Maximum Power Targeting for a PEM Fuel Cell
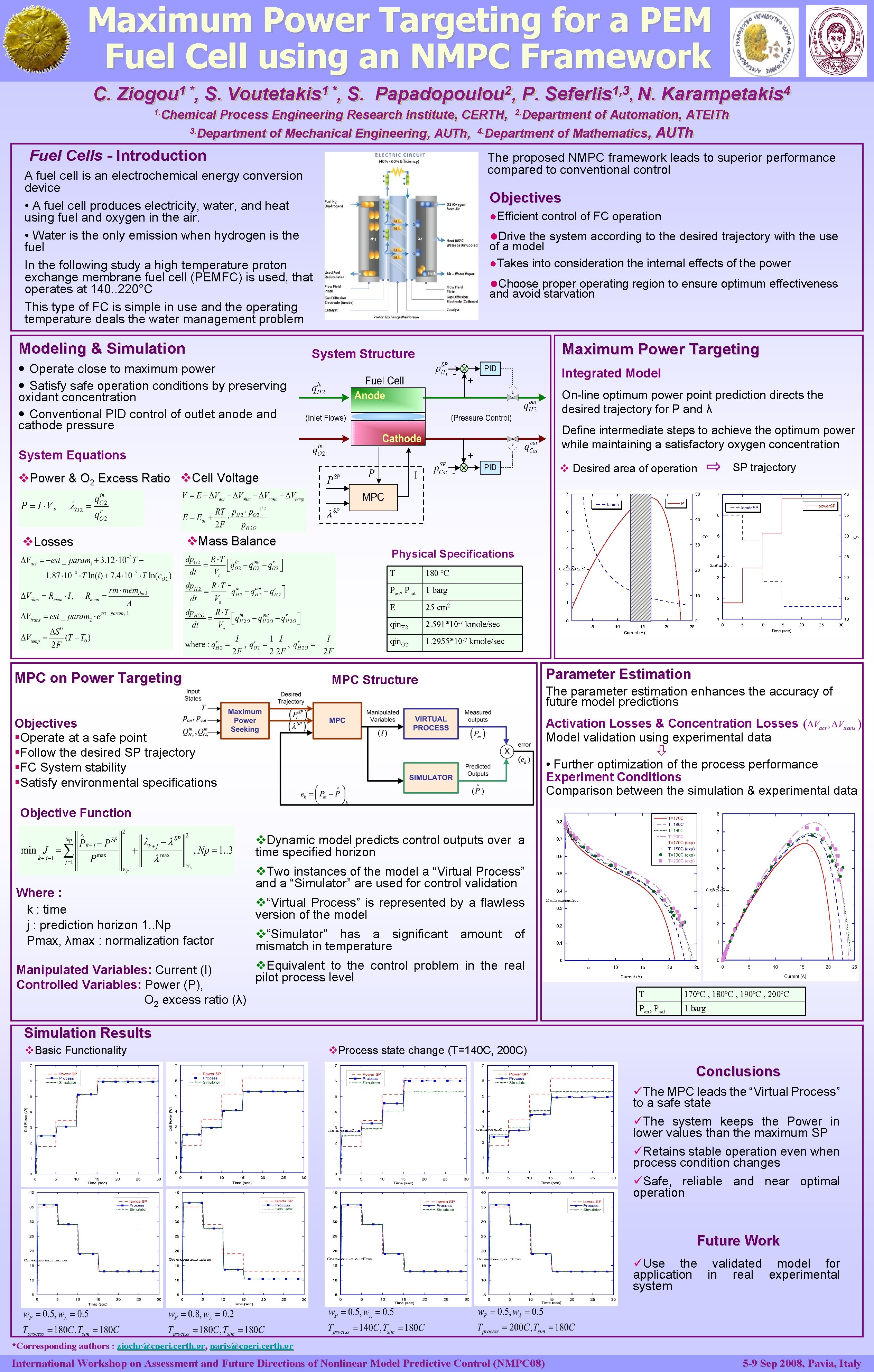
- Slides: 1
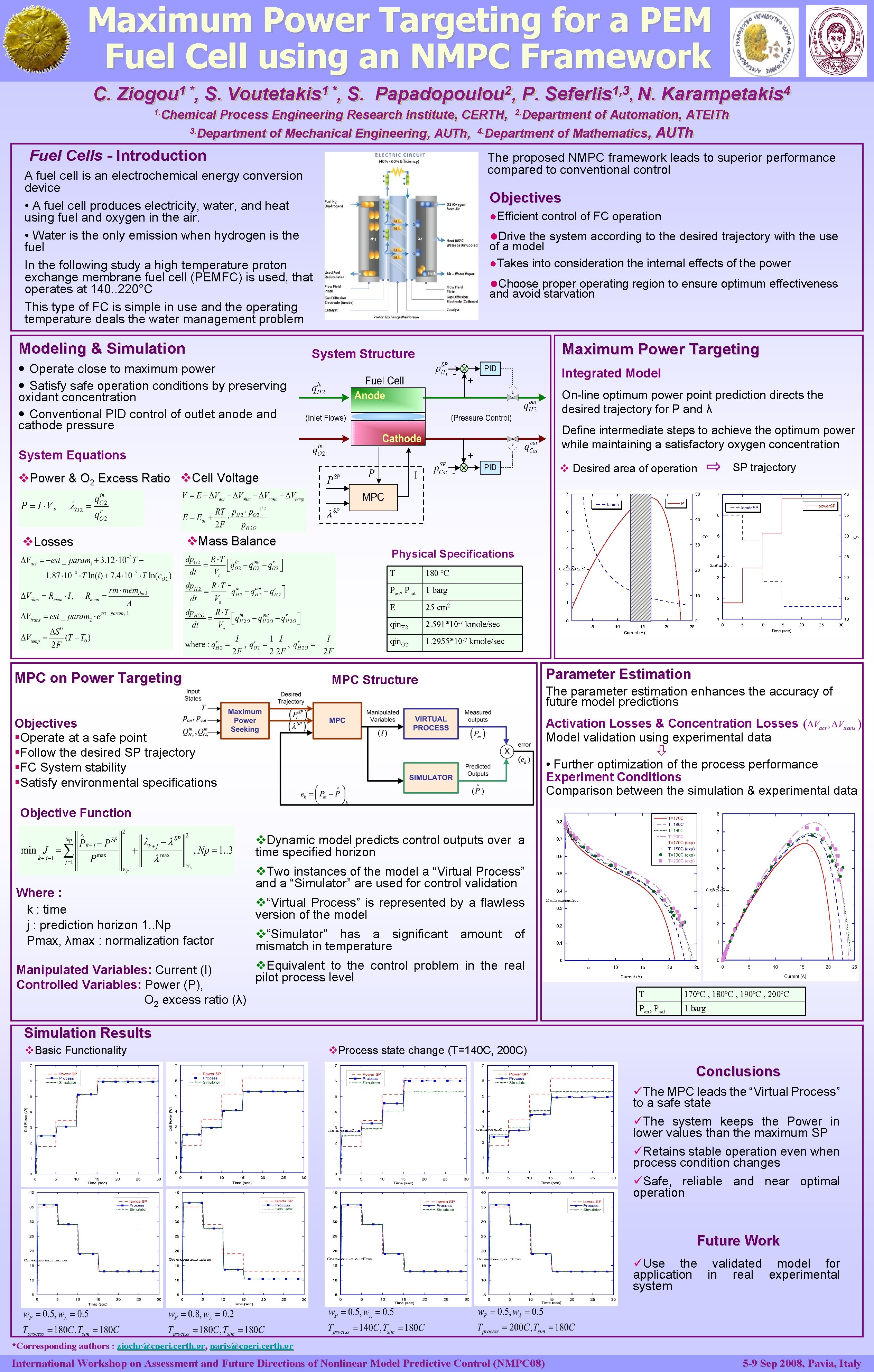
Maximum Power Targeting for a PEM Fuel Cell using an NMPC Framework 1 * 1 * 1 C. Ziogou , S. Voutetakis *, S. 2 1, 3 4 2 1, 3 Papadopoulou , P. Seferlis , N. Karampetakis 4 1. 1. Chemical Process Engineering Research Institute, CERTH, 2. 2. Department of of Automation, ATEITh , AUTh 3. 3. Department Engineering, , AUTh Department of of Mechanical Engineering AUTh, , 4. 4. Department of of Mathematics Fuel Cells - Introduction The proposed NMPC framework leads to superior performance compared to conventional control A fuel cell is an electrochemical energy conversion device • A fuel cell produces electricity, water, and heat using fuel and oxygen in the air. • Water is the only emission when hydrogen is the fuel In the following study a high temperature proton exchange membrane fuel cell (PEMFC) is used, that operates at 140. . 220°C This type of FC is simple in use and the operating temperature deals the water management problem Modeling & Simulation • Operate close to maximum power • Satisfy safe operation conditions by preserving Objectives ●Efficient control of FC operation ●Drive the system according to the desired trajectory with the use of a model ●Takes into consideration the internal effects of the power ●Choose properating region to ensure optimum effectiveness and avoid starvation Maximum Power Targeting System Structure Integrated Model On-line optimum power point prediction directs the desired trajectory for P and λ oxidant concentration • Conventional PID control of outlet anode and cathode pressure Define intermediate steps to achieve the optimum power while maintaining a satisfactory oxygen concentration System Equations v Desired area of operation v. Power & O 2 Excess Ratio v. Cell Voltage v. Losses v. Mass Balance MPC on Power Targeting SP trajectory Physical Specifications T 180 ºC Pan, Pcat 1 barg E 25 cm 2 qin. H 2 2. 