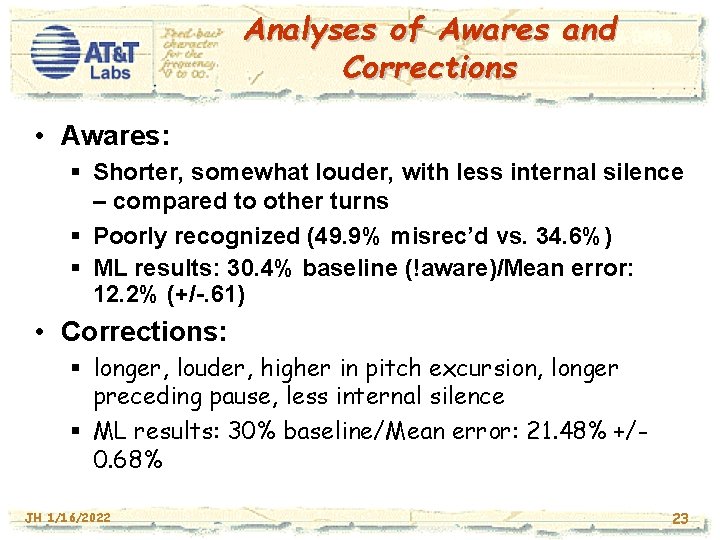
Analyses of Awares and Corrections • Awares: § Shorter, somewhat louder, with less internal silence – compared to other turns § Poorly recognized (49. 9% misrec’d vs. 34. 6%) § ML results: 30. 4% baseline (!aware)/Mean error: 12. 2% (+/-. 61) • Corrections: § longer, louder, higher in pitch excursion, longer preceding pause, less internal silence § ML results: 30% baseline/Mean error: 21. 48% +/0. 68% JH 1/16/2022 23
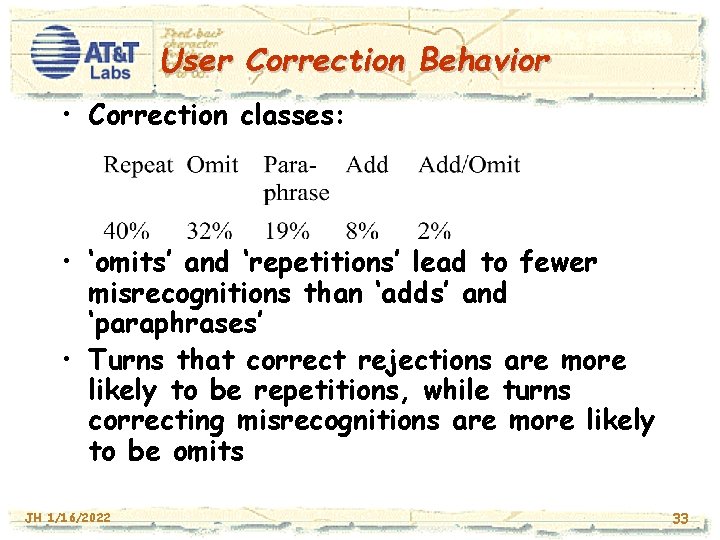
User Correction Behavior • Correction classes: • ‘omits’ and ‘repetitions’ lead to fewer misrecognitions than ‘adds’ and ‘paraphrases’ • Turns that correct rejections are more likely to be repetitions, while turns correcting misrecognitions are more likely to be omits JH 1/16/2022 33
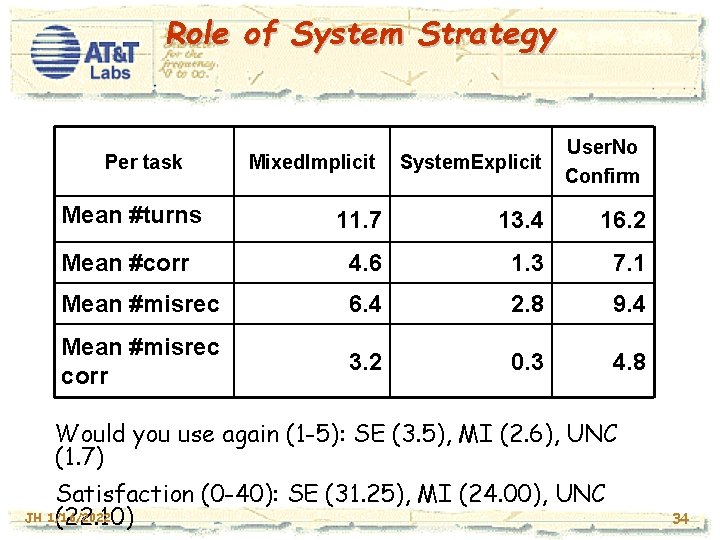
