Leveraging Software for Predictive Analytics George Gonzlez Director
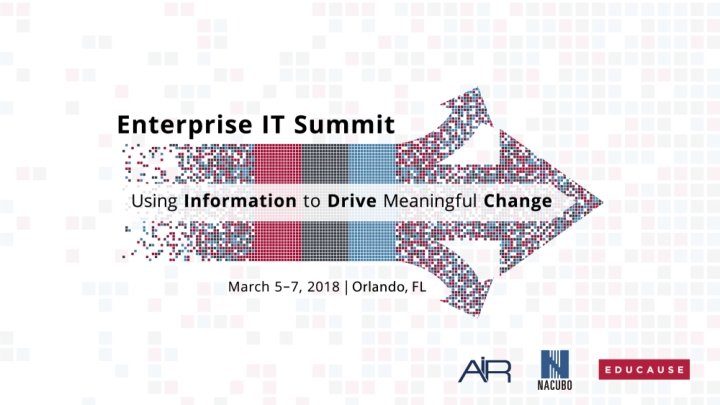
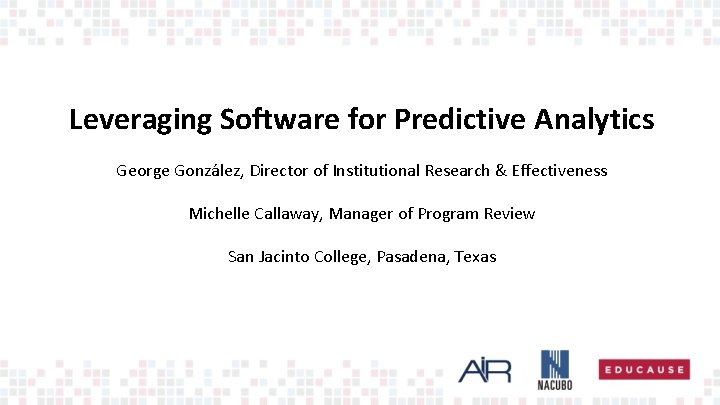
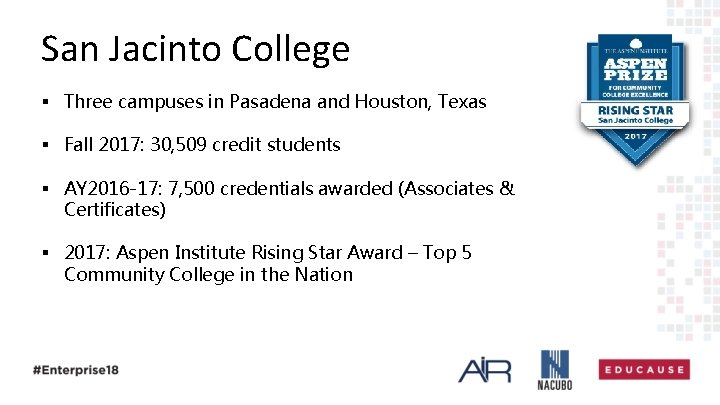
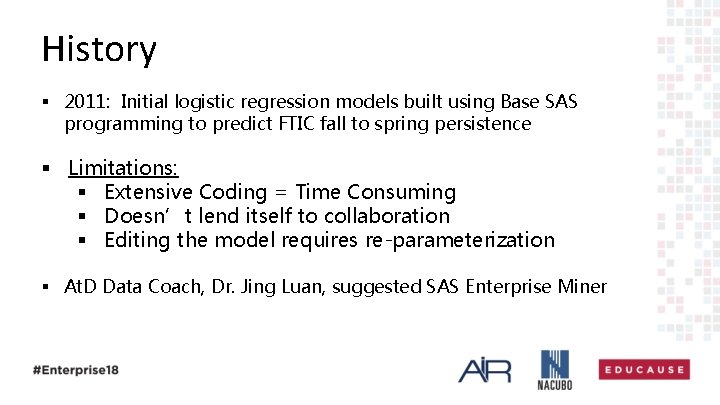
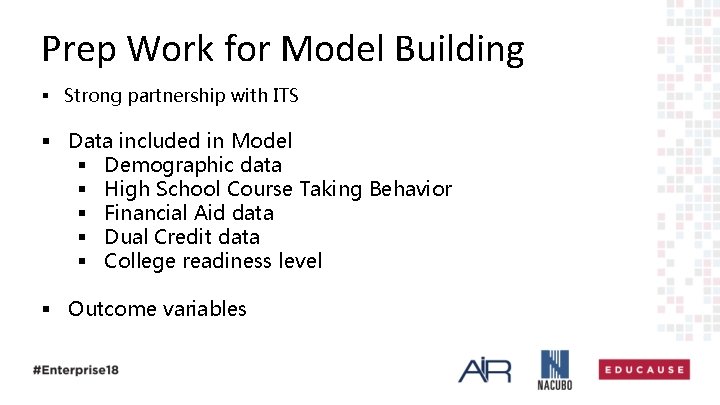
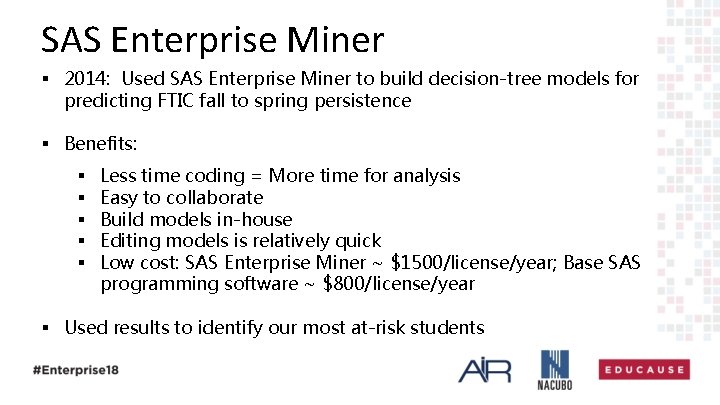
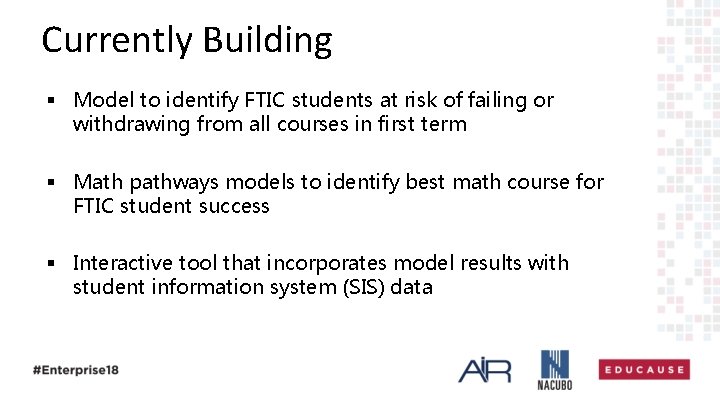
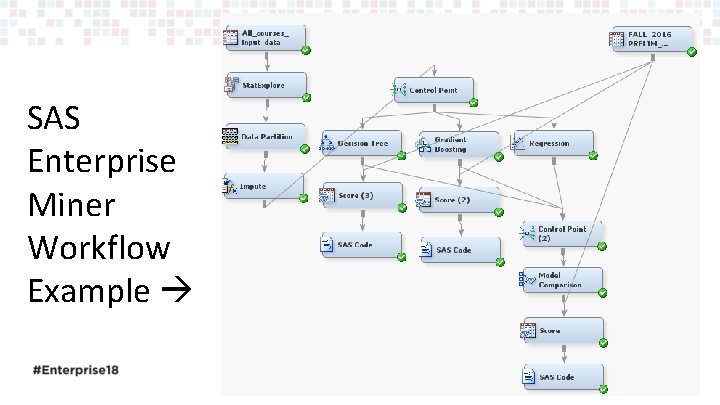
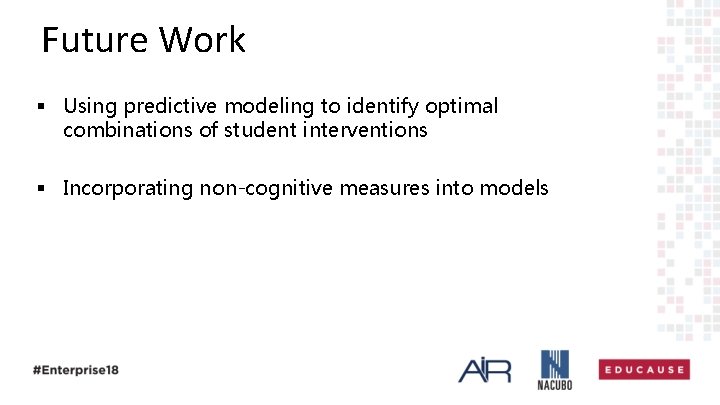
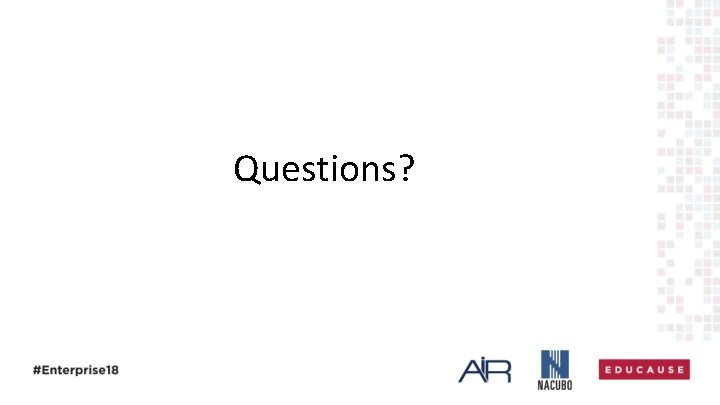
- Slides: 10
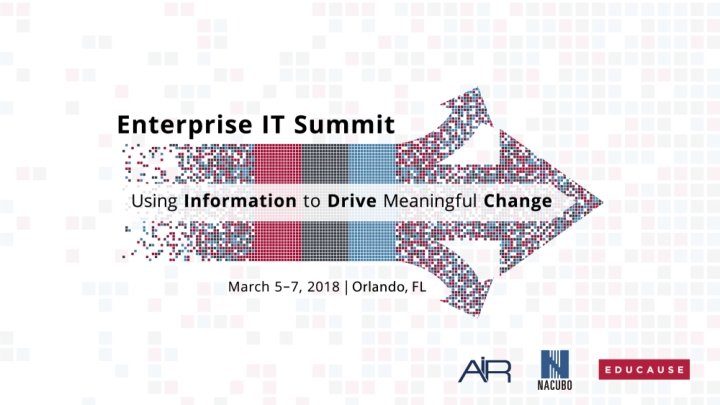
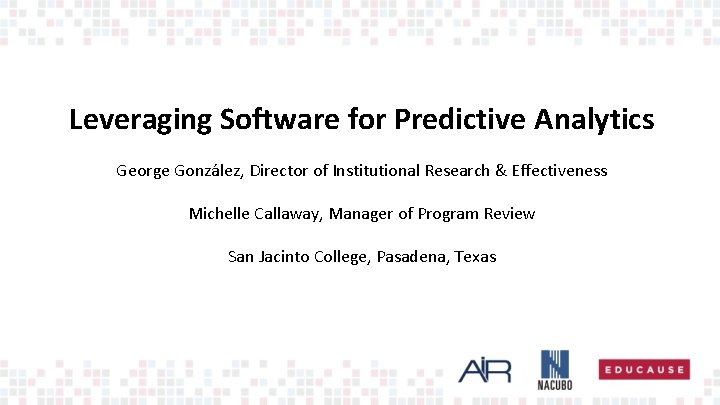