591*10 -7 kmole/sec qin. O 2 1. 2955*10 -7 kmole/sec Parameter Estimation MPC Structure The parameter estimation enhances the accuracy of future model predictions Activation Losses & Concentration Losses Model validation using experimental data Objectives §Operate at a safe point §Follow the desired SP trajectory §FC System stability §Satisfy environmental specifications • Further optimization of the process performance Experiment Conditions Comparison between the simulation & experimental data Objective Function v. Dynamic model predicts control outputs over a time specified horizon Where : k : time j : prediction horizon 1. . Np Pmax, λmax : normalization factor v. Two instances of the model a “Virtual Process” and a “Simulator” are used for control validation v“Virtual Process” is represented by a flawless version of the model v“Simulator” has a significant mismatch in temperature amount of v. Equivalent to the control problem in the real Manipulated Variables: Current (I) pilot process level Controlled Variables: Power (P), O 2 excess ratio (λ) T 170ºC , 180ºC , 190ºC , 200ºC Pan, Pcat 1 barg Simulation Results v. Basic Functionality v. Process state change (T=140 C, 200 C) Conclusions üThe MPC leads the “Virtual Process” to a safe state üThe system keeps the Power in lower values than the maximum SP üRetains stable operation even when process condition changes üSafe, reliable and near optimal operation Future Work üUse the validated model for application in real experimental system *Corresponding authors : ziochr@cperi. certh. gr, paris@cperi. certh. gr International Workshop on Assessment and Future Directions of Nonlinear Model Predictive Control (NMPC 08) 5 -9 Sep 2008, Pavia, Italy