Role of System Strategy Per task Mixed. Implicit System. Explicit User. No Confirm Mean #turns 11. 7 13. 4 16. 2 Mean #corr 4. 6 1. 3 7. 1 Mean #misrec 6. 4 2. 8 9. 4 Mean #misrec corr 3. 2 0. 3 4. 8 Would you use again (1 -5): SE (3. 5), MI (2. 6), UNC (1. 7) Satisfaction (0 -40): SE (31. 25), MI (24. 00), UNC JH 1/16/2022 (22. 10) 34
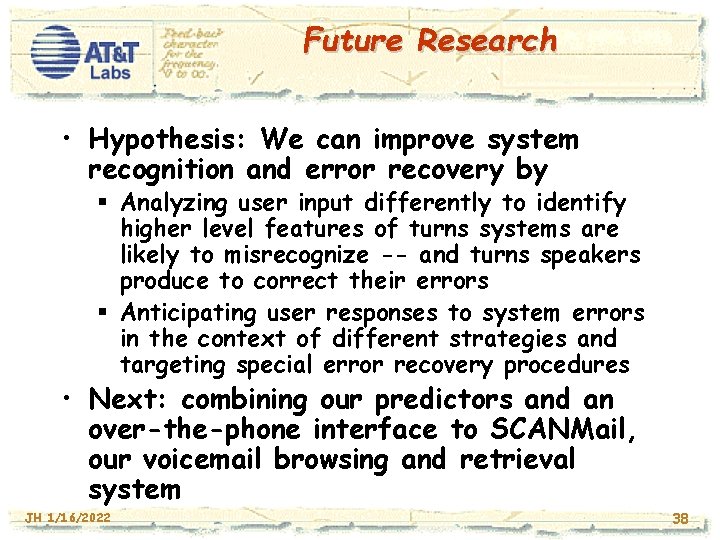
Future Research • Hypothesis: We can improve system recognition and error recovery by § Analyzing user input differently to identify higher level features of turns systems are likely to misrecognize -- and turns speakers produce to correct their errors § Anticipating user responses to system errors in the context of different strategies and targeting special error recovery procedures • Next: combining our predictors and an over-the-phone interface to SCANMail, our voicemail browsing and retrieval system JH 1/16/2022 38
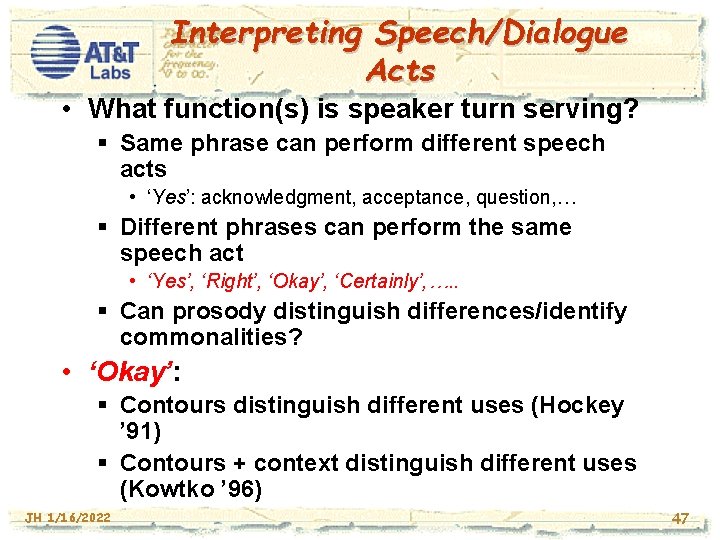