Leveraging Software for Predictive Analytics George González, Director of Institutional Research & Effectiveness Michelle Callaway, Manager of Program Review San Jacinto College, Pasadena, Texas
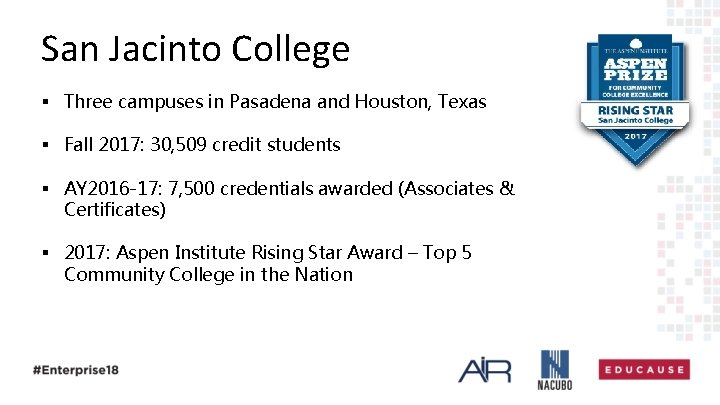
San Jacinto College § Three campuses in Pasadena and Houston, Texas § Fall 2017: 30, 509 credit students § AY 2016 -17: 7, 500 credentials awarded (Associates & Certificates) § 2017: Aspen Institute Rising Star Award – Top 5 Community College in the Nation
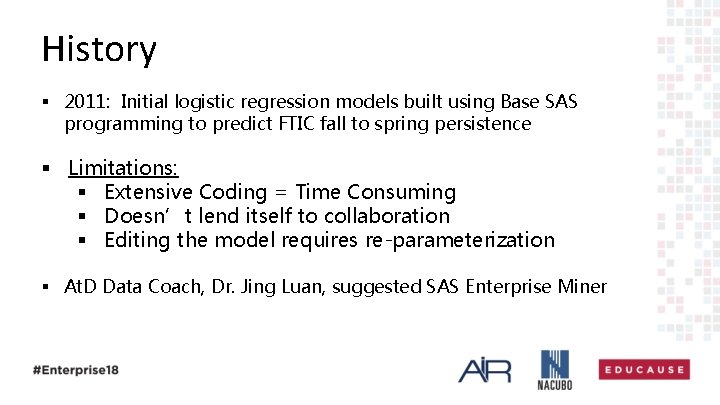
History § 2011: Initial logistic regression models built using Base SAS programming to predict FTIC fall to spring persistence § Limitations: § Extensive Coding = Time Consuming § Doesn’t lend itself to collaboration § Editing the model requires re-parameterization § At. D Data Coach, Dr. Jing Luan, suggested SAS Enterprise Miner
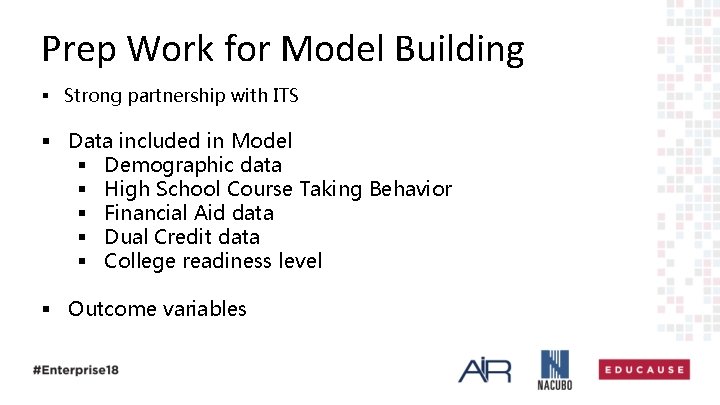
Prep Work for Model Building § Strong partnership with ITS § Data included in Model § Demographic data § High School Course Taking Behavior § Financial Aid data § Dual Credit data § College readiness level § Outcome variables
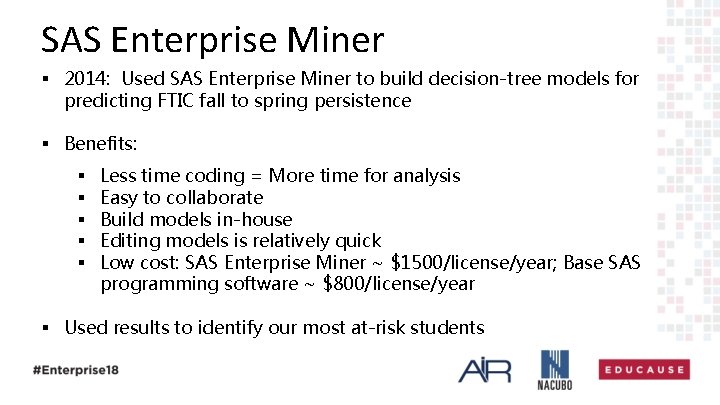
SAS Enterprise Miner § 2014: Used SAS Enterprise Miner to build decision-tree models for predicting FTIC fall to spring persistence § Benefits: § § § Less time coding = More time for analysis Easy to collaborate Build models in-house Editing models is relatively quick Low cost: SAS Enterprise Miner ~ $1500/license/year; Base SAS programming software ~ $800/license/year § Used results to identify our most at-risk students
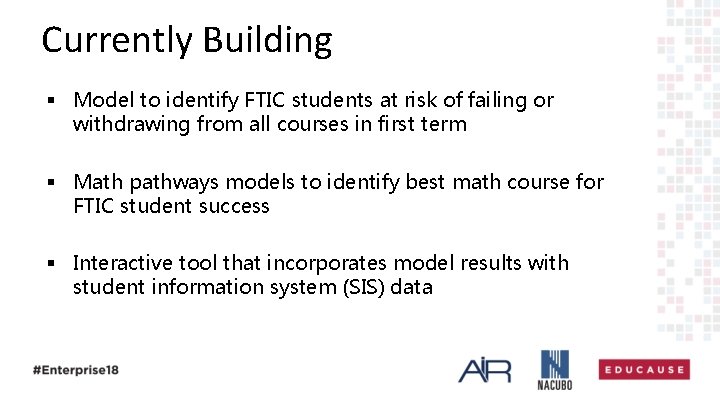
Currently Building § Model to identify FTIC students at risk of failing or withdrawing from all courses in first term § Math pathways models to identify best math course for FTIC student success § Interactive tool that incorporates model results with student information system (SIS) data
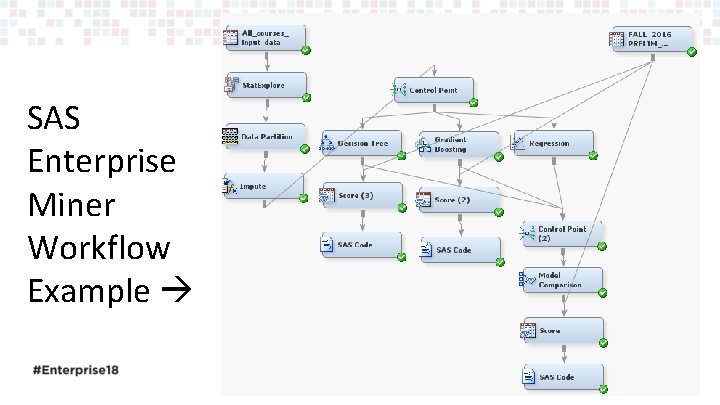
SAS Enterprise Miner Workflow Example