Interpreting Speech/Dialogue Acts • What function(s) is speaker turn serving? § Same phrase can perform different speech acts • ‘Yes’: acknowledgment, acceptance, question, … § Different phrases can perform the same speech act • ‘Yes’, ‘Right’, ‘Okay’, ‘Certainly’, …. . § Can prosody distinguish differences/identify commonalities? • ‘Okay’: § Contours distinguish different uses (Hockey ’ 91) § Contours + context distinguish different uses (Kowtko ’ 96) JH 1/16/2022 47
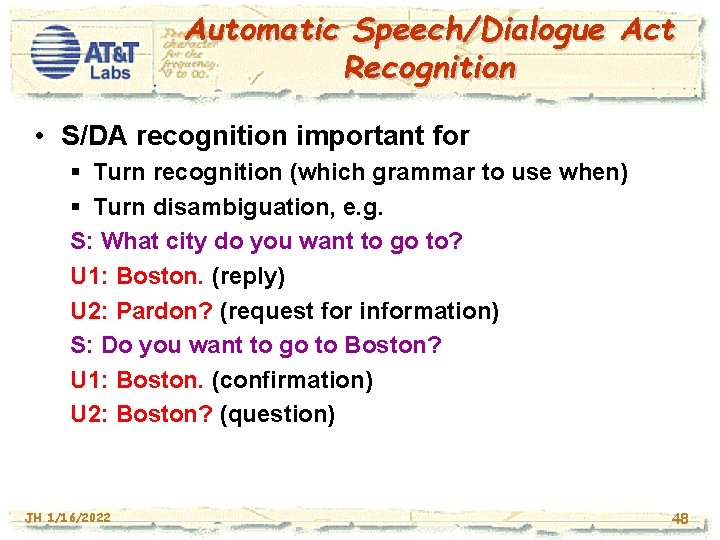
Automatic Speech/Dialogue Act Recognition • S/DA recognition important for § Turn recognition (which grammar to use when) § Turn disambiguation, e. g. S: What city do you want to go to? U 1: Boston. (reply) U 2: Pardon? (request for information) S: Do you want to go to Boston? U 1: Boston. (confirmation) U 2: Boston? (question) JH 1/16/2022 48
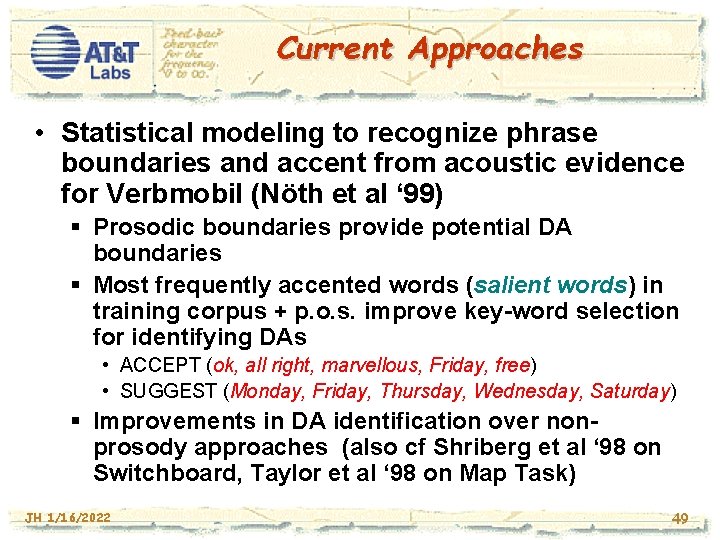
Current Approaches • Statistical modeling to recognize phrase boundaries and accent from acoustic evidence for Verbmobil (Nöth et al ‘ 99) § Prosodic boundaries provide