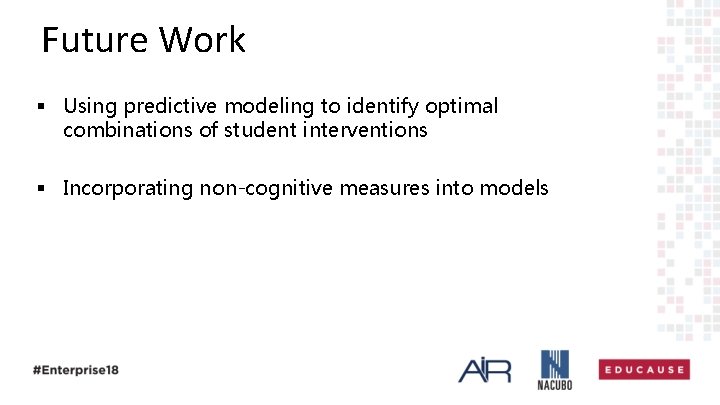
Future Work § Using predictive modeling to identify optimal combinations of student interventions § Incorporating non-cognitive measures into models
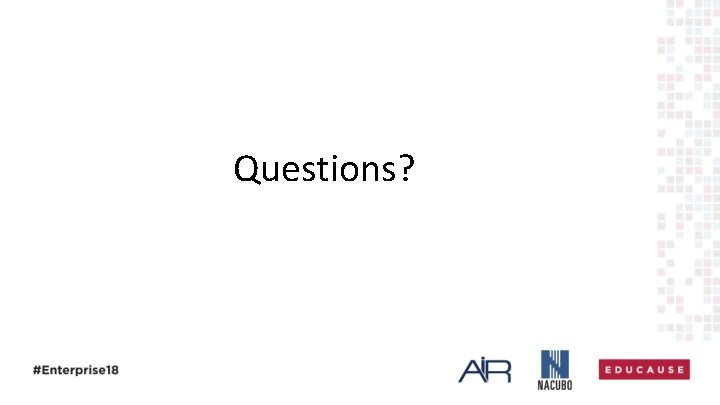
Questions?
Predictive analytics software for higher education
Quotes on big data analytics
Sap predictive analytics demo
Sql server machine learning
Predictive analytics reporting (par) framework
Data analytics lifecycle case study
Align staffing
Knime predictive analytics
Capacity planning and performance management
Challenges of implementing predictive analytics
Predictive prescriptive analytics