potential DA boundaries § Most frequently accented words (salient words) in training corpus + p. o. s. improve key-word selection for identifying DAs • ACCEPT (ok, all right, marvellous, Friday, free) • SUGGEST (Monday, Friday, Thursday, Wednesday, Saturday) § Improvements in DA identification over nonprosody approaches (also cf Shriberg et al ‘ 98 on Switchboard, Taylor et al ‘ 98 on Map Task) JH 1/16/2022 49
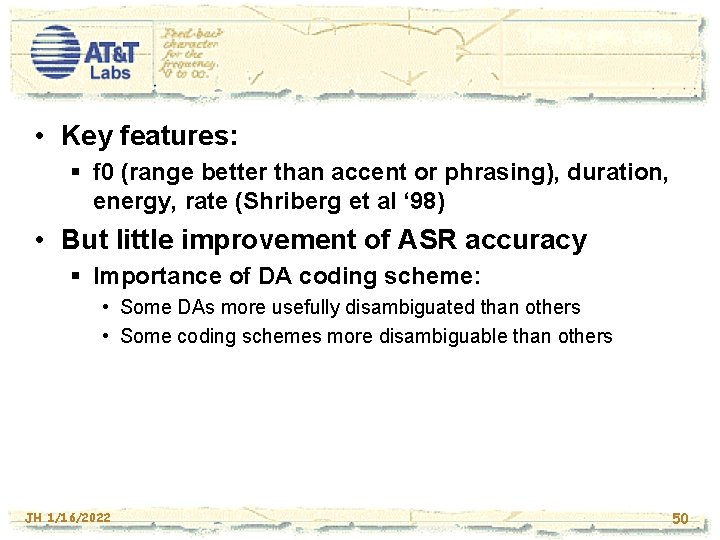
• Key features: § f 0 (range better than accent or phrasing), duration, energy, rate (Shriberg et al ‘ 98) • But little improvement of ASR accuracy § Importance of DA coding scheme: • Some DAs more usefully disambiguated than others • Some coding schemes more disambiguable than others JH 1/16/2022 50
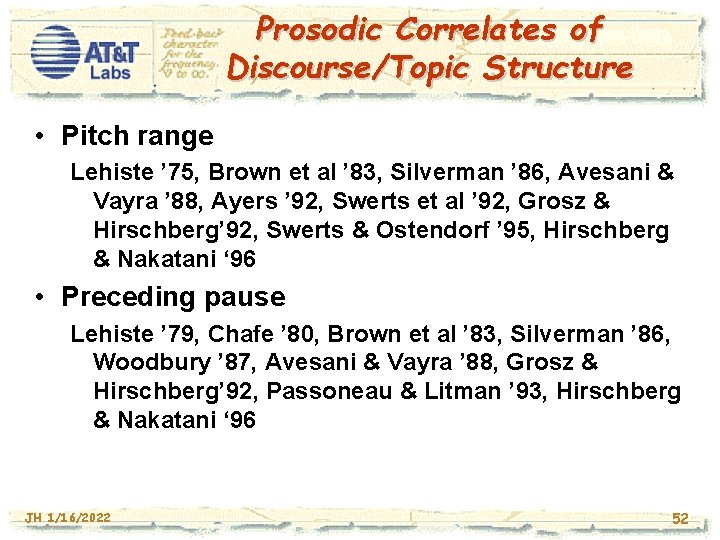
Prosodic Correlates of Discourse/Topic Structure • Pitch range Lehiste ’ 75, Brown et al ’ 83, Silverman ’ 86, Avesani & Vayra ’ 88, Ayers ’ 92, Swerts et al ’ 92, Grosz & Hirschberg’ 92, Swerts & Ostendorf ’ 95, Hirschberg & Nakatani ‘ 96 • Preceding pause Lehiste ’ 79, Chafe ’ 80, Brown et al ’ 83, Silverman ’ 86, Woodbury ’ 87, Avesani & Vayra ’ 88, Grosz & Hirschberg’ 92, Passoneau & Litman ’ 93, Hirschberg & Nakatani ‘ 96 JH 1/16/2022 52
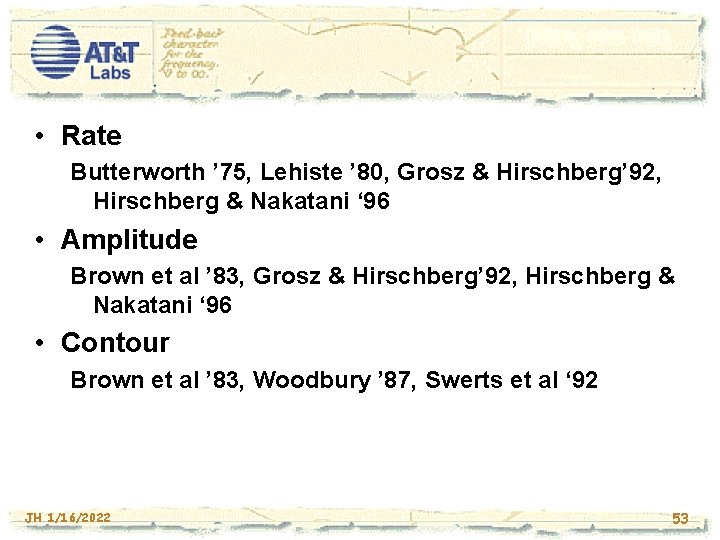
• Rate Butterworth ’ 75, Lehiste ’ 80, Grosz & Hirschberg’ 92, Hirschberg & Nakatani ‘ 96 • Amplitude Brown et al ’ 83, Grosz & Hirschberg’ 92, Hirschberg & Nakatani ‘ 96 • Contour Brown et al ’ 83, Woodbury ’ 87, Swerts et al ‘ 92 JH 1/16/2022 53
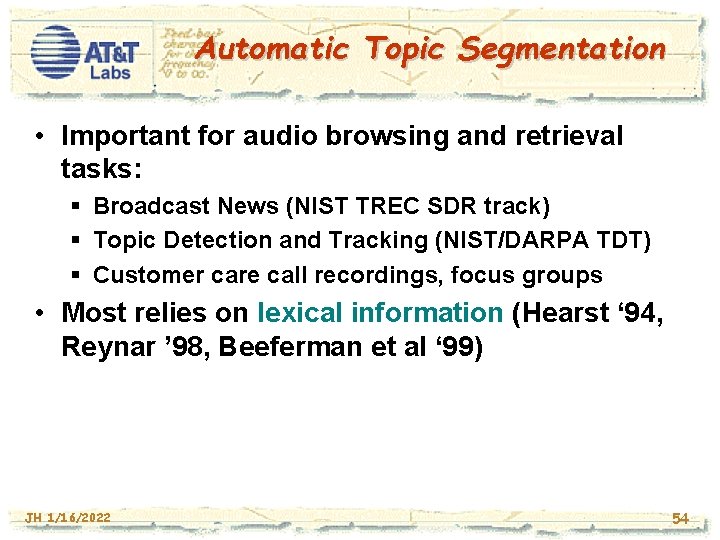
Automatic Topic Segmentation • Important for audio browsing and retrieval tasks: § Broadcast News (NIST TREC SDR track) § Topic Detection and Tracking (NIST/DARPA TDT) § Customer care call recordings, focus groups • Most relies on lexical information (Hearst ‘ 94, Reynar ’ 98, Beeferman et al ‘ 99) JH 1/16/2022 54
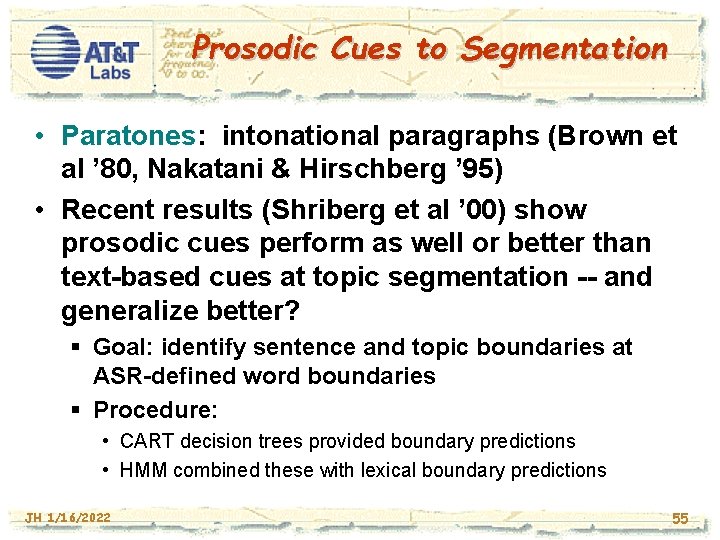
Prosodic Cues to Segmentation • Paratones: intonational paragraphs (Brown et al ’ 80, Nakatani & Hirschberg ’ 95) • Recent results (Shriberg et al ’ 00) show prosodic cues perform as well or better than text-based cues at topic segmentation -- and generalize better? § Goal: identify sentence and topic boundaries at ASR-defined word boundaries § Procedure: • CART decision trees provided boundary predictions • HMM combined these with lexical boundary predictions JH 1/16/2022 55
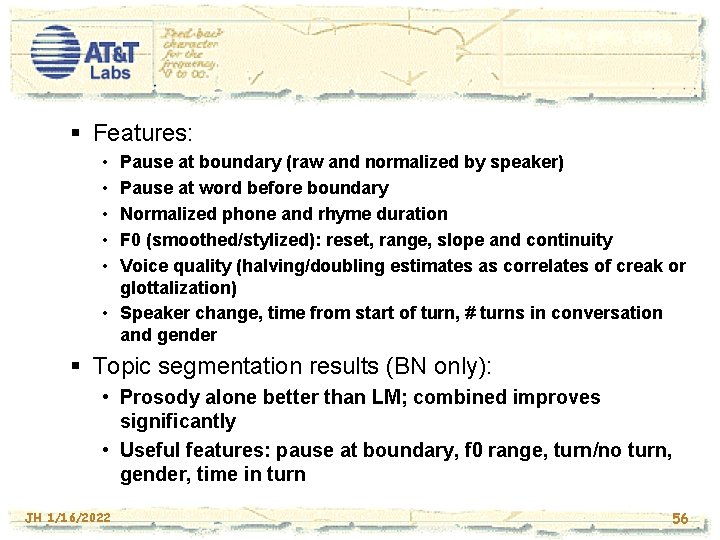
§ Features: • • • Pause at boundary (raw and normalized by speaker) Pause at word before boundary Normalized phone and rhyme duration F 0 (smoothed/stylized): reset, range, slope and continuity Voice quality (halving/doubling estimates as correlates of creak or glottalization) • Speaker change, time from start of turn, # turns in conversation and gender § Topic segmentation results (BN only): • Prosody alone better than LM; combined improves significantly • Useful features: pause at boundary, f 0 range, turn/no turn, gender, time in turn JH 1/16/2022 56
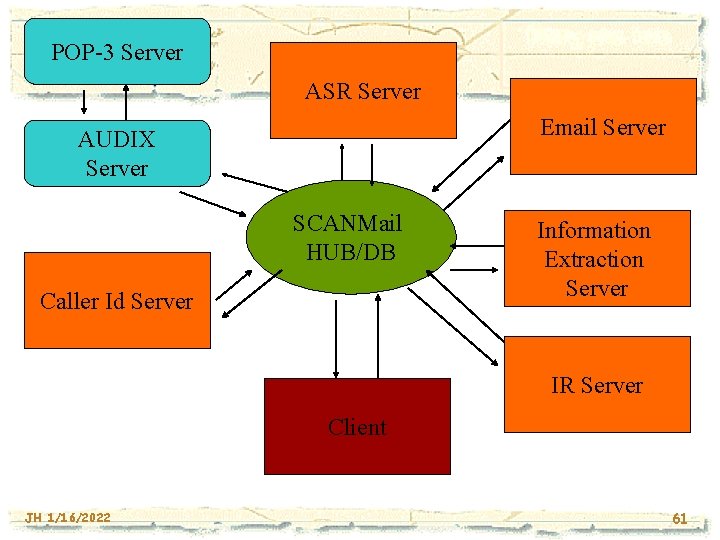
POP-3 Server ASR Server Email Server AUDIX Server SCANMail HUB/DB Caller Id Server Information Extraction Server IR Server Client JH 1/16/2022 61
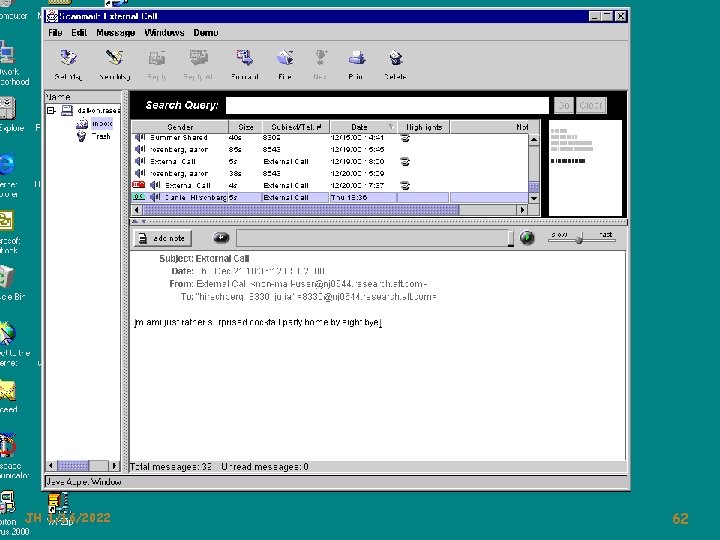
JH 1/16/2022 62
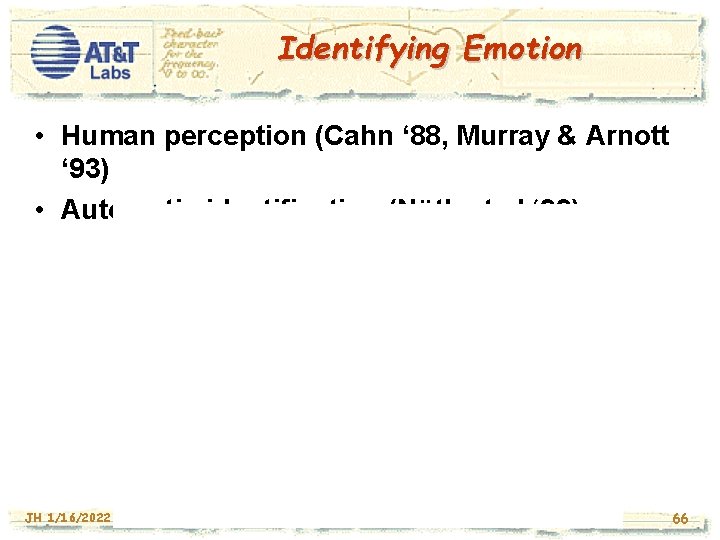
Identifying Emotion • Human perception (Cahn ‘ 88, Murray & Arnott ‘ 93) • Automatic identification (Nöth et al ‘ 99) JH 1/16/2022 66
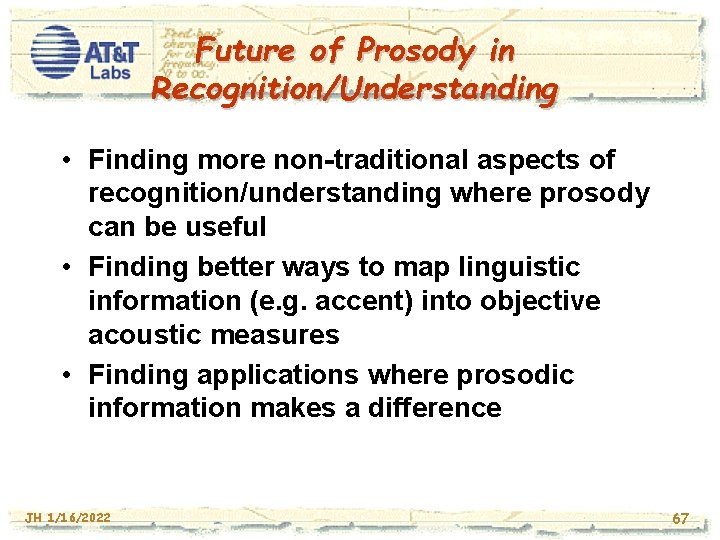
Future of Prosody in Recognition/Understanding • Finding more non-traditional aspects of recognition/understanding where prosody can be useful • Finding better ways to map linguistic information (e. g. accent) into objective acoustic measures • Finding applications where prosodic information makes a difference JH 1/16/2022 67